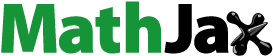
ABSTRACT
We create a simulated financial market and examine the effect of different levels of active and passive investment on fundamental market efficiency. In our simulated market, active, passive, and random investors interact with each other through issuing orders. Active and passive investors select their portfolio weights by optimizing Markowitz-based utility functions. We find that higher fractions of active investment within a market lead to an increased fundamental market efficiency. The marginal increase in fundamental market efficiency per additional active investor is lower in markets with higher levels of active investment. Furthermore, we find that a large fraction of passive investors within a market may facilitate technical price bubbles, resulting in market failure. By examining the effect of specific parameters on market outcomes, we find that that lower transaction costs, lower individual forecasting errors of active investors, and less restrictive portfolio constraints tend to increase fundamental market efficiency in the market.
1. Introduction
Passive investing has steadily grown relative to active investing over the last decades (Anadu et al., Citation2020; Blitz, Citation2014) and is expected to overtake active investing by 2026 in the US equity market (Seyffart, Citation2021). In the US domestic equity-fund market, passive vehicles have already overtaken active ones due to the large number of passive funds tracking the S&P 500 index (Seyffart, Citation2021). Passive investors select the portfolio weights of assets within the risky fraction of their portfolio based on asset market capitalization (Sharpe, Citation1991). Therefore, passive investors only need to decide about the fraction they want to invest in the risk-free asset and the risky market portfolio for each period (Pedersen, Citation2018). In contrast to passive investors, active investors usually trade based on the assessment of asset mispricings. As these assessments tend to change frequently, active investors generally trade more often than passive investors (Sharpe, Citation1991).
For instance, the fraction of actively managed mutual funds and ETFs relative to the total fund market in the US has decreased from 81% in 2010 to 60% in 2020 (ICI, Citation2021).
Additionally, active shares and fees of active mutual funds have fallen (Cremers & Petajisto, Citation2009; ICI, Citation2020; Stambaugh, Citation2014), resulting in active investment management becoming more similar to passive investment management. Due to higher expense ratios of active management, passive investment management tends to outperform active investment management after fees (Carhart, Citation1997; Fama & French, Citation2010). However, there is a need for active investment in financial markets since the security analysis of active investors connects market prices to fundamental asset values and keeps financial markets efficient (Blitz, Citation2014; Pedersen, Citation2018). Therefore, the shift from active to passive investment may reduce the information contained in individual asset prices (Sushko & Turner, Citation2018) and lead to higher systematic market risks (Anadu et al., Citation2020). As the shift from active to passive investment constitutes a major trend for global financial markets, it is essential to examine it further and analyze its implications.
Against the backdrop of the rise of passive investment, it is crucial to understand the implications of this significant trend for financial markets and their participants. Therefore, financial researchers have begun to empirically examine different aspects of the shift from active to passive investment. These empirical analyses suggest that this shift may have reduced the information contained in individual asset prices (Sushko & Turner, Citation2018) and led to higher systematic market risks (Anadu et al., Citation2020). In this work, we want to shed more light on how different forms of investment affect financial markets. Concretely, we address the research question:
RQ:
How do different levels of active and passive investment affect fundamental price efficiency?
By answering this research question, we evaluate the implications of the ongoing shift from active to passive investing. Furthermore, we investigate different market conditions that may mitigate potential adverse effects on market efficiency.
Besides shifts across investment types, specific investment forms are also evolving. Consistent with findings that machine learning models can improve financial market forecasting (Fischer & Krauss, Citation2018; Gu et al., Citation2020; Rasekhschaffe & Jones, Citation2019), a large proportion of professional active investors have adopted machine learning models for trading and portfolio management (BarclayHedge, Citation2018; Petropoulos et al., Citation2022). Given this trend, it is essential to assess the impact of differences in the accuracy of active investors’ market forecasts on underlying market efficiency.
To answer the stated research question, we create a simulated financial market and closely examine the impact of different investor compositions and other market parameters on fundamental market efficiency. Different stylized investors interact with each other in a simulated market through issuing orders, which are matched via common continuous double auctions. Thereby, market prices are solely set by the investor behavior without external interventions. While there exist different interpretations and levels of market efficiency in the literature (e.g., see (Fama, Citation1970, Citation1991)), in this paper, we focus on market efficiency in terms of the deviation between market prices and fundamental asset values. In this work, we denote this form of market efficiency with the term fundamental market efficiency.
In the financial literature, so-called noisy rational expectation models have been applied to study similar research settings as simulated financial market models (Blume et al., Citation1994; D. W. Diamond & Verrecchia, Citation1981; Grossman & Stiglitz, Citation1980; Milgrom & Stokey, Citation1982), for which these models generally find closed-form solutions. However, these models differ from our study regarding the exogeneity and endogeneity of market parameters. Furthermore, the theoretical models rely on many assumptions and restrictions to arrive at their equilibriums, such as market clearing (Brown & Jennings, Citation1989; D. W. Diamond & Verrecchia, Citation1981), limiting cases (Brown & Jennings, Citation1989), or a single period (D. W. Diamond & Verrecchia, Citation1981). Thus, we use a simulation approach to find results for scenarios that rely on fewer assumptions. At the same time, this approach allows us to combine different real-world settings into a single model instead of creating multiple models to examine different market enviornments to ensure a closed-form solution. Hence, our simulation approach enables us to generate novel insights, as well as to verify assumptions and results made in different noisy rational expectation models (e.g., the assumption of a higher information level in the market for a higher share of active investors Grossman and Stiglitz (Citation1980)).
Our paper has two main contributions. First, we show how different investor compositions affect fundamental market efficiency. In doing so, we find that larger fractions of active investment and lower fractions of passive investment within a market results in higher market efficiency. Thereby, the marginal increase in market efficiency is lower for an additional active investor if there is a high level of active investment within a market. We find that large portions of passive investment within a market may facilitate price bubbles and lead to market failure. Second, we evaluate the impact of different market parameters, for instance market frictions and individual target price forecasting errors, on fundamental market efficiency to increase the robustness of our results in the context of constantly evolving financial markets. We find that market frictions in the form of higher transaction costs and stricter portfolio constraints reduce fundamental market efficiency. Additionally, we find that a lower level of risk aversion of individual investors, tends to increase fundamental market efficiency. Lastly, we show that less volatile individual target price forecasts of active investors translate into higher fundamental efficiency of market prices.
2. Related work
2.1. Implications of active and passive investment
Anadu et al. (Citation2020) empirically investigate the implications of the past shift from active to passive investment strategies for financial stability. They find that this shift may decrease liquidity transformation risks but increase market volatility. Furthermore, Anadu et al. (Citation2020) present evidence indicating that passive investment facilitates the co-movement of assets.
Pedersen (Citation2018) challenges William Sharpe’s equality, which states that “before costs, the return on the average actively managed dollar will equal the return on the average passively managed dollar” (Sharpe, Citation1991). Pedersen (Citation2018) argues that the assumption of a never-changing market portfolio does not hold for real-world financial markets and presents ways how active traders can generate profits. For instance, adequately informed active traders could outperform non-informed active traders, influenced by cognitive biases. Additionally, only active investors can identify and participate in profitable IPOs. Furthermore, passive investors need to trade when the composition of their respective benchmark index changes, which can be anticipated and exploited by active investors. Pedersen (Citation2018) concludes that there exists an equilibrium level of active and passive investment that keeps the market close to but not perfectly efficient.
Sushko and Turner (Citation2018) examine the effect of the shift from active to passive investing on security markets. They find that active mutual funds are subject to persistent outflows in stress periods, while the flows of passive mutual funds remain relatively stable. Additionally, the authors find a positive relationship between the weight of a company in the Bank of America Merrill Lynch Global Broad Market Corporate Index and the company’s leverage factor. According to Sushko and Turner (Citation2018), a further expansion of the passive fund management industry may have two main consequences: First, security-specific information could decrease in prices because passive fund managers do not use this information for their valuations. Second, security pricing can be influenced by an increasing number of passive managers selling or buying the entire set of index constituents by tracking an index. These transactions could lead to the prices of the assets in the index to be subject to higher co-movement.
Schwert (Citation2003) shows that market anomalies published in the academic financial literature are integrated into trading strategies and hence disappear after publication. Therefore, he states that research on market anomalies makes financial markets more efficient.
