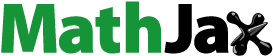
ABSTRACT
Parenthood have a significant impact on engagement in the labor force. Parents may decide to look for jobs with specific characteristics, and in some cases, they may decide to not participate in the labor market. Using survey data from Chile, we use a propensity score matching estimation to compare various labor outcomes of women and men with and without children. We found that maternity has an impact on several dimensions of labor engagement. To study how these multidimensional effects are compounded at the individual level, we use a quality of employment (QoE) index to estimate the drop in job quality post-childbirth. We found a drop in quality only for mothers. Moreover, for less educated women, the drop in QoE is equivalent to no longer being hired under contract. For single mothers, the drop is equivalent to a reduction in wages that would make the family fall below the poverty line.
1. Introduction
Gender gaps manifest in many forms in the labor force. They can be found when comparing wages, earnings, hours worked and labor force participation (Angelov et al., Citation2016; Gasparini & Marchionni, Citation2017; Petrongolo & Olivetti, Citation2008). One potential cause of these gaps are differences in gender composition throughout the labor force. For instance, there are different gender compositions across sectors, firm size and other firm characteristics (Petrongolo & Ronchi, Citation2020). One potential mechanism for these differences in gender composition in the labor market is childbirth, because parenthood can impact labor market decisions differentially across genders. The main reason for this differential impact of a child in how to engage in the labor market is related to the decision of how to provide childcare within the household (Kleven, Landais, & Søgaard, Citation2019; Kleven, Landais, Posch, et al., Citation2019). Consequently, maternity is key to understand the overall gender gap, because if mothers are more impacted than fathers in the labor market, then the composition of mothers in the labor force may explain part of the difference in the gender composition in the labor market, and ultimately the gender gaps.
There is an abundance of empirical evidence of how maternity can change the labor engagement of mothers (Angelov et al., Citation2016; Berniell et al., Citation2020, Citation2021, Citation2023; Kleven & Landais, Citation2017; Kleven, Landais, & Søgaard, Citation2019; Lundborg et al., Citation2017). Interestingly, the impact of maternity is not only related to the effect of maternity leave on the job ladder but maternity also affects the characteristics of the job post-childbirth in terms of hours, wage schedule, preferred sectors type of contract and overall job quality. Therefore, one important step when studying labor gender gaps is to understand the changes that maternity produces in how mothers engage with the labor market. Kleven, Landais, and Søgaard (Citation2019) presented an approach to study how maternity can affect the way mothers participate and engage in post-childbirth job search. Using an event study with Danish administrative data, Kleven illustrates the different labor paths and labor histories of women with and without children. He showed that having a child affected labor force participation, hours worked, earnings and wages. More importantly, he also showed that childbirth may change the type of firms mothers ended up working for. These conclusions are consistent with the situation of women in Latin America. Many recent studies have showed the negative effects on wages, working hours, labor formality, paid work, and others important labor outcomes that childbirth could have for the women of this region (Aguilar-Gomez et al., Citation2019; Berniell et al., Citation2020, Citation2021, Citation2023; Querejeta & Bucheli, Citation2021).
In their study, Berniell et al. (Citation2021) examine the impact of maternity on women’s occupational structure in Chile. They explore various aspects of labor engagement and labor quality, including labor supply, earnings, temporary employment, self-employment, and more, focusing on changes that occur after the birth of the first child. The study reveals a significant shift towards informal employment as the primary effect, which offers a more flexible work schedule. It is worth noting that transitioning from formal to informal employment entails simultaneous changes across multiple dimensions of labor engagement. Berniell et al. (Citation2020, Citation2023) show that the effects of maternity in several dimensions of the labor market are also present in various Latin American countries. These findings indicate that motherhood leads to a structural transformation in how women participate in the labor market. Therefore, we propose the adoption of a multidimensional job quality index to encompass all the diverse adjustments that mothers undergo following the birth of their first child. Using this multidimensional index allows us to quantify the overall effect of maternity on labor engagement.
The excellent quality of the data available in Chile allows us to tackle some of the interesting questions arising from Kleven’s results. First, we study the multidimensional effect of childbirth on mother´s labor engagement using an estimation that relies on a propensity score matching process. As expected, we found that having a child mostly affects mothers, and that the effect goes beyond earnings and hours. In particular, we also found effects on many other labor dimensions such as participation in the labor force, contractual status, size of the firm, and the probability of being self-employed. Interestingly, we found that educational attainment plays an important role in how motherhood affects labor outcomes. In particular, we found that childbirth affects more mothers with less education.
However, the propensity score matching estimations, or the event study used in various papers in the literature (for instance Kleven, Landais, & Søgaard, Citation2019; Kleven, Landais, Posch, et al., Citation2019) does not allow to study if these multidimensional effects impact mothers simultaneously, or they are just an array of potential effects. In other words, the aforementioned estimation strategies are unable to capture the total effect of maternity, because they estimate the effect of childbirth on specific dimensions of the labor market engagement. Thus, to assess the full impact of maternity on the labor engagement, we should study whether the total maternity impact is the sum of these different partial effects, or whether it is more common to observe a single effect in each mother.
We use the Quality of Employment index (QoE) developed by Sehnbruch et al. (Citation2020), to estimate the change in employment quality after childbirth.Footnote1 The notion of employment quality allow us to capture the multidimensional aspect of the change in labor engagement post-childbirth. Additionally, once we estimate the multidimensional effect, we can calculate its equivalent one-dimensional effect, and compare it with the existing literature. Our estimations show that the impact of having a child for less educated women is equivalent, in terms of a drop in the QoE, to no longer being hired under contract. For single mothers, childbirth has an effect that is equivalent to a reduction in wages severe enough to put the family below the poverty line.
