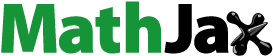
ABSTRACT
This paper examines the role of addiction in influencing the demand for e-cigarettes using the Nielsen Retail Scanner Data and the Nielsen Consumer Panel Data between 2012 and 2017. With a comparison of a myopic addiction model, a forward-looking model, and a rational addiction model, this paper tests whether the consumption of e-cigarettes is addictive and rational. Results from both the macro data and the microdata support the rational addictiveness of e-cigarettes. Applying an OLS method and an instrumental variable method in causal inference, results show that the long-run price elasticity estimates are larger than the estimates of the short-run price elasticity of demand for e-cigarettes. Estimates of both long-run and short-run elasticities are greater than one, −1.50 and −1.05, suggesting e-cigarette demand is elastic in both the long run and short run.
1. Background
Most e-cigarettes contain nicotine, a highly addictive substance (U.S. Department of Health and Human Services, Citation2016). Nicotine is also toxic and harmful, especially for youth and pregnant women. However, e-cigarettes are the most used tobacco product among youth now. About one in three high school students reported current e-cigarette use in 2019; average monthly sales of e-cigarettes increased 122 percent from 2014 to 2020 as the price fell (Ali et al., Citation2020). This paper asks whether this relatively new tobacco product is addictive using data on consumption.
Becker and Murphy (Citation1988) propose a rational addiction model incorporating the influences of past and current consumption for lifetime utility-maximizing consumers. In their model, for addictive goods: high levels of past consumption reinforce the desire for current consumption and increase the marginal utility of current consumption. Their model also indicates that consumers are rational or forward-looking in that they anticipate the expected future consequences of their current actions. This feature differentiates their model from myopic models of addictive behavior which ignore the effect of future consumption when consumers make consumption decisions. Therefore, the rational addiction model of Becker and Murphy (Citation1988) suggests that the consumption of an addictive good depends on both future and past consumption. It has the inter-temporal, dependent demand structure or “adjacent complementarity” (Ryder & Heal, Citation1973). This rational addiction model is also a type of rational choice model (Calvert, Citation1985), which focuses on the dynamics of current consumption in response to future prices of addictive goods.
Tests of addiction have been applied to several different goods. However, there is no research investigating the role of addiction in influencing the demand for e-cigarettes. Since most e-cigarettes contain nicotine, a highly addictive substance, it is worth evaluating how addiction to e-cigarettes influences the demand. Evidence from this research can facilitate policymakers’ decisions on regulating the consumption of e-cigarettes and provide evidence to address concerns of public health.
Regarding empirical research on addiction, several researchers test cigarette addiction and find evidence of that. Among them, using aggregated state-level yearly purchase data in 1955–1985 and fixed-effects two-stage least squares (2SLS) estimations, Becker et al. (Citation1994) reach an estimate of −0.78 for the long-run price elasticity of cigarette consumption and an estimate of −0.44 for the short-run price elasticity. With similar data, Baltagi and Griffin (Citation2001) revisit the estimation of Becker et al. (Citation1994) but use a forward-filter first-difference 2SLS and a generalized method of moments (GMM) estimator. They find evidence that is consistent with rational addiction but reach different estimates from different estimators: the short-run price elasticity ranges between −0.28 and −0.86 and the long-run price elasticity varies from −0.56 to −2.55. Instead of using aggregated macro data, Chaloupka (Citation1991) applies a micro data set and finds a long-run price elasticity of cigarette consumption ranging between −0.48 and −0.27.
Other researchers also evaluate cigarette taxation and demand using a dynamic model that incorporates consumers’ rational addiction. For example, Gordon and Sun (Citation2015) use Nielsen Household Panel Data from two submarkets in a large Midwestern city over 118 weeks; they reach a long-run price elasticity of cigarette consumption at −0.63 and a short-run price elasticity of −0.35. Sung et al. (Citation1994) investigate the effects of cigarette taxes on cigarette yearly consumption in 11 western states and find evidence of rational addiction; they obtain a short-run demand elasticity of −0.40 and an estimate of −0.48 for the long-run demand elasticity. The results of these articles show that a model incorporating addiction is valid and a tax increase reduces consumption more in the long run than in the short run.
Moreover, researchers have also empirically analyzed rational addiction to other goods. For example, Grossman and Chaloupka (Citation1998) show evidence of rational addiction to cocaine by young adults and find an estimate of − 1.35 for the long-run price elasticity of consumption. On alcohol rational addiction, Baltagi and Griffin (Citation2002) get a short-run price elasticity of −0.10 and a long-run price elasticity of −1.24; Grossman et al. (Citation1998) show that alcohol is less addictive than cigarettes, and they get an average short-run price elasticity of −0.41 and an average long-run price elasticity of −0.65. Additionally, Olekalns and Bardsley (Citation1996) test caffeine addiction in coffee consumption; Cameron (Citation1999) examines rational addiction and the demand for cinema; Cawley (Citation2000) examines the rational addiction to calorie consumption regarding eating patterns; Mobilia (Citation1993) provides evidence of rational addiction in gambling; Kwon et al. (Citation2016) suggest that consumers are rationally addicted to mobile social apps. Richards et al. (Citation2007) show evidence of carbohydrate addiction.
Most of this literature uses ordinary least squares (OLS) and fixed-effects 2SLS methods to estimate aggregate data. In comparison, the results of 2SLS are more variable than the ordinary least squares (OLS) estimates. Although typically the coefficients on the lag and lead of consumption are positive, some specifications present negative or statistically insignificant coefficient estimates for past and future consumptions. This might be due to the good not being addictive or consumers not anticipating future price movements. Lastly, estimates of the discount factor are highly variable and, in some cases, negative.