Sullivan and Xiong (Citation2012) analyze the effects of increased index trading. The authors find that the rising fraction of passively managed equity indices leads to an increased systematic risk of equity markets. The higher amount of passive investing amplifies the trading commonality of the index assets caused by interactions between market participants. This commonality increases systematic fluctuations in aggregate demand, which have a fundamental impact on markets and portfolio compositions. Moreover, Sullivan and Xiong (Citation2012) state that more equity index trading leads to increasing stock return correlations. Additionally, the authors find that equity betas have risen and converged between 1997 and 2010.
Appel et al. (Citation2019) empirically analyze the effect of passive investors on investment strategies and investment results of active investors. The authors find that if passive mutual funds own the shares of a company to a more considerable degree, activists are more likely to be represented on the company’s board. Moreover, they show that a high fraction of passive traders owning a company’s stocks is positively related to the number of proxy fights and settlements of a company. Finally, Appel et al. (Citation2019) concludes that free-rider problems are weakened by the increasing number of passive institutional investors.
Qin and Singal (Citation2015) examine the external effects of indexing on stock price’s efficiency. By analyzing a sample of stocks, they find that a higher degree of indexing is related to less efficient stock prices. Concretely, Qin and Singal (Citation2015) find a higher post-earnings-announcement drift and a more significant random walk deviation of stock prices for higher degrees of indexing. Qin and Singal (Citation2015) suggest that price efficiency is decreased by indexing and passive trading, as passive investing lowers the appeal of information collection and arbitrage.
In his work, Blitz (Citation2014) addresses the shortcomings of passive investment strategies. First, he outlines that passive investment constitutes free-riding, as passive investors rely on active investors to keep markets efficient. Blitz (Citation2014) states that the relationship between fundamentals and asset prices would be destroyed if there were only passive investors. Moreover, he describes specific security characteristics, which have been shown to cause a weak asset performance. Passive investors cannot avoid investing in these securities when they are present in the respective passively replicated index.
Belasco et al. (Citation2012) examine how passive investing affects corporate valuations by analyzing the relation between index fund money flow and company valuations. They find that valuations of index constituents are positively correlated with index fund money flow, whereas valuations of non-constituents are not. Moreover, they find that this impact does not directly divert since money flow also impacts valuations the following month after the flow. Belasco et al. (Citation2012) conclude that mispricing caused by index fund investing could reduce stock market efficiency and manipulate how investors evaluate the performance of actively managed funds.
French (Citation2008) analyzes the cost of active investing and finds that active investors pay 0.67% of the total market value of a stock for search costs. Moreover, he shows that society faces capitalized price discovery costs, which can amount to up to ten percent of a stock’s market capitalization. French (Citation2008) finds that between the years 1980 and 2006, the average annual return of a typical active investor could have risen by 67 basis points if the investor switched from active to passive investing.
2.2. Financial market simulation
Ponta et al. (Citation2011) simulate an artificial stock market that consists of zero-intelligence traders and analyze the effect of dividend and external cash flows on the market outcome. Traders randomly allocate a fraction of their wealth into different stocks, whereby asset prices are determined by aggregating demand and supply. Despite using zero-intelligence traders, the authors can reproduce several stylized facts (e.g., volatility clustering) in the resulting price series. The stylized facts reproduction does not depend on dividend payments and external cash flows.
Cocco et al. (Citation2017) simulate an artificial agent-based cryptocurrency market in which heterogeneous agents trade bitcoins. The authors model several market characteristics of the bitcoin market (e.g., bitcoin mining and investor distribution). Cocco et al. (Citation2017) examine whether their simulated market exhibits stylized facts known from real-world financial markets. They define two types of agents, namely momentum-based technical traders and random traders, whose orders are matched via limit order books. The authors find that the price series data of the artificial market shows three stylized facts of real financial time-series data: Unit-root property, fat tails, and volatility clustering.
Bertella et al. (Citation2014) use an agent-based artificial market to analyze the effect of (over-)confidence (Kahneman & Riepe, Citation1998) on market outcomes. In their model, agents can either be fundamental or technical traders. Fundamental traders estimate future asset values using the Gordon dividend growth model, whereas the technical traders use a moving average with different time horizons to estimate asset values. Both stylized agent types aim to maximize a utility function based on constant absolute risk aversion. Prices are calculated based on a market impact function based on Farmer and Joshi (Citation2002) for varying levels of the agents’ market confidence. The authors find that higher confidence levels are positively correlated with investment returns. However, market confidence also has negative effects, leading to increased price volatility.
Benhammada et al. (Citation2017) implement an artificial stock market based on continuous double auctions to identify sources of bubbles and crashes in financial markets. The modeled agents can be grouped into fundamental, noise, technical, and hybrid traders. Noise traders issue orders randomly, which increases market liquidity. Fundamental traders issue orders based on a function to calculate the fundamental value, while technical traders issue their orders based on the direction of the forecasted price and the market liquidity. Hybrid traders change their stylized trading behavior between technical and fundamental trading based on the market state. Benhammada et al. (Citation2017) find that prices deviate from fundamental values if technical traders dominate the artificial market. However, these markets lack characteristics of financial price bubbles in the real world. The price bubbles become more realistic when hybrid traders are predominant within the market. The authors find no evidence for the existence of market bubbles in the case that fundamental traders dominate the market.
Goykhman (Citation2017) implements a sentiment-driven artificial financial market and examines how the wealth of different agents develops over time. In the market, agents do not maximize a utility function but issue orders depending on three time-series processes: buy/sell imbalance, jump volatility, and trading intensity. These orders are matched via limit order books. The author finds that non-trivial volatility sentiment processes result in large stock returns different from the log-normal distribution. The use of non-trivial buy/sell sentiments leads to predictable price trends. Furthermore, Goykhman (Citation2017) finds that the results do not depend on the initial wealth distribution among the agents, as the wealth is distributed very quickly with a power-law Pareto tail among the agents in any case.
Katahira et al. (Citation2019) create an agent-based artificial asset market and analyze stylized market facts. They model agents as technical traders and calculate asset prices for each period from the aggregated excess demand, assuming sufficient market liquidity. They find several stylized facts in the time-series data of resulting market price returns (e.g., heavy tails and conditional heavy tails) but cannot reproduce the gain/loss asymmetry.
Khashanah and Alsulaiman (Citation2017) construct an agent-based artificial market in which agents can trade between a risk-free asset and a risky asset. They aim to identify the causes of market instability depending on the information flow between the agents. Agents are randomly selected to adopt four different strategies: random, fundamental, momentum/technical, and adaptive trading (using neural networks to predict asset prices). Thereby all but the technical traders optimize a utility function based on Markowitz (Citation1952). Asset prices are calculated based on the bid and ask prices, but the underlying market mechanism does not utilize order books. The authors simulate jump events that affect the market and test whether agents can react appropriately to these jumps. They find that the outputs of the scenarios depend on the market state regarding information awareness. In states of systematic ignorance, mean volatility and the volatility index are lowest. The volatility index and fear index increase for a larger number of hubs or hermits in a network.
Moiseev and Akhmadeev (Citation2017) implement an agent-based artificial stock market and examine resulting wealth distribution and price movements. In their simulation, agents randomly issue orders, and the turnover maximization criterion determines prices. The authors find that wealth distribution becomes increasingly positively skewed over time. The behavior patterns of the agents influence the speed of inequality growth. Thereby, the inequality of the wealth distribution grows the fastest in a setting in which most agents are issuing bid orders, followed by a setting in which most agents issue sell orders. The wealth inequality grows the slowest when there is a balance between buy and sell orders in the market.
Ponta and Cincotti (Citation2018) simulate an agent-based artificial stock market to examine the influence of agents’ networks on the structure of the market. Agents decide about issuing orders based on their vision of the market trend and the average sentiment towards all assets. The resulting supply and demand curves are matched to obtain market prices. The authors find an intrinsic structural resilience of the stock market. Moreover, the inclusion of the network between agents leads to a higher number of stylized facts reproduced in the artificial market.
Wu et al. (Citation2018) implement an agent-based stock market in order to investigate the stock price dynamics in agent networks. Agents are fundamental or technical traders and aim to maximize their individual utility function, which is based on constant absolute risk aversion. All agents are connected via networks, which allows them to collect information from their neighbors. Orders are matched via continuous double auctions. The authors find that small-world networks lead to a decreasing kurtosis of returns. The return kurtosis is lower for a higher reconnection probability between nodes. Wu et al. (Citation2018) find that changing the network structures does not affect the standard deviation of returns. Finally, the authors conclude that the level of information efficiency has a manifold impact on the market outcomes for diverse network structures.