These equivalencies show that when accounting for the multidimensional effects, the impact of childbirth on women is higher than the effects estimated using just a single dimension. Thus, these equivalences show that the impact of maternity is expressed in various nuanced changes throughout the different individual dimensions of the labor engagement.
2. Literature review
The gender gap has been widely studied in the literature during the last decades and it has been analyzed from many different dimensions. One important goal of this research is to understand the many different causes of this inequality. One dimension of gender inequality is the difference in the labor force participation rate across genders. Fortunately, in Latin America, the employment rate of women has risen more than that of men, meaning that women absorb most of the jobs created, and informal work and the informal gap between genders has also been reduced (Abramo & Valenzuela, Citation2005). Despite that this gap has been reduced over the last decades, the speed of growth in female labor participation in recent years has slowed down in Latin America (Gasparini & Marchionni, Citation2015, Citation2017).
Female labor force participation is well below the OECD average in several Latin American countries (Contreras et al., Citation2011). Moreover, research shows that poor women’s unemployment rate has risen and the unemployment gap between men and women has, in some cases, widened (Gasparini & Marchionni, Citation2015, Citation2017). Work formality for working women has increased, but the proportion of women working in the informal sector as a percentage of the total female employed population is higher than the proportion of men in the same situation (Abramo & Valenzuela, Citation2005). In poor countries, girls acquire less education than boys, and there is less investment in women’s health (Dollar & Gatti, Citation1999). In many Latin American countries, specifically in Chile, females currently exhibit, on average, more years of education than males but the work inequalities between genders are still present (Ñopo, Citation2007). Most of the research studying the causes of these various gender gaps indicates the importance of many different mechanisms. For instance, gender gaps are related to labor force composition (Macpherson & Hirsch, Citation1995), differences in human capital accumulation (Kuépié, Citation2016), fertility (Kleven & Landais, Citation2017), childcare and intrahousehold production (Kaul, Citation2018), gender differences in values and preferences (Farre & Vella, Citation2013), employment access (Galarza & Yamada, Citation2017) and macroeconomic development.
Measuring the gender income gap using hourly wages typically leads to a considerable gender gap. However, this result may conceal more nuanced gender differences in the labor force composition. It is common to find different sectorial compositions across genders, or gender differences when comparing labor composition using age, education and other individual characteristics. Additionally, it is common that far more women work in part-time jobs than men. This fact is important, because women working full-time and women working part-time are very different in characteristics and have very different jobs. The part-time penalty for identical women doing the same job is 3% in the UK when accounting for occupation (Manning & Petrongolo, Citation2008). And a statistically significant relationship between age and participation is found (Contreras & Plaza, Citation2010). Therefore, a simple mean comparison across genders understates the potential differences in labor trajectories and how different are the labor engagements across genders.
Two important potential causes of gender differences are fertility decisions and home production. The presence of young children in the household seems to discourage women’s participation in the labor force (Contreras & Plaza, Citation2010). The effects found on women’s labor outcomes attributable to children are considerably larger than men’s and these effects do not decrease over time. A measurable “child penalty” has been found in the literature (Andresen & Nix, Citation2021; Angelov et al., Citation2016; de Quinto et al., Citation2021; Kleven & Landais, Citation2017; Kleven, Landais, & Søgaard, Citation2019; Kleven, Landais, Posch, et al., Citation2019): the careers of men and women evolve at the same rate until the birth of their first child, diverge immediately and never converge again. This child penalty is around 20% (Kleven, Landais, & Søgaard, Citation2019) and seems to come from at least three different sources: participation, hours worked and income.
Women are particularly disadvantaged in their ability to match desired work hours to actual work hours given their responsibility for child and family care (Kuziemko et al., Citation2018; Ruppanner et al., Citation2018). In many occupations, earnings have a nonlinear relationship with respect to hours, and hours of work are worth more when given more continuously (Goldin, Citation2014). Thus, many women might choose not to work, especially if they have a partner that contributes a sufficient income (Angelov et al., Citation2016; Cohen & Blanchi, Citation1999). Furthermore, women tend to lower their rank in the company they work for after having children or change their working status from full-time to part-time, usually making a downward occupational move and moving to a more family friendly workplace or industry (Kleven, Landais, & Søgaard, Citation2019; Manning & Petrongolo, Citation2008). It is important to note that the income gap exacerbates this cycle, because it affects intrahousehold decisions through the differences in partner income.
There are many women not working or working in part-time jobs not using the full extent of their skills and losing occupational experience, even though they invested almost the same in education as men, therefore paying the “child penalty” after being mothers (Angelov et al., Citation2016; Kleven, Landais, & Søgaard, Citation2019; Kleven, Landais, Posch, et al., Citation2019; Manning & Petrongolo, Citation2008). Although greater levels of education increase the probability of women participating in the labor force and women with more years of education have become increasingly likely to commit hours to market work overtime, returns to education are significantly lower for women than men (Cohen & Blanchi, Citation1999; Contreras & Plaza, Citation2010; Gasparini & Marchionni, Citation2015, Citation2017; Montenegro, Citation2001). This difference in returns to education may also lead to differences in human capital accumulation decisions and exacerbate the gender differences in occupation.
