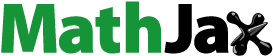
ABSTRACT
This study addresses an open debate in the literature about the direct effects of measures of school quality on workers’ earnings in the labor market. Card and Kureges (1996) argue that the young sample that Betts (Citation1995) uses understates the effects of the measures of school quality on earnings. The main objective of this study is to investigate the effects of the measures of school quality on earnings at older age, using a recent version of the 1979 National Longitudinal Survey of Youth. The replication of Betts’ analysis show that the measures of school quality did not affect earnings when workers were young. However, the estimation shows that the percentage of teachers with a graduate degree significantly affects workers’ prime-age earnings. The findings support Card and Krueger’s claim that the measures of school quality affected workers’ earnings at older age. More importantly, the results reconcile different findings from two strands of studies in the literature using state-level and individual-level data.
1. Introduction
There is an open debate about the effects of measures of school quality on labor market earnings. Betts (Citation1995) investigates the direct effects of measures of public high-school quality – teacher-student ratio, relative teacher salaries, and the percentage of teachers with a master’s degree – on individuals’ earnings between 1979 and 1990 using the 1979 National Longitudinal Survey of Youth (NLSY79) data. He finds no direct effects of the measures of school quality on individuals’ earnings.
Card and Krueger (Citation1996) argue that Betts’ sample is relatively young as the average age of individuals in the sample is only 23.8 years old. They claim that the effects of the measures of school quality on earnings are understated among young individuals. Their claims are based on two central arguments. First, earnings observations of young individuals may not convey complete information about individuals’ earnings potential in the labor market. Many young individuals were not in the labor market because they attended school. Even if they were in the labor market, many worked part-time.
As shown in , there was a considerable share of young individuals in the 1979–1990 sample who attended school. In addition, about 96 percent of individuals who attended schools worked part-time jobs. I also show that there was also a considerable share of young individuals – more than 40 percent – who worked part-time in Betts’ sample. Though not shown in the data, most of the young individuals were also at an early stage of their careers. In this stage, young individuals might have spent quite an amount of time for job training (Lynch, Citation1992) or might have had a higher tendency to change jobs (Topel & Ward, Citation1988).
Second, OLS estimates of the effects of the measures of school quality are biased downward due to a selection issue. Individuals who attended schools with lower measures of quality are less likely to enroll in a college and are more likely to participate in the labor market. On the other hand, individuals who attended schools with higher measures of quality are more likely to enroll in a college, work part-time jobs, and earn relatively lower earnings. I analyze the 1979–1990 NLSY79 sample and find suggestive evidence that indeed the measures of school quality are positively correlated with workers’ decisions to attend school (see online appendix A).
This study addresses Card and Krueger’s claim by analyzing the effects of measures of school quality on earnings using more recent data from the NLSY79. The data provide extended earnings observations, which convey better information about the potential of individuals in the labor market. More importantly, the data allow us to analyze the effects of measures of school quality on earnings at older age and reduce the issue of selection into schooling considerably.
I compiled unbalanced panel data using a public version of the NLSY79 data, including earnings observations from 1979 to 2010, and the Geocode version of the NLSY79 data. For comparability with Betts’ sample, I proceed in three steps. First, I replicate Betts’ sample restriction procedure to approximate his 1979–1990 sample as closely as possible. Second, I use the recent NLSY79 to obtain post-1990 data on earnings, the number of weeks worked, and other individuals’ characteristics. Third, I conducted regression analysis using Betts’ main specification to investigate the effects of measures of school quality on earnings at older age.
Even after closely following Betts’ sample restriction procedure, my sample has more individuals than Betts’ sample. Retrospective revisions of the NLSY79 data post-1990 explain this difference. Nevertheless, I replicate Betts’ findings that the measures of school quality do not affect individuals’ earnings in the analysis of the 1979–1990 sample. However, I find that the percentage of teachers with a master’s degree positively affected individuals’ earnings at 40 or older. The effects of the percentage of teachers with a master’s degree on individuals’ earnings increase over individuals’ life cycles. Finally, I find that the effect of the percentage of teachers with a master’s degree is concentrated among high school graduates. These results are not sensitive to additional essential covariates such as parents’ education, family wealth, and individuals’ age adjusted AFQT scores. These findings are significant as they suggest that selection into better quality schools is not an issue. Overall, the conclusions of this paper support the claim that measures of school quality affect earnings at older age.
