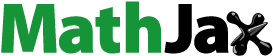
ABSTRACT
This study firstly analyzes the impact of energy intensities and income on CO2 emissions in Russia, applying different estimation methods to the data period from 1990 to 2020. In addition, the study forecasts CO2 emissions considering 2030 targets under different assumptions and assesses the achievability of the set target. The estimation results concluded that the GDP and fossil fuel intensities of GDP have a statistically positive impact on CO2 emissions. Also, we found that the forecasted value for 2030, for the business-as-usual case, is 1750 MtCO2, with 95% confidence interval values of 1703 MtCO2 and 1796 MtCO2. This result shows that Russia needs to undergo substantial policy interventions to achieve its targets to reduce CO2 emissions. As the fifth biggest emitter, Russia missing its emissions targets will have undesirable implications for the rest of the world. Based on the projection results, the paper discusses some potential policy interventions.
1. Introduction
Increases in greenhouse gas (GHG) concentrations have generated a steady rise in global temperatures, especially in the last four decades. Each of these decades has been warmer than any preceding one since 1850 (IPCC, Citation2021). In absolute terms, in 2021, energy-related global CO2 emissions relative to 2020 increased by over 2 billion tonnes, the largest in history. In energy-related CO2 emissions, primary growth occurred due to the increased consumption of coal for power generation (IEA, Citation2022a). As a result of an increase in CO2 emissions, the average surface temperature has risen globally, and the global upper ocean (0–700 m) has warmed since the 1970s. Oxygen levels have dropped in many upper ocean regions, and human-caused CO2 emissions have been the main driver of ocean acidification (IPCC, Citation2021). According to Climate Watch data, Russia is among the top five emitters of total GHGs and CO2 emissions (Climate Watch, Citation2022b).
As a result of considerable levels of emissions in 2020, the average temperature in Russia was estimated to have been 4 °C higher than the average temperature from 1850 to 1900. This period (1850–1900) is often used as a pre-industrial baseline for global temperature targets (Berkeley Earth, Citation2021). This extreme heat led to permafrost thawing, which could generate further threats like infrastructure collapse, coastline erosion, and methane escaping from the previously frozen ground (Shapovalova, Citation2020). In the subsequent comparison for the Russian case, in 2021, the temperature was 1.5 °C higher than the 1951–1980 average (Berkeley Earth, Citation2022). Without deep reductions in CO2 and other greenhouse gas emissions, the average temperature seems to keep increasing. Meinshausen et al. (Citation2022) estimate that Russian per capita emissions for 2030 will be 21% higher relative to 2019 levels. The extreme heat generated major wildfires in Russia for the third successive year. Federal Forestry Agency of Russia reports that during the summer in Yakutia number of fires reached 2295, burning 8.9 million hectares of forest (WMO, Citation2022).
Additionally, Russia has one more challenge to cope with: a decrease in energy exports and economic growth. The Paris Agreement parties pledged emission reduction targets with their Nationally Determined Contributions (NDC). The fulfillment of all these NDCs will lead to a 25% reduction in Russian energy exports by 2030, which will bring about a striking decline in its economic growth rates (IPCC, Citation2022). Also, Azevedo, Sartori, and Campos (Citation2018) find that economic growth is the main driving force for the growth of CO2 emissions in the Russian case.
Furthermore, especially in a relatively shorter period of time, like 20 years, the global warming potential (GWP) of methane (CH4) is about 80 times bigger than CO2. Because of this reason, initiatives like Global Methane Pledge intended to develop a collective effort to reduce global methane emissions by at least 30 percent between 2020 and 2030. Fortunately, this methane reduction goal can potentially eliminate global warming by over 0.2 °C by 2050 (Global Methane Pledge, Citation2022). CO2 and CH4 comprise 96 % of total GHG emissions in the Russian case (Climate Watch, Citation2022a). Supporters of the Global Methane Pledge are from 121 countries. However, together with India and China, Russia does not join this commitment (EC, Citation2022). If we look at the sectoral breakdown of methane emissions in these countries, we can find the reason laying behind that kind of indifference: In China and India, energy and agriculture are the primary sectors emitting methane. In the Russian case, the energy sector emits 75 % of all methane (IEA, Citation2022b). Also, to relate national commitments with global gains, another determinant must be considered: aggregate CO2-equivalent emissions create ambiguity in global temperature outcomes. A Group of scientists stressed the need to individually specify the contributions of all gases to global temperature in future targets (Allen et al., Citation2022).
Being one of the top emitters, Russia’s emission reduction target is to lower GHG emissions by at least 30 % below 1990 levels by 2030. Its long-term climate strategy includes net-zero GHG emissions for 2060. This strategy targets an 80% reduction in GHG emissions by 2050 relative to 1990 levels (CAT, Citation2022). On the one hand, The Energy Strategy to 2035, which was adopted in 2021, promotes the extraction, consumption, and export of fossil fuels. This strategy may pretty well contradict the goals mentioned above. On the other hand, the IEA roadmap for the global energy sector to achieve net-zero emissions by 2050 suggests that no new investments should take place in coal, gas, and oil projects from 2021 on (IEA, Citation2022a). Previous targets relied on two essential components: firstly, making the Russian industry 40 percent more energy efficient by 2020 and, secondly, utilizing the potential of Russian forests to absorb carbon dioxide (Pao, Yu, & Yang, Citation2011).
As IPCC (Citation2022) suggests, limiting warming to around 1.5 C requires global greenhouse gas emissions to be reduced by 43 % by 2030. When carbon dioxide emissions reach net zero, only then will the global temperature stabilize (Intergovernmental Panel on Climate Change (IPCC), Citation2022 Citation2021). Even though the planet needs better commitment from one of the biggest emitters, as stated earlier, the net-zero target for Russia is 2060. Meinshausen et al. (Citation2022) find that current NDC pledges are far from limiting warming to 1.5 C. Their study implies that countries are supposed to bring their net-zero dates forward and adopt net negative targets. Our study intends to shed additional light on this argument for the Russian case. It is noteworthy that the energy consumption, economic growth, and CO2 emissions relationship seems quite intuitive. In this manner, the novelty of the present study on this topic is two-fold. Firstly, it provides an extension that earlier studies need. This study’s sound estimation results can be considered a contribution to existing literature. That is, by adopting a state-of-the-art methodological approach and model specification, the current study manages to come up with concrete estimation results. Additionally, it challenges the decoupling path for the Russian case, which was previously found between economic output and carbon emissions. In a nutshell, the novelty of this study consists of two primary strands: contribution to existing literature and providing more conclusive findings for the Russian case.
As of 24 February 2022, when the unprovoked Russian invasion of Ukraine started, the first concern was about the global food and hunger crisis. This outcome has a solid justification: Russia and Ukraine produce a third of the world’s wheat and barley exports. Also, these two countries provide more than 70 % of sunflower oil exports (Europe & Lancet Regional Health – Europe, Citation2022). Furthermore, depending on the length of the war, emissions from tanks, military aircraft, and trucks could amount to “as much as a small or medium-sized country in an entire year” (WSJ, Citation2022). Also, there is scientific evidence that emissions from fires in oil plants can have long-distance impacts. In 1991, Kuwait’s oil fires burned more than 4.5 million barrels per day. Among many other polluting impacts, these fires were responsible for 2% of global CO2 emissions (Hobbs and Radke, Citation1992). In this manner, strikes targeting fuel depots and refineries prompt large fires, generating the additional release of carbon dioxide and methane.
Last but not least, western sanctions can push Russia to consume more fossil fuels domestically. This kind of shift in the energy mix landscape will negatively impact the Russian case’s CO2 emissions targets and commitments. Russia is a big producer of minerals that are vital for clean energy technologies. For example, 20% of the supply of Class 1 nickel, which is needed for batteries, comes from Russia. The country ranks globally second place for cobalt production and fourth place in graphite production. Increasing Russia’s international isolation (Kim, Citation2022) might have negative implications for global green energy transitions.