This article uses both macro data and microdata to test the rational addiction to e-cigarettes. The macro data is Nielsen Retail Scanner Data for weekly e-cigarette purchases throughout 2012–2017 in each region.Footnote1 The microdata is Nielsen Consumer Panel Data for quarterly e-cigarette purchases throughout 2012–2017 for each household. The purchase data are used to approximate the consumption of a representative consumer. This limitation may affect the generalization of the results. This article starts with a myopic model to test addiction and shows that consumers are addicted to e-cigarettes. Then, with a forward-looking model, this article finds evidence that consumers consider the future of their current e-cigarette consumption. With this evidence, we test both the elements of addiction and rationality in rational models using the OLS method and the strategy of fixed-effects 2SLS. In the estimations, we compare results from OLS with 2SLS which uses policies, prices, and taxes as IVs.
Results in all these models are consistent with the rational model predictions. With the macro data, we reach a short-run price elasticity of demand around −1.05 and a long-run price elasticity estimate of −1.50 from the 2SLS estimations. In contrast, with the microdata, we find a long-run price elasticity estimate of −1.50 from both the OLS and the 2SLS estimations. The long-run price elasticity estimate is −1.58 for e-cigarette ever-users and −1.48 for all households in the full sample when the depreciation rate of the additive stock is 100%. The results could not provide a precise estimate for the discount factor but we show that restricting the discount factor to different numbers does not influence estimates of the elasticities. Similarly, testing different numbers of the depreciation rate of the additive stock, the sign of the coefficients still shows that e-cigarettes are rationally addictive.
The next section introduces the theory behind the model and the model specification in our empirical analysis. The data section explains the data source and variables in the model. The last two sections review the results and conclusions.
2. Methods
According to the nature of addiction, consumption is dynamic. Utility in the current period depends on consumption in the same period and that in the previous period. In this article, we respectively apply two versions of rational addiction models for the macro and micro data.
2.1. Rational addiction model for macro data
The first version of the rational addiction model follows Becker et al. (Citation1994) (BGM) in which the consumer’s problem is to maximize the lifetime utility:
where assuming the rate of interest is equal to the rate of time preference,
and
are the quantities of e-cigarettes consumed in period
and period
. Furthermore,
is the consumption of a composite commodity in period
and is taken as the numeraire,
denotes the impact of unmeasured life-cycle variables on utility,
measures the initial level of e-cigarette consumption in period zero,
is the price of e-cigarettes in period
, and
is the present value of wealth, ignoring any effect of
on earnings or wealth.
This two-goods model assumes the utility function is quadratic in ,
, and
. Solving the first-order conditions for
, and substituting the result into the first-order condition for
, BGM gets a first-difference equation, in which the current e-cigarette consumption is a function of the past and future e-cigarette consumption, the current price of e-cigarettes, and the shift variables
and
:
In this equation, measures the effect of changes in past or future consumption on current consumption depending on the sign of this term. The definition is that a good is addictive when
. The larger is
, the larger the degree of addiction. See (Becker et al., Citation1994 pp. 398–399) for the restrictions on the coefficients. Recognizing that
is serially correlated affecting utility in each period and consumption at all periods through the optimizing behavior, BGM treat
and
as endogenous. To resolve the issue of potential inconsistent parameter estimates by OLS estimations, they use past and future prices as instrumental variables (IVs) for
and
, if the unobservables are uncorrelated with prices in these periods. Also, BGM includes other exogenous variables such as income, short and long-distance smuggling indexes, and taxes. Alternatively, Olekalns and Bardsley (Citation1996) provide a rational addiction model including leads and lags of prices as independent variables.
For our empirical estimation, we write a variant of (3) for a representative consumer as follows:
where the subscript denotes the
th region and the subscript
denotes the
th week in year
.
is the log of the total consumption of e-cigarettes (measured as total units of e-cigarettes purchased) for region
at week
in year
. Similarly,
denotes the log of the e-cigarette consumption in the previous week; and
represents the e-cigarette consumption in the one-period following week.
is the log of the purchase weighted average weekly retail price of e-cigarettes per unit.
indicates the per capita income in region
and year
. As in BGM,
measures the addictiveness of e-cigarettes;
is the rate of time preference assuming it is equal to the rate of interest. Positive coefficient estimates for
and
indicate that e-cigarettes are economically addictive, and consumers are forward-looking for e-cigarette consumption, respectively.
Furthermore, is a matrix of related observable policy covariates. These policies contain a continuous change in cigarette excise taxes per pack compared to 2009 in region
, year
, and week
; whether a region in a week of a year requires licenses for over-the-counter sales of e-cigarettes, restricts sales of e-cigarettes to minors, and has a smoke-free area (SFA) law that restricts uses of e-cigarettes.
is a vector of unknown parameters, representing the average mean effects of each of these policies over time. Moreover,
is region fixed-effects, controlling for unobserved non-time varying differences in demand across regions, for example, the marginal utility of wealth for a region in a model with perfect foresight;
is year-week or weekly fixed-effects, controlling for unobserved differences in demand over time that are commonly shared by regions; and
represents the unobserved time and region varying error term. With these fixed-effects applied in the model,
reflects forces associated with region-specific changes in the marginal utility of wealth over time.