The findings in this study reconcile two strands of studies in the literature that fail to reach a consensus regarding the effects of school quality on earnings. In one strand, researchers use measures of school quality observed at the state or aggregate level, such as per-student expenditure, teacher-student ratio, and state average teacher salary (Card & Krueger, Citation1992a, Citation1992b, Citation1994, Citation1996; Nechyba, Citation1990; Rizzuto & Wachtel, Citation1980; Wachtel, Citation1976). The use of aggregate-level measures aims to average out endogenous measures of school quality and errors. These studies find significant effects of measures of school quality on earnings with elasticities ranging from 0.08 to 0.16.
The other strand of the literature investigates the effects on individual earnings of school-level measures of school quality (Betts, Citation1995, Citation2001; Strayer, Citation2002a; Grogger, Citation1996; Tobias & Li, Citation2003). It is argued that an analysis using school-level measures is superior as it eliminates aggregation bias in studies using state or aggregate-level measures of school quality (Betts, Citation1995). These studies find no effect of school quality on earnings, even in a study using predicted earnings of individuals when they were 40–55 years old (Betts, Citation1996).
This study is also related to a recent US study that estimates the effects of measures of school quality, more specifically classroom quality, on earnings (Chetty et al., Citation2011). They found no significant impact of smaller class sizes on earnings, but their estimates suggest that students who were taught by more experienced teachers earned higher than students who were taught by less experienced teachers. This study is also related to studies investigating the effects of school quality on earnings in developing countries (Bedi & Edwards, Citation2002) and among immigrants (Bratsberg & Terrell, Citation2002). These studies find significant effects of measures of school quality on individuals’ earnings.
The rest of the paper is organized as follows. Section 2 discusses the NLSY79 dataset used in the regression analyses. I also discuss a replication of Betts (Citation1995) sample selection procedures, and I compare descriptive statistics of my sample to those in Betts (Citation1995). Section 3 discusses the regression specifications and the estimation results. Finally, I state my conclusion in the last section.
2. Data
2.1. Sample selection procedures
I obtain the data for analyses from the public version of the National Longitudinal Survey of Youth (NLSY79) and the Geocode version of the NLSY79. The data provide a representative sample of 12,686 young individuals, and the data record individuals’ information from 1979 to 2010. Annual interviews were conducted for these individuals through 1994, and biennial interviews were conducted in subsequent periods until 2010. The data provide labor market behaviors and outcomes, education history, family background, and government participation programs. Using the Geocode version of the NLYS79, I obtained individuals’ states of residence from 1979 to 2010 and states of schools individuals attended. I use these variables to calculate the relative salaries of teachers with a B.A. degree and in the regression specification to control for state-specific shocks. More importantly, the states of residence variables allow me to replicate Betts’ estimation. In the Appendix, I list the key variables used in the model specifications in .
Using the NLSY79, I obtained the measures of school quality from a survey of high schools attended by NLSY respondents conducted in 1979. The survey reported many school characteristics such as the number of students enrolled, the number of full-time teachers, the teachers’ educational attainment, academic-related facilities, the curriculum, and the school activities. The main measures of school quality used in the typical regression analyses are the teacher-student ratio, the salaries of teachers with a B.A. degree, and the percentage of teachers with a Master’s degree. I choose these measures for direct comparability with the primary analyses in Betts (Citation1995, Citation1996). In addition, I adjust teachers’ salaries with 1980 per-capita personal income in the state to calculate relative teacher salaries.Footnote1
I conduct a three-step sample restriction procedure to obtain the 1979–2010 sample. First, I follow Betts’ sample selection procedure. Individuals with missing or declining years of schooling between 1979–1990 are excluded from the sample. Individuals in the military subsample, whose measures of school quality are not observed, those who did not attend a public high school, male Hispanics, male Blacks, and females are also excluded from the sample. Lastly, some individuals were not enrolled in a junior high school during the 1979 high-school survey. They were either very young, were currently enrolled in a junior high school at the time of the survey or did not attend a high school in 1979. I exclude these individuals since we do not observe the measures of school quality. From these restriction criteria, I obtained a sample of about 2,077 white-male individuals who attended public high schools in 1979.