Having mentioned the crucial role of reducing the Russian GHG emissions level in the overall global climatic-environmental umbrella, firstly, it necessitates exploring the factors shaping the evolution of these emissions; secondly, their potential future trajectory; and lastly, assessing the attainability of the set targets. Hence, aiming to address the issues mentioned above, our study contributes to the existing literature from different aspects. Firstly, we develop a framework that relates CO2 emissions to energy consumption indicators. This framework allows making scenario simulations for CO2 emissions and assessing the achievability of certain targets, such as net-zero CO2 emissions targets, under certain assumptions for energy consumption indicators. Secondly, it models the impacts of CO2 emissions and reveals the factors driving them using the most updated data. Thirdly, in modeling exercises, it uses techniques with statistical superiority, such as machine learning multi-search algorithms. The used Autometrics machine learning algorithm enables the detection of historical exogenous interventions whose omission might result in biased parameter estimations. In addition, this algorithm allows testing a battery of diagnostic tests for model quality and parameter (non)constancy. Other merits of the used technique are provided in the Methodology section. Since Russia is committed to certain targets by 2030 and later on, forecasting CO2 emissions will have important policy implications. Therefore next, utilizing the estimated model, the study makes forecasts considering different potential scenarios. Then, based on the forecasts, it assesses if the set targets are attainable. In addition, we also make some alternative policy scenario simulations that might reach the set 2030 targets. Lastly, the paper discusses potential policy interferences in terms of reaching the set targets. Kilinc-Ata and Dolmatov (Citation2022a) suggest that in terms of providing favorable market accessibility and development environment, existing renewable energy policies are insufficient in Russia. Bringing this policy suggestion together with the findings of our study, we intend to shed additional light on the importance of CO2 emissions targets.
Jacobson et al. (Citation2022) estimate that in the case of a 100 % clean energy transition payback time of the capital cost will be 5.5 years. Way, Ives, Mealy, and Farmer (Citation2022) find that a fast energy transition will provide net savings. Kilinc-Ata and Dolmatov (Citation2022b) suggest that Russia has hurdles to commercializing renewable energy consumption. IMF (Citation2022) opines that by getting closer to 2030, fossil fuel exporting countries will face more considerable challenges, and a chance for a relatively smooth energy transition will be missed. At this time, estimating CO2 emissions and providing striking forecast results will provide additional scientific evidence for the urgency of emission reduction targets. Seven years left to reach 2030, and chances of averting catastrophic climate disruptions are getting lower. Steel, DesRoches, and Mintz-Woo (Citation2022) consider that the risk of climate collapse demands careful scientific investigation. In light of all these recent studies, this study provides additional scientific evidence further to clarify the importance of the ongoing climate emergency.
The remaining sections of the paper are structured as follows: Section 2 provides a review of the related literature. Section 3 derives the model specification, and section 4 presents the econometric methodology. Data used for empirical estimations are presented in section 5. Empirical estimations results are delivered in section 6. Section 7 describes assumptions for forecasting exercises, and section 8 provides the forecasting results. Discussion of the empirical findings and forecasting are given in section 9; section 10 concludes the paper and shares the policy implications of the paper.
2. Literature review
This section’s primary goal is to look at the studies investigating the CO2 emissions impacts in Russia. However, there are a few time-series studies in the case of Russia; therefore, we also examine the research conducted for the country group, including Russia. details the reviewed literature.
Table 1. A summary of reviewed empirical studies.
Table 2. Unit root test results.
Table 3. Cointegration tests’ results.
Table 4. Long-run estimation results.
Pao et al. (Citation2011) examined CO2 emissions-income relationship employing VECM in the data period from 1990 to 2007. They applied cointegration techniques with 17 observations and concluded that the EKC hypothesis does not hold for the Russian case. The study started estimation with the quadratic functional form, but then the squared term was excluded due to the multicollinearity. The estimation results revealed Russia’s monotonically decreasing relationship between income and CO2 emissions. In addition, Yang et al. (Citation2017) investigated CO2 emissions-income link using time series data. The study results indicated that the EKC hypothesis holds in the case of Russia, which is surprising given that this finding is generally true for developed/advanced economies.
On the other hand, some studies analyzed the CO2 emissions-income relationship using mixed panel and time series analysis. To avoid readers from misinterpreting the emissions-income link in Russia, solely time-series results of these studies will be interpreted. Shuai et al. (Citation2017) and Chisti and Sinha (Citation2022) used both panel and time series analysis to evaluate the relationship between income and CO2 emissions in the case of a country group (including Russia). Shuai et al. (Citation2017) found the existence of an inverted U-shaped relationship, while Chisti and Sinha (Citation2022) found a U-shaped link for Russia. Furthermore, Danish (2019) revealed a U-shaped relationship between income and CO2 emissions by employing the AMG method in Russia’s case. Also, Kilinc-Ata and Likhachev (Citation2022) found a U-shaped relationship for Russia using the ARDL method to the data period of 1990-to 2020.
Moreover, there are several panel studies (including Russia), such as Tamazian and Rao (Citation2010), Apergis and Payne (Citation2010), and Al-Mulali et al. (Citation2016) reached an inverted U-shaped curve. In contrast, Narayan et al. (Citation2016) revealed a U-shaped curve, and Perez-Suarez and Lopez-Menendez (Citation2015) found no specific pattern. In addition, Zakarya et al. (Citation2015), Mitic et al. (Citation2017), and Ito (Citation2017) concluded a monotonically increasing CO2 emissions-income link. Additionally, Brizga et al. (Citation2013) found an L-shaped link between income and CO2 emissions using data spanning from 1990 to 2010. Hasanov et al. (Citation2021) investigated the impact of GDP on CO2 emissions utilizing the CS-ARDL technique to data period of 1990–to 2017 for BRICS countries. They found a positive and statistically significant impact of GDP on CO2 emissions.
In addition, there are several studies in the literature that take into account the effects of energy intensity on carbon emissions, which is one of the focuses of our study. However, there are very few studies (mainly panel data) investigating the impact of energy intensity on carbon emissions in the case of Russia. Among these studies, Dong et al. (Citation2019a) examined the impact of energy intensity, renewable energy consumption, non-renewable energy, economic growth, and population size on CO2 emission in the case of 128 countries utilizing the panel AMG and CCEMG techniques to the data spanning from 1992 to 2014. The AMG and the CCEMG revealed that a 1% rise in energy intensity leads to a 0.53% increase in CO2 emissions and a 1% rise in energy intensity leads to a 0.92% increase in CO2 emissions for Europe & Eurasia countries (including Russia), respectively. It implies that energy intensity has a positive impact on CO2 emissions.
Additionally, Abban, Wu, & Mensah, Citation2020) concluded a positive impact of energy intensity on CO2 emissions for high-income economies (including Russia) by applying the AMG panel data estimation method to the data during 1995–2015. Inglesi-Lotz (Citation2017) employed the LMDI decomposition test to evaluate the energy intensity-carbon emissions nexus in the case of BRICS countries for the data period of 1990–2014. The estimation results showed that energy intensities raised CO2 emissions for Russia. In addition, Brizga et al. (Citation2013) revealed that there is a positive and significant effect of energy intensity on CO2 emissions for 15 former Soviet countries (including Russia).
As can be seen from the studies mentioned above, no time-series research has been conducted in the case of Russia to model CO2 emissions, especially using machine learning algorithms in addition to conventional cointegration techniques, as well as forecasting emissions considering 2030 targets. In this case, time-series research should be conducted, considering the aforementioned limitations. To fill the gap in the related literature, the current article explores determinants of CO2 emissions utilizing several time-series cointegration approaches and makes projections for CO2 emissions considering 2030 targets.