Vanfossan et al. (Citation2020) construct an artificial stock market to evaluate the success of different trading strategies. They model agents as investors and mutual funds, whereby investors have a lower buying power but are more frequent than mutual funds. The agents are connected via media networks and social media. Based on the network and their strategy, investors issue buy or sell orders, which are matched through continuous double auctions. Finally, the authors calculate the mean returns for each strategy for a 50-day period. They find that the strategy based on relative asset strength constitutes the most successful strategy, whereby the strategy based on market index acceleration is the least successful.
Mathieu and Brandouy (Citation2010) introduce an API for artificial stock markets that allows for a broad spectrum of configurations. Agents randomly place orders without utilizing information from the market or other agents. These orders are matched via order books. Mathieu and Brandouy (Citation2010) highlight that the asset returns of the artificial market are similar to the ones of a real-world data sample and that stylized facts can be reproduced successfully.
3. Methodology
We introduce a simulated financial market where different heterogeneous agents buy and sell stocks based on their respective utility functions, following (Lebaron, Citation2001). Relative to other financial market simulation frameworks, our market model exhibits a rather high degree of complexity, as we create a limit-order market in which heterogeneous agents conduct individual utility-based portfolio optimization and trade multiple assets. We choose this relatively high model complexity, to increase the external validity of our results. However, it still constitutes, like economic models in general, a significant simplification of the real world. In this Chapter, we present the market framework and market parameters. We systematically modify central market aspects (e.g., agent composition and agent target price forecasting errors) to increase the robustness of our results with regard to different market situations and to analyze the impact of these central market aspects on market outcomes. To further increase the robustness of our results, we run every simulation setting on eight different random seeds, whereby each individual simulation consists of 100 simulation rounds. One simulation round is considered a quarter of a year in the real world. Agents adjust their portfolio holdings once per simulation round based on their stylized behavior and utility functions.
3.1. Assets
The agents’ portfolios consist of four different risky assets and risk-free cash holdings. We choose a number of four risky assets to still enable a relative weighting between the different risky assets, while ensuring a feasible computational complexity of the portfolio optimization problem. Holding cash is equivalent to investing into a risk-free asset with zero return, which is a commonly used economic model (Adam-Müller & Panaretou, Citation2009; Clarkson et al., Citation1996; Ovtchinnikov & McConnell, Citation2009). Stocks are traded on the simulated market and have an underlying fundamental value and a publicly observable market value. At the time of inception, every artificial stock is assigned a random real-world equivalent. For every random seed, real-world equivalent stocks are sampled randomly among the constituents of the S&P 500 index as of the start of 1996. We calculate these stocks’ quarterly total-return time series using data from the CRSP/Compustat merged database and exclude stocks that have not been traded publicly constantly between 1986 and 2020. We further exclude other stocks that do not have complete and uninterrupted time series during that time, which are necessary for our simulation setting where we need a real-world total return for each round. We acknowledge that this exclusion induces a survivorship bias in the remaining return series. However, we argue that this survivorship bias should not significantly affect the critical results of our analysis of the individual stocks, as the survivorship bias would only affect random seeds, in which a company with an incomplete return series would be sampled into one of the four risky stocks of a market. Even in these cases, the return forecasting process of active agents is the same for different return levels. We use the first 10 years of the resulting time series, namely the data from the beginning of 1986 until the end of 1995, for parameter initialization (e.g., variance-covariance matrix) and use the remaining data from the beginning of 1996 to the end of 2020 to run the simulation. Specifically, at the end of each simulation round, the fundamental value for each artificial stock is updated based on the total quarterly return of the respective real-world stock. We denote the fundamental value of stock at time
with
and the total number of risky assets with
. While this fundamental price is not directly observable for market participants, every stock also has a publicly observable market price,
, that gets updated continuously throughout the simulation rounds and is exclusively determined by the trading activity in the simulated market. A market capitalization-weighted combination of all risky assets constitutes the market portfolio at a given point in time. At the beginning of the simulation, we initialize all artificial stocks with a fundamental value and a market value of 100 US dollars.
3.2. Market mechanism
We implement continuous double auctions to aggregate supply and demand in our simulated market. We choose continuous double auctions over a simpler market clearing mechanism, as continuous double auctions are used within most real-world stock markets and therefore make the market setting more realistic (Lebaron, Citation2001). Over each simulation round, the traders gradually place their buy and sell orders for the different stocks. Orders are matched (partially) with suitable orders in the respective order book, if possible. Parts of the order that cannot be executed directly are added to the respective order book. At the beginning of each simulation round, all order books are cleared.
3.3. Agents
In our simulated market setting, we distinguish between three different stylized agent types: active investors, passive investors, and random investors. We choose a total number of 500 agents for each simulated market setting, as we find that this parameter value leads to a stable convergence of market prices while ensuring computational feasibility. In every simulation round, each agent determines their target portfolio weights once and issues limit buy or sell orders based on these target portfolio weights. Active investors and passive investors both maximize the following commonly used utility function (Bodie et al., Citation2018) based on the modern portfolio theory (Markowitz, Citation1952):
where is the estimated utility,
is the estimated portfolio return,
is the estimated portfolio return variance,
is the risk aversion, which is randomly initialized with a value between two and six (see Cincotti et al. (Citation2003), Bodie et al. (Citation2018)) at the beginning of the simulation for each agent, and 0.5 is a scaling convention. While active investors and passive investors have the same utility function, they differ in the way they generate their return estimates, as we describe in detail in Subsection 3.3.1 and Subsection 3.3.2. For a given simulation round, the optimization and trading activities of all agents occur sequentially with a randomized agent order. Specifically, the trading process for a simulation round is divided into
segments, where
represents the total number of agents. At a given point in time
, the
th randomly selected trader determines their target portfolio weights based on their stylized agent behavior and issues limit buy or sell orders to obtain their target portfolio composition. For a buy order, the limit buy price for stock
at time
,
, is computed by:
where represents the highest bid price for stock
at time
and
represents a random draw from the Gaussian distribution
. Conversely, the sell price for asset
at time
,
, is computed by:
where denotes the lowest ask price for asset
at time
and
represents a random draw from the Gaussian distribution
. Following the rationale of Raberto et al. (Citation2001), Raberto, Cincotti et al. (Citation2003), Raberto and Cincotti (Citation2005), Ponta et al. (Citation2011), and Cocco et al. (Citation2017), this means that agents who want to buy an asset marginally overbid the current best bid price on average and agents who seek to sell an asset tend to marginally underbid the current best ask price in the market. Therefore, buy (sell) orders with prices higher (lower) than the best ask (bid) price for an asset are equivalent to market orders, given a sufficient market depth. Similar to the cited approaches, we tune the system with different parameters (
) and select the parameter combination (
), for which the highest number of the stylized facts of real-world financial markets described in Section 3.5.3 is fulfilled.
3.3.1. Active investors
Our active investors estimate the fundamental values for the individual stocks and optimize their individual portfolio weights based on these estimates. Mathematically, at time , agent
estimates the end-of-round fundamental value of asset
,
with:
where denotes the forecasting error, determined by a random draw from the Gaussian distribution
. Since there is limited evidence about target price forecasting errors of financial analysts in academic research (Bonini et al., Citation2010) and forecasting errors can vary strongly for different markets and countries (Bilinski et al., Citation2013), we run the simulations with different values for
. Bilinski et al. (Citation2013) evaluate the twelve-month target price forecasting errors of financial analysts,
, in different countries. We use the average analyst target price accuracy identified in Bilinski et al. (Citation2013) to calculate the starting value for
and scale it to a three-month horizon under the assumption of unbiased and normally distributed forecast errors and a Brownian motion forecast error development over time (see Appendix C). This yields a starting value of
for
and equivalently
for
. We consider this our baseline setting, but as it is based on a number of assumptions, we run our simulation with different values for the individual target price forecasting errors to ensure the validity of our results in different settings. This results in a final value set for
of
and equivalently a final value set for
of
.
Utilizing their price estimate for risky asset
for the end of the round,
, agent
estimates the end-of-round return for asset
at time
,
, as follows:
where denotes the market price of asset
at time
.