Preferences also play a role in female labor force participation. The idea that a lower female participation for mothers in the labor market is related to biological components driven has been disproved by the recent literature. Kleven et al. (Citation2021) show that the child penalty is similar for parents who gave birth and who adopted a child, whilst Andresen and Nix (Citation2021) show that female same sex couples do present a child gap initially, but it disappears after four years. These studies support the idea that the child penalty is mostly driven by culture and beliefs, rather than biologically. The perception of women as homemakers is found to be associated with lower female participation and women’s labor outcomes. There is a negative correlation between cultural values upholding traditional gender roles and a woman’s decision to participate in the labor market (Contreras & Plaza, Citation2010; Fortin, Citation2005). On the one hand, a flexible work culture benefits mothers, especially those who are less likely to choose a full-time job (Ruppanner et al., Citation2018). On the other hand, childbirth increases the likelihood of women participating in the informal labor market due to its flexible schedules (Berniell et al., Citation2021). Interestingly, public policies aimed to decrease gender gaps and child penalty in women have shown only small effects on a mother’s labor outcomes and long-term child penalty. For instance, equating the parental leave across genders to reduce difference across genders have not shown long-term effect on mother’s child penalty (Andresen & Nix, Citation2021; Kleven et al., Citation2020; Kleven, Landais, Posch, et al., Citation2019). In the same way, heavily subsidized childcare had little to no effect in the decline of the gender gaps (Kleven et al., Citation2020; Kleven, Landais, Posch, et al., Citation2019).
The effects discussed above indicate that after the birth or adoptionFootnote2 of the first child, mothers change the way they engage in the labor market in several dimensions. Therefore, gauging the impact of motherhood in one specific dimension of the labor market may underestimate the overall effect of maternity on the labor market. We use the job quality index developed by Sehnbruch and her team (Sehnbruch et al., Citation2020) to study how childbirth affects the overall quality of employment. These estimations would also allow us to create equivalencies between the multidimensional effects of quality of employment due to childbirth and a one dimensional change. These equivalencies help us to improve our understanding of the major changes in labor market engagement associated with maternity.
3. Data
Similarly than Berniell et al. (Citation2021), we use Chile’s Social Protection Survey, (“Encuesta de protección social”, EPS for its Spanish acronym) from the Undersecretary of Social Security, for both the matching process and to obtain the characteristics of labor engagement.Footnote3 The EPS is available for the years 2002, 2004, 2006, 2009 and 2015. The survey collects detailed information of the respondents’ current labor status, job characteristics, and other socioeconomic variables of all household members. The survey has a panel structure, which allows us to construct labor trajectories. The survey also contains individual labor information, socioeconomics characteristics, as well as variables related to children, such as year of birth and number of children. These variables allow us to construct a set of dependent variables to generate the match required for the estimation strategy.
Using the year of childbirth, we construct the database for the propensity score matching methodology. Our sample consists of 483,147 individuals between 18 and 65 years, of which 241,332 are men and 241,815 are women. From these women, 36.1% have children. Since we are studying a period of 14 years and we only have information on 6 of the 14 years, we restrict the sample of mothers and fathers to those that have at least one observation before and one observation after the birth of their first child. Additionally, to keep the set of information homogeneous across the sample, we restrict the information used to five years prior and five years after the child was born. For the control group we randomly assign a “year of birth” and keep the information to five years before and five years after that year, so that the gap between the births is as similar as possible to the ex-ante and ex post observations of the treated groups.
We construct several measures of job engagement that are widely used in the existing literature: hourly wage (in logs); full-time; contractual status; indefinite duration contract; whether the worker is self-employed or an employer; unionized job; public sector job; and categorical variables for size of the firm where the woman works: small (less than 10 employees), medium (between 10 and 199 employees), and large firms (200 or more employees). We also construct three measures of labor supply – participation, employment, and weekly hours worked – to compare our results to previous studies of the effects of children on labor outcomes. shows the summary statistics of the presence of children for women and men respectively, by existence of children.Footnote4As shown in , around 70% of women without children participated in the labor force during the period, while 62% were employed. Interestingly, for mothers the percentages are 76% and 66% respectively. This information initially suggests that women with children participate more in the labor force than women without children. However, we are not only interested on participation decision, but also we want to study other aspects of labor engagement. For instance, women with children have fewer permanent jobs and are more often self-employed or employers. For men, as shown in , participation in the labor force is greater for those with children, where 99% participate, compared with 91% participation of males without children. They also work more, 96% of men with children are employed compared to the 86% employment rate of men without children. In contrast to women, men with children have a higher proportion of permanent jobs with a contract than men without children, and less men with children are self-employed or employers in comparison with men without children.
Table 1. Summary statistics by presence of children (only women, regression sample).
Table 2. Summary statistics by presence of children (only men, regression sample).
4. Methodology
Our methodological approach has two steps. The first step is to provide evidence of the multidimensional effect of childbirth. We use a propensity score matching estimation to present evidence of the impact of childbirth on various dimensions of labor engagement. This step allow us to provide ample evidence of the multidimensional effect of childbirth. The second step of our methodology is to use the QoE index to encompass these multidimensional effects into one aggregated indicator. We present and discuss this two-step methodology in more detail in the following subsections.