This model does not directly estimate the effects of e-cigarette taxes on the current period of consumption but infers their effects indirectly when these taxes are used as IVs for lagged and future consumption if consumption is endogenous. In that case, the results section further discusses variables, in addition to e-cigarette taxes, used as IVs in regressions. Moreover, prices, taxes, and income are inflation-adjusted to the level of 2017 December with the monthly consumer price index (CPI)Footnote2 obtained from the Bureau of Labor Statistics. Furthermore, this model does not capture smuggling indexes. This is because, unlike cigarette taxes, current e-cigarette taxes are newly imposed and have lower rates on average if a region has a tax in place. As a result, e-cigarette taxes may not cause sizable “border purchasing effects” or smuggling effects like those of cigarettes (Becker et al., Citation1994) or alcohol ({Baltagi & Griffin, Citation1995 #72}; Baltagi & Griffin, Citation1995).
Also, following BGM, solving the second-order condition of EquationEquation 11(11)
(11) obtains the roots of the following quadratic equation:
The two roots are
with by concavity. Both roots are real and positive if and only if e-cigarettes are addictive (
). These estimates can be used to estimate derivatives of consumption changes with respect to e-cigarette price changes (see Appendix A in Becker et al., Citation1994 for a series of consumption responses to price changes). As the price and consumption in EquationEquation 11
(11)
(11) are in log forms, the derivatives obtained represent elasticities in this case.
These elasticity estimates contain short- and long-run demand elasticities for e-cigarettes, own-price anticipated effects and unanticipated effects, future-price unanticipated effects, as well as past-price unanticipated effects on the current consumption, . The cross-price elasticities between e-cigarette consumption levels at different times reflect the importance of addiction to aggregate e-cigarette consumption. In the model, when
decreases, it will increase e-cigarette consumption in the current period,
, and that in the next period,
, if e-cigarettes are addictive. If this price decrease is anticipated, the rise in the current period consumption also stimulates the consumption in the previous period,
. By accumulating decreases in the current price and future prices, a permanent decrease in e-cigarette price has a larger effect on current consumption than a temporary fall in price has.
2.2. Rational addiction model for microdata
The second version of the rational addiction model follows Chaloupka (Citation1991) in which the consumer’s utility is:
In EquationEquation 11(11)
(11) ,
is concave and has negative second derivatives,
is the quantities of e-cigarettes consumed in period
,
is the cumulative past e-cigarette consumption or the “addictive stock”, and
is a vector including inputs into the production of the composite good and health. Moreover, EquationEquation 11
(11)
(11) is a simple investment function describing the stock accumulation process, where
is the constant rate of depreciation of the addictive stock over time. E-cigarette consumption at time
can be considered as a gross investment in the addictive stock.
Assuming the rate of interest is equal to the individual’s rate of time preference
. Taking the first-order conditions for
,
, and
, then maximizing out with respect to
. Converting to discrete time, and using the resulting first-order conditions for
and
, we derive the demand equation as follows:
where is a matrix of related observable policy covariates for a region where household
resides in year
and quarter
. These policies contain a continuous change in e-cigarette taxes per milliliter, whether requiring licenses for over-the-counter sales of e-cigarettes, restricting sales of e-cigarettes to minors, and whether having a smoke-free area (SFA) law that restricts the use of e-cigarettes.
is a vector of unknown parameters, representing the average mean effects of each of these policies over time. Moreover,
is household fixed-effects, controlling for unobserved non-time varying differences in households;
is year-quarter or quarterly fixed-effects, controlling for unobserved differences in demand over time that are commonly shared by households; and
represents the unobserved time and household varying error term.
In the above demand equation, current consumption is predicted to be negatively related to current prices but positively related to past and future prices. Similarly, if e-cigarette is addictive, current consumption is expected to be positively related to past consumption. Also, if individuals are rational then current consumption is expected to be positively related to future consumption.
After testing the addictiveness and rationality of e-cigarette consumption, the next research interest is to estimate the long-run price elasticity of demand for e-cigarettes. Assuming, in the long run, a steady-state level of consumption is reached, it equals the depreciation of the optimal level of the addictive stock,
=
, where
is the optimal level of the addictive stock. When an anticipated permanent rise in price changes consumption and the optimal stock in each period, a new steady-state equilibrium can be achieved. The resulting long-run elasticity is
Similar to Becker and Murphy, the derived e-cigarette demand EquationEquation 11(11)
(11) is a second-order difference equation in current consumption. To describe the dynamic aspects of e-cigarette consumption, it is useful to obtain the two roots of the difference equation. The assumption of concavity implies that these roots are real. The two roots will be positive if and only if e-cigarettes are addictive. Thus, the signs of the roots depend on
, which is positive when consumption is addictive. The two roots for demand EquationEquation 11
(11)
(11) are
The small root, , shows the change in current consumption resulting from a shock to future consumption. The inverse of the larger root,
, indicates the impact of a shock to past consumption on current consumption. Shocks resulting from a change in any factor, including changes in past or future e-cigarette prices, affect the demand for e-cigarettes.
3. Data
The consumption data for e-cigarettes have two parts, macro data for purchases in regions and microdata for purchases by households.
3.1. Macro data
As to macro data, weekly purchase quantities and prices are from the Nielsen Retail Scanner Data between 1 January 2012, and 30 December 2017,Footnote3 in the U.S. The earliest usableFootnote4 data for e-cigarette purchases in this dataset begins in 2012. E-cigarette sales include total e-cigarette starter kits, refill cartridges, and disposables for a variety of outlets such as convenience stores, drug stores, and mass merchandisers. The total number of regions is 52, including all states and the District of Columbia, except for Illinois and Maryland; these two states are represented by five regions: Chicago, Cook County in Illinois (excluding Chicago), the rest of Illinois, Montgomery County in Maryland, and the rest of Maryland. Purchases for Alaska, Puerto Rico, U.S. Virgin Islands, and Hawaii are not available in the Nielsen data set, so they are not included in the list of regions. There are 313 weeks in total. Overall, these regions and weeks constitute 16,276 observations of e-cigarette purchases in region-week combinations. Regions have no gaps in purchases in the data.