Based on EquationEquation 5(5)
(5) agent
generates return estimates for all risky assets, which, combined with the risk-free return of zero, yield return estimate vector
. At time
, the fundamental agent
selects their desired portfolio weights
by maximizing their utility based on EquationEquation 1.
(1)
(1) Thereby, the estimated portfolio return,
, is given by
with
and
where denotes the minimum individual portfolio weight, and
denotes the maximum individual portfolio weight. For these portfolio optimization tasks, we utilize the cvxpy package (S. Diamond & Boyd, Citation2016) in combination with the ecos solver (Domahidi et al., Citation2013). Furthermore, the estimated portfolio variance of agent
at time
,
, is given by
where denotes the estimated portfolio variance of agent
,
denotes the estimated variance-covariance matrix (
) of all asset returns. All agents estimate the variance-covariance matrix at a specific time from the 40 most recent asset returns. The variance of the risk-free asset and its covariance with other assets is zero per definition. We focus on active agent’s forecasts of prices and, related to that, returns, as returns are the critical input parameter in mean-variance portfolio optimisation (Best & Grauer, Citation1991). We choose the simplification of a joint variance-covariance matrix, as, similar to individual target price forecasting errors, modeling individual errors would be subjected to several assumptions. As this assumptions already constitute Chopra and Ziemba (Citation1993) compare the importance of different input parameters on mean-variance portfolio optimization and show that returns have about eleven times as much influence on the portfolio selection as portfolio variances. Further, we restrict the individual portfolio weights to be non-negative with an upper bound of
, where
constitutes our base case. We define these portfolio weight restrictions to increase the robustness of portfolio optimization. In reality, short-selling usually comes with high costs and certain types of investors are restricted to participate in short-selling. Imposing short-scale constraints is equivalent to shrinking larger elements of the covariance matrix towards zero (Jagannathan & Ma, Citation2003). Jagannathan and Ma (Citation2003) argue that the most extreme covariance estimates are likely to be caused by downward-biased or upward-biased estimation errors. Therefore, this shrinking may reduce the overall estimation error. Furthermore, mean-variance optimization tends to lead to extreme portfolio weight results, and the introduction of an upper weight bound can ensure a certain level of portfolio diversification and lower the risk of extreme events (Eichhorn et al., Citation1998; Grauer & Shen, Citation2000; Jagannathan & Ma, Citation2003). Finally, the difference between the desired portfolio weights
and actual portfolio weights
determines the order vector
for agent
at time
:
After determining their order vector, agent issues orders with the corresponding order quantities, where we round the exact number of shares to integer values.
3.3.2. Passive investors
The passive investors do not estimate stock values of individual securities but instead combine the market with the risk-free asset to form their overall portfolio. All risky assets are weighted based on their market capitalization within the market portfolio. Thus, passive investors face a more simple portfolio optimization problem than active investors, as they only determine which fraction of their wealth they invest in the stock market and do not actively select the individual portfolio weights of the risky assets. Specifically, at time , passive agent
selects the portfolio weights
that maximize the utility function specified in EquationEquation 1.
(1)
(1) The estimated portfolio return,
, is given by:
with
and
where denotes the minimum portfolio weight and
is a vector of length two that includes the expected market return of passive investors, the average return of the market portfolio over the last 40 observations, and the expected return of cash, zero. The estimated portfolio variance
is given by:
where denotes the estimated return variance-covariance matrix (
) at time
, whereby the market return variance is estimated over the last 40 simulation rounds and the estimated covariance between the market portfolio return and cash, as well as the variance of the risk-free return, is zero. Corresponding to EquationEquation 10
(10)
(10) , the passive agent
issues orders with trading quantities based on the difference between their desired portfolio weights and their actual portfolio weights. Again, the exact number of shares is rounded to integer values.
3.3.3. Random investors
Our third stylized investor group consists of agents that have a completely randomized behavior. These random behaviors can stabilize the trading system of a simulated market and are often described in the academic literature as a thermal bath to evaluate other stylized trading behavior (Cincotti et al., Citation2003; Cocco et al., Citation2017; Raberto et al., Citation2003). Researchers have been able to reproduce various stylized facts of real-world financial markets in simulated markets consisting of only random investors (Mathieu & Brandouy, Citation2010; Ponta et al., Citation2011). In our study, random investors may denote a multitude of different investor types who have in common that their aggregated trading behavior is not related to fundamental asset values and, hence, does not have a systematic directed impact on asset prices. Same as the other types of investors, random investors rebalance their portfolio once per simulation round. For the random agents, we loosely follow the agent design of Ponta et al. (Citation2011). At the time , the desired risky portfolio weight for random agent
is drawn from a uniform distribution between zero and one.
The buy and sell prices of these orders are given by EquationEquations (2)(2)
(2) and (Equation3
(3)
(3) ). The desired market weight of a random agent
at time
is given by:
where denotes a random draw from the continuous uniform distribution
. Correspondingly, the desired weight of the portfolio cash fraction of random agent
at time
is given by:
Furthermore, the desired weights of each individual risky asset within the risky portion of the portfolio are each drawn from the continuous uniform distribution . These draws are normalized to sum up to one and subsequently scaled by the desired risky portfolio portion
. Combined with the desired cash weight, this gives the desired portfolio weight vector of random agent
at time
,
. Parallel to the other stylized investors, random agent
issues orders based on the difference between actual and desired portfolio weights (see EquationEquation 10
(10)
(10) ), rounding the exact number of shares to integer values.
3.4. Parameter overview and sensitivity analysis
gives an overview over our parameter choices in the standard market setting. However, as mentioned above, we aim to understand the impact of major market parameters on market results and hence repeatedly run the simulation on all random seeds with systematically altered simulation parameters. Concretely, we repeat the simulation with the following, varying parameter specifications:
Fraction of random agents relative to all market participants of 40 percent and 90 percent
Individual active investors’ absolute target price forecasting errors (aTPE) of 0.225 and 0.9
Individual risk aversion factors fixed at two and six
Transaction costs of 0.5 percent
Active investors’ upper portfolio weight constraints of 0.33 and 1.
Table 1. Overview of parameter choices in the standard market setting.
3.5. Evaluation
To assess our simulated market’s quality and create comparability to a real-world stock market, we use different established measures, i.e., price deviation, trading volume, market depth, and quoted spread. These measures are calculated for each market configuration by aggregating the results over all assets, simulation rounds, and random seeds. Additionally, we test whether selected stylized facts of real-world financial time series are reproduced in our baseline simulated market.
3.5.1. Fundamental market efficiency
We measure fundamental market efficiency by calculating the mean absolute deviation between the market price of the simulation, from the fundamental market prices,
, at time
for each asset
:
To receive relative price deviation as a percentage value, we divide the absolute value in the above formula by and multiply by 100, which results in
3.5.2. Trading volume
Another measure we use is the trading volume, which describes the number of shares traded in each round multiplied by their corresponding prices. Trading volume generally indicates market activity and constitutes a basis for many liquidity measures of financial markets (Sarr & Lybek, Citation2002).
3.5.3. Stylized facts of real-world financial markets
To further evaluate our simulation model, we investigate if our time series of returns follow the same statistical patterns found in many financial time series, so-called stylized facts of financial markets. We check whether the resulting market return series of our simulated market elicits these stylized facts to evaluate the external validity of our results. Cont (Citation2001) list eleven different stylized facts that have been observed and studied repeatedly over the last decades. While these statistical properties are typical for real-world data, Cont (Citation2001) also describe that it is very challenging to create a synthetic market model that can reproduce all stylized facts. Furthermore, since stylized facts generalize and simplify, they are a more qualitative measure by nature. This qualitative character of many stylized facts impedes the comparability of different market scenarios based on these properties. Therefore, we focus on the following selected stylized facts, as they allow for a certain level of quantitative analysis. Since the stylized facts are usually found in return time series with a higher data frequency (Cont, Citation2001), we further split our simulation rounds into 90 equal proportions, which then results in a resolution of daily returns. We test for the existence of each stylized fact on every random seed and every trader composition in the baseline market setting.