4.1. Estimating the impact of maternity on various labor outcomes
Several challenges arise when analyzing the potential impact of maternity on labor outcomes. The first issue is how to obtain a “counterfactual” world in which we can compare the labor profile of the same female worker with and without children. Another issue is the timing of the comparison. Is motherhood only affecting labor engagement in the short run or in the long run? Finally, the data set required for a correct analysis must contain rich information about both the worker and the job in order to study the effect of maternity on various aspects of labor engagement.
We use a counterfactual comparison to study the impact of motherhood on different labor outcomes. Using a propensity score matching process, we compare the labor profile of similar individuals with the only difference being motherhood or fatherhood. Moreover, we choose different sets of characteristics for the matching process to improve the precision of the procedure. For instance, when studying the effect of motherhood on hours worked, we use labor force participation as one of the characteristics in the matching process, but when we study labor force participation as the outcome, we use a more reduced set of characteristics. In this way, we compare individuals who are as similar as possible to each other for each particular labor outcome. In the subsection of propensity score matching, we present all the matching characteristics and results of the average treatment effects on the treated estimation using the matching results.
Our methodology relies on the conditional independence assumption, which indicates that treatment assignment cannot be dependent on unobservable variables that might also determine potential outcome variables. Under this assumption, childbearing should not be determined by variables that might also determine labor outcomes for individuals. For this to be true we must assume that childbearing for each individual is independent of other labor market decisions. This assumption may be questionable since advancements in technology and medicine make it easier for family planning. To address this problem, we rely on Fröhlich’s (Citation2007) method that shows the versatility of the propensity score matching approach can be used to study gender gaps even in the absence of iid and the conditional independence assumption.
First, we estimate a propensity score. This propensity score P(X) estimates, using a probit regression, the probability of each individual to become a parent, given individual characteristics. The individual characteristics include measures that may affect the variable we are matching for, such as the decision to participate in the labor market as well as employment outcomes. Therefore, we choose a specific set of variables to generate the most precise matching for each labor outcome variable. We use the following individual characteristics: years of education, age, years of work experience, having a partner and employment outcomes. For employment outcomes, we include variables in the matching process that can affect the outcome but are not determinant of it. For instance, for participation we decided to exclude employment outcomes, because we are matching individuals in and out of the labor force. However, for type of contract, we include participation in the matching process. The variables used for the matching process of each outcome are presented in Appendix 1.
A general approach to propensity score matching for the average treatment effect on the treated is the form
This estimator is simply the mean difference in outcome between the parents (treatment group) and the non-parents (control group), weighted by the distribution of the likelihood of being a parent.
There are many different algorithms to weigh the control group observations, in order to obtain a distribution of its observables characteristics to be as similar as possible to the treatment group. One of the most common critiques of the propensity score matching methodology is that the use of different matching methodologies yields different results for the same sample and variables used. Consequently, we analyzed eight different matching methodologiesFootnote5 to examine if the results were sensitive to the specific matching processes. We also compared these results with a t-test and an OLS regression with the same variables as the ones used in the matching process plus a treatment binary variable. A summary of the main results comparing the matching methodologies can be found in appendices 2.1. and 2.2. In general, we found that the results are consistent across methodologies, and the only differences were the significance level for some variables, and small magnitude changes. Therefore, we would focus our discussion on the results obtained using the kernel biweight methodology, which shows better-adjusted distribution after matching. The graphs of the distribution adjusted after the matching process are in Appendix 3.
The estimation of the propensity score matching average treatment effect on the treated utilizing the kernel biweight approach is given by:
The average impact is calculated as the difference between the objective variable of an individual of the treatment group,, and the weighted average of the objective in all the control group
.
is the number of individuals in the treatment group and the weights of each observation are given by:
Where is the kernel biweight function
,
is the bandwidth and
is the number of individuals in the control group.
4.2. Estimating the impact of maternity on job quality
The propensity score matching estimation allow us to study how childbirth affects a dimension of labor engagement, but only one at a time. However, this approach is unable to indicate if these multidimensional effects occur simultaneously, or in general, different individuals are impacted differently, and they are only affected in one (or very few) dimension(s). This distinction is important, because if the effects are compounded, the overall impact of maternity is larger than the results indicated by one dimension in particular. Therefore, we turn our attention to methodologies capturing multidimensional effects on the labor market.
Historically, labor market analyses have focused on wages and participation, and the idea of job quality in the academic literature was dominated by the notion of earnings. However, during the last three decades, the concept of job quality has been broadened to include more labor outcomes. In particular, new definitions of job quality include dimensions that could affect a wider perspective of well-being (Brown et al., Citation2007). Despite the lack of consensus, the most common dimensions used to evaluate the quality of a job are wages, contractual status, tenure, work hours, and social protections (Piasna et al., Citation2019).
One of the main challenges for researchers and institutions that study job quality has been collecting a group of key indicators that could summarize this issue. Under the “Decent Work” agenda, the International Labor Organization (Citation2013) has defined 52 “Decent Work Indicators” that include job conditions and socioeconomics variables. On the other side, the European Union has built a synthetic index of job quality that includes dimensions such as wages, labor flexibility, work-life balance, working conditions and security, skills and career development and unionized work (Leschke & Watt, Citation2014). It is important to note that both approaches have been used in macro-national contexts.
In terms of how to measure the job quality of workers, the academic literature has explored multiple approaches, ranging from studies that only account for an intrinsically objective aspects of job quality to research exploring the workers’ subjective perceptions. Notable contributions along these lines are the proposal by Korner and Emese (Citation2009), Green et al. (Citation2013), and Sehnbruch et al. (Citation2020). We use the index proposed by Sehnbruch et al. because of its properties, which we discuss in the following section.