3. Functional model specification
Numerous papers have attempted to estimate a relationship between environmental indicators and their drivers empirically since the pioneering work by Grossman and Krueger (Citation1991) and Shafik and Bandyopadhyay (Citation1992), which was based on the concept of an environmental Kuznets curve (EKC). In the EKC literature, an environmental indicator, such as CO2 emissions, CH4 emissions, etc., is modeled as a function of income proxy. Although there is not a unique, straightforward, mathematically derived, theoretically, well-grounded functional specification comprising all the relevant explanatory variables, different variables are used in applied work as divers of environmental degradation. These explanatory variables are mainly included in specifications based on conceptual intuition rather than theoretical derivation. The well-known EKC framework is only a special case of a broader pollution income relationship. Even there are studies (see Hendry, Citation2020) questioning the use of income as a driver of CO2 emissions and papers inquiring about the reliability of findings by conventional polynomial EKC approaches (Wagner, Citation2008). For the discussion of the rationale behind of EKC framework and different approaches for its theoretical justification, see Lieb (Citation2003), Kijima, Nishide, and Ohyama (Citation2010), and Mikayilov et al. (Citation2018), inter alia. In the empirical studies, the so-called IPAT (Environmental Impacts (I), Population (P), Affluence (A), and Technology (T)) identity proposed by Enrlich and Holdren (Citation1971) and its another formulation called Kaya identity suggested by Kaya (Citation1990) are mainly used as an initial point to start with and derive different functional specifications, depending on the purpose of the research. For example, the widely used STIRPAT (Stochastic Impacts by Regression on Population, Affluence, and Technology) framework (Dietz & Rosa, Citation1994, Citation1997) is a modified, econometrically estimable version of an IPAT identity. Since we are modeling the CO2 emissions and targeting to make forecasts based on fuel-type energy scenarios, we used Kaya identity to derive our functional specification. It also enables avoiding problems such as using energy consumption as a driver of calculated CO2 emissions based on consumption figures (Jaforullah & King, Citation2017).
Moreover, there is a need for a functional specification for assessing the possible future trajectories of CO2 emissions and other GHGs, making different assumptions for the energy consumption inputs. This specification would allow assessing achievability of some targets for CO2 emissions, such as net-zero emissions targets set by certain years. Considering that having energy consumption variables as right-hand side variables might result in some issues, as Jaforullah and King (Citation2017) discussed. In addition, the estimated coefficients need to have economic interpretations. This section, hence, derives such a specification as follows.
First, building on Hendry (Citation2020) and targeting the coefficients to have economic interpretation, we derived the functional specification which links the consumption of fuel types contributing to CO2 emissions. The rationale for having fuel demand proxies in the specification first relies on the theoretical phenomenon. As is well known, energy consumption drives CO2 emissions. In addition, we are proposing a framework that allows an assessment of different fuel type scenarios on a CO2 emissions trajectory. This section develops the related derivations. The well-known Kaya-type identity (Kaya, Citation1990) can be expressed as follow:
Where is energy intensity of GDP,
is the carbon intensity of energy.
We can assume that (Based on the historical data for Russia
Then (1) becomes:
Considering that (2) can be written as:
Where are oil consumption, natural gas consumption, and coal consumption, respectively. Accordingly,
are oil, natural gas, and coal intensities of GDP.
Taking the logarithm of both sides of equation (3), we get:
Applying Taylor expansion to the second term of the right-hand side of equation (4) and dropping higher-order and cross-product terms, it can be expressed as an estimable relationship as follow:
To avoid having variables in different units and for the sake of better econometric relationships, proxying level variables with their logarithmic counterpart’s equation (5) can be written as below:
Specifications (5) and (6) give room for playing with oil, natural gas, and coal consumption scenarios to see their impact on CO2 emissions. In addition, the population variable could be dropped from equation (1), which gives another alternative specification with overall CO2 emissions and GDP numbers instead of per capita values. Furthermore, in equations (5) and (6), total energy intensity could be used depending on the purpose of the research question. Moreover, energy’s carbon intensity could also be kept in the relationships as a driver of CO2 emissions.
4. Econometric methodology
To have a tool to assess the achievability of the set targets through forecasting, initially, we analyze the effects of GDP per capita, oil, natural gas, and coal intensities of GDP on CO2 emissions, applying different time-series methods. We will first test the used variables for a unit root in the empirical part. The Augmented Dickey-Fuller (Dickey & Fuller, Citation1981, ADF) unit root test will be applied to assess the non-stationarity properties of variables.
Next, if the degrees of integration of the variables are the same, the cointegration test(s) will be performed to see if they are cointegrated. For this exercise, we utilize the Bounds Testing Approach to Autoregressive Distributed Lagged (ARDLBT, Pesaran & Shin, Citation1999; Pesaran, Shin, & Smith, Citation2001) and the PcGive cointegration test (Banerjee, Dolado, & Mestre, Citation1998, inter alia). Additionally, the General to Specific (Gets) modeling approach is utilized to estimate the long-run impacts of GDP per capita, oil, natural gas, and coal intensities of GDP on CO2 emissions. This methodology suggests considering both the applicability of the theory and being in line with the Data Generating Process (DGP). The general unrestricted model (GUM) is first constructed in this approach, including all the theory-related prospective variables with corresponding lag lengths. Then in the first stage, maintaining all the theory-related variables, one looks for if there is an “additional” part of DGP that can be explained with intervention dummies. The procedure utilizes all possible dummies. To put it differently, it utilizes and examines impulse (Impulse Indicator Saturation, IIS), step (Step Indicator Saturation, SIS), blip (Differenced Impulse Indicator Saturation, DIIS), and break-in trend (Trend Indicator Saturation, TES) dummies for every single observation. The multipath search algorithm tests the relevance/significance of used intervention dummies. The chosen dummies are kept fixed in the second step, and one searches for theory-relevant variables. Similar to the first step, this is also made by applying a multipath search algorithm. The search technique tries to “assess” the so-termed local data-generating process, aiming for the final model’s congruency (no loss of information) to the GUM. In both steps, since the number of parameters to be estimated becomes bigger, the algorithm uses a block search. The role of machine learning algorithms like Autometrics (see Hendry & Doornik, Citation2014, among others) in this objective is indisputable, as multipath and block search techniques are challenging to deal with. For additional aspects of the Gets methodology, interested readers can refer to Hendry and Doornik (Citation2014) and Doornik and Hendry (Citation2018). In the early phase, the model includes the following theory-related variables: per capita CO2 emissions (dependent variable), real per capita GDP, oil, natural gas, and coal intensities of GDP. All variables are put into the first specification with two lags. After including the theory-related variables and applying the practice of the Gets approach in the initial step, they were included in the search process, and the saturation indicators were selected utilizing the Autometrics algorithm. After selecting relevant intervention dummies, they were kept unsearched in the second step. The final model chose the theory-related variables (See Castle, Doornik, & Hendry, Citation2021; inter alia, on the entire selection process). Finally, ARDL, FMOLS, and Structural Time Series Modeling (STSM) approaches are used for robustness checks. Dickey and Fuller (Citation1981) for the ADF test, Harvey (Citation1989) for the STSM approach, Phillips and Hansen (Citation1990) for the FMOLS method, Pesaran and Shin (Citation1999), and Pesaran et al. (Citation2001) for the ARDL approach, among others, have presented comprehensive descriptions and could be referred to for detailed information.
5. Data
This paper uses annual data for the variables mentioned below from 1990 through 2020. The used sample is chosen based on the data availability. CO2 is CO2 emissions from fuel combustion in MtCO2 and sourced from Enerdata (Citation2022). The historical trajectory of CO2 emissions is depicted in .