Heavy Tails: Real-world financial return time series tend to be heavy tailed and non-Gaussian (Bradley & Taqqu, Citation2003; Cont, Citation2001). These series often appear to exhibit a power-law tail with a tail index between two and five (Cont, Citation2001; Katahira et al., Citation2019). To test whether a return series follows a normal distribution, we calculate its excess kurtosis and conduct a Kolmogorov-Smirnov test (Kolmogorov, Citation1933; Massey, Citation1951; Smirnov, Citation1948) to show that it is non-Gaussian and heavy tailed. Following Katahira et al. (Citation2019), we also calculate the alphas (i.e., tail-indices) of the heavy tail power-law distributions and calculate the log-likelihood ratio (Wilks, Citation1938) between a power-law and an exponential distribution given our observed return distribution sample. In total, we calculate the excess kurtosis and p-values of the Kolmogorov-Smirnov test, the power-law alpha, as well as log-likelihood ratios including corresponding p-values for a goodness-of-fit comparison between a power-law and an exponential distribution.
Conditional Heavy Tails: Real-world returns also often show so-called conditional heavy tails, these are given when a return series is corrected for volatility clustering and still shows heavy tails (Cont, Citation2001). However, the excess kurtosis of the corrected returns is smaller than the excess kurtosis for the unconditional return distribution. Therefore, we calculate the excess kurtosis of the residuals gained from a GARCH (Bollerslev, Citation1986) model trained to account for volatility clustering. Again, we run the Kolmogorov-Smirnov test to calculate each corresponding p-value. We consider the stylized fact as fulfilled if the calculated excess kurtosis is positive but lower than the kurtosis calculated for the unconditional heavy tails and if the Kolmogorov-Smirnov test shows statistical significance at the 95% confidence level.
Gain Loss Asymmetry: Gain loss asymmetry is the difference in the upward and downward movement of returns. In real-world financial markets, prices tend to fall faster than they rise Cont (Citation2001). To test for the fact in a quantifiable way we mainly follow the procedure of Jensen et al. (Citation2003). We set a positive and a negative return level of ten percent and minus ten percent respectively, and then count the time steps needed (i.e., the investment horizons) until the asset reaches this return level. This is done for each asset and at each time step. We then calculate the two density functions of the negative and positive investment horizons and determine their maxima to compare whether, in general, the positive or the negative return level is reached more quickly. We consider the stylized fact as fulfilled if the maximum of the density function for the negative return level lies before the positive return level.
4. Results
In this section, we present the results of the different simulation runs described in Section 3. Concretely, we analyze the effect of different market settings and parameters on our key metric fundamental market efficiency and on market activity. Furthermore, we evaluate our market model based on the quantifiable stylized facts described in Section 3.5.3.
4.1. Standard market setting
shows the relative fundamental price deviation for varying fractions of active and passive investors over all random seeds for our standard scenario (i.e., 80 percent random investors). We find a lower relative fundamental price deviation for a higher fraction of active investors. Specifically, market prices on average deviate by 55.17 percent from fundamental prices in the market setting without active investors and 100 (i.e., 20 percent) passive investors and by 25.86 percent in the market setting with 100 (i.e., 20 percent) active investors and zero passive investors. On average, the substitution of a passive investor with an active investor increases the fundamental market efficiency, as the fitted cubic function is monotonously decreasing between and
. However, the marginal increase in fundamental market efficiency for this substitution is lower for higher levels of active investment in the market, as the second-order derivative of the function is strictly positive in the area under consideration. Furthermore, as detailed in , the mean trading volume increases for a larger fraction of active investors, nearly doubling from the setting without active investors and the setting with 100 (i.e., 20 percent) active investors.
Figure 1. Fundamental price deviation by fraction of active investors (relative to all active and passive investors) detailed over all random seeds in the standard setting.

Table 2. Fundamental price deviation and trading volume for different investor compositions in the standard setting averaged over all random seeds.
4.2. Sensitivity to different levels of random investment
shows the relative fundamental price deviation and mean trading volume for different fractions of random agents of the total number of market participants (i.e., 40% and 90%). For each scenario, we run the simulation on multiple random seeds, repeatedly altering the combinations of active and passive investors by five percentage points. We find that a lower portion of random agents, which corresponds to a higher combined fraction of passive and active investors, leads to a higher discrepancy between the market composition without active investors and compositions with a high share of active investors. Concretely, in the scenario with 40 percent random agents, the mean relative fundamental price deviation starts at 74.42 percent with no active investors in the market. It decreases to 19.10 percent for the setting with 300 (i.e., 60 percent) active investors. In the scenario with a share of 90 percent random agents, the mean relative fundamental price deviation starts at only 47.64 percent in the setting where all non-random investors are passive investors. However, it decreases to just 31.53 for the market composition in which all-remaining investors are active investors.
Table 3. Fundamental price deviation and trading volume for different investor compositions in the market settings with a share of 40 percent and 90 percent random investment averaged over all random seeds.
Table A1 and Figure B2 present the corresponding results for a market composition with only 20 percent random investors. These results allow us to further examine the effects of passive investment on fundamental market efficiency. For instance, the average relative price deviation from fundamental prices is 65.24 percent in the market with 100 active, 300 passive, and 100 random investors and amounts to 25.86 percent in the market with 100 active, zero passive, and 400 random investors (see ). Combined with the in Section 4.1 presented findings, this indicates that a large fraction of passive investors, contrary to a large fraction of active and random investors, impairs fundamental market efficiency. While it is clear that the trading behavior of active investors links market prices to fundamental values, it is noteworthy that this link is distinctly stronger in market environments with a lower amount of passive investment. This finding may be driven by the similar trading patterns and generally lower trading activity within the group of passive investors. Regarding the latter, shows that the average absolute weight change of all risky assets per simulation round is distinctly higher for active investors than for passive investors.
Table 4. Mean absolute changes of portfolio weights between simulation rounds by agent type and agent composition.
Additionally, large fractions of passive investment may lead to price bubbles and market failure. shows that some compositions that have a high share of passive investment exhibit a particularly high price deviation from fundamental values. This is driven by specific simulation runs, in which market values deviate strongly from fundamental values. , which details the results over the different random seeds, shows that these large price bubbles do not occur in all market settings with a high fraction of passive investment. However, these settings enable price bubbles, as we do not observe bubbles of similar magnitude in settings with a generally lower level of passive investment (see ). Figure B1 illustrates the mechanism of a price bubble at the example of the simulation run for random seed and a market composition of 90 active, 210 passive, and 200 random investors. After a series of high market returns, passive investors gradually increase their risky portfolio fractions. Simultaneously, active investors realize that the market becomes overpriced and sell as many units of the risky assets as they can, given their portfolio constraints. However, due to the large share of passive investors, in these scenarios active investors cannot correct for the overpricing and the link between market prices and fundamental values breaks down.
4.3. Sensitivity to different individual target price forecasting errors of active investors
und present the effect of varying individual target price forecasting errors of active investors on fundamental market efficiency. Generally, for market compositions with active investors, we find that lower individual errors lead to a higher level of fundamental market efficiency and vice versa. In our setting, a bisection of individual target price forecasting errors compared to the standard market setting reduces the mean fundamental price deviation by up to 4.56 percentage points, which occurs for the composition of 100 active, zero passive, and 400 random investors. Doubling the individual target price forecasting errors results in an average increase in fundamental price deviation of up to 8.00 percentage points, occurring for the market composition of 20 active, 80 passive, and 400 random investors. Furthermore, we find that a lower (higher) individual active forecasting error results in a lower (higher) overall trading volume. For lower (higher) individual forecasting errors, the estimated fundamental prices of active investors become more (less) similar and it is less (more) likely for active investors to trade with each other and more (less) likely to compete for buying or selling the same stocks.
Figure 4. Fundamental price deviation by fraction of active investors (relative to all active and passive investors) detailed over all random seeds in the market settings with active investors’ individual absolute target price forecasting errors (aTPE) of 0.225 and 0.9.

Table 5. Relative fundamental price deviation for different investor compositions in the market settings with active investors’ individual absolute target price forecasting errors (aTPE) of 0.225 and 0.9 averaged over all random seeds.
4.4. Sensitivity to changes in individual risk aversion
To analyze the influence of individual risk aversion on market outcomes, we formulate two additional scenarios in which the risk aversion factor is fixed at values of two and six, respectively. and show that changes in individual risk aversion only have a minor impact in markets with a higher share of active investment. In market settings with a low share of active investment, we observe that lower individual risk aversion increases mean fundamental price deviation. This effect might be explained by the fact that lower risk aversion leads to more extreme portfolio weights, which, in turn, may drive prices faster away from fundamental values in markets that only have a weak link between fundamentals and market prices (i.e., low share of active investors).
Figure 5. Fundamental price deviation by fraction of active investors (relative to all active and passive investors) detailed over all random seeds in the market setting with individual investors’ risk aversion of and
.