4.2.1. Quality of employment index
The Quality of Employment (QoE) index (Sehnbruch et al., Citation2020) is an adaptation of the Alkire and Foster (Citation2011) indicators. Following the Sen (Citation1992) principles, the dimensions considered for its construction are worker’s wage, job stability and employment conditions. The last two dimensions are divided into two sub-dimensions each: occupational status, tenure, social security affiliation, and excessive working hours. The QoE can take values between 0 and 1, where 0 indicates that the individual is not deprived in any dimension, and 1 indicates that the individual is deprived in all dimensions and sub-dimensions. Each dimension and sub-dimension have the same value, 1/3 and 1/6 respectively. The equation will be:
Therefore, the intensity of the deprivation will depend on the number of deprived dimensions, and the cut-off for considering one individual deprived of the quality of employment is 1/3. This means it is necessary to be deprived in at least one dimension and at least one additional sub-dimension to be considered deprived of quality employment. shows the different dimensions, sub-dimension weights and variables used in our case. One of the main advantages of the QoE index over other labor market indicators is that it is measured at individual level. At the same time, a concise indicator can be used for policy. An individual level indicator allows for comparisons across populations, and across space and time. At the same time allows to study if a particular dimension is inducing the change in the overall indicator.
Table 3. Dimensions, weights and variables used in the QoE Index calculation.
Table 4. Average treatment effects on the treated, Kernel Biweight.
Table 5. Average treatment effects on the treated for men and women by educational level, Kernel Biweight.
Table 6. Likelihood of increasing job quality deprivation, women’s sample – marginal effects.
Table 7. Likelihood of increasing job quality deprivation, men’s sample – marginal effects.
Table 8. Likelihood of increasing job quality deprivation, all sample – marginal effects.
There are numerous papers studying and providing evidence about job conditions for different groups, sectors or regions. However, the majority of these papers focus on pecuniary or non-pecuniary labor conditions, without proving a more comprehensive analysis of the differences. Fabry et al. (Citation2022) and Apablaza et al. (Citation2021) are part of a more recent literature using an index to study the multidimensional differences across jobs. Fabry et al. study how global food value chains have influenced heterogeneously the job conditions of groups of workers, such as female, young and immigrants. Apablaza et al. study how the overall quality of employment varies across the territory.
4.2.2. Labor force sample selection
One of the main challenges of studying the changes in job quality due to parenthood is that labor participation is one of the dimensions affected by the birth of a child. Therefore, in some cases we do not observe individuals participating in the labor market after childbirth. We can observe labor market participation for every individual, but changes in QoE are only observable for individuals that are working both before and after childbirth. We solve the bias of this non-random selection using a two-step Heckman estimation model.
We constructed an unbalanced panel of 2,020 women and 1,923 men that were surveyed in at least two of the years that the instrument was fielded. Thus, for each individual in the sample, we calculate the QoE in both periods, and we examine factors that may explain the change in the QoE. In particular, we examine if the birth of a child between the first and second period affects QoE.
The first step consists of estimating a selection model using a probit estimator, with participation in the labor market as the binary outcome variable. For this model, we utilize commonly used variables in the literature that determine labor market participation in our sample. In our case, these variables are having children at any given moment of time, having a partner, years of education and years of work experience. From the residuals of this estimation, we generate a vector of inverse Mills ratios, which are interpreted as the estimated expected errors.
In the second step, a binary variable that indicates the increase of the deprivation in the QoE index is regressed on a vector of explanatory variables and the inverse Mills ratio vector, by a maximum likelihood estimator. Hence, since this increase in QoE deprivation is only observed for individual participating in the labor market, the second stage regression will return the estimation including the expected error as another covariate, eliminating the part of the error term that is correlated with the dependent variable.
5. Results
shows the results of average treatment effect on the treated using the kernel biweight matching process for men and women. Results indicate that women with children are 11.9% less likely than women without children to participate in the labor force and 11.7% less likely to work, both with a 1% significance. Women with children are also 13.4% less likely to have a permanent job and 13.8% more likely to work in a firm with more than 200 employees. In contrast, for men, we observe no significant differences in any labor outcome, indicating that there are no differences between men with children and men without children. These results suggest that there are differences in participation and employment between women with and without children, and no difference between men. Blau and Kahn (Citation2017) show that human capital variables are an important determinant of both labor participation and employment and that educational attainment is the most important. Thus, we estimate the effect of parenthood for men and women using subsamples classified by educational level. An individual is classified as being more educated if they reported, on the EPS survey, having more than 12 years of education, while an individual with 12 years of education or less is classified as being less educated.
shows the results of more and less educated men and women. More educated mothers are 12.7% less likely to participate in the labor market than more educated childless women, but there are no significant differences between both groups in the likelihood of being employed. That is, conditional on participating, employment is not impacted by motherhood for more educated women. A slightly different effect is observed for less educated women. In this case, the difference on participation and employment between women with and without children are both statistically significant and indicate that mothers are 8.7% less likely to participate in the labor force and 9.4% less likely to work. Thus, motherhood also affects women engaged in the labor market. Moreover, less educated mothers are 14.4% less likely to have a permanent job than less educated childless women, but this effect is only 12.3% for more educated mothers.