Some individuals in this subsample have missing earnings and the number of weeks worked observations. Some individuals also reported zero earnings in the survey. These observation points, but not the individuals, are excluded from the sample in these cases. Some individuals are also missing observations on marital status, residence in SMSA, and state of residence. In these cases, I assume that individuals keep the same status as in the previous period and impute the missing observations using values from the previous period.
Lastly, observations of individuals who lived outside the United States in any given year are also excluded from the sample. These restrictions reduce the 1979–1990 sample to an unbalanced panel consisting of 1,568 individuals. The average number of wage observations per individual in this sample is 10.2. For comparison, the number of individuals in Betts’ sample was 1,134.
compares the number of individuals and observations in Betts’ 1979–1990 sample and those in this study. Ideally, I want to obtain a sample identical to Betts (Citation1995). However, I obtain a significantly higher number of observations for the 1979–1990 sample. This difference can partly be explained by retrospective revisions or imputations by the surveyor to recover information lost in the previous survey years. Specifically, the report on the BLS website states: “from this information and other retrospective information, a longitudinal record spanning from the date of, and to some extent the time preceding, the first interview through the most current interview date can be constructed for each respondent. The longitudinal record is maintained even for respondents who are not interviewed in interim years. Each year’s questionnaire incorporates retrospective questions designed to recover as completely as possible information lost (or incorrectly reported or recorded) during previous survey years.” We also note that there are also several errata for the 1979–2006 data release, which are subsequently corrected by the BLS.
Table 1. A summary of the sample restriction procedure for the 1979–1990 sample.
I present the summary statistics of Betts’ and my 1979–1990 samples in . I find that the averages and standard deviations of most of the variables are close to those in Betts (Citation1995). However, there are a couple of noticeable differences. First, the average number of weeks worked in our sample is higher by about 32 weeks. Second, the average relative salaries of teachers are also higher by 0.20 in our sample. I may have used a different adjustment variable as Betts’s (Citation1995) link is no longer available. Hanushek (Citation1986) documented measures of school quality such as the teacher-student ratio and the percentage of teachers between 1960 and 1980. I find that the averages of these measures calculated from the NLSY79 data are quite close to the ones documented in Hanushek (Citation1986).
Table 2. Summary statistics of the 1979–1990 sample.
Second, I use the recent version of NLSY79 to obtain extended earnings observations between 1991 and 2010. Several variables have missing, or declining values post 1990. I use the following procedures to impute many missing values of the explanatory variables in the subsequent observation periods. First, I assume that individuals did not enroll in school after 30. This is a fair assumption as the data suggests that only about 2.23 percent of individuals aged 30 or older were enrolled in school. These individuals were either enrolled in a college or a graduate program. I then impute missing or declining years of schooling of individuals aged 30 or older with values from the preceding period. Second, I exclude observation periods with missing earnings and the number of weeks worked in each period. I obtain, on average, additional 10.6 periods of observations for everyone in the sample between 1991–2010. The total number of individuals in the unbalanced panel is 1,568, with 26,802 observations.
2.2. Correlations between the measures of school quality
There are several variables in the NLSY79 that can be used to measure school quality. I focus on the teacher-student ratio, the relative teacher salaries, and the percentage of teachers with a master’s degree, which are commonly used as measures of school quality in the literature.
I also analyze the number of students enrolled, the percentage of economically disadvantaged students, the percentage of grade 10 students who dropped out, the percentage of teachers who left the school, the percentage of black students, and the number of books per student to gain a better understanding about the main measures of school quality. Heckman et al. (Citation1996) suggest that it is important to understand how different measures of school quality are correlated with each other. They argue that it is possible for the measures of school quality to be negatively correlated, for example, due to budget constraints.
I report pairwise correlation coefficients between the measures of school quality in . There is a positive correlation between the percentage of teachers with a master’s degree and the relative salaries of teachers with a B.A. degree. This finding suggests that, on average, high schools that employed a higher percentage of teachers with a master’s degree also paid higher salaries to teachers with a B.A. degree. Thus, high schools might have increased the classroom size due to limited budgets, leading to a negative correlation between the relative salaries and the teacher-student ratio. Schools with a higher percentage of teachers with a master’s degree tend to have a larger enrollment, leading to a negative correlation between the percentage of teachers with a master’s degree and the teacher-student ratio.