Aside from the purchase data from Nielsen, the population data for each region in each year estimated on July 1, in thousands of persons, is from the U.S. Census Bureau. Purchases of e-cigarettes are stated per population of 1000. The per capita personal income in a region and a year is from the Bureau of Economic Analysis. It is total personal income divided by the total midyear population. The policy data is from the Centers for Disease Control and Prevention (CDC)’s State Tobacco Activities Tracking and Evaluation (STATE) System (2020b, Citation2020), the Campaign for Tobacco-Free Kids (Citation2020a, Citation2020b), and the Public Health Law Center (Citation2020), government websites, and consultations with designated officials of state and local jurisdictions.
In the models of this article, e-cigarette taxes are in dollars per milliliter ($/ml). If a region-week combination has an e-cigarette tax, the tax variable indicates the dollar amounts per ml; otherwise, the tax variable is zero. This article converts the original policy data of e-cigarette taxes into this measurement by first matching the purchase data with e-cigarette characteristicsFootnote5 (See Appendix for details of the conversion). The reason for the conversion is that the original policy data for e-cigarette taxes are not in the same measurement. Some jurisdictions apply ad valorem taxes by percentages of wholesale or retail prices, other jurisdictions tax e-cigarettes by specific excise taxes per ml of the liquid contained in the product. For example, Minnesota taxed e-cigarettes at 95% of the wholesale price in 2013 March and after; North Carolina imposed an e-cigarette tax at $0.050 per ml of the liquid in 2015 October and after. Aside from e-cigarette taxes, changes in cigarette excise taxes are dollars per pack compared to 2009 with zero indicating no tax changes; for other policies, if a region-week combination has a particular policy in place, the corresponding policy variable equals one, otherwise, it equals zero. Additionally, prices, income, and taxes are all inflation-adjusted by CPI to the level of 2017 December. shows a descriptive analysis of the variables in this paper.
Table 1. Descriptive analysis.
3.2. Microdata
To be compatible with the macro data, the micro dataset covers the same time window for estimations. Quarterly purchase quantities and prices are from the Nielsen Consumer Panel Data between 1 January 2012, and 30 December 2017, in the U.S. The e-cigarette purchase quantities and frequencies are relatively small so we have aggregated the weekly purchase data for each household to the quarter level. Then, we calculated the total quantity of e-cigarettes in milliliters (ml) that a household purchased and the average price for each ml of e-cigarettes purchased in each quarter.
The full sample includes 381,596 household-quarter observations from 26,175 households. From this full sample, we have extracted a subsample of “ever-users” which are households that have ever purchased e-cigarettes at least once. In all households of the full sample, 10.9% (2,865 households) are ever-users of e-cigarettes. In a quarter, on average, approximately 10.4% of households purchased e-cigarettes (2,716 households). The policy variables are similar to those in the macro data. shows the summary statistics of the full sample and the subsample of ever-users regarding purchases and corresponding policies.
4. Results
4.1. Macro data estimations
We start the estimation strategy with a myopic addiction model and then continue with a forward-looking model in . With these results as a foundation, we test a rational addiction model in . shows the results of myopic addiction behaviors and forward-looking behaviors separately. An addiction model predicts that the previous consumption reinforces the current consumption. If the goods are addictive, the coefficient of previous consumption is positive. Since the previous price () is a strong predictor of the previous consumption (
), using OLS estimation, column (1) provides some evidence that e-cigarettes are economically addictive as the coefficient for
is negative. Similarly, incorporating the previous consumption directly without the previous-period price, the coefficient of
is positive (not shown). However, as the model indicates, OLS estimates with the past consumption incorporated directly are inconsistent as
depends on
through the optimizing behavior implied in the first-order conditions. To resolve the endogenous variable problem for past consumption, following the literature, we obtain 2SLS estimates of myopic models of addiction.
Table 2. Estimates of E-Cigarette myopic addiction and forward-looking behaviors.
Table 3. Estimates of E-Cigarette rational addiction: Dependent variable = .
In column (2), past price and other explanatory variables in EquationEquation 11(11)
(11) are the instruments for past consumption. On top of column (2), column (3) adds the one-period lag values of the e-cigarette tax to the instruments; column (4) further adds the current period e-cigarette tax; on top of all these, column (4) further adds the two-period lagged price and the corresponding tax to the instrument list. According to the parameter estimates of the myopic model in , current price negatively relates to current e-cigarette consumption, but income positively relates to that. The positive and statistically significant estimates for past consumption indicate that e-cigarette use is addictive. Except for restrictions on sales to minors get a negative coefficient, all policies get positive coefficient estimates for all estimations.
The forward-looking specifications suppose consumers consider the amount that they would consume in the future for their current levels of e-cigarette consumption. Like past consumption, future consumption is endogenous if incorporated directly into the model for current consumption because depends on
through optimizing behavior. The results of such a regression with OLS estimation indicate that consumers are forward-looking for e-cigarette consumption (not shown). In a similar fashion as the myopic model, column (6) shows that future price negatively influences current consumption, again suggesting that consumers are forward-looking. Columns (7) – (10) use different sets of instruments for future consumption. In column (7), future price and other explanatory variables in EquationEquation 11
(11)
(11) are the instruments for future consumption. On top of column (7), column (8) adds the one-period lead values of the e-cigarette tax to the instruments; column (9) further adds the current period e-cigarette tax; on top of all these, column (10) further adds the two-period lagged price and the corresponding tax to the instrument list. All these specifications show that consumers are forward-looking as the coefficient estimates for future consumption are all positive and statistically significant.