Table 6. Relative fundamental price deviation for different investor compositions in the market settings with individual investors’ risk aversion of and
averaged over all random seeds.
4.5. Sensitivity to different transaction costs
and present the results of a simulation scenario of a market that features relative trading costs of 0.5 percent. Introducing transaction costs reduces fundamental market efficiency in the market settings with a higher share of active investors. Specifically, the mean fundamental market deviation increases for all tested agent compositions with more than 50 active agents. Furthermore, it only decreases to 28.21 percent for the composition with 100 active, zero passive, and 400 random investors, compared to 25.86 percent in the market setting without transaction costs (see ). Moreover, an increase in transaction costs leads to lower trading volumes, as investors incorporate the expected trading costs into their utility functions and prefer smaller portfolio changes.
Figure 6. Fundamental price deviation by fraction of active investors (relative to all active and passive investors) detailed over all random seeds in the market setting with 0.5% transaction costs.

Table 7. Fundamental price deviation and trading volume for different investor compositions in the market setting with 0.5 percent transaction cost averaged over all random seeds.
4.6. Sensitivity to portfolio weight restraints
and present the results for different upper bounds for the individual portfolio weights of the different assets. We find that less restrictive individual portfolio weights (i.e., ) lead to higher fundamental market efficiency and higher trading activity on average. In contrast, the opposite is true for more restrictive individual portfolio weights (i.e.,
). In our market setting with 100 active, zero passive, and 400 random investors, the less restrictive upper bound of
results in a mean fundamental price deviation of 22.03 percent. For the same market composition, the more restrictive bound of
leads to a mean fundamental price deviation of 30.29 percent.
Figure 7. Fundamental price deviation by fraction of active investors (relative to all active and passive investors) detailed over all random seeds in the market setting with individual investors’ upper portfolio constraints of 0.33 and 1.

Table 8. Fundamental price deviation and trading volume for different investor compositions in the market setting with individual investors’ upper portfolio constraints of and
averaged over all random seeds.
4.7. Replication of stylized facts of real-world financial markets
Table A2 presents the results of our quantitative tests for the replication of stylized facts of financial return time series detailed over all compositions and random seeds in our standard market setting. We find that all return series exhibit an excess kurtosis of at least two, and the Kolmogorov-Smirnov tests (Kolmogorov, Citation1933; Massey, Citation1951; Smirnov, Citation1948) show that all series are found to be non-Gaussian at a 95% confidence level. The alpha of a fitted power-law distribution is in 71% of all simulated market outcomes in the range between two and five. Furthermore, the log-likelihood tests show that in 81% of market outcomes, the observed distribution rather follows a power-law distribution than an exponential distribution. While these tests are only in 17% of these cases significant at the 95% confidence level, they never significantly indicate that any of the observed return series rather follow an exponential distribution. Additionally, we find that the created return times series exhibit conditional heavy tails in 41% of all market outcomes. The stylized fact gain loss asymmetry is fulfilled in 64% of all simulated market outcomes.
5. Discussion
This simulation study sheds light on the impact of two essential investor types, namely active and passive investors, on fundamental market efficiency. It becomes apparent that high levels of passive investment within a market may foster price bubbles and impair fundamental market efficiency. This finding has implications for financial regulators, who should incorporate the level of passive investment and existing market frictions when assessing risks to financial market stability. In the light of an increase in passive investment due to the emergence of ETFs and robo-advisory, this issue has become increasingly relevant. However, while it is possible to approximate the level of passive investment through the volume of passively managed funds, it is challenging to differentiate between various types of active investors without conducting concrete interviews.
Additionally, the increase of fundamental market inefficiency for a higher fraction of passive investment is influenced by active investors’ trading frictions and portfolio restrictions. Thereby, markets with less restricted active investors may incorporate a higher fraction of passive investors without creating large price bubbles, as less restricted active investors can trade more and correct a higher level of mispricing. This finding emphasizes the need for a careful situational assessment by regulators when imposing measures that constrain trading.
The study further shows that the trading behavior of active investors, who individually have imprecise but unbiased target price forecasts, links the market prices of assets to their fundamental values. The marginal increase in fundamental market efficiency for an additional fundamental investor entering the market decreases for higher levels of existing fundamental investment. However, due to the imprecision of individual forecasts, market prices generally may not exactly reflect fundamental asset values at a certain point in time. Our results also show that more accurate target price forecasts, as, for instance, generated by the increased use of machine learning methods (Gu et al., Citation2020), leads to a higher fundamental efficiency of market prices.
Generally, we find that our results are in line with related work (e.g., (Anadu et al., Citation2020; Qin & Singal, Citation2015; Sullivan & Xiong, Citation2012; Sushko & Turner, Citation2018)), which finds that a certain share of active investors is necessary for functioning and efficient financial markets. Our simulation setting allows to provide further details on the underlying mechanisms of this issue and evaluates the impact of different market environments.
An explicit limitation of this study is its simulation setting. While we generally select simulation parameters carefully based on empirical evidence, it is unavoidable to make certain assumptions during parameter selection. Furthermore, the simulation setting significantly simplifies real-world financial markets. For instance, while mean-variance utility functions are still commonly used in finance, they may overly simplify the utility functions of investors. Hence, we aim to draw general conclusions about financial markets’ functioning rather than narrow down on the exact magnitude of specific numeric results. We aim to reduce this limitation by testing whether the return times series generated by our simulated market exhibit quantifiable stylized facts that are often observed in real-world financial markets. We find that all generated return series exhibit excess kurtoses and further tests suggest that most of the generated time series exhibit heavy tails and a gain-loss asymmetry. However, we only identify the stylized fact of conditional heavy tails in 42% of all generated return series. Overall, these tests show that our simulated market exhibits some but not all properties of real-world financial markets.
As this study focuses on the impact of fundamental and passive investment on fundamental market efficiency, future research could shed further light on the decision of investors to invest based on fundamental analysis or to invest passively. As our study shows, markets become increasingly fundamentally efficient with higher fractions of active investors. However, in markets with higher shares of active investment, the competition to trade mispriced assets also increases, leading to a lower utility of individual active investors. Hence, researchers could investigate the existence of a possible equilibrium level of active and passive investment in a financial market and examine further influencing factors, such as the use of other utility functions that have been shown to resemble the real world more realistically (e.g., prospect theory (Kahneman & Tversky, Citation1979)).
6. Conclusion
In this simulation study, we analyze the effects of different levels of active and passive investment on fundamental market efficiency. We examine various market settings to understand the impact of other market parameters on our results. For the selected market settings, we generally find that larger fractions of active investment within a market increase overall fundamental market efficiency. The marginal positive effect on fundamental market efficiency per additional fundamental investor decreases for larger fractions of fundamental investment. In addition, a less restrictive trading environment for active investors in the form of relaxed portfolio constraints or lower trading costs facilitates trading and increases fundamental market efficiency. Furthermore, more accurate price forecasts of individual active investors, caused, for instance, by the proliferation of machine learning approaches within the asset management industry, tend to increase fundamental market efficiency. We also find that large fractions of passive investment within a financial market may lead to market failure and facilitate technical price bubbles.
Supplemental Material
Download Zip (148.5 KB)Disclosure statement
No potential conflict of interest was reported by the author(s).
Supplementary material
Supplemental data for this article can be accessed online at https://doi.org/10.1080/15140326.2023.2188634
Additional information
Funding
Notes on contributors
Patrick Jaquart
Patrick Jaquart is a postdoctoral researcher at the Institute of Information Systems and Marketing (IISM), Karlsruhe Institute of Technology (KIT). His research interests include asset pricing, financial market simulation, data science, and machine learning. His research has been published in journals such as The Journal of Finance and Data Science and proceedings of international Finance and Information Systems conferences.
Marvin Motz
Marvin Motz is a PhD student at the Institute of Information Systems and Marketing (IISM), Karlsruhe Institute of Technology (KIT). His research interests include sustainable finance, ecological investment, and asset pricing.
Lutz Köhler
Lutz Köhler is a research fellow at the Chair of Statistical Methods and Econometrics (ECON), Karlsruhe Institute of Technology (KIT). He currently pursues his graduate studies at KIT. His research interests include asset pricing, financial market simulation, and data science.