In the early 90ths CO2 emissions level have been declining following the country’s economic and social structural change, shifting from the “golden periods” of the former Soviet time to the newly established state. Undoubtedly, it was not a result of higher environmental concerns and demand for a better environment; rather, it had resulted due to the worsening economic situation that had lasted by the early 2000s. Hence, it is crucial to consider this fact while modeling CO2 emissions in the case of Russia and similar countries. Ignoring the historical evaluation of the CO2 emissions path might result in misleading results, such as negative income impact or an inverted U-shaped relationship, which is less likely for the developing country.
GDP is a gross domestic product in billions of Rubles, 2016 constant prices. It is retrieved from Oxford Economics (Citation2022). CO2 emissions and GDP data are expressed per capita using the population data from Oxford Economics (Citation2022).
Oil intensity of GDP is oil consumed per unit of GDP. The natural gas intensity of GDP is natural gas consumed per unit of GDP. Coal intensity of GDP is coal consumed per unit of GDP. The corresponding oil, natural gas and coal intensities of GDP are used for the corresponding oil, natural gas and coal consumption numbers. Data for oil, natural gas, and coal are taken from Enerdata (Citation2022). The fuel consumption data are in Mt. In empirical estimations, the specification (6) is used; hence, all variables are expressed in logarithmic form.
6. Empirical estimation results
At the beginning of empirical estimation, the unit-root properties of the utilized variables are tested by applying the ADF unit-root test. The ADF test results are presented in .
The results of the ADF test revealed that all variables are I(1), which means that they are stationary at the first difference. As a result, we can evaluate the possibilities of a long-term cointegration link. The results of cointegration analyses are depicted in .
The employed Bounds test and PcGive test for cointegration conclude the existence of a long-run cointegration relationship. As mentioned in the methodology section, to investigate the relationship between variables of interest, we have used the General to Specific methodology using the Autometrics algorithm (Hendry & Doornik, Citation2014). Utilizing the Gets approach, the theory-related variables were not included in the initial stage of the search procedure. The search was performed for the intervention dummies with a tighter significance level. Taking into account the available number of observations, we used two as a maximum lag length.
Consequently, having chosen the saturation dummies in the first stage, they were kept fixed in the second step, and the search was repeated for theory-related variables. The search algorithm is utilized for the second step, allowing a 10% significance level. The search algorithm is accomplished utilizing the PcGive 15.10 element of the OxMetrics 8.10 software platform. The found equation in dynamic form is modified to see the final static form of the long-run equation, as suggested by Doornik and Hendry (Citation2018). The long-run estimation results of the Gets approach are provided in .
Moreover, (in the Appendix) provides the diagnostic test results for the selected final model. As the results in demonstrate, the selected model passes all the diagnostic tests. We also tested the parameters for the potential non-linearity utilizing the non-linearity and conventional RESET tests, and the findings are given in (in Appendix). As the results show, there is not enough evidence to conclude non-linearity in the parameters.
As was mentioned in the Methodology section, we also utilized ARDL, FMOLS, and STSM estimation techniques for the robustness check, and the estimation results are also given in . As evident from , all estimation techniques produced quite similar results for the parameters. Even the ARDL results are identical to the Gets results. These findings show the robustness of our empirical findings.
To check the in-sample replicating quality of the estimated model, we performed the dynamic forecast using the sample data, and the results are given in Figure A1 (Appendix). As the figure demonstrates, the in-sample forecasting quality of the model is quite high.
7. Forecast assumptions
As mentioned in the introduction, Russia targets lowering GHG emissions by at least 30% below 1990 levels by 2030. CO2 emissions comprise almost 80% of overall GHG for Russia, based on 2018 data point (Climate Watch, Citation2022a). Making the same assumption as a target for CO2 emissions, this target translates to having a CO2 emissions level of around 1530 MtCO2 in 2030. This number is 200 MtCO2 below the 2019 value (considering the Covid-19 impact in 2020, we preferred to compare it with the 2019 value). To see the output for the case when the economy goes in the pace as it is, we first created a business as usual (Sce1) scenario. In the Sce1 scenario, assuming that the drivers of CO2 emissions on average will not substantially change their behaviors for the coming ten years, 2010–2020 average growth rates are taken as assumptions for all independent variables. The detailed forecast assumptions are described in .
Table 5. Forecast assumptions.
Considering that coal is more CO2 intensive compared to natural gas and oil, and it is a primary source of a recent increase in energy-related CO2 emissions globally (IEA, Citation2022a), one direction for lowering the CO2 emissions level might be relying less on coal. Hence, in the other two scenarios, we consider reducing coal consumption. In other words, we created two additional scenarios, Sce2 and Sce3, to see the potential path of CO2 emissions in case coal consumption is reduced. In Sce2, natural gas remains as in 2020; coal decreases by 15% annually, oil consumption and GDP are as in Sce1; in Sce3, natural gas remains as in 2020, coal decreases by 10% annually, oil consumption and GDP are as in Sce1. These assumptions are used only for comparison purposes to assess if the 2030 target is rational. Since there are a number of different potential assumptions for the fuel mix trajectories, the relevant ones could be chosen depending on the set policy strategy.
8. Forecasting results
Utilizing the estimated model for CO2 emissions and using the assumptions made in the previous section, we have made projections until 2030, and the results are depicted in . In forecasting exercises, the Gets resulted model is used. demonstrates the forecasting results for scenario 1 or the base case scenario, with 95% confidence intervals.
In this case, the forecasted value for 2030 is 1750 MtCO2, with 95% confidence interval values of 1703 MtCO2 and 1796 MtCO2. The results of CO2 emissions projections for all three scenarios are presented in .
9. Discussion of empirical findings and forecasting results
The current study explores the effects of GDP per capita, oil, natural gas, and coal intensities of GDP on CO2 emissions, using different time-series techniques for Russia. Another distinctive feature of this study is that it forecasts emissions by 2030 and compares the findings with targets to assess their achievability. According to the utilized unit root test, all variables are integrated of order one. Additionally, the PcGive and Bound cointegration tests showed the presence of a long-run link among the variables. Then, we evaluated the long-term effects of the used variables on CO2 emissions and presented the findings in . The signs of all mentioned variables are found to be consistent with the expectations, as discussed in the Functional Model Specification section. According to the results, a 1% rise in the GDP per capita results in a 1.022% rise in CO2 in the long run. This result is consistent with the theoretical approach of the Functional Model Specification section and the findings of several studies such as Apergis and Payne (Citation2010), Zakarya et al. (Citation2015), Yang et al. (Citation2017), Shuai et al. (Citation2017), Ketenci (Citation2018), Cheng et al. (Citation2019), Dong et al. (Citation2019a), Dong et al. (Citation2019b), Danish (2019), Hasanov et al. (Citation2021) Chisti and Sinha (Citation2022) and inter alia. Our income elasticity, in terms of the sign, is different from the findings of Pao et al. (Citation2011) and Kilinc-Ata and Likhachev (Citation2022) in the case of Russia. The different signs of the same variable can be explained by the size and frequency of the studied periods, used empirical methods, inter alia. The negative impact of income on emissions level in the case of Russia as a developing country does not seem to be relevant. Since Russia had undergone a new structural reshaping during the 90s, after the collapse of the former Soviet Union, special attention should be paid while modeling the relationships representing true economic environment phenomenon. This also applies equally to CO2 emissions modeling. Furthermore, according to environmental theories, higher GDP will result in increased CO2 emissions: increased economic activity or income leads to increased consumption of intermediate and final goods and services, resulting in increased CO2 emissions. Hence, we believe that our finding of positive income impact is more aligned with economic reasoning, considering the country’s development stage.