What separates a myopic addiction model from a rational addiction model is that the rational addiction model supposes that consumers are both addictive and forward-looking for e-cigarette consumption, while the myopic addiction model implies that consumers would not take the future amount of consumption into account in the current period of consumption. Thus, if the data supports the myopic addiction model, the coefficient of the lagged consumption would be positive and statistically significant, and the coefficient of the lead consumption or price would not be statistically significant. In contrast, the rational addiction model predicts the coefficient of lead consumption would be positive and statistically significant. The OLS estimates in column (1) of show evidence of rational addiction.
To resolve the inconsistency problem as in , we use IVs for the endogenous lagged and lead consumptions in the 2SLS specifications. In , the instruments in column (2) consist of the one-period lag and lead of prices respectively for the corresponding consumption and other explanatory variables in the model. On top of column (2), column (3) adds the one-period lag and lead of taxes to the corresponding consumption. With these IVs included, column (4) further adds the current period tax as an IV; column (5) additionally adds the two-period lagged price and tax to the instrument list. All these specifications are consistent with the hypothesis that consumers are rationally addicted to e-cigarettes as the coefficient estimates for future and past consumptions are both positive and statistically significant. In these specifications, using taxes as IVs assumes consumers have more information about taxes than prices. The inclusion of both taxes and prices as IVs allows for the possibility that consumers have information about the tax-exclusive price.
Based on the corresponding columns in shows additional parameter estimates and elasticities of e-cigarette consumption in response to various price changes at the sample means of price and consumption. Consistent with the predictions of the rational model, the estimates of the discount factor are positive and the two roots of EquationEquation 11
(11)
(11) are positive. An unanticipated price change assumes that the price change is not anticipated until the corresponding period. In the 2SLS estimations, a 10-percent temporary increase in the current price of e-cigarettes decreases current consumption by 6.9–8.8 percent if the price change is anticipated; in contrast, it decreases current consumption by 6.1–8.5 percent if it is unanticipated (see rows for own price anticipated and unanticipated in ). As to cross-price effects, a 10-percent unanticipated increase in current price leads to a 2.3–2.6 percent decrease in the previous period’s consumption (see the row for future price unanticipated) and to a 1.2–1.8 percent decrease in the next period’s consumption (see the row for past price unanticipated).
Table 4. Parameters and elasticities based on model results of rational addiction.
Moreover, the long-run response of a permanent price change of 10 percent leads to a 13.6–14.9 percent decrease in demand. A 10-percent price increase causes a 10.5–11.8 percent decrease in e-cigarette consumption in the short run. The estimates of the long-run price elasticity are 1.2–1.4 times those for the short-run. All these elasticity estimates provide evidence that e-cigarettes are addictive and rational: past and future price changes have a statistically significant influence on current consumption. However, the estimates for the discount factor in these specifications correspond to negative interest rates ranging between −0.323 to −0.513. Additionally, the OLS estimation provides support for rational addiction though the coefficient estimates could be inconsistent. Column (1) in indicates that the long-run price elasticity in the OLS model is −3.304 and the short-run elasticity is −1.007. Therefore, OLS and 2SLS estimations all provide evidence that consumers are rationally addicted to e-cigarettes.
4.2. Microdata estimations
With the microdata for households, we test if consumers are rationally addicted to e-cigarettes in . Results show estimates of the demand EquationEquation 11(11)
(11) , the long-run price elasticity of demand, and the roots of the difference equation. The upper panel displays OLS estimations and the lower panel reports 2SLS estimations. These estimations contain estimates for both the full sample and the subsample of e-cigarette ever-users. The left two columns in each panel contain estimations under the assumption of a 100 percent rate of depreciation, while the right two columns contain estimates with no assumption imposed about the rate of depreciation. Imposing a 100 percent rate of depreciation lead to the exclusion of past and future prices from the demand equation. However, this assumption does not imply that e-cigarettes are not addictive since past, current, and future consumption are still included in estimations.
Table 5. Estimates of E-Cigarette rational addiction in restricted models.
The 2SLS estimations tackle the endogeneity of past and future consumption. As in the demand equation, the current consumption is a function of one lag of consumption, one lead of consumption, and one lag, current, and one lead of e-cigarette prices. Thus, current consumption is independent of other past and future prices. In the fully reduced form, e-cigarette consumption at any time is a function of all past, current, and future e-cigarette prices, implying that further lags and leads of prices are suitable instruments for lagged and led consumption. Consequently, the set of IVs includes four lags of price, current price, and four leads of price and four lags, current, and four leads of e-cigarette taxes. The set of taxes is intended to reduce collinearity problems.
As the model predicts, if the goods are addictive, the coefficient of previous consumption is positive; if the consumers are forward-looking, the coefficient of future consumption is also positive. In all estimations, the coefficients on past and future consumption conform to the predictions for rational addiction, consistent with the predictions of the Berker-Murphy theoretical model. Also, as anticipated, current consumption is negatively related to the current price. Past and future prices, when included, have a positive effect on current consumption.
With the assumption of a 100 percent rate of depreciation, the estimated long-run price elasticity for the full sample ranges from −1.48 to −1.50, and for the subsample of ever-users ranges from −1.57 to −1.58. The average of the long-run price elasticities of demand reported is around −1.50. With no assumed rate of depreciation, the estimations support the rational addictiveness of e-cigarettes but the estimates for the long-run price elasticity are unstable. For accuracy, we use the estimates from the assumption of a 100 percent rate of depreciation as the final results for the microdata.