Christof Weinhardt
Christof Weinhardt is director of the Institute for Information Systems and Marketing (IISM) at the Karlsruhe Institute of Technology (KIT). He is also a director and founder of the Karlsruhe Service Research Institute and director of the Research Center of Information Technology. Since the beginning of 2019, he is Editor-in-Chief for the Journal Business & Information Systems Engineering (BISE). Professor Weinhardt was a member of the expert group Business & Economics of the German National Research Foundation and has acted as expert adviser in the Enquete Commission of the German Parliament for Internet and Digital Society. He has published more than 150 articles and books and has received a number of awards for his research and teaching.
References
- Adam-Müller, A. F. A., & Panaretou, A. (2009). Risk management with options and futures under liquidity risk. Journal of Futures Markets: Futures, Options, and Other Derivative Products, 29(4), 297–31. https://doi.org/10.1002/fut.20362
- Anadu, K., Kruttli, M. S., McCabe, P. E., Osambela, E., & Shin, C. (2020). The shift from active to passive investing: Potential risks to financial stability? Financial Analysts Journal, 76(4), 23–29. https://doi.org/10.1080/0015198X.2020.1779498
- Appel, I. R., Gormley, T. A., & Keim, D. B. (2019). Standing on the shoulders of giants: The effect of passive investors on activism. The Review of Financial Studies, 32(7), 2720–2774. https://doi.org/10.1093/rfs/hhy106
- BarclayHedge. (2018). BarclayHedge survey: Majority of hedge fund pros use AI/machine learning in investment strategies. https://www.barclayhedge.com/insider/barclayhedge-survey-majority-of-hedge-fund-pros-use-ai-machine-learning-in-investment-strategies
- Belasco, E., Finke, M., & Nanigian, D. (2012). The impact of passive investing on corporate valuations. Managerial Finance, 38(11), 1067–1084. https://doi.org/10.1108/03074351211266793
- Benhammada, S., Amblard, F., & Chikhi, S. (2017). An asynchronous double auction market to study the formation of financial bubbles and crashes. New Generation Computing, 35(2), 129–156. https://doi.org/10.1007/s00354-017-0010-6
- Bertella, M. A., Pires, F. R., Feng, L., & Stanley, H. E. (2014). Confidence and the stock market: An agent-based approach. PLoS One, 9(1), 1–9. https://doi.org/10.1371/journal.pone.0083488
- Best, M. J., & Grauer, R. R. (1991). On the sensitivity of mean-variance-efficient portfolios to changes in asset means: Some analytical and computational results. The Review of Financial Studies, 4(2), 315–342. https://doi.org/10.1093/rfs/4.2.315
- Bilinski, P., Lyssimachou, D., & Walker, M. (2013). Target price accuracy: International evidence. The Accounting Review, 88(3), 825–851. https://doi.org/10.2308/accr-50378
- Blitz, D. (2014). INVITED EDITORIAL COMMENT: The dark side of passive investing. The Journal of Portfolio Management, 41(1), 1–4. https://doi.org/10.3905/jpm.2014.41.1.001
- Blume, L., Easley, D., & O’hara, M. (1994). Market statistics and technical analysis: The role of volume. The Journal of Finance, 49(1), 153–181. https://onlinelibrary.wiley.com/doi/abs/10.1111/j.1540-6261.1994.tb04424.x
- Bodie, Z., Kane, A., & Marcus, A. J. (2018). Investments. McGraw Hill Education.
- Bollerslev, T. (1986). Generalized autoregressive conditional heteroskedasticity. Journal of Econometrics, 31(3), 307–327. https://linkinghub.elsevier.com/retrieve/pii/0304407686900631
- Bonini, S., Zanetti, L., Bianchini, R., & Salvi, A. (2010). Target price accuracy in equity research. Journal of Business Finance & Accounting, 37(9–10), 1177–1217. https://doi.org/10.1111/j.1468-5957.2010.02209.x
- Bradley, B. O., & Taqqu, M. S. (2003). Financial risk and heavy tails. Woodhead Publishing Limited. https://doi.org/10.1016/B978-044450896-6.50004-2
- Brown, D. P., & Jennings, R. H. (1989, May). On technical analysis. The Review of Financial Studies, 2(4), 527–551. https://doi.org/10.1093/rfs/2.4.527
- Carhart, M. M. (1997). On persistence in mutual fund performance. The Journal of Finance, 52(1), 57–82. https://doi.org/10.1111/j.1540-6261.1997.tb03808.x
- Chopra, V. K., & Ziemba, W. T. (1993). The effect of errors in means, variances, and covariances on optimal portfolio choice. The Journal of Portfolio Management, 19(2), 6–11. https://doi.org/10.3905/jpm.1993.409440
- Cincotti, S., Focardi, S. M., Marchesi, M., & Raberto, M. (2003). Who wins? Study of long- run trader survival in an artificial stock market. Physica A: Statistical Mechanics and Its Applications, 324(1–2), 227–233. https://doi.org/10.1016/S0378-4371(02)01902-7
- Clarkson, P., Guedes, J., & Thompson, R. (1996). On the diversification, observability, and measurement of estimation risk. The Journal of Financial and Quantitative Analysis, 31(1), 69–84. https://doi.org/10.2307/2331387
- Cocco, L., Concas, G., & Marchesi, M. (2017). Using an artificial financial market for studying a cryptocurrency market. Journal of Economic Interaction and Coordination, 12(2), 345–365. https://doi.org/10.1007/s11403-015-0168-2
- Cont, R. (2001). Empirical properties of asset returns: Stylized facts and statistical issues. Quantitative Finance, 1(2), 223–236. http://www.cmap.polytechnique.fr/
- Cremers, K. J. M., & Petajisto, A. (2009). How active is your fund manager? A new measure that predicts performance. The Review of Financial Studies, 22(9), 3329–3365. https://doi.org/10.1093/rfs/hhp057
- Diamond, S., & Boyd, S. (2016). CVXPY: A Python-embedded modeling language for convex optimization. Journal of Machine Learning Research, 17(1), 2909–2913.
- Diamond, D. W., & Verrecchia, R. E. (1981). Information aggregation in a noisy rational expectations economy. Journal of Financial Economics, 9(3), 221–235. https://www.sciencedirect.com/science/article/pii/0304405X8190026X
- Domahidi, A., Chu, E., & Boyd, S. (2013). ECOS: An SOCP solver for embedded systems. In 2013 European Control Conference (ECC), Zurich, Switzerland, (pp. 3071–3076).