Table 3. Correlation coefficients of the measures of school quality.
The results suggest that a higher percentage of teachers with a master’s degree seems to be an indicator of better school quality. For instance, the percentage of teachers with a master’s degree is negatively correlated with the percentage of disadvantaged students. It is also negatively correlated with the percentage of grade 10 students who dropped out and the percentage of teachers who left the school. Overall, the results show that the teacher-student ratio, relative teacher salaries, and percentage of teachers with a master’s degree do not indicate school quality improvement. For this reason, I do not combine the main measures of school quality into a single index.
In the next section, I estimate regression models for different age ranges to analyze the effects of measures of school quality on earnings using the extended earning observations. First, I focus on the teacher-student ratio, the relative teacher salaries, and the percentage of teachers with a master’s degree as the main measures of school quality. Next, the extended earning observations allow me to estimate the effects of the measures of school quality when individuals were older. Specifically, I estimate the model in two age ranges: when the individuals were 17–32 and when the individuals were 40 or older. These age ranges allow me to compare estimation results with Betts (Citation1995, Citation1996).
I keep the same group of individuals for each age range to avoid a composition effect. Thus, I exclude individuals from the sample if the individuals have no earnings observation in one or any of the two age ranges. The total number of unique individuals with earning observations in the two age ranges is 1,038. For comparison, the number of individuals observed in each age range is 1,568 and 1,038. Regressions with a different group of individuals yield similar results.
3. Results and discussion
3.1. The effects of the measures of school quality on earnings
The main objective of the analysis in this section is to replicate Betts’ estimation and investigate the effects of the measures of school quality on earnings at older age. I start by replicating Betts’ estimations using the 1979–1990 NLSY79 sample, in which individuals were 17–32 years old. For the estimation, I use Betts’ model specification:
Specification 2
where i indicates an individual in the sample at time t. As discussed in the previous section, I estimate this model using the sample of observed individuals in the two age ranges.
Note that the data include the earnings of individuals who worked. Thus, I acknowledge that my analyses may be subject to the sample selection issues (Heckman, Citation1979; Vella, Citation1998). For example, suppose that individuals with a low measure of school quality are more likely to be out of work in a given time. In addition, suppose that these individuals have lower reservation wages. Assuming a positive effect of the measure of school quality, the estimated effect will be lower than the true effect of school quality. If indeed that is the case in general, then the estimated effect of school quality using only individuals who worked will produce a lower bound on the effect of school quality.
The left-hand side variable is a log of weekly earnings. However, results are similar in regressions with a log of hourly earnings. The regressors are the years of schooling (S), the measures of school quality (Q), a quartic function of experience (E), and a vector of control variables (X). The measure of labor market experience at a given period is the sum of the number of weeks worked in previous periods. The control variables include eight dummy variables for residences in the census regions, interaction terms between the census region dummies and the years of schooling, a dummy variable for residence in SMSA, and a dummy for the marital status and year dummies. Betts’s (Citation1995) uses heteroscedasticity-robust standard error estimators for pooled OLS regressions. However, the error terms are likely to be correlated over time for a given individual owing to the data structure. For this reason, I consider an additional baseline specification with standard errors clustered at the individual level (Cameron & Miller, Citation2015; Stock & Watson, Citation2008).
I first estimate the model using the 1979–1990 sample to compare the results with Betts’. I report my estimates and Betts’ estimates in . I present Betts’ estimates in Column 1, and I present my estimates in Column 2 and Column 3. Betts’ estimates show that measures of school quality do not affect individuals’ earnings. My estimates in Column 2 show that the teacher-student ratio positively affects individuals’ earnings. However, the significance goes away once I cluster the standard errors at the individual level. In all model specifications, the percentage of teachers with a master’s degree and the relative teacher salaries do not affect individuals’ earnings.
Table 4. The estimated effects of measures of school quality on earnings.