The estimated roots from the demand equation demonstrate the intertemporal effects of a shock to past and future consumption. Analyzing the estimated roots using the full sample and the assumption of a 100 percent rate of depreciation, one can find that a shock that would decrease consumption by 10 percent in the future would result in a decrease in current consumption between 3 and 5 percent. Similarly, shocks that reduced past consumption by 10 percent would decrease current consumption by between 2 and 5 percent. These effects are quite similar for the subsample of ever-users.
5. Discussion
The estimations using macro data do not provide reliable estimates for the discount factor. The estimate for the discount factor is 1.072, corresponding to an interest rate of −0.040 Follow BGM, imposes the discount factor a priori, ranging from 0.70 to 0.95 (interest rates ranging from 5.3 percent to 42.9 percent). This means that we constrain the coefficient for future consumption to be equal to the imposed discount factor multiplied by the estimated coefficient of past consumption. Model specifications in are similar to those of columns (2) and (3) in except for separating the year-week fixed effects into year fixed-effects, month fixed-effects, and week fixed-effects. The unrestricted estimates for price coefficients, past-consumption coefficients, long-run price elasticities, and short-run elasticities are very close to those of . Therefore, the results of are comparable to those of the two tables.
Table 6. Estimates of E-Cigarette rational addiction: Dependent variable= (Evidence from microdata).
Estimates of indicate that regardless of the restriction of the discount factor, estimates of the long-run and short-run price elasticities are very similar to each other. Further comparing these estimates in , column (4) is the preferred specification, which reaches a long-run price elasticity of about −1.50 and the short-run price elasticity of −1.05, as taxes seem to be important predictors for consumption. The main difference between the OLS and 2SLS estimates is that OLS estimates −3.30 for the long-run price elasticity estimate and −1.01 for the short-run estimate.
The results in and 6 do not pin down the discount factor with precision. This problem is also found in other studies of aggregate consumption, the consumption of specific goods, or the consumption of leisure over time. Some of these studies reach very high-interest rates while others obtain low or negative interest rates (Bover, Citation1991; Epstein & Zin, Citation1991; Hansen & Singleton, Citation1983; Hotz et al., Citation1988; Mankiw et al., Citation1985). Overall, our model estimates are not sensitive to the choice of discount factors. The long-run and short-run price elasticity estimates reached from different sets of instruments are comparable to each other.
Comparing the results from the macro data with those from the microdata, both support the rational addictiveness of e-cigarettes. The estimated long-run price elasticities of demand are both around −1.50 when using micro and macro data. Nonetheless, the estimated intertemporal links in consumption are somewhat stronger using microdata than using macro data. Additionally, the models rely on assumptions that include consumers’ time-consistent preferences and complete information for decision-making. Potential violations of assumptions could affect the results.
6. Conclusions
We investigate addictive and forward-looking behavior and find evidence for both behaviors. With macro data, we test a rational addiction model inspired by BGM. Similarly, we test a rational addiction model inspired by Chaloupka using microdata. Results provide evidence that e-cigarettes are rationally addictive rather than myopically addictive. Results from both datasets support the rational addictiveness of e-cigarettes. With the rational addiction models, the estimated long-run price elasticities of demand are both around −1.50. Our estimate for the short-run price elasticity is −1.05 when we use macro data. These results indicate that e-cigarettes are price elastic. Using the same data source and analysis methods, we have conducted similar regressions for the consumption of cigarettes as a comparison for e-cigarettes (not shown, available upon request). Results indicate that the estimated coefficient for e-cigarette addictiveness (0.26–0.39) is smaller than that for cigarettes (0.49).
Furthermore, the estimate of short-run price elasticity of cigarette consumption is −0.48 while the estimate for e-cigarettes is very close to one (−1.05). This comparison shows that raising taxes on e-cigarettes, if the taxes influence consumption by increasing price, may not generate as much revenue as cigarette taxes in the short run; however, e-cigarette taxes could be a more effective temporary tool for tobacco control of e-cigarettes as the demand of the product is elastic. For both products, the long-run tax effects would be stronger than the short-run effects.
List of abbreviations
Two-Stage Least Squares | = | (2SLS) |
Generalized Method of Moments | = | (GMM) |
Ordinary Least Squares | = | (OLS) |
Becker, Grossman, & Murphy | = | (BGM) |
Instrumental Variables | = | (IVs) |
Consumer Price Index | = | (CPI) |
Centers for Disease Control and Prevention | = | (CDC) |
State Tobacco Activities Tracking and Evaluation | = | (STATE) |
Fixed-Effects | = | (FE) |
Authors’ contributions
XD conceptualized the research design, analyzed data, interpreted the results, and wrote the original draft. YZ and JB reviewed and edited the manuscript. All authors read and approved the final manuscript.
Consent for publication
The e-cigarette consumption data is from Nielsen and given consent for publication.
The e-cigarette characteristics are from Cotti et al. (Citation2018) and Cotti et al. (Citation2020). These data are also given consent by them.
Acknowledgments
Cotti et al., (Citation2018) and Cotti et al., (Citation2020) are acknowledged for their generosity in providing e-cigarette characteristics data.
Disclosure statement
No potential conflict of interest was reported by the author(s).
Data availability statement
The consumption datasets analyzed during the current study are available from Nielsen Consumer LLC and marketing databases provided through the Nielsen IQ Datasets at the Kilts Center for Marketing Data Center at The University of Chicago Booth School of Business.
The e-cigarette characteristics datasets used during the current study are available from the corresponding authors of Cotti et al. (Citation2018) and Cotti et al. (Citation2020) on reasonable request.
Additional information
Notes on contributors
Xueting Deng
Xueting Deng, is an Economist in the Career Science Lab, BOSS Zhipin, Beijing, China. Her research interests are policy evaluation, health economics, and labor economics.