- Eichhorn, D., Gupta, F., & Stubbs, E. (1998). Using constraints to improve the robustness of asset allocation. Journal of Portfolio Management, 24(3), 41. https://doi.org/10.3905/jpm.1998.409642
- Fama, E. F. (1970). Efficient capital markets: A review of theory and empirical work. The Journal of Finance, 25(2), 383–417. https://doi.org/10.2307/2325486
- Fama, E. F. (1991). Efficient capital markets: II. The Journal of Finance, 46(5), 1575. https://doi.org/10.1111/j.1540-6261.1991.tb04636.x
- Fama, E. F., & French, K. R. (2010). Luck versus skill in the cross-section of mutual fund returns. The Journal of Finance, 65(5), 1915–1947. https://doi.org/10.1111/j.1540-6261.2010.01598.x
- Farmer, J. D., & Joshi, S. (2002). The price dynamics of common trading strategies. Journal of Economic Behavior & Organization, 49(2), 149–171. https://doi.org/10.1016/S0167-2681(02)00065-3
- Fischer, T., & Krauss, C. (2018). Deep learning with long short-term memory networks for financial market predictions. European Journal of Operational Research, 270(2), 654–669. https://doi.org/10.1016/j.ejor.2017.11.054
- French, K. R. (2008). Presidential address: The cost of active investing. The Journal of Finance, 63(4), 1537–1573. https://doi.org/10.1111/j.1540-6261.2008.01368.x
- Goykhman, M. (2017). Wealth dynamics in a sentiment-driven market. Physica A: Statistical Mechanics and Its Applications, 488, 132–148. https://doi.org/10.1016/j.physa.2017.06.023
- Grauer, R. R., & Shen, F. C. (2000). Do constraints improve portfolio performance? Journal of Banking & Finance, 24(8), 1253–1274. https://doi.org/10.1016/S0378-4266(99)00069-2
- Grossman, S. J., & Stiglitz, J. E. (1980). On the impossibility of informationally efficient markets. The American Economic Review, 70(3), 393–408. Retrieved August 30, 2022, from http://www.jstor.org/stable/1805228
- Gu, S., Kelly, B., & Xiu, D. (2020). Empirical asset pricing via machine learning. The Review of Financial Studies, 33(5), 2223–2273. https://doi.org/10.1093/rfs/hhaa009
- ICI. (2020). Trends in the expenses and fees of funds. Retrieved July 21, 2022, from https://www.ici.org/system/files/private/2021-04/per27-03.pdf
- ICI. (2021). Investment company fact book 2021. Retrieved July 21, 2022, from https://www.ici.org/system/files/2021-05/2021/factbook.pdf
- Jagannathan, R., & Ma, T. (2003). Risk reduction in large portfolios: Why imposing the wrong constraints helps. The Journal of Finance, 58(4), 1651–1683. https://doi.org/10.1111/1540-6261.00580
- Jensen, M. H., Johansen, A., & Simonsen, I. (2003). Inverse statistics in economics: The gain-loss asymmetry. Physica A: Statistical Mechanics and Its Applications, 324(1–2), 338–343. https://doi.org/10.1016/S0378-4371(02)01884-8
- Kahneman, D., & Riepe, M. W. (1998). Aspects of investor psychology. Journal of Portfolio Management, 24(4), 52–65. https://doi.org/10.3905/jpm.1998.409643
- Kahneman, D., & Tversky, A. (1979). Prospect theory: An analysis of decision under. Source: Econometrica, 47(2), 263–292. http://www.jstor.org/stable/1914185%5Cnhttp://www.jstor.org/%5Cnhttp://www.jstor.org/action/showPublisher?publisherCode=econosoc.%5Cnhttp://www.jstor.org
- Katahira, K., Chen, Y., Hashimoto, G., & Okuda, H. (2019). Development of an agent-based speculation game for higher reproducibility of financial stylized facts. Physica A: Statistical Mechanics and Its Applications, 524, 503–518. https://doi.org/10.1016/j.physa.2019.04.157
- Khashanah, K., & Alsulaiman, T. (2017). Connectivity, information jumps, and market stability: An agent-based approach. Complexity, 2017(Financial Networks – Article ID 6752086), 1–16. https://doi.org/10.1155/2017/6752086
- Kolmogorov, A. (1933). Sulla determinazione empirica di una lgge di distribuzione. Giorn Dell’inst Ital Degli Att, 4(1), 83–91.
- Lebaron, B. (2001). A builder’s guide to agent-based financial markets. Quantitative Finance, 1(2), 254–261. https://doi.org/10.1088/1469-7688/1/2/307
- Markowitz, H. (1952). Portfolio selection. The Journal of Finance, 7(1), 77–91. https://doi.org/10.1111/j.1540-6261.1952.tb01525.x
- Massey, F. J. (1951). The Kolmogorov-Smirnov test for goodness of fit. Journal of the American Statistical Association, 46(253), 68–78. https://doi.org/10.1080/01621459.1951.10500769
- Mathieu, P., & Brandouy, O. (2010). A generic architecture for realistic simulations of complex financial dynamics. In Y. Demazeau, F. Dignum, J. M. Corchado, & J. B. Pérez (Eds.), Advances in practical applications of agents and multiagent systems (Vol. 70, pp. 185–197). Springer.
- Milgrom, P., & Stokey, N. (1982). Information, trade and common knowledge. Journal of Economic Theory, 26(1), 17–27. https://www.sciencedirect.com/science/article/pii/0022053182900461
- Moiseev, N. A., & Akhmadeev, B. A. (2017). Agent-based simulation of wealth, capital and asset distribution on stock markets. Journal of Interdisciplinary Economics, 29(2), 176–196. https://doi.org/10.1177/0260107917698781
- Ovtchinnikov, A. V., & McConnell, J. J. (2009). Capital market imperfections and the sensitivity of investment to stock prices. The Journal of Financial and Quantitative Analysis, 44(3), 551–578. https://doi.org/10.1017/S0022109009990081
- Pedersen, L. H. (2018). Sharpening the arithmetic of active management. Financial Analysts Journal, 74(1), 21–36. https://doi.org/10.2469/faj.v74.n1.4
- Petropoulos, F., Apiletti, D., Assimakopoulos, V., Babai, M. Z., Barrow, D. K., Taieb, S. B., Bergmeir, C., Bessa, R. J., Bijak, J., Boylan, J. E., Browell, J., Carnevale, C., Castle, J. L., Cirillo, P., Clements, M. P., Cordeiro, C., Cyrino Oliveira, F. L., De Baets, S., Dokumentov, A. … Ziel, F. (2022). Forecasting: Theory and practice. International Journal of Forecasting, 38(3), 705–871. https://doi.org/10.1016/j.ijforecast.2021.11.001
- Ponta, L., & Cincotti, S. (2018). Traders’ networks of interactions and structural properties of financial markets: An agent-based approach. Complexity, 2018(Advances on the Resilience of Complex Networks – Article ID 9072948), 1–9. https://doi.org/10.1155/2018/9072948
- Ponta, L., Raberto, M., & Cincotti, S. (2011). A multi-assets artificial stock market with zero-intelligence traders. EPL (Europhysics Letters), 93(2), 28002. https://doi.org/10.1209/0295-5075/93/28002
- Qin, N., & Singal, V. (2015). Indexing and stock price efficiency. Financial Management, 44(4), 875–904. https://doi.org/10.1111/fima.12102
- Raberto, M., & Cincotti, S. (2005). Modeling and simulation of a double auction artificial financial market. Physica A: Statistical Mechanics and Its Applications, 355(1), 34–45. https://doi.org/10.1016/j.physa.2005.02.061
- Raberto, M., Cincotti, S., Focardi, S. M., & Marchesi, M. (2001). Agent-based simulation of a financial market. Physica A: Statistical Mechanics and Its Applications, 299(1–2), 319–327. https://doi.org/10.1016/S0378-4371(01)00312-0
- Raberto, M., Cincotti, S., Focardi, S. M., & Marchesi, M. (2003). Traders’ long-run wealth in an artificial financial market. Computational Economics, 22(2–3), 255–272. https://doi.org/10.1023/A:1026146100090
- Rasekhschaffe, K. C., & Jones, R. C. (2019). Machine learning for stock selection. Financial Analysts Journal, 75(3), 70–88. https://doi.org/10.1080/0015198X.2019.1596678
- Sarr, A., & Lybek, T. (2002). Measuring liquidity in financial markets. IMF Working Papers, 2002, 232. https://doi.org/10.5089/9781451875577.001
- Schwert, G. W. (2003). Anomalies and market efficiency. In Handbook of the economics of finance (Vol. 1, pp. 939–974). Elsevier. http://www.sciencedirect.com/science/article/pii/S1574010203010240
- Seyffart, J. (2021). Passive likely overtakes active by 2026, earlier if bear market.
- Sharpe, W. F. (1991). The arithmetic of active management. Financial Analysts Journal, 47(1), 7–9. https://doi.org/10.2469/faj.v47.n1.7
- Smirnov, N. (1948). Table for estimating the goodness of fit of empirical distributions. Annals of Mathematical Statistics, 19(2), 279–281. https://doi.org/10.1214/aoms/1177730256
- Stambaugh, R. F. (2014). Presidential address: Investment noise and trends. The Journal of Finance, 69(4), 1415–1453. https://onlinelibrary.wiley.com/doi/abs/10.1111/jofi.12174
- Sullivan, R. N., & Xiong, J. X. (2012). How index trading increases market vulnerability. Financial Analysts Journal, 68(2), 70–84. https://doi.org/10.2469/faj.v68.n2.7
- Sushko, V., & Turner, G. (2018, March). The implications of passive investing for securities markets. BIS Quarterly Review, March(1), 113–131.
- Vanfossan, S., Dagli, C. H., & Kwasa, B. (2020). An agent-based approach to artificial stock market modeling. Procedia Computer Science, 168, 161–169. https://doi.org/10.1016/j.procs.2020.02.280
- Wilks, S. (1938). The large-sample distribution of the likelihood ratio for testing composite hypotheses. Annals of Mathematical Statistics, 9(1), 60–62. https://www.jstor.org/stable/2957648
- Wu, S., He, J., & Li, S. (2018). Effects of fundamentals acquisition and strategy switch on stock price dynamics. Physica A: Statistical Mechanics and Its Applications, 491, 799–809. https://doi.org/10.1016/j.physa.2017.09.072