In addition, the estimation results revealed that oil, natural gas, and coal intensities of GDP have a statistically significant and positive impact on CO2 emissions. Based on the results, in ceteris paribus, a 1% rise in oil, natural gas, and coal intensities of GDP raises CO2 emissions by 0.230%, 0.503%, and 0.307%, respectively, in the long run. This means that natural gas has the greatest influence on CO2 emissions, followed by coal and oil. This finding coincides with the characteristics of Russia and the theoretical expectations, as discussed in section 3. Russia’s primary source of GHG emissions was the energy industry, accounting for almost 1.67 billion tons of CO2 equivalent (STATISTA, Citation2022). Combustion of fossil fuels accounts for 80% of all greenhouse gas emissions in Russia. Approximately half of them (47%) are created by energy sectors, such as electricity and heat generation, while 30% are produced by the transportation sector, industrial output, and building (Stepanov & Makarov, Citation2021). Besides, the findings of the study conducted by Yang et al. (Citation2017) support the results of our research. Thus, he stated that around 65% of all emissions were attributable to energy usage (representing the largest proportion). According to his analysis, the use of natural gas, which accounted for more than 45% of all energy-related emissions, was the primary source of emissions. About 36% of emissions were produced by the use of coal and coal-derived goods, and 17% of emissions were caused by the consumption of crude oil and crude oil-derived products. In addition, our research findings are aligned with the conclusions of previous studies like Dong et al. (2019) for Europe & Eurasia countries (including Russia), Abban et al. (2019) for high-income economies (including Russia), Inglesi-Lotz (Citation2017) for BRICS countries, Brizga et al. (Citation2013) for 15 former Soviet countries (including Russia) which revealed a positive impact of energy intensity on CO2 emissions.
According to the forecasting exercises results, if the current situation, in terms of economic activities and policies followed, goes as it is, the CO2 level is expected to reach above 1700 Mt of CO2 emissions. In this case, the 2030 target seems hard to achieve. However, in case of a bit ambiguous no coal scenario, the 2030 numbers for CO2 emissions are found to be 1011 Mt and 1200 Mt in scenarios 2 and 3, accordingly. Both SCE2 and Sce2 produce CO2 levels below the country’s 2030 target. Although it might require additional and substantial efforts to eliminate coal consumption, considering its vast availability, a shift to renewables could be a feasible way to follow, in parallel with exporting more fossil fuels or utilizing the so-called circular carbon economy direction.
10. Conclusion and policy implications
This paper first proposes a functional specification that relates CO2 emissions to energy consumption indicators, modifying the so-called Kaya identity. Then using the developed framework, the article examines the impact of GDP per capita, oil, natural gas, and coal intensities on CO2 emissions. According to the unit root tests, all variables have the same integration order (I (1)). As a result, the presence of a long-run cointegration connection may be assessed. According to the cointegration test, the variables move together in the long term. The General to Specific modeling approach, ARDL, FMOLS, and STSM, is applied to evaluate the long-run impact of independent variables on the dependent variable (CO2 emissions). A machine-learning multipath search algorithm, Autometrics, is used as the main estimation tool. The estimation findings stated that all independent variables (GDP per capita, oil, natural gas, and coal intensities of GDP) have a statistically positive impact on CO2 emissions. These findings align with both our expectations and the theory of economy. Thus, when oil prices rise, Russia earns more oil revenues as an oil-rich country, resulting in GDP increases. As a result, the income of economic units increases, leading to higher consumption of products, which leads to a rise in CO2. In addition, it is widely known that CO2 emissions are mainly derived from the combustion of fossil fuels. As fossil fuel consumption increases, this results in more CO2 emissions. In 2019, Russia’s most significant volume of fuel-burning based CO2 emissions was from natural gas, with 854 million metric tons of CO2. The coal and oil industries’ CO2 emissions grew over the previous year, reaching 431 and 312 million metric tons, respectively (STATISTA, Citation2022).
The forecasting practice findings showed that in the current modus operandi, the set 2030 target is less likely to achieve. Since Russia is one of the top emitters, missing the 2030 target will keep contributing to global warming during the upcoming 7 years. The main reason behind this conclusion is that atmospheric CO2 concentrations result from cumulative emissions. However, as the no or fewer coal scenarios concluded, this target could be achieved with a substantial structural reshaping of the way of fueling the economy. These could be achieved by phasing out the use of coal (alternatively or simultaneously oil) and shifting to renewable energy sources. Considering the country’s regional dimensions, which provide substantial sources of renewables, this shift could be an alternative for better environmental quality. Another alternative for lowering CO2 emissions and reaching the target could be initiating the so-called circular carbon economy approach.
Moreover, considering the gravity of climate change-related environmental deterioration and the negative impact of subsidizing fuel prices, Russian policymakers may consider phasing out the fossil fuel subsidies. Parry et al. (Citation2021) found that the production and burning of global fossil fuel subsidies summed up to 5.9 trillion USD in 2020. In addition to ranking among the top three subsidizers in terms of absolute amounts, Russia is also among the top five subsidizers in terms of amounts per person. They find that, in case of raising fossil fuel prices, globally fuel-related CO2 emissions will be 36 % lower in 2025, state fiscal revenues will increase by 4.2 trillion USD, and 0.9 million lives will be saved by 2025. That is, funding will cover spending needs for Sustainable Development Goals. IEA (Citation2022a) pathway suggests a global 14 million job creation effect of clean energy investments. For the under 1.5 C scenario, IRENA (Citation2022) finds that by 2030 Russian economy will lose 1.6 million fossil fuel jobs, and 1.3 million jobs will be created with the implementation of the energy transition process. Net job loss will require bigger investments. That is, diversification towards clean energy is not only vital for climate concerns but also, it is important for social welfare. In 2019, the country’s total cumulative installed wind capacity was 100 MW. Whereas only in 2020, the newly installed wind capacity equal 800 MW. These facts signal the potential of the Russian economy for clean energy resources. It is estimated that wind industry employment is around 12,000 workers (IRENA, Citation2022). The above-mentioned policy interventions might help the country to rationalize energy consumption behavior and continue using available alternative energy sources while mitigating environmental deterioration. Recent studies show that countries around the globe, including Russia, are in the era of ample and profitable alternatives to lower CO2 emissions. For example, in the analysis covering 145 countries, Jacobson et al. (Citation2022) estimate that the present value cost of a 100 % transition to clean energy by 2035 would be 61.5 trillion dollars. The striking conclusion of this study suggests that a 100 % clean energy transition will generate 28.4 million full-time jobs, and the payback time of the capital cost is 5.5 years. On the other hand, Way et al. (Citation2022) came up with three different energy transition scenarios: Fast, slow, and no. Their findings suggest that a fast transition is likely cheaper than other scenarios and generates 12 trillion USD net present savings. The main driver of this saving is the rapid fall in solar and wind power costs. Even though Russia has significant renewable energy potential, Kilinc-Ata and Dolmatov (Citation2022b) find that consumers face technological constraints, which in turn lowers commercial renewable energy consumption. Eventually, the country fails to attract the necessary investment. The lack of the suitable investment climate that can provide commercialization of renewable energy sources slows down the smooth energy transition.
Last but not least, one of the recent studies found that to reach the goals set in Paris Agreement, greenhouse gas emissions should be reduced by 25 % in 2030, relative to 2022. That is, gone are the days when there was a smooth transition to a more-carbon neutral planet, and this transition is now becoming excessively challenging once a procrastinated stance further sets in. Estimations show that annual growth will be reduced between 0.15 and 0.25 % a year in the scenario of acting earlier, whereas sticking to inaction will generate higher costs, especially for fossil fuel exporting countries (IMF, Citation2022). In this case, policymakers will need to consider long-term output losses stemming from climate change. In a nutshell, countries like Russia should consider the job creation potential of renewable industries, the declining costs of renewable energy sources, and the urgency of the transition to a carbon-neutral era and act accordingly.