Our results indicate that motherhood affects the type of firm that less educated women work for. For instance, less educated childless women are more likely to work in small firms (less than 9 employees) but less educated mothers are more likely to work in large firms (more than 200 employees). As expected, there are no differences between fathers and childless men for all educational levels. Therefore, the impact of parenthood is only observed in women and is relatively stronger for less educated mothers. Childbirth affects female labor participation, employment, hours worked, job permanency, and the type of firm that mothers work for.
In the next set of results, we use a job quality index to encompass all the dimensions affected by parenthood. Moreover, our previous results indicate that educational attainment may play an important role on how maternity affects the quality of employment. present the results of the estimation using the QoE index separately for more and less educated men and women. It is important to note that since we are estimating the change of a job quality deprivation index, a positive estimator indicates that job quality deprivation increased after childbirth, whereas a negative estimator indicates that job quality deprivation decreased after childbirth. At the same time, the index takes values between 0 and 1. The results show that job quality deprivation only increased for less educated women with children, whereas for more educated women with children and men there are no statistical significance changes in QoE. Moreover, in , the coefficient of 0.309 associated with childbirth for all women and 0.334 for less educated women indicates that having a child increases the QoE by 0.3, which is roughly 1/3. We will discuss the importance of this coefficient at the end of this section.
Estimating the model by gender may generate an additional bias due to the different distribution of QoE across genders. Thus, changes in QoE among men are not necessarily comparable to changes in QoE among women. Therefore, we estimate the Heckman model for the entire sample and for the educational attainment subsamples, but without splitting the sample by gender. We add an interaction terms to capture the overall effect of having a child and the specific effect of having a child for women. As expected, the results presented in indicate that having a child produces a drop in job quality, and this effect is stronger for women. Interestingly, these results are mainly driven by the sample of less educated women and are very consistent with the results presented in .
Using an index to describe the overall effect of maternity on the quality of employment allow us to illustrate the severity of the change in labor market engagement that childbirth produces. Not only the use of an index allow us to capture the multidimensional effect of maternity in labor engagement, but also allow us to create equivalencies. We transform the observed drop in QoE, which was approximately 1/3, into an equivalent drop in one specific dimension (or sub-dimension) to better understand the magnitude of the impact of maternity. In other words, after the estimation of the overall effect of maternity in several labor engagement dimensions, we calculate the change in one dimension that would produce an equivalent change in the QoE. In this way, we can illustrate that the compounding effect is significantly higher than the estimation of a single dimension using methodologies such as the propensity score matching. For instance, the observed drop in QoE for less educated mothers is equivalent to no longer being hired under contract (or to start working informally), and simultaneously ceasing to contribute to the individual pension account.Footnote6 For single mothers, the drop in QoE is equivalent to a reduction in wages that would put the family below the poverty line.Footnote7
6. Conclusions
One of the difficulties of measuring the impact of childbirth on the labor outcomes of mothers is its multidimensionality. The literature has documented changes in labor force participation, wages, hours worked, type of contract and type of firms where mothers work after the birth of their first child. In this paper, we use Chilean data to present evidence of these changes using a propensity score matching procedure to compare labor outcomes of individuals with and without children. The propensity score matching allows us to estimate the impacts of childbirth on various labor outcomes. We show that having a child changes labor force participation, employment, hours worked, wages, the type of labor relationship (hired under contract or self-employed) and firm size for women. We found that the effects are especially important for less educated mothers and implicate an overall change in labor engagement.
However, these methodologies focus on identifying the effect of childbirth on a specific dimension, but it can be the case that childbirth is affecting several dimensions simultaneously. We propose to use a Quality of Employment index to study the overall effect of childbirth on labor engagement. In this way, we measure how maternity may affect all the dimensions concurrently. We found a significant drop in quality, or as is defined by the index, a significant increase in quality of employment deprivation. The drop in quality of employment is more pronounced for less educated mothers, about a 33% of the index. To contextualize this drop, we present equivalent drops in the index caused by a one-dimensional change. Thus, our results indicate that, for women, having a child is equivalent to no longer being hired under contract (or start working informally), and simultaneously ceasing contributions to the individual pension account. For single mothers, the drop in QoE is equivalent to a reduction in wages that would put the family below the poverty line. These equivalencies highlight the importance of understand the child penalty as a multidimensional effect on labor engagement.
Disclosure statement
No potential conflict of interest was reported by the author(s).
Additional information
Funding
Notes on contributors
Juan Pedro Eberhard
Juan Pedro Eberhard holds a PhD degree in Economics from Yale University. Before joining Universidad Andres Bello, Juan was an assistant professor at Marshall School of Business at the University of Southern California, and an assistant professor at the Escuela de Negocios of Universidad Adolfo Ibañez. He was the Director of the Center of Economics and Regional Policy at Universidad Adolfo Ibañez. He is also a Research Fellow at IZA.
Javier Fernandez
Javier Fernandez holds a master’s degree in economics from Universidad Adolfo Ibañez. He is currently a lecturer at Universidad Diego Portales, and he was senior research assistant at the Center of Economics and Regional Policy at Universidad Adolfo Ibañez.
Catalina Lauer
Catalina Lauer holds a master´s degree in economics from Universidad Adolfo Ibañez, and is currently a student in the Graduate School of Economics, Finance and Management, Goethe University, Germany, where she plans to obtain a PhD. She was a senior research assistant at the Center of Economics and Regional Policy at Universidad Adolfo Ibañez.
Notes
1 Our estimations use the Heckman procedure to correct for potential selection bias.
2 Throughout the text, we use childbirth to express the concept of a child arriving to the household; therefore it encompasses both biological births and adopted children.
3 Technical reports by the agency that collected the data suggest that attrition was not systematically correlated to observable characteristics. We also compared characteristics of individuals that persist in the panel with those that dropout and find them similar.
4 We only present the average across waves, but the comparison by wave offers similar statistics.
5 The following matching processes were examined: one to one matching, nearest neighborhood matching, radius matching, normal kernel matching, biweight kernel matching, epan kernel matching, uniform kernel matching and tricube kernel matching.
6 In Chile, the pension system works through contributions to a personal pension account.
7 This interpretation is only valid for single mothers because the poverty line is defined as income per capita within a household. Therefore, for non-single mothers we must account for the partner’s income.
References
- Abramo, L., & Valenzuela, M. (2005). Women’s labor force participation rates in Latin America. International Labor Review, 144(4), 369–22. https://doi.org/10.1111/j.1564-913X.2005.tb00574.x
- Aguilar-Gomez, S., Arceo-Gomez, E., & De la Cruz Toledo, E. (2019). Inside the Black Box of Child Penalties. Available at SSRN https://ssrn.com/abstract=3497089.
- Alkire, S., & Foster, J. (2011). Understandings and misunderstandings of multidimensional poverty measurement. Journal of Economic Inequality, 9, 289–314.
- Andresen, M., & Nix, E. (2021). What causes the child penalty? evidence from adopting and same-sex couples. Forthcoming Journal of Labor Economics, 40(4), 971–1004. https://doi.org/10.1086/718565
- Angelov, N., Johansson, P., & Lindahl, E. (2016). Parenthood and the gender gap in Pay. Journal of Labor Economics, 34(3), 545–579. https://doi.org/10.1086/684851
- Apablaza, M., Sehnbruch, K., González, P., & Mendez, R. (2021) Regional inequality in multidimensional quality of employment (QoE): Insights from Chile, 1996-2017. International Inequalities Institute Working Papers (61). International Inequalities Institute, London School of Economics and Political Science,
- Berniell, I., Berniell, L., de la Mata, D., Edo, M., Fawaz, Y., Machado, M., & Marchionni, M. (2020). Motherhood and the allocation of talent: Harmonized evidence on 29 countries. CEDLAS Working Paper 270, Center for Distributive, Labor, and Social Studies
- Berniell, I., Berniell, L., Mata, D. D. L., Edo, M., & Marchionni, M. (2021). Gender gaps in labor informality: The motherhood effect. Journal of Development Economics, 150, 102599. https://doi.org/10.1016/j.jdeveco.2020.102599
- Berniell, I., Berniell, L., Mata, D. D. L., Edo, M., & Marchionni, M. (2023). Motherhood and flexible Jobs: Evidence from Latin American countries. World Development, 167, 106225. https://doi.org/10.1016/j.worlddev.2023.106225
- Blau, F. D., & Kahn, L. M. (2017). The gender wage gap: Extent, trends, and explanations. Journal of Economic Literature, 55(3), 789–865. https://doi.org/10.1257/jel.20160995
- Brown, A., Charlwood, A., Forde, C., & Spencer, D. (2007). Job quality and the economics of New Labour: a critical appraisal using subjective survey data. Cambridge journal of economics, 31(6), 941–971.
- Cohen, P. N., & Blanchi, S. M. (1999). Marriage, children, and women’s employment: What do we know. Monthly Lab Rev, 122(12), 22–31.
- Contreras, D., De Mello, L., & Puentes, E. (2011). The determinants of labor force participation and employment in Chile. Applied Economics, 43(21), 2765–2776. https://doi.org/10.1080/00036840903373303
- Contreras, D., & Plaza, G. (2010). Cultural factors in women’s labor force participation. Feminist Economics, 16(2), 27–46. https://doi.org/10.1080/13545701003731815
- de Quinto, A., Hospido, L., & Sanz, C. (2021). The child penalty: Evidence from Spain. SERIEs, 12(4), 585–606. https://doi.org/10.1007/s13209-021-00241-9
- Dollar, D., & Gatti, R. (1999). Gender inequality, income, and growth: Are good times good for women?. World Bank Development Research Group.
- Fabry, A., Van den Broeck, G., & Maertens, M. (2022). Decent work in global food value chains: Evidence from Senegal. World Development, 152, 105790. https://doi.org/10.1016/j.worlddev.2021.105790
- Farre, L., & Vella, F. (2013). The intergenerational transmission of gender role attitudes and its implications for female labor force participation. Economica, 80(318), 219–247. https://doi.org/10.1111/ecca.12008
- Fortin, N. (2005). Gender role attitudes and the labor market outcomes of women across OECD countries. Oxford Review of Economic Policy, 21(3), 416–438. https://doi.org/10.1093/oxrep/gri024
- Frölich, M. (2007). Nonparametric IV estimation of local average treatment effects with covariates. Journal of econometrics, 139(1), 35–75.
- Galarza, F., & Yamada, G. (2017). Triple penalty in employment access: The role of beauty, race, and sex. Journal of Applied Economics, 20(1), 29–47. https://doi.org/10.1016/S1514-03261730002-8
- Gasparini, L., & Marchionni, M. (2015). Bridging gender gaps?: The rise and deceleration of female labor force participation in Latin America. CEDLAS, Center for Distributive, Labor and Social Studies, Facultad de Ciencias Económicas, Universidad Nacional de La Plata.