Yuqing Zheng
Yuqing Zheng, is the H. B. Price Associate Professor at the Department of Agricultural Economics, University of Kentucky. His research interests are food marketing/policy, and food safety and labeling.
J.S. Butler
J.S. Butler, is a Professor at the Martin School of Public Policy and Administration, University of Kentucky. His research interests are econometrics and statistics, as well as their application in diverse fields.
Notes
1 In most cases, a region is the same as a state, except for adjusting local jurisdictions with e-cigarette taxes; accordingly, Illinois and Maryland become five regions including Chicago, Cook County in Illinois (excluding Chicago), the rest of Illinois, Montgomery County in Maryland, and the rest of Maryland.
2 We apply non-cigarette tobacco CPI for e-cigarette prices and taxes, cigarette CPI for cigarette excise taxes, and all goods CPI for per capita income.
3 The latest data available when we started this article was the 2017 data. Later, Nielsen has the 2018 data available. Adding additional data from one more year would not change much of our results, as when the data in 2012 or 2017 are removed, results are similar to that with the data included.
4 Some e-cigarette purchases happening before 2012 are missing because many states did not sell e-cigarettes then.
5 These e-cigarette characteristics are from Cotti et al. (Citation2018) and Cotti et al. (Citation2020). These authors are acknowledged for their generosity in sharing the data.
References
- Ali, F. R. M., Diaz, M. C., Vallone, D., Tynan, M. A., Cordova, J., Seaman, E. L., Trivers, K. F., Schillo, B. A., Talley, B., King, B. A., & Centers for Disease Control and Prevention. (2020). E-cigarette unit sales, by product and flavor type — United States, 2014–2020. MMWR Morbidity and Mortality Weekly Report, 69(37), 1313–21. https://doi.org/10.15585/mmwr.mm6937e2
- Baltagi, B. H., & Griffin, J. M. (1995). A dynamic demand model for liquor: The case for pooling. The Review of Economics and Statistics, 77(3), 545–554. https://doi.org/10.2307/2109914
- Baltagi, B. H., & Griffin, J. M. (2001). The econometrics of rational addiction: The case of cigarettes. Journal of Business & Economic Statistics, 19(4), 449–454. https://doi.org/10.1198/07350010152596691
- Baltagi, B. H., & Griffin, J. M. (2002). Rational addiction to alcohol: Panel Data analysis of liquor consumption. Health Economics, 11(6), 485–491. https://doi.org/10.1002/hec.748
- Becker, G. S., Grossman, M., & Murphy, K. M. (1994). An empirical analysis of cigarette addiction. The American Economic Review, 84(3), 396–418. https://doi.org/10.3386/w3322
- Becker, G. S., & Murphy, K. M. (1988). A theory of rational addiction. Journal of Political Economy, 96(4), 675–700. https://doi.org/10.1086/261558
- Bover, O. (1991). Relaxing intertemporal separability: A rational habits model of labor supply estimated from panel data. Journal of Labor Economics, 9(1), 85–100. https://doi.org/10.1086/298260
- Calvert, R. L. (1985). The value of biased information: A rational choice model of political advice. The Journal of Politics, 47(2), 530–555. https://doi.org/10.2307/2130895
- Cameron, S. (1999). Rational addiction and the demand for cinema. Applied Economics Letters, 6(9), 617–620. https://doi.org/10.1080/135048599352736
- Campaign for Tobacco-Free Kids. (2020a). State cigarette excise tax rates & rankings. https://www.tobaccofreekids.org/assets/factsheets/0097.pdf
- Campaign for Tobacco-Free Kids. (2020b). State excise tax rates for non-cigarette tobacco products.
- Cawley, J. H. (2000). Rational addiction, the consumption of calories, and body weight.
- Centers for Disease Control and Prevention. (2020). State Tobacco Activities Tracking and Evaluation (STATE) system.
- Chaloupka, F. (1991). Rational addictive behavior and cigarette smoking. Journal of Political Economy, 99(4), 722–742. https://doi.org/10.1086/261776
- Cotti, C., Courtemanche, C., Maclean, C., Nesson, E., Pesko, M., & Tefft, N. 2020. The effects of E-Cigarette taxes on e-cigarette prices and tobacco product sales: Evidence from retail panel data. NBER Working Paper, (w26724).