A top emitter like Russia’s missing CO2 emissions target and not being part of the Global Methane Pledge will accelerate climate change and global warming. In this manner, we have modeled the historical relationship of CO2 emissions and explored its future path for the Russian case. Similar exercises might be devoted to methane emissions as a separate research direction. Disaggregating GHG emissions like that will provide a broader picture of the climate impacts of national commitments for the Russian case.
This paper analyzes Russia’s emission targets by assuming the willingness of Russian policymakers to fulfill already-announced commitments. Since conducting the current study started long before 24 February 2022, that is, starting date of the Russian invasion of Ukraine, the paper does not have any capacity to consider potential deterioration in the global cooperation on climate goals. There is still uncertainty about how the Russian policymakers will return to the climate negotiations. Tensions related to the Russian invasion of Ukraine may exacerbate Russia’s need for fossil fuel to cover fiscal deficits and secure an externally healthy economy and also trigger major disruption for climate negotiations and initiatives. It will require another study with new assumptions and scenario simulations. Since mentioned novel study will most likely find a more considerable deviation from Russian commitments, still current study is an important contribution to the existing literature by providing peacetime evaluations. Another limitation of this study is about the current global greenhouse gas emissions information landscape. NASEM (Citation2022) finds that there are numerous methods to keep track of GHG emissions. For example, Böttcher et al. (Citation2021) opine significant uncertainties regarding flaring emissions in the Russian case. Since there is little coordination between all these different approaches, NASEM (Citation2022) suggests a single global clearinghouse for greenhouse gas information. Countries worldwide need to develop their methods for high-quality emissions reporting systems. United Nations climate change conference COP27 may handle this issue, and only then may there be better data available for research.
Acknowledgments
The views expressed in this paper are those of the authors and do not necessarily represent their affiliated organizations. Authors thank to anonymous referees for their valuable comments on the analysis of the paper.
Disclosure statement
No potential conflict of interest was reported by the author(s).
Additional information
Notes on contributors
Sarvar Gurbanov
Sarvar Gurbanov holds PhD degree in Economics. He has been Fulbright Visiting Scholar at the University of Southern California, in Los Angeles, USA and conducted research on energy economics and governance. His current research areas engage with ongoing scholarly discussions related to mitigation of CO2 and methane emissions. He is Assistant Professor of Economics at ADA University`s School of Public and International Affairs (SPIA) in Baku, Azerbaijan.
Jeyhun I. Mikayilov
Jeyhun I. Mikayilov is a research fellow at KAPSARC. He received his B.A. and M.S. from Azerbaijan State University (now Baku State University) in mathematics. Jeyhun holds a Ph.D. in applied mathematics and a D.S. in econometrics. Before joining KAPSARC in September 2017, Jeyhun was an associate professor at the Department of Statistics and Econometrics at Azerbaijan State University, and the Department of Economics at Qafqaz University, where he taught econometrics, statistics, and mathematical economics. His other roles have included director of the Research Institute for Social Sciences and Humanities, and head of the Center for Socio-Economic Research. Jeyhun was a postdoctoral researcher at Indiana University Bloomington, United States (U.S.). He has also been a visiting researcher at several institutions, including the Center for Econometric Research, Sungyunkwan University in Seoul, South Korea; Vistula University, Warsaw, Poland; the University of North Texas, and the University of South Texas, both in the U.S.Jeyhun’s research is focused on applied time series econometrics, the economics of energy, the environment and sustainable development. He has authored over 40 scientific articles published in peer-reviewed journals and is an editorial board member of the International Journal of Advanced Multidisciplinary Research and Review, the Journal of Management, Economics and Industrial Organization, and the Journal of Socio-Economic Studies, and a member of the International Association for Energy Economics.
Shahriyar Mukhtarov
Dr. Shahriyar Mukhtarov is an associate professor of the Department of Economics, at Vistula University, Poland. Dr. Mukhtarov received his M.A. from Marmara University in 2012 and completed his Ph.D. in Banking at Marmara University in 2016.The holder of several national and international awards, Dr. Mukhtarov has realized various research projects in Macroeconomics and Microeconomics and published numerous scientific articles on Monetary and Fiscal Policy, Sustainable Development, Energy Economics and Banking in peer-reviewed journal which are indexed by Web of Science and Scopus databases. He has served on many editorial boards and as referee of the many journals. Mukhtarov was Awarded second place in the competition ”Victory Award in Science-2021” organized by the Science Development Foundation under the President of the Republic of Azerbaijan, the Azerbaijan National Academy of Sciences (ANAS), the Islamic World Organization for Science, Education and Culture (ICESCO) and the International Engineering and Technology Institute (IETI). In addition, Mukhtarov completed research entitled “Exploring Exports Driven Growth Through Free Trade Agreements among CAREC Members: Learning from Pak-China Free Trade Agreement” under the CAREC Institute CTTN Research Grants Program.He also delivers lectures in Research Methods, Microeconomics, Macroeconomics and Monetary Policy. Mukhtarov's research interests include the energy economics, sustainable development, monetary economics, building and applying econometric models for policy purposes.
Sakit Yagubov
Sakit Yagubov graduated from the Azerbaijan Institute of National Economy (AINE). He has a Ph.D. and Doctor of Sciences in Statistics from the Moscow Institute of Economics.From 1975-1977 he served as an economist, chief economist, and director of the “Agricultural Statistics” department of the Central Statistical Office of Azerbaijan SSR (now the State Statistics Committee of the Azerbaijan Republic). From 1980-1990 he worked as an instructor at the “Statistics” department of AINE and the Baku branch of the Leningrad Institute of Finance and Economics.Professor Yagubov worked as a professor at the “Statistics” department of the Azerbaijan State Institute of Economics from 1993-2000 and from 2000 to 2017 as the head of the “Statistics” department of the Azerbaijan State University of Economics. From September 2017 to February 2018, he worked as the head of the department of “Statistics and Econometrics.”Professor Yagubov is the Vice-Rector for Science and Innovations at the Azerbaijan State University of Economics since February 2018. At the same time, he is a professor in the Statistics and Econometrics department.Yagubov is the author of more than 115 scientific-methodical papers.Professor Yagubov is a “Statistical Council” member under the State Statistics Committee of the Republic of Azerbaijan and editor-in-chief of the scientific-practical journal “Statistical News.”
References
- Abban, O. J., Wu, J., & Mensah, I. A. (2020). Analysis on the nexus amid CO2 emissions, energy intensity, economic growth, and foreign direct investment in belt and road economies: Does the level of income matter? Environmental Science and Pollution Research, 27(10), 11387–26.
- Allen, M. R., Peters, G. P., Shine, K. P., Azar, C., Balcombe, P., Boucher, O., … Tanaka, K. (2022). Indicate separate contributions of long-lived and short-lived greenhouse gases in emission targets. Npj Clim Atmos Sci, 5(5). doi:10.1038/s41612-021-00226-2
- Al-Mulali, U., Ozturk, I., & Solarin, S. A. (2016). Investigating the environmental Kuznets curve hypothesis in seven regions: The role of renewable energy. Ecological Indicators, 67, 267–282.
- Apergis, N., & Payne, J. E. (2010). The emissions, energy consumption, and growth nexus: Evidence from the commonwealth of independent states. Energy Policy, 38(1), 650–655.
- Azevedo, V. G., Sartori, S., & Campos, L. M. S. (2018). CO2 emissions: A quantitative analysis among the BRICS nations. Renewable and Sustainable Energy Reviews, 81(1), 107–115.
- Banerjee, A., Dolado, J. J., & Mestre, R. (1998). Error-correction mechanism tests for cointegration in a single equation framework. Journal of Time Series Analysis, 19(3), 267–283.
- Berkeley Earth. (2021). Global temperature report for 2020. January, 14. http://berkeleyearth.org/global-temperature-report-for-2020/ (01.Feb.2022).
- Berkeley Earth. (2022). Global Temperature Report for 2021. http://berkeleyearth.org/global-temperature-report-for-2021/ (01.Feb.2022).
- Böttcher, K., Paunu, -V.-V., Kupiainen, K., Zhizhin, M., Matveev, A., Savolahti, M., … Karvosenoja, N. (2021). Black carbon emissions from flaring in Russia in the period 2012–2017. Atmospheric Environment, 254, 118390.
- Brizga, J., Feng, K., & Hubacek, K. (2013). Drivers of CO2 emissions in the former Soviet Union: A country level IPAT analysis from 1990 to 2010. Energy, 59, 743–753.
- Castle, J. L., Doornik, J. A., & Hendry, D. F. (2021). Robust discovery of regression models. Econometrics and Statistics, Econometrics and Statistics. doi:10.1016/j.ecosta.2021.05.004
- Cheng, C., Ren, X., Wang, Z., & Yan, C. (2019). CO2 emissions, renewables, environmental patents, and economic growth - Evidence from BRIICS. Science of the Total Environment, 668, 1328–1338.
- Chishti, M. Z., & Sinha, A. (2022). Do the shocks in technological and financial innovation influence the environmental quality? Evidence from BRICS Economies, Technology in Society, 68. doi:10.1016/j.techsoc.2021.101828
- Climate Action Tracker (CAT). 2022. Russian Federation. https://climateactiontracker.org/countries/russian-federation/ (11March 2022).
- Climate Watch. (2022a). Historical GHG emissions. https://www.climatewatchdata.org/ghg-emissions?breakBy=gas&chartType=percentage®ions=RUS&source=CAIT (03March 2022).
- Climate Watch. (2022b). Historical GHG emissions (World Resources Institute). 11March 2022 https://www.climatewatchdata.org/ghg-emissions?breakBy=countries&chartType=percentage&end_year=2018&gases=co2®ions=TOP&source=CAIT&start_year=1990
- Dickey, D., & Fuller, W. (1981). Likelihood ratio statistics for autoregressive time series with a unit root. Econometrica, 49(1981), 1057–1072.
- Dietz, T., & Rosa, E. A. (1994). Rethinking the environmental impacts of population, affluence and technology. Human Ecology Review, 1, 277–300.
- Dietz, T., & Rosa, E. (1997). Effects of population and affluence on CO 2 emissions. Proceedings of the National Academy of Sciences, 94(1), 175–179.
- Dong, K., Dong, X., & Dong, C. (2019a). Determinants of the global and regional CO 2 emissions: What causes what and where? Applied Economics, 51(46), 5031–5044.
- Dong, K., Dong, X., & Jiang, Q. (2019b). How renewable energy consumption lower global CO2 emissions? Evidence from countries with different income levels. The World Economy, 43(6), 1665–1698.
- Doornik, J. A., & Hansen, H. (1994). A practical test for univariate and multivariate normality. Discussion paper, Nuffield College.
- Doornik, J. A., & Hendry, D. F. (2018). Empirical econometric modelling using PcGive (8th, Vol. I). London: Timberlake Consultants Press.
- EC (European Commission). (2022). Launch by United States, the European Union, and partners of the global methane pledge to keep 1.5C within reach. https://ec.europa.eu/commission/presscorner/detail/en/statement_21_5766 (03.March.2022).
- Enerdata. (2022). https://www.enerdata.net/ (03.March.2022).
- Engle, R. F. (1982). Autoregressive conditional heteroscedasticity, with estimates of the variance of United Kingdom inflation. Econometrica, 50(4), 987–1007.
- Enrlich, P., & Holdren, J. (1971). Impact of population growth. Science, 171(3977), 1212–1217.
- Europe, & Lancet Regional Health – Europe, T.; The Lancet Regional Health. (2022). The regional and global impact of the Russian invasion of Ukraine. The Lancet Regional Health - Europe, 15(2022), 100379.
- Global Methane Pledge. (2022). Fast action on methane to keep a 1.5°C future within reach. https://www.globalmethanepledge.org/ (03.March.2022).
- Godfrey, L. G. (1978). Testing for higher order serial correlation in regression equations when the regressors include lagged dependent variables. Econometrica, 46(6), 1303–1313.
- Grossman, G. M., & Krueger, A. B. (1991). Environmental impacts of a north American free trade agreement. In Working paper (pp. 3914). Cambridge: National Bureau of Economic Research.
- Harvey, A. C. (1989). Forecasting, structural time series and the kalman filter. Cambridge, UK: Cambridge University Press.
- Hasanov, F. J., Khan, Z., Hussain, M., & Tufail, M. (2021). Theoretical framework for the carbon emissions effects of technological progress and renewable energy consumption. Sustainable Development, 1–13. doi:10.1002/sd.2175
- Hendry, D. F. (2020). First in, first out: econometric modelling of UK annual CO2 emissions, 1860–2017. Accessed on October 9, 2022. https://www.nuffield.ox.ac.uk/economics/papers/2020/2020W02_CO2UKEmissionsModel20.pdf
- Hendry, D. F., & Doornik, J. A. (2014). In Empirical model discovery and theory evaluation. Cambridge, MA: MIT Press.
- Hobbs, P. V., & Radke Lawrence, F. (1992). Airborne studies of the smoke from the Kuwait oil fires. Science, 256(5059), 987–991.
- Inglesi-Lotz, R. (2017). Decomposing the south African CO2 emissions within a BRICS Countries context: Signalling potential energy rebound effects. Energy. doi:10.1016/j.energy.2017.12.150
- Intergovernmental Panel on Climate Change (IPCC). (2021). AR6 climate change 2021: The physical science basis. https://www.ipcc.ch/report/ar6/wg1/downloads/report/IPCC_AR6_WGI_SPM_final.pdf (01.Feb.2022).
- Intergovernmental Panel on Climate Change (IPCC). (2022). Climate change 2022: Mitigation of climate change. Working group III contribution to the IPCC sixth assessment report (AR 6). https://www.ipcc.ch/report/ar6/wg3/ (28.April.2022).
- International Energy Agency (IEA). (2022a). Net Zero by 2050: A roadmap for global energy sector 2021 (Paris). https://www.iea.org/reports/net-zero-by-2050 (22.May.2022).
- International Energy Agency (IEA). (2022b). Global CO2 emissions rebounded to their highest level in history in 2021 (Paris). https://www.iea.org/news/global-co2-emissions-rebounded-to-their-highest-level-in-history-in-2021 (11.March.2022).
- International Monetary Fund (IMF). (2022). World economic outlook report, chapter 3: Near-term macroeconomic impact of decarbonization policies (Washington, DC). https://www.imf.org/en/Publications/WEO/Issues/2022/10/11/world-economic-outlook-october-2022 (10.Oct.2022).
- International Renewable Energy Agency (IRENA). (2022). World energy transitions outlook 2022: 1.5°C pathway (Abu Dhabi). https://www.irena.org/publications/2022/Mar/World-Energy-Transitions-Outlook-2022 (22.May.2022).
- Ito, K. (2017). CO2 emissions, renewable and non-renewable energy consumption, and economic growth: Evidence from panel data for developing countries. International Economics, 151, 1–6.
- Jacobson, M. Z., von Krauland, A.-K., Coughlin, S. J., Dukas, E., Nelson, A. J. H., Palmer, F. C., & Rasmussen, K. R. (2022). Low-cost solutions to global warming, air pollution, and energy insecurity for 145 countries. Energy & Environmental Science, 15(8), 3343–3359.
- Jaforullah, M., & King, A. (2017). The econometric consequences of an energy consumption variable in a model of CO 2 emissions. Energy Economics, 63, 84–91.
- Kaya, Y. (1990). Impacts of carbon dioxide emission control on GNP growth: Interpretation of proposed scenarios. In Proceedings of the IPCC Energy and Industry Subgroup, Response Strategies Working Group, Paris, France.
- Ketenci, N. (2018). The environmental Kuznets curve in the case of Russia. Russian Journal of Economics, 4(2018), 249–265.
- Kijima, M., Nishide, K., & Ohyama, A. (2010). Economic models for the environmental Kuznets curve: A survey. J. Econ Dynam Contr, 34(7), 1187–1201.
- Kilinc-Ata, N., & Dolmatov, I. A. (2022a). The Russian federation’s renewable energy development determinants: Evidence from empirical research. International Journal of Energy Sector Management, ahead-of-print No. ahead-of-print. doi:10.1108/IJESM-04-2022-0018.
- Kilinc-Ata, N., & Dolmatov, I. A. (2022b). Which factors influence the decisions of renewable energy investors? Empirical evidence from OECD and BRICS countries. Environmental Science and Pollution Research. doi:10.1007/s11356-022-22274-8
- Kilinc-Ata, N., & Likhachev, V. L. (2022). Validation of the environmental kuznets curve hypothesis and role of carbon emission policies in the case of Russian Federation. Research Square, Preprint. doi:10.21203/rs.3.rs-1279598/v1.
- Kim, T.-Y. (2022). Critical minerals threaten a decades-long trend of cost declines for clean energy technologies. https://www.iea.org/commentaries/critical-minerals-threaten-a-decades-long-trend-of-cost-declines-for-clean-energy-technologies?utm_content=buffer46523&utm_medium=social&utm_source=twitter.com&utm_campaign=buffer (20.May.2022).
- Lieb, C. M. (2003). The environmental Kuznets curve—a survey of the empirical evidence and of possible causes. University of Heidelberg discussion paper, No. 391.
- Meinshausen, M., Lewis, J., McGlade, C., Gütschow, J., Nicholls, Z., Burdon, R., & Hackmann, B. (2022). Realization of Paris agreement pledges may limit warming just below 2 °C. Nature, 604(7905), 304–309.
- Mikayilov, J. I., Marzio, G., & Hasanov, F. J. (2018). The Impact of Economic Growth on CO2 Emissions in Azerbaijan. Journal of Cleaner Production, 197, 1558–1572.
- Mitic, P., Ivanovic, O. M., & Zdravkovic, A. (2017). A cointegration analysis of real GDP and CO2 emissions in transitional countries. Sustainability, 9(4), 1–18.
- Narayan, P. K., Saboori, B., & Soleymani, A. (2016). Economic growth and carbon emissions. Economic Modelling, 53, 388–397.
- National Academy of Sciences, Engineering and Medicine (NASEM). (2022). Greenhouse gas emissions information for decision making: A framework going forward. Washington, DC: The National Academies Press. doi:10.17226/26641
- Osobajo, O. A., Otitoju, A., Otitoju, M. A., & Oke, A. (2020). The Impact of Energy Consumption and Economic Growth on Carbon Dioxide Emissions. Sustainability, 12(19), 7965.
- Oxford Economics. (2022). Oxford economics global economic model database. March release. https://www.oxfordeconomics.com/service/subscription-services/macro/global-economic-model/ (01.Feb.2022).
- Pao, H., Yu, H., & Yang, Y. (2011). Modelling the CO2 emissions, energy use, and economic growth in Russia. Energy, 36(8), 5094–5100.
- Parry, I. W. H., Black, S., & Vernon, N. (2021). Still not getting energy prices right: A global and country update of fossil fuel subsidies, IMF Working Paper, No. 2021/236 (10.11.)
- Perez Suarez, R., & Lopez-Menendez, A. J. (2015). Growing green? Forecasting CO2 emissions with environmental kuznets curves and logistic growth models. Environmental Science & Policy, 54, 428–437.
- Pesaran, M. H., & Shin, Y. (1999). An autoregressive distributed lag modeling approach to cointegration analysis. In S. Strom (Ed.), Econometrics and economic theory in the 20th century: The Ragnar Frisch Centennial Symposium. Cambridge, UK: Cambridge University Press, 371–413.
- Pesaran, M. H., Shin, Y., & Smith, R. J. (2001). Bounds testing approaches to the analysis of level relationships. Journal of Applied Econometrics, 16(3), 289–326.
- Phillips, P. C. B., & Hansen, B. E. (1990). Statistical inference in instrumental variables regression with I(1) processes. The Review of Economic Studies, 57(1), 99–125.
- Ramsey, J. B. (1969). Tests for specification errors in classical linear least squares regression analysis. Journal of the Royal Statistical Society, Series B, 31, 350–371.
- Shafik, N., & Bandyopadhyay, S. (1992). Economic growth and environmental quality: Time series and cross-country evidence. Background paper for the world development report 1992. The World Bank, Washington, D.C.
- Shapovalova, D. (2020). Oil spill in Siberia: Are we prepared for permafrost thaw? https://www.thearcticinstitute.org/oil-spill-siberia-prepared-permafrost-thaw/ (01.Feb.2022).
- Shuai, C., Chen, X., Shen, L., Tan, Y., Tan, Y., & Tan, Y. (2017). The turning points of carbon Kuznets curve: Evidences from panel and time-series data of 164 countries. Journal of Cleaner Production, 162, 1031–1047.
- STATISTA, (2022). https://www.statista.com/statistics/1048675/greenhouse-gas-emissions-by-sector-russia/. (15.May.2022).
- Steel, D., DesRoches, C. T., & Mintz-Woo, K. (2022). Climate change and the threat to civilization. Proceedings of the National Academy of Sciences, 119(42). doi:10.1073/pnas.2210525119
- Stepanov, I. A., & Makarov, I. A. (2021). Greenhouse gas emissions regulation in fossil fuels exporting countries: Opportunities and challenges for Russia. Post-Communist Economies. doi:10.1080/14631377.2021.1943918
- Stolyarova, E. (2013). Carbon dioxide emissions, economic growth and energy mix: Empirical evidence from 93 countries. Conference Paper: EcoMod, Prague, Czech Republic. https://ecomod.net/system/files/CO2_Growth_Energy_Mix.pdf.accessed on 01.18.2018. (01 Feb 2022).
- Tamazian, A., & Rao, B. B. (2010). Do economic, financial and institutional developments matter for environmental degradation? Evidence from transitional economies. Energy Economics, 32(1), 137–145.
- Wagner, M. (2008). The carbon Kuznets curve: A cloudy picture emitted by bad econometrics? Resour. Resource and Energy Economics, 30(3), 388–408.
- Wall Street Journal (WSJ), (2022). Russia’s war in Ukraine could have environmental impact that lasts decades. https://www.wsj.com/articles/russias-war-in-ukraine-could-have-environmental-impact-that-lasts-decades-11650801603 (12.May.2022).
- Way, R., Ives, M. C., Mealy, P., & Farmer, J. D. (2022). Empirically grounded technology forecasts and the energy transition. Joule, 6(9), 1–26.
- White, H. (1980). A heteroscedasticity consistent covariance matrix estimator and a direct test of heteroscedasticity. Econometrica, 48(4), 817–818.
- World Meteorological Organization (WMO), (2022). State of the global climate 2021 (WMO-No. 1290). https://library.wmo.int/index.php?lvl=notice_display&id=22080 (20.May.2022).
- Yang, X., Lou, F., Sun, M., Wang, R., & Wang, Y. (2017). Study of the relationship between greenhouse gas emissions and the economic growth of Russia based on the environmental Kuznets curve. Applied Energy, 193, 162–173.
- Zakarya, G. Y., Mostefa, B., Abbesa, S. M., & Seghir, G. M. (2015). Factors Affecting CO2 Emissions in the BRICS countries: A panel data analysis. Procedia Economics and Finance, 26, 114–125.
Appendix
Table A1. Diagnostic tests results for the Gets approach.
Figure A1.