We can observe that the estimated standard errors are higher in the specification using the clustered standard errors. This increase is expected in time-ordered earnings observations as the error terms are positively correlated among individuals (Cameron & Miller, Citation2015). For this reason, I cluster the standard errors at the individual level in subsequent specifications to control for the correlations of the error terms across time. I acknowledge that the point estimates for the measures of school quality are different than those in Betts’s (Citation1995). These differences are not unexpected because the regression sample in Betts’ and the sample in this study are different. Nevertheless, I obtain similar qualitative results to Betts’ that the measures of school quality do not affect individuals’ earnings.
To analyze the effects of the measures of school quality on earnings at older age, I estimate Specification 2 at different age ranges: 17–32 and beyond 40. Then, I estimate the model using the same sample to avoid composition effects and cluster the standard errors at the individual level. Finally, I present the estimates for the two age ranges in Column 3 and Column 5 of , respectively. The estimates in Column 3 of show that no measures of school quality appear to be significantly different from zero when individuals were 17–32. However, the estimates in Column 5 of show that a higher percentage of teachers with a Master’s degree led to significantly higher earnings at older age. The point estimate suggests that a one-standard-deviation increase in the percentage of teachers with a master’s degree increases the prime-age earnings by 0.07 standard deviation. For comparison, a one-standard-deviation increase in the years of schooling increases the prime-age earnings by 0.23 standard deviation.
I then analyze whether the marginal effects of the measures of school quality vary with the years of schooling. Finally, following Betts’s (Citation1995), I include interaction terms between the years of schooling and the measures of school quality:
Specification 3
where and
are schooling, and school quality variables centered at their means, respectively. The centering of these variables allows us to interpret the estimated parameters conditional on the averages of the interacted variables. For instance, the estimated parameters
capture the marginal effects of the measures of school quality for individuals with average years of schooling. This simple manipulation also allows us to compare these estimates with estimates using the model specification in Specification 2.
I present the marginal effects of the measures of school quality on earnings conditional on the average years of schooling in Columns 4 and 6 of . Note that the average years of schooling is about 12.8, which implies that on average the individuals are high-school graduates. There are no significant interaction effects between years of schooling and the measures of school quality, and the inclusion of the interaction terms does not improve the model’s fit. Nevertheless, the result is similar to one in the baseline model: the percentage of teachers with a master’s degree is significant only at older age. This result is consistent with findings in Wachtel (Citation1976), who used district-level per-student expenditure as a measure of school quality. He finds that the estimated parameter of the per-student expenditure is larger at older age.
I re-estimate Specification 3 using different sets of controls to investigate whether the effects of the measures of school quality are robust to addition of important variables such as parents’ level of education, family wealth, and a proxy of ability (AFQT score). I also test the robustness of the estimates by adding state fixed effects or state by year fixed effects in the model specification. For these estimations, I use the sample of individuals at older age, and I present the results in . These sensitivity analyses show that the effects of the percentage of teachers with a master’s degree are quite robust.
Table 5. The estimated effects of the measures of school quality on earnings.
So far, I focus on the teacher-student ratio, relative teacher salaries, and percentage of teachers with a master’s degree as the main measures of school quality. However, as shown in Section 3.2, these measures of school quality are correlated with other quality metrics such as the percentage of black students, the percentage of poor students, the percentage of teachers who left the school, the percentage of grade 10 dropouts, and library books per students. I investigate whether the estimated effects of the main measures of school quality are robust by augmenting Specification 2 with one quality metric at a time. I present the results of the estimations in . We can observe that the estimated coefficients of the percentage of teachers with a master’s degree are not sensitive to the addition of other quality metrics. In all specifications, we reject the null that the percentage of teachers with a master’s degree does not affect individuals’ earnings. It is interesting to note that the percentage of economically disadvantaged students has a negative effect on individuals’ earnings. The magnitude of its effect is similar to that of the percentage of teachers with a master’s degree.
Table 6. The estimated effects of the measures of school quality on earnings.
3.2. The effects of the measures of school quality over the life cycle
The results so far suggest that the effect of the percentage of teachers with a master’s degree is higher at older age. Thus, the logical next step is to estimate the effects of the measures of school quality over individuals’ life cycles. First, I estimate a model with an interaction between schooling and age and an interaction between the percentage of teachers with a master’s degree and age:
I report the results of the regressions in . I find a significant interaction effect between schooling and age and a significant interaction effect between the percentage of teachers with a master’s degree and age. These estimates suggest that the effect of schooling and the effect on the percentage of teachers with a master’s degree increases with age. However, I find no interaction effect between age and the other measures of school quality. This suggests that the teacher-student ratio and the relative salaries of teachers’ effects on earnings are constant over individuals’ life cycles.
Table 7. The effects of schooling and the measures of school quality over the life cycle.
It is important to obtain the effects of the measures of school quality at a certain age and to investigate whether the effects are significant or not. I calculate the partial effects of schooling and partial effects of the percentage of teachers with a master’s degree at each age point and the corresponding standard errors and 95 percent confidence intervals. I plot the partial effects of schooling and the percentage of teachers with a master’s degree and their corresponding confidence intervals in . First, we can observe that the estimated partial effects increase monotonically with age. The percentage of teachers with a master’s degree did not affect earnings when individuals were below 30. However, the percentage of teachers with a master’s degree has positive and significant effects on earnings when individuals were 30 or older. The pattern of the marginal effects of the percentage of teachers with a master’s degree is similar to the pattern of marginal effects of schooling. I also estimate the effects of the teacher-student ratio on earnings over the life cycle of individuals. The estimated marginal effects are positive at older age. However, they are not statistically significant. The estimated marginal effects of relative teacher salaries on earnings are zero.Footnote2
Figure 2. Marginal effects of the years of schooling and the percentage of teachers with a master’s degree by age.

The fact that the percentage of teachers with a master’s degree is significant at older age is important in the literature. A direct comparison of the results in this study is the results in Betts (Citation1996), who investigated the effects of the measures of school quality on earnings at ages 40–55. Betts (Citation1996) uses the earnings of individuals aged 40–55 in the 1980 Census to predict NLSY79 individuals’ earnings when they are 40–55 years old. In his study, the estimated parameter of the percentage of teachers with a master’s degree is negative, although it is statistically not different from zero.
I argue that there are two explanations for the difference. First, the 1980 Census is cross-sectional data. Hence, we can only observe one earning observation for each individual. One needs different individuals to construct a series of earnings data for ages 40–55. However, each individual has different observed and unobserved characteristics, which may significantly affect earnings in different ways. Second, the earnings obtained from the 1980 Census cannot capture the actual state of the economy and technological progress, which significantly affect occupation-specific earnings and earnings growth. Therefore, earnings data for a specific occupation in the 1980 Census are not comparable to the post-1990 earnings data in the NLSY79.
3.3. The effects of the measures of school quality by years of schooling completed
The results show that the percentage of teachers with a master’s degree indeed affects earnings, and the effects are stronger at older age. In this section, I analyze whether the effects of the measures of school quality differ by years of schooling completed. I identify the highest years of schooling that everyone completed. I then estimate the model in Specification 2 by the highest years of schooling completed: less than high school, high school, some college, four-year college, and post-graduate. For each schooling group, I estimate the model using the sample when individuals were 17–32 years old and the sample when individuals were 40 years or older. Thus, I have the same group of individuals for each schooling group in both samples.
I report the results in . Consistent with the previous results, the measures of school quality have no effects on earnings when individuals were young irrespective of the schooling group. On the other hand, the percentage of teachers with a master’s degree affects earnings at older age but only among individuals who did not finish high school and individuals who completed high school. This is an interesting finding because individuals with at most a high school diploma attended high schools with a relatively lower share of teachers with a master’s degree. For example, the average percentage of teachers with a master’s degree for individuals with at most a high school degree is 42.8 percent. However, the average for individuals with a 4-year college degree is 49.6 percent. This result suggests that attending a better-quality school matter for individuals who did not attend college.
Table 8. The effects of the measures of school quality by highest years of schooling completed.
3.4. Does return to schooling vary by measures of school quality?
I now investigate whether the marginal effects of the return to schooling vary with the measures of school quality. This analysis is important as the state-level studies in the literature. For example, Card and Krueger (Citation1992a) find that the measures of school quality have significant relationships with the return to education. For this analysis, I categorize each measure of school quality into quartiles and estimate Specification 2 for each quartile. I estimate the model using the sample when individuals were 40 years or older. presents the estimates of the return to schooling for each quartile of the measures of school quality. The results show that individuals who attended schools with the lowest teacher-student ratio or individuals who attended schools with the highest percentage of teachers with a master’s degree earned higher returns to schooling. One of the explanations is that the percentage of teachers with a master’s degree is negatively correlated with the teacher-student ratio.
Table 9. Return to schooling by the measures of school quality.
4. Conclusion
There is an open debate in the literature that looks at the effects of the measures of school quality on labor market earnings. Studies using state or aggregate-level measures of school quality such as teacher-student ratio and relative teacher wage find positive effects on labor market earnings. On the other hand, studies using school-level measures of school quality, such as Betts’s (Citation1995), find no effect of the measures of school quality on labor market earnings. Card and Krueger (Citation1996) argue that Betts’ results are driven by the use of a relatively young sample. A follow-up paper by Betts (Citation1996) addresses this criticism by using the 1980 Census to predict the prime-age earnings of individuals in the NLSY79 sample. He finds no significant effects of the measures of school quality on earnings. While this study supports Betts’s (Citation1995), I argue that there are many drawbacks to the wage-matching method used in this study.
This study reopens this debate by analyzing the direct effects of the measures of school quality on earnings at older age. The more recent NLSY79 dataset provides an opportunity to analyze the effects of the measures of school quality when individuals are 40 or older. Specifically, the dataset allows a comparison of the effects of the measures of school quality when individuals were young and when they were older. This analysis is essential to address Card and Krueger’s claim that the use of a relatively young sample in Betts’s (Citation1995) understates the effects of the measures of school quality.
I find strong evidence that the measures of school quality affect earnings at older age. The estimates are quite consistent across model specifications even after controlling family characteristics and a measure of individuals’ ability. The point estimates suggest that a one-standard-deviation increase in the percentage of teachers with a master’s degree increases the prime-age earnings of those with the average years of schooling by 0.06 to 0.07 standard deviation. I also find that the estimated effects of the percentage of teachers with a master’s degree decrease with the years of schooling. The effects are significant among individuals who completed high school or one year of college.
The results of this study have strong implications for the literature. First, the results support Card and Krueger’s claim that the measures of school quality affect earnings only at older age. Second, the results in this study reconcile the findings between studies that used state- and school-level data. Finally, together with state-level studies, the results in this study conclude that the measures of school quality affect individuals’ earnings in the labor market.
Ethical clearance
The study protocol has been reviewed and approved by the University of Pittsburgh Institutional Review Board with ID PRO14030603.
Acknowledgments
I thank Daniel Coen-Pirani, Marla Ripoll, Daniel Berkowitz, Sera Linardi, Lindsay Page, and the University of Pittsburgh macroeconomics brown bag seminar participants for their valuable comments and inputs.
Disclosure statement
No potential conflict of interest was reported by the author(s).
Additional information
Notes on contributors
Gumilang Aryo Sahadewo
Gumilang Aryo Sahadewo is an Assistant Professor at the Department of Economics Universitas Gadjah Mada. His research interests are economics of education, labor economics, the economics of tobacco control, and experimental economics.
Notes
1 I obtain per-capita personal income data from Table SA1–3 in the BEA Regional Economic Accounts website.
2 See Figure A1 for the marginal effects of teacher-student ratio and Figure A2 for the marginal effects of relative teacher salaries.
References
- Bedi, A. S., & Edwards, J. H. Y. (2002). The impact of school quality on earnings and educational returns—evidence from a low-income country. Journal of Development Economics, 68(1), 157–20. https://doi.org/10.1016/S0304-3878(02)00010-X
- Betts, J. R. (1995). Does school quality matter? evidence from the national longitudinal survey of youth. The Review of Economics and Statistics, 77(2), 231–250. https://doi.org/10.2307/2109862
- Betts, J. R. (1996). Do school resources matter only for older workers? The Review of Economics and Statistics, 78(4), 638–652. https://doi.org/10.2307/2109951
- Betts, J. R. (2001). The impact of school resources on women’s earnings and educational attainment: Findings from the national longitudinal survey of young women. Journal of Labor Economics, 19(3), 635–657. https://doi.org/10.1086/322076
- Bratsberg, B., & Terrell, D. (2002). School quality and returns to education of US immigrants. Economic Inquiry, 40(2), 177–198. https://doi.org/10.1093/ei/40.2.177
- Cameron, A. C., & Miller, D. L. (2015). A practitioner’s guide to cluster-robust inference. The Journal of Human Resources, 50(2), 317–372. https://doi.org/10.3368/jhr.50.2.317
- Card, D., & Krueger, A. B. (1992a). Does school quality matter? returns to education and the characteristics of public schools in the United States. The Journal of Political Economy, 100(1), Art. 3358. https://doi.org/10.1086/261805
- Card, D., & Krueger, A. B. (1992b). School quality and black-white relative earnings: A direct assessment. The Quarterly Journal of Economics, 107(1), Art. 3713. https://doi.org/10.2307/2118326
- Card, D., & Krueger, A. B. (1994). The economic return to school quality: A partial survey (No. 334; Industrial Relations Section).
- Card, D., & Krueger, A. B. (1996). Labor market effects of school quality: Theory and evidence (No. 5450; NBER Working Paper Series).
- Chetty, R., Friedman, J. N., Hilger, N., Saez, E., Schanzenbach, D. W., & Yagan, D. (2011). How does your kindergarten classroom affect your earnings? evidence from project STAR. The Quarterly Journal of Economics, 126(4), 1593–1660. https://doi.org/10.1093/qje/qjr041
- Colin Cameron, A., & Miller, D. L. (2015). A practitioner’s guide to cluster- robust inference. The Journal of Human Resources, 50(2), 317–372. https://doi.org/10.3368/jhr.50.2.317
- Grogger, J. (1996). Does school quality explain the recent black/white wage trend? Journal of Labor Economics, 14(2), 231–253. https://doi.org/10.1086/209810
- Hanushek, E. A. (1986). The economics of schooling: Production and efficiency in public schools. Journal of Economic Literature, 24(3), 1141–1177.
- Heckman, J. J. (1979). Sample selection bias as a specification error. Econometrica: Journal of the Econometric Society, 47(1), 153–161. https://doi.org/10.2307/1912352
- Heckman, J., Layne-Farrar, A., & Todd, P. (1996). Human capital pricing equations with an application to estimating the effect of schooling quality on earnings. The Review of Economics and Statistics, 78(4), 562–610. https://doi.org/10.2307/2109948
- Lynch, L. M. (1992). Private-sector training and the earnings of young workers. The American Economic Review, 82(1), 299–312.
- Nechyba, T. J. (1990). The southern wage gap, human capital and the quality of education. Southern Economic Journal, 57(2), 308–322. https://doi.org/10.2307/1060612
- Rizzuto, R., & Wachtel, P. (1980). Further evidence on the returns to school quality. The Journal of Human Resources, 15(2), 240–254. https://doi.org/10.2307/145333
- Stock, J. H., & Watson, M. W. (2008). Heteroskedasticity-robust standard errors for fixed effects panel data regression. Econometrica, 76(1), 155–174. https://doi.org/10.1111/j.0012-9682.2008.00821.x
- Strayer, W. (2002). The returns to school quality: college choice and earnings. Journal of Labor Economics, 20(3), 475–503. https://doi.org/10.1086/339674
- Tobias, J. L., & Li, M. (2003). A finite-sample hierarchical analysis of wage variation across public high schools: Evidence from the NLSY and high school and beyond. Journal of Applied Econometrics, 18(3), 315–336. https://doi.org/10.1002/jae.696
- Topel, R. H., & Ward, M. P. (1988). Job mobility and the careers of young men. National Bureau of Economic Research Cambridge.
- Vella, F. (1998). Estimating models with sample selection bias: A survey. The Journal of Human Resources, 33(1), 127–169. https://doi.org/10.2307/146317
- Wachtel, P. (1976). The effect on earnings of school and college investment expenditures. The Review of Economics and Statistics, 58(3), 326–6535. https://doi.org/10.2307/1924954
Appendix
Figure A1. Marginal effects of teacher-student ratio by age.

Figure A2. Marginal effects of teachers’ salary by age.

Table A1. Key variables from the NLSY79.