- Gasparini, L., & Marchionni, M. (2017). Deceleration in female labor force participation in Latin America. Economía, 18(1), 197–224. https://doi.org/10.31389/eco.55
- Goldin, C. (2014). A grand gender convergence: Its last chapter. American Economic Review, 104(4), 1091–1119. https://doi.org/10.1257/aer.104.4.1091
- Green, F., Mostafa, T., Parent-Thirion, A., Vermeylen, G., Van Houten, G., Biletta, I., & Lyly-Yrjanainen, M. (2013). Is job quality becoming more unequal?. ILR Review, 66(4), 753–784.
- Kaul, T. (2018). Intra-household allocation of educational expenses: Gender discrimination and investing in the future. World Development, 104, 336–343. https://doi.org/10.1016/j.worlddev.2017.12.017
- Kleven, H., & Landais, C. (2017). Gender inequality and economic development: Fertility, education and norms. Economica, 84(334), 180–209. https://doi.org/10.1111/ecca.12230
- Kleven, H., Landais, C., Posch, J., Steinhauer, A., & Zweimüller, J. (2019). Child penalties across countries: Evidence and explanations. AEA Papers and Proceedings, 109, 122–126. https://doi.org/10.1257/pandp.20191078
- Kleven, H., Landais, C., Posch, J., Steinhauer, A., & Zweimüller, J. (2020). Do family policies reduce gender inequality? Evidences from 60 years of policy experimentation. NBER. Vol 28082.
- Kleven, H., Landais, C., & Søgaard, J. E. (2019). Children and gender inequality: Evidence from denmark. American Economic Journal Applied Economics, 11(4), 181–209. https://doi.org/10.1257/app.20180010
- Kleven, H., Landais, C., & Søgaard, J. E. (2021). Does biology drive child penalties? Evidence from biological and adoptive families. American Economic Review: Insights, 3(2), 183–198. https://doi.org/10.1257/aeri.20200260
- Korner, T., & Emese, S. (2009, June 12–13). Federal Statistical Office. Report on the indicators on quality of employment in the field of skill develop and life-long learning ( Working Paper No. 11). Task force meeting on the quality of employment, Paris.
- Kuépié, M. (2016). Determinants of labor market gender inequalities in Cameroon, Senegal and Mali: The role of human capital and fertility burden. Canadian Journal of Development Studies / Revue Canadienne D’études du Développement, 37(1), 66–82. https://doi.org/10.1080/02255189.2016.1122580
- Kuziemko, I., Pan, J., Shen, J., & Washington, E. (2018). The mommy effect: Do women anticipate the employment effects of motherhood? NBER, No. 24740
- Leschke, J., & Watt, A. (2014). Challenges in constructing a multi-dimensional European job quality index. Social indicators research, 118, 1–31.
- Lundborg, P., Plug, E., & Rasmussen, A. W. (2017). Can women have children and a career? IV evidence from IVF treatments. American Economic Review, 107(6), 1611–1637. https://doi.org/10.1257/aer.20141467
- Macpherson, D., & Hirsch, B. (1995). Wages and gender composition: Why do women´s jobs pay less? Journal of Labor Economics, 13(3), 426–471. https://doi.org/10.1086/298381
- Manning, A., & Petrongolo, B. (2008). The part-time pay penalty for women in Britain. Economic Journal, 118(526), 28–51. https://doi.org/10.1111/j.1468-0297.2007.02115.x
- Manual, I. L. O. (2013). Decent work indicators.
- Montenegro, C. (2001). Wage distribution in chile: Does gender matter? A quantile regression approach. World Bank Development Research Group, 20, 1–35.
- Ñopo, H., (2007). The gender wage gap in Chile 1992-2003 from a matching comparisons perspective, IZA discussion paper series (2698).
- Petrongolo, B., & Olivetti, C. (2008). Unequal pay or unequal employment? A cross-country analysis of gender gaps. Journal of Labor Economics, 26(4), 1–52. https://doi.org/10.1086/589458
- Petrongolo, B., & Ronchi, M. (2020). Gender gaps and the structure of local labor markets. Labor Economics, 64(April), 101819. https://doi.org/10.1016/j.labeco.2020.101819
- Piasna, A., Burchell, B., & Sehnbruch, K. (2019). Job quality in European employment policy: one step forward, two steps back?. Transfer: European Review of Labour & Research, 25(2), 165–180.
- Querejeta, M., & Bucheli, M. (2021). Motherhood penalties: The effect of childbirth on women’s employment dynamics in a developing country. Universidad de La República – Documentos de Trabajo 2021/1. https://hdl.handle.net/20.500.12008/26530
- Ruppanner, L., Lee, R., & Huffman, M. (2018). Do mothers benefit from flexible work? Cross-national evidence for work time. Job Quality, and Satisfaction, International Journal of Sociology, 48(2), 170–187. https://doi.org/10.1080/00207659.2018.1446119
- Sehnbruch, K., González, P., Apablaza, M., Méndez, R., & Arriagada, V. (2020). The Quality of Employment (QoE) in nine Latin American countries: A multidimensional perspective. World Development, 127, 104738. https://doi.org/10.1016/j.worlddev.2019.104738
- Sen, A. (1992). The political economy of targeting. World Bank.
Appendix 1
Table A1. Propensity Score Matching Variables.
Table A2. Average Treatment Effects on the Treated Results for Every Method of Propensity Score Matching (Only Women’s Sample).
Table A3. Average Treatment Effects on the Treated Results for Every Method of Propensity Score Matching (Only Men’s Sample).
Appendix 2