- Cotti, C., Nesson, E., & Tefft, N. (2018). The relationship between cigarettes and electronic cigarettes: Evidence from household panel data. Journal of Health Economics, 61, 205–219. https://doi.org/10.1016/j.jhealeco.2018.08.001
- Epstein, L. G., & Zin, S. E. (1991). Substitution, risk aversion, and the temporal behavior of consumption and asset returns: an Empirical Analysis. Journal of Political Economy, 99(2), 263–286. https://doi.org/10.1086/261750
- Gordon, B. R., & Sun, B. (2015). A dynamic model of rational addiction: Evaluating cigarette taxes. Marketing Science, 34(3), 452–470. https://doi.org/10.1287/mksc.2014.0885
- Grossman, M., & Chaloupka, F. J. (1998). The demand for cocaine by young adults: A rational addiction approach. Journal of Health Economics, 17(4), 427–474. https://doi.org/10.1016/S0167-62969700046-5
- Grossman, M., Chaloupka, F. J., & Sirtalan, I. (1998). An empirical analysis of alcohol addiction: Results from the monitoring the future panels. Economic Inquiry, 36(1), 39–48. https://doi.org/10.1111/j.1465-7295.1998.tb01694.x
- Hansen, L. P., & Singleton, K. J. (1983). Stochastic consumption, risk aversion, and the temporal behavior of asset returns. Journal of Political Economy, 91(2), 249–265. https://doi.org/10.1086/261141
- Hotz, V. J., Kydland, F. E., & Sedlacek, G. L. (1988). Intertemporal preferences and labor supply. Econometrica: Journal of the Econometric Society, 56(2), 335–360. https://doi.org/10.2307/1911075
- Kwon, H. E., So, H., Han, S. P., & Oh, W. (2016). Excessive dependence on mobile social apps: A rational addiction perspective. Information Systems Research, 27(4), 919–939. https://doi.org/10.1287/isre.2016.0658
- Mankiw, N. G., Rotemberg, J. J., & Summers, L. H. (1985). Intertemporal substitution in macroeconomics. The Quarterly Journal of Economics, 100(1), 225–251. https://doi.org/10.2307/1885743
- Mobilia, P. (1993). Gambling as a rational addiction. Journal of Gambling Studies, 9(2), 121–151. https://doi.org/10.1007/BF01014864
- Olekalns, N., & Bardsley, P. (1996). Rational addiction to caffeine: An analysis of coffee consumption. Journal of Political Economy, 104(5), 1100–1104. https://doi.org/10.1086/262054
- Public Health Law Center. (2020). U.S. E-Cigarette regulations - 50 state review (2020). https://publichealthlawcenter.org/resources/us-e-cigarette-regulations-50-state-review
- Richards, T. J., Patterson, P. M., & Tegene, A. (2007). Obesity and nutrient consumption: A rational addiction? Contemporary Economic Policy, 25(3), 309–324. https://doi.org/10.1111/j.1465-7287.2007.00047.x
- Ryder, H. E., & Heal, G. M. (1973). Optimal growth with intertemporally dependent preferences. The Review of Economic Studies, 40(1), 1–31. https://doi.org/10.2307/2296736
- Sung, H. Y., Hu, T. W., & Keeler, T. E. (1994). Cigarette taxation and demand: An empirical model. Contemporary Economic Policy, 12(3), 91–100. https://doi.org/10.1111/j.1465-7287.1994.tb00437.x
- U.S. Department of Health and human services. 2016. e-cigarette use among youth and young adults: A report of the surgeon general.
APPENDIX Appendix: Two Methods for Converting E-Cigarette Taxes to Dollars Per Milliliter
Jurisdictions tax e-cigarettes mainly in two ways: ad valorem taxes, based on the wholesale or retail price, or specific excise taxes, according to the volume of the liquid contained. These taxes are levied on wholesalers or retailers. We call the ad valorem taxes price tax (P.Tax) and use the volumetric tax (V.Tax) to refer to specific excise taxes. By the end of 2017, Minnesota, the District of Columbia, California, and Montgomery County in Maryland tax e-cigarettes by percentages of the wholesale price; Pennsylvania taxes e-cigarettes by percentages of the retail price. In contrast, North Carolina, Louisiana, West Virginia, Kansas, and Cook County in Illinois tax e-cigarettes per fluid milliliter (ml) of the liquid. The city of Chicago in Illinois uses V.Tax not only via taxing per fluid ml of the liquid but also adds taxes per unit of e-cigarettes. To compare taxes in these regions, it is necessary to measure all taxes in the same unit. Thus, we convert P.Tax and the V.Tax in the city of Chicago to the dollar amounts per ml. We reach very similar results when using the following two, completely different ways to convert the P.Tax.
a E-Cigarette Tax Conversion Based on Wholesale Price Calculations
The first method conducts the conversion by calculating the wholesale price. It uses retail prices in a region to compute wholesale prices based on guidelines of cigarette minimum price laws. According to the guidelines of Publication 509 from the Department of Taxation and Finance in New York State (2020), the retail price of cigarettes is at least 7% higher than the agent-to-retail-dealers minimum selling price. We assume the pricing mechanisms are the same for e-cigarettes, i.e., the price raising rates are the same from manufacturers, to wholesale dealers, to retail dealers, then to consumers. Thus, we have three steps. First, we divide the average retail price of e-cigarettes per ml for region in week
by 1.07, giving us the post-tax wholesale price. Second, by dividing this price with
, we get the pre-tax wholesale price. Third, by multiplying the pre-tax wholesale price with the tax rate for the current region-week observation, we get the e-cigarette tax per ml in this region-week combination.
Specifically, the formula is:
The formula for calculating the average retail price of e-cigarettes per ml is:
b E-Cigarette Tax Conversion Based on DC Taxes
The second method also has three steps for conversion. In the first step, it uses the District of Columbia’s (DC’s) tax to compute the dollar amounts for each percentage point of P.Tax, since the e-cigarette tax rate in DC matches 100% of the traditional cigarette excise tax at the state level. In the second step, we reach the total tax revenues for this region-week combination by multiplying the total percentage points of the ad valorem taxes by the value of each percentage point (PPV) from the first step and the total sales quantities in units for the region in week
. In the third step, dividing the tax revenues by the total sales quantities in ml gets the tax rate per ml. Since e-cigarette taxes in DC vary each year due to yearly changes in cigarette excise taxes, we reach different PPVs each year. Generally, the value increases by about $0.002 each year. For example, values in 2015 and 2016 were $0.043 and $0.045 respectively. We adjust the PPV each year and use these values in our calculations to be accurate.
Specifically, the formula for this conversion is:
E-Cigarette Tax in the City of Chicago, Illinois
Chicago initially taxes e-cigarettes at $0.80 per unit plus $0.55 per ml. Cook County, to which Chicago belongs, implements an e-cigarette tax of $0.20 per ml, therefore making the total tax rate per ml $0.75 in Chicago. For taxes in Chicago, we use the following formula: