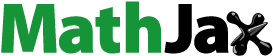
ABSTRACT
What drives income inequality in emerging market economies? To end it, we approach this topic utilizing Bayesian model averaging and 27 emerging market economies panel dataset from 1990 to 2019. First, differently, we find a U-shaped Kuznets curve in emerging market economies rather than the notable inverted U-shaped curve. Besides, we find four strictly robust determinants of income inequality in emerging market economies, including the population aging, the female labor force participation, the unemployment level and the share of labor compensation in output. These results remain stable through a series of robustness checks. In most cases, the government expenditure and real exchange rate could also robustly drive the dynamics of income inequality in emerging market economies. Further, a comparative analysis suggests that financial development, inflation, human capital condition, total factor productivity and urbanization are distinct determinants of income inequality in the market economies.
1. Introduction
To determine the law of income distribution is an essential issue in political economy. It has been widely recognized that the reduction in income inequality is crucial for economic and social development as unequal income distribution may lead to economic, political and social instabilities (Alesina & Rodrik, Citation1994; Inagaki, Citation2010). Even more, as suggested by Rajan (Citation2010) and Kumhof et al. (Citation2015), income inequality sows the seeds of the 2007–2008 global financial crisis and contributes to financial instability (Wang & Luo, Citation2023).
The classical Kuznets nexus (Kuznets, Citation1955) of income inequality and economic growth have been widely examined in the market economies (MEs) for the last decades. The regular-U structure (Piketty, Citation2014) vs an inverted-U structure, the positive relationship (List & Gallet, Citation1999) and even the S-shaped relationship (Costantini & Paradiso, Citation2018) are documented in the US and other MEs. In addition, financial development contributes to the income inequality in the US via financial firms’ rent-seeking channels (Stiglitz, Citation2013). Beck et al. (Citation2010) suggest that lower-income shares benefit from financial development. Furthermore, the MEs have employed the fiscal measures, e.g., progressive taxes (Piketty et al., Citation2014), transfer payment (OECD, Citation2012) and public expenditure on education (Agnello & Tavares, Citation2014; Hidalgo-Hidalgo & Iturbe-Ormaetxe, Citation2018; Roine et al., Citation2009), to tackle the inequality. Besides, physical (Baldi, Citation2013; Piketty, Citation2014) and human capital accumulation (Sequeira et al., Citation2017), price stability (Menna & Tirelli, Citation2017) are regarded as the determinants of income inequality in the MEs.
However, fewer studies have been conducted on the income inequality of the emerging market economies (EMEs) probably due to the data unavailability. As shown in , almost all EMEs witness acute income inequality for the last quarter-century with the Gini coefficients always being above 0.40-the “alerting line” as advised by the United Nations. In addition, the patterns of income inequality diverge significantly across countries. A bullish trend of income inequality is for China while a slight drop for Brazil and an inverted-U shape for Argentina, Bolivia, Russia and Ukraine. Given the stylized facts of income inequality, however, no enough efforts have been taken by researchers to investigate the causes of income inequality of the EMEs.
Figure 1. Dynamics of Gini coefficients in the EMEs.

Before revisiting the theories of income inequality of the EMEs in Section 2, we conclude methodologically that previous studies on the determinants of income inequality mainly employ the panel data models within the frequentist estimation framework, i.e., the static panel model estimated by the LSDV method and the dynamic panel model estimated by the system GMM. However, previous studies only focus on the specific variables with prior motivation.
This paper intends to fill these gaps by utilizing the Bayesian model averaging (BMA) approach to investigate the determinants of income inequality in 27 EMEs ranging from 1990 to 2019. By employing the BMA approach,Footnote1 we can include as many independent variables mentioned in the literature as possible without any prior distinctions. Within the traditional method, the arbitrary selection of control variables due to lack of uniform theoretical guidance may cause the fragility of regression analysis, namely model uncertainty (E. Leamer & Leonard, Citation1983). The BMA approach can overcome this problem and selects the robust determinants of income inequality.
We investigate the determinants of income inequality of the EMEs using the BMA strategy and conduct a series of robustness checks, including excluding the recent crisis period, alternating the model and coefficient priors, dropping the variable with many missing data, reducing the business cycle, using the extreme bound analysis (EBA) and the stochastic search variable selection (SSVS), adapting two machine learning strategies (Lasso and Random forest). First, differently, we find a U-shaped Kuznets curve in emerging market economies rather than the notable inverted U-shaped curve. We also find four strictly robust determinants of income inequality in the EMEs, including the population aging, the female labor force participation, the unemployment level and the share of labor compensation in output. These results remain stable through above robustness checks. In most cases, the government expenditure and real exchange rate could also robustly drive the dynamics of income inequality in the EMEs. Further, we build a comparison analysis on the drivers of income inequality between the EMEs and the MEs and find that financial development, inflation, human capital condition, total factor productivity and urbanization are distinct determinants of income inequality in the market economies.
The contributions of this paper are four-fold. First, to the best of our knowledge, this is the first paper to investigate the determinants of income inequality in the EMEs under an extensive uniform framework. Prior literature always investigates a specific variable while leaving others as the control variables or even neglecting them. Second, by reviewing the literature, we find that bulks of variables have been considered as the driven-factors of income inequality. Nondiscriminatory inclusion of factors in the regression specification may incur model uncertainty. This paper overcomes the model uncertainty by utilizing the BMA approach. Third, we find many interesting conclusions which may provide new research directions and reflections for the theorists and empiricists, such as the U-shaped Kuznets curve, the roles of population aging and female labor force participation, and why financial development is not a robust determinant of income inequality in the EMEs? Fourth, in the robustness checks, we employ two machine learning approaches, i.e., Lasso and Random forest, which helps broaden the toolkits of economists.
The remainder of the paper is organized as follows. Section 2 reviews the existing theories on the determination of income inequality in the EMEs. Section 3 develops the panel data model, the BMA strategy and describes the data. Section 4 presents the empirical results and a series of robustness checks. Section 5 conducts a comparative study based on the EMs sample. Section 6 discusses the conclusions and reflects the potential directions and revisions in this field. Section 7 concludes.
2. Theories of income inequality of EMEs revisited
2.1. Economic growth and income inequality
The first theory of income inequality is its relationship with economic growth. In seminal work of Kuznets (Citation1955), he argued that the effects of growth on income inequality is dependent on the labor movements from the agricultural sectors to industrial sectors. Before the nineteenth century (or before the first industrial revolution), the agricultural sectors dominated and the farmer, instead of the peasant, benefit from the expansion of agricultural production (i.e., economic growth), thus income inequality increased. After the nineteenth century, the industrial sectors began to inflate (i.e., economic growth), which attracted amounts of labors move from the agricultural sectors to industrial sectors and increased the per capita income, thus income inequality reduced.
However, many competing mechanisms may channel growth to inequality. First, the sociopolitical theories argue that income inequality may incentivize behaviors detrimental to economic growth, e.g., crime, political instability and revolution (Alesina & Rodrik, Citation1994). Besides, to avoid revolution or regime change, governments will intervene income redistribution as inequality increases, while these intervenes generate distortions (Barro, Citation2000) and cause the reductions in economic growth. Second, the saving rate theories presume that the marginal propensity to save increases as income increases, thus the low-income have a higher marginal propensity to save than the high-income. Therefore, this theory implies that as income inequality increases, the saving rate in the economy will increases, which will translate to more domestic investments and economic growth.
Besides, increasing literature starts to reconcile existing studies by modelling a nonlinear effect of income inequality on growth conditional on the economic development stages. Galor and Tsiddon (Citation1997), Galor (Citation2000) and Galor and Moav (Citation2004) argued that in the early physical capital driven stage of economic development, income inequality could stimulate economic growth by shifting resources from the high-income because of their higher marginal propensity to save than the low-income; when economic development enters the human capital driven stage, income inequality will be harmful for growth because of credit constraints on human capital accumulation. Bandyopadhyay and Basu (Citation2005) agreed that the inequality-growth nexus is conditional on the nature of technology and the extent of redistribution. If the knowledge could spill over with low barriers, the technology is highly skill-intensive and the degree of redistribution is high, the effect of income inequality on growth will be positive; otherwise, the effect will be negative. There will be a sign reversal if allowing the nature of technology and the extent of redistribution change. Empirically, Lin et al. (Citation2009) and Lin et al. (Citation2014) confirm the nonlinear nexus between income inequality and economic growth along the development process.
2.2. Globalization and income inequality
The distributive effects of globalization have been widely discussed but do not reach a consistent prediction about how globalization affects inequality (Dorn et al., Citation2018). Stolper and Samuelson (Citation1941) employed the classical Heckscher-Ohlin (HO) model to analyze the effect of globalization on inequality. According to HO model, countries should specialize in production in their relatively abundant factor and export these products. Trade will induce relative changes in product prices and thus increase returns to factors used intensively. The Stolper and Samuelson theorem predicts that trade will increase income inequality in advanced countries where capital and skilled labor are relatively abundant; however, trade could decrease income inequality in developing countries because unskilled labor is relatively abundant.
The HO model focuses on between-sector reallocation in production while neglecting within-sector reallocation and vertical specializations across countries. Therefore, the offshored and outsourced activities along the value chain may be relatively skill-intensive for the developing countries (Feenstra & Hanson, Citation1999). For example, FDI could increase the demand for the skilled labor and wage of the skilled labor, thus may widen the income gap in the developing countries (Feenstra & Hanson, Citation1997).
Cragg and Epelbaum (Citation1996) argued that because of the rising exposure to import competition, the traded sector in the developing countries will be more skill-intensive than before, thus the wage gap between skilled and unskilled labor broadens. Many other studies (Sampson, Citation2014; Yeaple, Citation2005) propose that the more productive firms are, the more probabilities of exporting firms have, thus exporting firms will pay higher wage to hire skilled labor.
2.3. Demographic variables and income inequality
Although different theoretical mechanisms have been proposed, it is widely agreed that population aging could increase income inequality. Two reasons contribute to the population aging, i.e., declining fertility rate and longer life expectancy. Santelli et al. (Citation2017) find that as the population is aging, the wealthier a family is, the lower fertility rate is. The fertility gap between the poor and the rich could increase the intergenerational income inequality (Dietzenbacher, Citation1989). Besides, population aging could affect income inequality via wealth inheriting. Miyazawa (Citation2006) modelled this mechanism with the assumption that income inequality in the same generation originates from the gap between the high-income and the low-income. Descendants of the high-income could inherit more wealth than those of the low-income, thus the former becomes richer, while the latter remains at the low-income level.
The effects of female labor force participation on income inequality are inconclusive (Sudo, Citation2017), the sign relies on which women work and which earn more. Female labor force participation could reduce income inequality when it improves the financial status of single-mother households. The disproportional increase in the labor supply of the poorly educated women would equalize income distribution (Esping-Andersen, Citation2007), this is the case when their partners become unemployed or reduce work hours for other reasons. Female labor force participation could also equalize income distribution when women live with a high-income man who withdrew partially or fully from the labor market (Cancian & Schoeni, Citation1998; Raaum et al., Citation2008). However, other channels may predict a positive nexus between female labor force participation and income inequality, for example, women living in a high-earned family or with assortative mating worked more (Esping-Andersen, Citation2007).
Human capital accumulation (e.g., educational expansion) has an ambiguous effect on income inequality (Knight & Sabot, Citation1983). Educational expansion affects income distribution by two offsetting effects, i.e., the composition effect and the wage compression effect. The initial educational expansion could increase the wage of more-educated workers, which rises wage inequality, namely “the composition effect”; as education expands, the supply of educated workers exceeds the demand, thus the wage premium of educated workers will diminish, and wage inequality will reduce.
2.4. Financial development and income inequality
Three major theoretical papers explain the nexus between financial development and income inequality, i.e., Banerjee and Newman (Citation1993), Galor and Zeira (Citation1993), Greenwood and Jovanovic (Citation1990). Banerjee and Newman (Citation1993) and Galor and Zeira (Citation1993) predict that financial development could reduce income inequality, the former argues households’ occupational choice dependent on credit availability, while the latter argues human capital investment dependent on credit availability. Differently, Greenwood and Jovanovic (Citation1990) predicted an inverted-U relation between financial development and income inequality. They highlighted that household could earn capital income by accessing the financial intermediaries, initially, the poor could not afford using banks for their savings, but the rich could, thus income inequality will exacerbate with financial development; as poor households become rich, who could access bank finance; thus, inequality will reduce with financial development.
2.5. Government intervene and income inequality
Government could redistribute resources via public expenditure to intervene income distribution (Doerrenberg & Peichl, Citation2014). For example, according to the human capital investment theory (Gruber, Citation2013) which argues education could increase a person’s skill and productivity, government could provide subsidies to the low-income households for early-education investments to improve their education attainments, which will decrease inequality and increase intergenerational mobility (Yang & Qiu, Citation2016).
Monetary policy could affect income inequality in an ambiguous way (Coibion et al., Citation2017). First, expansionary monetary policy could increase inequality via asset prices and inflation. The rising of asset prices benefits the capital returns of the high-income, and the inflation will depreciate the income of the low-income because of their possessions of liquid assets. Second, expansionary monetary policy could reduce inequality. An unexpected expansion of monetary policy could benefit the borrowers, always the low-income (Doepke & Schneider, Citation2006). Labor earnings of the low-income are most affected by the economic activity (Heathcote et al., Citation2010), the expansionary monetary policy could inflate the economy, thus could reduce income inequality. We will use inflation (Monnin, Citation2014; Székely, Citation2003) as a proxy of monetary policy because the direct measure of exogenous monetary policy is absent.
2.6. Other theories
Besides the above widely discussed theories, exchange rate (Jeanneney & Hua, Citation2001; Min et al., Citation2015), physical investment (Thorbecke & Charumilind, Citation2002), total factor productivity (Espoir & Ngepah, Citation2021), the share of labor compensation in output (Hémous & Olsen, Citation2022), unemployment level (Acemoglu, Citation1999; Dabla-Norris et al., Citation2015), urbanization (Oyvat, Citation2016) are often covered in the previous literature, we will also introduce them into our analysis.
Exchange rate could affect the relative price of tradable products and untradeable products, by which affect the income gap between skilled and unskilled workers. Skilled worker could benefit from the technology change, thus technology change increases income inequality by rising the wage premium of skilled workers (Dabla-Norris et al., Citation2015). Unemployment could increase inequality via directly affecting the share of labor income (Furceri & Ostry, Citation2019).
3. Methodology and data
3.1. Fixed effect model
We utilize the following two-way fixed effect panel data model to examine the effect of various factors on the income inequality. In practice, we conduct the Hausman Test (see ) and accept the specifications of the fixed effect (FE) model:
Table 1. Determinants of income inequality in the EMEs: FE results.
where and
are the country and time fixed effect, respectively,
denotes a country,
denotes time.
is used to control those time-invariant variables but changing with countries. For example, the law system may affect the income distribution, but the law system doesn’t change in a short time.
is used to control those country-invariant variables but changing with time. For example, the financial crisis shock may affect the income inequality of all countries but only change with time.
is the disturbance term that is unobservable and uncorrelated with the independent variables and distributes identically and independently. We collect 16 potential determinants of income inequality by reviewing the existing literature extensively.
, the logarithm of real output per capita, and its quadratic term,
, are used to exploit the potential inverted U axis between economic development and income inequality. We expect that
and
. Two price variables,
and
, capture the effects of the real exchange rate and inflation on the income inequality, respectively, but the sign of the coefficients,
and
, are ambiguous.
is the government expenditure scaled by GDP and is expected to be a negative sign.
is the domestic investment scaled by GDP and represents the physical capital formation and is expected to be a negative sign.
, equaling the export scaled by GDP, is incorporated in the analysis on trade and inequality based on the literature and is expected to be a negative sign. We incorporate the growth rate of inward foreign direct investment,
, into the analysis and expect that it has an ambiguous sign.
, the bank credit scaled by GDP, is a proxy of financial development in the EMEs and is expected to be a negative sign.
is the human capital based on years of schooling and returns to education and is expected to be a negative sign.
is the total factor productivity and mirrors the technological development and is expected to be an positive sign.
is the share of labor compensation in output and embodies the structure of factor income and is expected to be a negative sign.
is the unemployment rate and is expected a positive sign. Finally, three population structure variables are included,
, population over 65 years old scaled by the total population (with an expected positive sign),
, urban population scaled by the total population (with an expected negative sign),
and the female workforce scaled by the total workforce (with an expected negative sign).
Due to the lack of the uniform theoretical framework, we cannot convincingly tell the determinants of income inequality in the EMEs. As the extant empirical literature often considers as many as possible variables in control variables, an econometric issue may arise, the model uncertainty which incurs the fragility of regressors (E. Leamer & Leonard, Citation1983). This paper intends to overcome this problem by employing the BMA strategy.
3.2. BMA approach
The basic idea of model averaging is to consider and estimate all candidate models and then report a weighted average as the estimate of the effect of interest variables (Moral-Benito, Citation2015). We select
independent variables through reviewing the literature. Thus, the number of candidate models is
.
where is the coefficient vector estimated by model averaging,
is the coefficient vector of the
model and
is the posterior model probability related to the
model.
Each model will be estimated in a Bayesian way, and the coefficients’ posterior distribution of the model is as follows:
where is the dependent variable,
is the likelihood function,
is the coefficients’ prior distribution of the
model.
Bayesian inference demonstrates that the posterior model probability can be regarded as the weight of model averaging. Given that the prior probability is P
, the posterior probability can be calculated by the Bayesian approach as follows:
The implementation of BMA entails two different types of prior probability: one is the prior probability of coefficient space, and another is the prior probability of model space. To calculate EquationEquation (4)(4)
(4) ,
, which is the marginal likelihood function, should be calculated in advance. By combing EquationEquation (3)
(3)
(3) and
, we can derive.
According to E. Leamer and Leonard (Citation1983), the posterior coefficient probability is
Point estimation can be obtained by the expectation and variance of the coefficient distribution, namely the posterior mean (P.M) and the posterior standard deviation (P.SD), respectively:
Besides, the BMA provides the posterior inclusion probability (P.IP) to convey the probability statement regarding the importance of a regressor. P.IP is the sum of the model posterior probability of the specific variable, P.IP=,
denotes the coefficient of the
regressor. Implementation of BMA procedures presents three challenges. First, we need to choose the prior model probability and the prior for coefficients. In the baseline study, following the specifications of Ley and Steel (Citation2012), we use the random model prior probability, which means that the likelihood of the
model containing
variables is
with
subjective to Beta distribution. Thus, the prior model size is
. Following Leòn-Gonzàlez and Montolio (Citation2015), the prior for the coefficients is specified as
type and the prior coefficients probability of the
model is
, where
. In the section of robustness check, we will alternate the prior probabilities. Second, the marginal likelihood (
) depends on an integral that cannot be solved analytically but can be calculated through a computationally intensive numerical approach. Finally, as the model space in our empirical application contains 65,536 models, a full estimation will be implemented.
3.3. Advantages of BMA
As mentioned before, BMA could be used to solve the model uncertainty issue. In this study, we regress the income inequality on as many as possible independent variables to identify the robust determinants of income inequality in the EMEs. Many competing theories of the determination of income inequality in the EMEs have been proposed as stated in Section 2. A natural question is which theory is robust? In traditional regression analysis, we often cover many potential independent variables in one model. However, it is problematic once we add the irrelevant factors to the model and omit the relevant factors, which is the model uncertainty issue (E. Leamer & Leonard, Citation1983).
The BMA strategy does not rely on any priors on the theories of income inequality, but robustly relies on two priors on the model space and the coefficient space under the Bayesian framework. In practice, the number of candidate models depends on the number of independent variables, any variable could be included or not, thus models exist (in practice, it is often unnecessary to consider all models). For each model, we set a prior model probability distribution, and we could obtain the posterior distribution after the Bayesian sampling. For each variable, we set a prior coefficient probability distribution and get the posterior distribution through the Bayesian procedure. Finally, we could estimate the coefficients by averaging the estimates of the different models under consideration, each weighted by its model probability. Searching the literature, we find Furceri and Ostry (Citation2019) is a similar paper. They also use the model averaging technique, specifically WALS (weighted-average least squares). However, their application is under the frequentist framework, ours is under the Bayesian framework.
EBA was ever a popular approach to investigate the determinants of variables of interest. However, EBA has been criticized for its lack of statistical foundations, and its implementation also restricted to a limited number of models (Chakrabarti, Citation2001). Berger and Sellke (Citation1987) have suggested that conventional sensitivity analyses (including EBA) overstate significance and confidence intervals in the absence of a full account of model uncertainty.
3.4. Data
The original data of the independent variables employed are mainly collected from two data libraries, Penn World Table 10.0 (PWT 10.0) and World Bank database (WB). The dependent variable is income inequality. Although there are diverse databases of income inequality, these databases have weaknesses of less comparability and limited coverage. The Standardized World Income Inequality Database (SWIID) provides a set of comparable income inequality data with the widest possible coverage across countries and over time (Solt, Citation2015). Many researchers have conducted studies using this database. For example, Acemoglu et al. (Citation2015) use SWIID to examine the impacts of democracy on the inequality. provides detailed information for each variable: definition, source and calculation. Given the availability of data, the sample covers a period from 1990 to 2019 with annual frequency. Although SWIID starts from 1960, at least two reasons constraint our sample from 1990. First, SWIID is severely data missing from 1960 to 1990. From 1960 to 2019, the number of missing of the SLOT database is 904, while the number of missing is 768 from 1960 to 1990. Second, the BMA approach we used require no more than three continuous missing observations. The sample contains 27 EMEs which constitute the J.P. Morgan Emerging Markets Bond Index Global (EMBI) or Morgan Stanley Capital International Emerging Market Index (MSCI-EMI) except for Israel. The list of EMEs is shown in . To avoid the influence of serial correlation, we adapt the interactive fixed effect (Bai, Citation2009) to deal with the original data.
reports the basic statistics of the variables involved. Gini_net and Gini_market are the two alternative dependent variables. The former is measured by net income while the latter is measured by market income. We find that Gini_market (mean value 46.4691) is often higher than Gini_net (mean value 41.84346), but the standard error reverses in overall and between groups. This fact implies that personal income taxation flattens income inequality to certain degree (Agnello & Tavares, Citation2014). The volatility of real exchange rate, inflation, bank credit and urbanization are greater than the others, implying that the disparities of these variables across country and time are significant. Thus, the inclusion of country and time fixed effects is reasonable.
To favor the empirical analysis in Section 4, we present a correlation matrix in as the preliminarily descriptive evidence. What we are interested in is the first column, which offers the correlation coefficients between income inequality and each independent variable. As we can see, real output per capita and its quadratic term, bank credits, female workforce ratio, government expenditure, human capital condition, the share of labor compensation in output, unemployment, urbanization and population aging are negatively correlated with the income inequality at least 5% significance level. Intuitively, the Kuznets curve may disappear because the coefficients of real output per capita and its quadratic term are both negative, implying the axis of symmetry locates the negative space. However, we need more cautious inference rather than a simple statistical analysis.
4. Empirical results and analysis
In this section, we present the empirical results. In addition to the baseline results, we consider the potential effects of the recent crisis period on the findings by excluding the sample during the crises period. We also conduct a series of robustness checks.
4.1. Baseline results
Without considering model uncertainty, presents the traditional panel estimation results under FE specification (1) and Hausman test statistics.Footnote2 First, the Hausman test rejects the null hypothesis with χ2 statistics of 25.96, implying that the FE model is preferred. Thus, we hereafter only report the FE results. Second, finds the coefficients of real output per capita and its quadratic term are significant negative and positive, respectively, which implies that the nexus between real output per capita and income inequality is positive once the real output per capita is larger than 11.6849 (=-(−10.6800)/(2 × 0.4570)). Therefore, supports the U-shaped instead of the inverted-U shaped Kuznets curve that exists in the EMEs, which is consistent with Bandyopadhyay and Basu (Citation2005), but different from Tsount and Osueke (Citation2014). Third, we find that only few factors in the left 14 potential drivers are statistically significant in shaping the inequality in the EMEs, i.e., the population aging, the government expenditure, the share of labor compensation in output, the real exchange rate and trade openness are significant at 1% and 5% levels, respectively. However, the traditional panel model provides limited information on the determination of inequality in the EMEs as some drivers advocated by the previous studies are not robust at least in the specification (1) due to model uncertainty.
reports the baseline results using the BMA approach with both Gini_net and Gini_market as the dependent variables, respectively. Following Raftery (Citation1995), evidence for a regressor with a P.IP of 0.50 to 0.75 is considered weak, of 0.75 to 0.95 positive, of 0.95 to 0.99 strong and above 0.99 very strong. Therefore, we only care about the independent variables with P.IP of 0.50 or above.
Table 2. Determinants of income inequality in the EMEs: baseline results.
The BMA strategy identifies nine determinants of income inequality in the EMEs when the dependent variable is Gini_net. We observe that the results support the U-shaped Kuznets curve in the EMEs as . The coefficients of GDP and GDP2 are −11.6982 and 0.4764, respectively. Then, we can deduce that the optimal point of logarithmic output per capita is 12.2777(=-(−11.6982)/[2× (0.4764)]). Combined with the statistics of GDP reported in , we speculate that the half of EMEs have crossed the threshold and positioned the right side of the U-shaped Kuznets curve, which means that income distribution will deteriorate as these economies perform better. Interestingly, if we select seven representative countries (i.e., Argentina, Bolivia, Chile, China, Colombia, Korea and Peru) as the sample, the results suggest that these countries have located the right side of the U-shaped Kuznets curve.
Combined with the results using Gini_market as the dependent variable, we find additional seven variables are robust determinants of income inequality in the EMEs, i.e., FDI, the government expenditure, population aging, the share of labor compensation in output, the unemployment, the real exchange rate and the female workforce ratio.
FDI is detrimental to income distribution in the EMEs, which is consistent with existing literature (Bogliaccini & Egan, Citation2017). Different from the previous studies (Cevik & Correacaro, Citation2015), we find that government expenditure (G) is a robust factor that could equalize the income distribution. Population aging (OLD) is negatively correlated with the income inequality robustly in the EMEs. This result is opposite to the existing literature, such as Dong et al. (Citation2018). The increase of the share of labor compensation in output (LAB) reflects the reduction of functional income inequality. In line with Dabla-Norris et al. (Citation2015), unemployment ratio (UNE) polarizes the income distribution in the EMEs because the low-income labours rather than the high-income labours become un-employees in the EMEs. Decrease in exchange rate (XR) increases the income inequality by increasing the cost of exported firms and decreases the wage of unskilled labors in the EMEs. A robust but often neglected variable is the female labor force participation (FEM), which could reduce the income inequality (Değirmenci, Citation2018).
Interestingly, we find that financial development (CRE) is not a robust determinant of income inequality in the EMEs. The idea that financial development reduces the income inequality by easing financial market constraints remains being suspected in the EMEs. Lack of diverse financial systems may pose an obstacle to financial access to the poor (Claessens & Perotti, Citation2007). We also find that trade (OPNESS) is not a key factor of income inequality in the EMEs, but FDI is.
4.2. Excluding the sample during the crisis period
The recent global financial crisis has substantially redistributive consequences (Goda et al., Citation2017), which may challenge the results above. To check the effect of financial crisis, we exclude the sample during the crisis (from 2005 to 2009) from the original sample. The results are reported in .
Table 3. Determinants of income inequality in the EMEs using the BMA: subtracting the crises.
The results suggest that the crises do affect the determination of income inequality in the EMEs to some extent. When the dependent variable is Gini_net, the BMA procedure selects 11 regressors as the driven-factors of income inequality in the EMEs. Different from the results above, bank credit (CRE) is a driver of income inequality with a posterior inclusion probability of 0.6603 in the EMEs. The Kuznets curve fails to be a robust component of income inequality, and exchange rate (XR) also play no roles in shaping the inequality. However, the human capital (HC), the trade openness (OPNESS) and urbanization (URB) become the determinants of income inequality in the EMEs. FDI, the female labor force participation (FEM), the population aging (OLD), the labor share (LAB) and the government expenditure (G) remain as the robust determinants of income inequality in the EMEs as . When the dependent variable is Gini_market, government expenditure (G), urbanization (URB) and physical capital investment (I) lose their explainable power as presented in .
Overall, no matter we subtract the crises period or not, we always find that foreign direct investment (FDI), female labor force ratio (FEM), the population aging (OLD), the unemployment level (UNE) are still the robust inequality drivers in the EMEs.
4.3. Robustness checks
We conduct a series of robustness checks, including alternating the model priors and the coefficient priors, excluding the variables with many missing values, using 5-year average sample to reduce business cycle, adapting the EBA approach, overcoming the multicollinearity issue via SSVS, and two machine learning techniques (i.e., Lasso and Random forest).
4.3.1. Alternate the model priors
In the baseline analysis, we employ the random model prior. Here, we alternate the model priors with the fixed model prior, uniform model prior and Posterior Inclusion Prior (PIP) model prior, which are the popular specifications in the BMA literature. Fixed model prior means that we set prior model size as 8 in mean, which implies that , thus
. Uniform model prior assigns the equal probability to each candidate model, P
. As to PIP prior, we assign the prior probability of the robust factors in the benchmark model with a low value and the remaining variables with a high value.Footnote3 Explicitly, we assign FDI, G, OLD, LAB, GDP, GDP2, UNE, XR, FEM with 0.01 of prior probability and others with 0.5 of prior probability.
The results are presented in . Compared to , we find that the baseline results are robust. Population aging (OLD), female workforce scaled by total workforce (FEM), unemployment (UNE) and labor income share (LAB) are identified as the most robust determinants under the three alternative specifications. Also, trade openness (OPNESS), human capital condition (HC) and urbanization (URB) are considered to be robust in models with different model priors.
Table 4. Determinants of income inequality in the EMEs using the BMA: alternative model priors.
Furthermore, we can check the robustness by examining the distribution of model size. As evidenced in , the significant disparity in prior model size induced by different specifications of prior probability in model space converges to the similar posterior model size. The average model size is 8.10, 8.11, 8.26, 7.23, respectively, meaning that the model with 7 to 8 robust determinants can sufficiently explain the dynamics of income inequality in the EMEs, which is significantly different from the traditional frequentist estimation results as presented in .
4.3.2. Alternate the parameter priors
Similarly, we alternate the type parameter priors in baseline specifications with three popular priors, the fixed-parameter prior, the empirical Bayesian local (EBL) parameter prior and Hyper-g parameter priors. The fixed parameter prior sets
with
, which is different from the baseline specification with
. EBL parameter prior sets the distinctive
for each model,
, and
is the standard OLS statistics for the
model (Hansen & Yu, Citation2001). The Hyper-g parameter prior set a shrinkage factor as
, where
(Liang et al., Citation2008).
By alternating the specifications of prior probability in coefficient space, shows the consistency of empirical results with the benchmark specifications. The robust determinants of income inequality in the EMEs are the female labor force ratio (FEM), the population aging (OLD), the unemployment levels (UNE), the labor income share (LAB) and the government expenditure (G) and other regressors as reported in .
Table 5. Determinants of income inequality in the EMEs using the BMA: alternative parameter priors.
As shown above, we investigate the model size distributions with three different parameter priors. Although the distributions of parameter priors diverge significantly, the posterior distributions are similar. The average model sizes are 11.69, 9.20, 9.12, respectively, which means that the model with 9 to 11 robust determinants can sufficiently explain the dynamics of income inequality in the EMEs. These results are consistent with .
4.3.3. Excluding the variables with many missing values
We find the variable, physical capital investment (I), suffers from many missing data, which leads to large amount of data loss because of the requirement of balanced panel. The data loss may make the baseline results biased; thus, we drop this variable and reconduct the analysis in this section.
The results of excluding I are reported in . We find that, both when Gini_net and Gini_market as the dependent variable, FDI, the government expenditure (G), the population aging (OLD), the labor share (LAB), the unemployment levels (UNE), the real exchange rate (XR) and the female labor force participation (FEM) are robust determinants of income inequality in the EMEs, which is consistent with the baseline results in . Our results remain stable after dropping the variables with many missing observations.
Table 6. Determinants of income inequality in the EMEs using the BMA: excluding I.
4.3.4. Reducing the business cycle
Our baseline results may suffer from the impacts of the business cycle. Following the strategy of Moral-Benito (Citation2012), to keep the data balance and avoid the serial correlation in the transitory component of the disturbance term, we split the sample into five-year periods. Besides, the stock variables are measured in the first year of each five years and the flow variables are measured as five-year averages. The results are reported in .
Table 7. Determinants of income inequality in the EMEs using the BMA: 5-year averages.
Compared with the baseline results, the BMA strategy reduces the number of common variables selected under different dependent variables to four, suggesting that the use of 5-year averages may indeed reduce some of the information contained in the original data. The coefficients of the population aging (OLD) variable are opposite to the baseline results, suggesting that increasing population aging increases income inequality. The impacts of unemployment (UNE) and real exchange rate (XR) on income inequality are consistent with the benchmark results. Trade openness (OPNESS) reduces the income inequality on average, which is consistent with the Stolper-Samuelson theorem and Jaumotte et al. (Citation2013). The low-skilled labors in the developing countries benefit from the trade liberalization, while competition leads to a reduction in the compensation of the high-skilled workers.
Overall, the population aging (OLD), the real exchange rate (XR), the unemployment level (UNE) and the government expenditure (G) remain as the robust determinant of income inequality in the EMEs as the baseline results of .
4.3.5. Eba
EBA was a popular procedure used to probe the determinants of the variable of interest. In this section, we employ the EBA strategy to check the baseline results although the EBA strategy has been criticized for many reasons as mentioned in Section 3.3. In practice, there are two representative methods of EBA, i.e., E. E. Leamer (Citation1985) and Sala-I-Martin (Citation1997). E. E. Leamer (Citation1985) considered a variable to be robust only if the upper and lower bounds of its coefficients both lie on the same side of zero. However, this standard is stringent and generally lead to results where all variables that we concern are not robust. In response to the shortcomings of E. E. Leamer (Citation1985), Sala-I-Martin (Citation1997) proposes an alternative approach that focuses on the entire distribution of estimates and assigns a certain level of confidence to the robustness of each variable. Specifically, a variable is robust if the major parts of its coefficient distribution lie simultaneously to the left or right of zero. Following Sala-I-Martin (Citation1997), the cut-off point is a CDF(0) value of 90 or higher. The EBA results are shown in .
Table 8. Determinants of income inequality in the EMEs using EBA.
The robust variables selected by the EBA procedure are fairly identical to the baseline results of . Besides, the EBA additionally reveals the significant effects of human capital condition (HC) and the trade openness (OPNESS) on income inequality in the EMEs.
4.3.6. Ssvs
indicates that the correlations between competing predictors are quite high in some cases. Stochastic Search Variable Selection (SSVS, George & McCulloch, Citation1993, Citation1997) is suggested to be less sensitive to multicollinearity issues than competing Bayesian alternatives based on Bayes factors. Therefore, we use SSVS as a robustness check to the baseline results. SSVS is another commonly used method for Bayesian variable selection. It identifies the set of models that may have better predictive performance by estimating the level of model uncertainty among all possible candidate models. Jetter et al. (Citation2022) summarize that SSVS is a methodological improvement over EBA in terms of dealing with multicollinearity, relative fit of different models and the uncertainty in the extreme-bound estimation (Brock & Durlauf, Citation2001; McAleer & Veall, Citation1989).
Like the BMA, SSVS uses marginal inclusion probabilities (MIP) to select variables, and we usually only care about the independent variables with MIP of 0.50 or above. SSVS results in shows that almost all the variables selected in BMA, including the unemployment level (UNE), the population aging (OLD), the female workforce as a ratio of total workforce (FEM), are proved to be important in the SSVS approach. In addition, like the results in , SSVS approach reveals that the trade openness (OPNESS) has a significant effect on income inequality.
4.3.7. Lasso: evidence from machine learning
Machine learning approaches are often used to select a significant predictor variable. It has been proven that machine learning performs significantly better than traditional econometric models in many aspects, especially in prediction (Varian, Citation2014). In this case, we additionally choose Least Absolute Shrinkage and Selection Operator models (Lasso, Tibshirani, Citation2011), which is often used as a comparison to SSVS and BMA in the previous literature (Bainter et al., Citation2020), as one of the robustness tests.
The Lasso model mainly focuses on the which minimizes the following objective function.
In EquationEquation (9)(9)
(9) , the left part is the RSS (sum of squared residuals) and the right part is the penalty term that is adjusted by the hyperparameter λ. The hyperparameter is exogenously generated by the researcher via a cross-validation process. When a new variable is introduced into the Lasso but has a negligible contribution to the reduction of RSS, the effect of the shrinkage penalty grows, which means that the Lasso considers the coefficient of this variable to be zero.
As shown in , the optimal parameter after 10-fold cross-validation is 0.2625, and the corresponding model size is 9. In this case, the variables chosen by the Lasso are FDI, the female labor force participation (FEM), the physical capital investment (I), the population aging (OLD), the human capital (HC), the total factor productivity (TFP), the labor share (LAB), the trade openness (OPNESS) and the real output per capita (GDP), which is basically identical to the baseline results of . Consistent with the results of other robustness tests, Lasso also places additional emphasis on three variables, i.e., the physical capital investment (I), the human capital condition (HC) and the trade openness (OPNESS). In addition, Lasso also chooses the total factor productivity (TFP) in the optimal model, but this finding does not receive any stronger evidence in the baseline results and other robustness tests.
Figure 5. Determinants of income inequality in the EMEs using the Lasso.

4.3.8. Random forest: evidence from machine learning
Random forest (Breiman, Citation2001) is another popular machine learning strategy used to select the predictor, which is a supervised learning and ensemble algorithm and constructed based on multiple decision trees. Random forest has attracted many attention in economics due to its versatility and superior ability in reducing overfitting and multicollinearity issues (Lindner et al., Citation2022).
In terms of variable selection, random forests can filter predictor variables in two aspects. First, MSE (mean square error) of model. In a random forest, each predictor is randomly assigned a value, and if the predictor is more important, the error in the model prediction increases when its value is randomly replaced. “%IncMSE”, as shown in , denotes the increase in MSE if one variable is randomly replaced. Thus, a larger “%IncMSE” indicates a higher importance of the variable. Second, the node purity (Gini index), which represents the heterogeneity effect of each variable on the observations at the nodes (Genuer et al., Citation2010). The higher the node purity (i.e., the smaller the Gini index), the more important the variable. The results of both indicators above are reported in .
Figure 6. Determinants of income inequality in the EMEs using the random forest.

After 10-fold cross-validations, the model with 10 or 12 variables, whose error is 2.0013 and 1.8307, respectively, was preferable. The unemployment level (UNE), the human capital (HC), the real exchange rate (XR), the population aging (OLD) and other variables remain robust. However, the predictive capacity of the trade openness (OPNESS) for income inequality, which performs favorably in other robustness tests, is questioned in the random forest model, suggesting that the effect of the trade openness on income inequality is still subject to model uncertainty, and the mechanism of the effect of the trade openness on EMEs remains to be further discussed and tested.
5. A comparative study with the MEs
We extend the BMA strategy to exploit the determinants of income inequality by employing the data from 27 widely accepted MEs, which is collected similarly to Section 2.3. A list of the MEs is presented in .
reports the results based on the MEs sample. It is evident that the determinants of income inequality in the MEs significantly differentiate from those in the EMEs but share some variables. In the full sample, the BMA strategy selects 13 robust determinants of income inequality in the MEs. In the sample subtracted crises period, 12 variables are selected as the robust factors of income inequality. Compared with , we find the government expenditure (G), the population aging (OLD), the labor share (LAB), the real exchange rate (XR) and the unemployment level (UNE) are also robust determinants of inequality in the MEs.
Table 9. Determinants of income inequality in the MEs using the BMA.
The results based on the full sample indicate that share of labor compensation, human capital condition (Sequeira et al., Citation2017), physical capital and inflation are the determinants of income inequality in the MEs. Share of labor compensation in GDP could remit the income inequality on average, implying that the MEs can reduce the income inequality by improving the factor structure. Similarly, human capital development is beneficial to the reduction of income inequality in the MEs. Accessing to education and professional training is easier than ever (Baldi, Citation2013) in the MEs and thus reduces the income inequality. Physical capital alleviates the income inequality on average in the MEs. Higher inflation is associated with the worsening income distribution (Walsh & Yu, Citation2012). Government expenditure and population aging are the robust determinants of income inequality in the MEs even when we exclude the data during the crises period. Government expenditure can reduce the income inequality on average, which is consistent with Agnello and Tavares (Citation2014) and Roine et al. (Citation2009). Population aging has the impact on the income inequality in the MEs.
Overall, different with the results of the EMEs in , the bank credit (CRE), the inflation (INF), the human capital (HC), the total factor productivity (TFP) and the urbanization (URB) are important factors affecting inequality in the MEs. Female labor force participation is no longer a robust determinant of income inequality in the MEs.
6. Discussions
Using the BMA procedure, we get many interesting results on the determination of income inequality in the EMEs and a comparative analysis with the MEs. These results may provide some new research directions and may push the theorists to revise the existing theories.
First, we find that in the EMEs, the notable inverted-U shaped Kuznets curve doesn’t exist, alternatively we find the evidence that support the U-shaped curve. This result indicates that many EMEs have surmounted the threshold value of economic development, the further development will worsen the income distribution. The classical Kuznets theory said that after the threshold, as the country performs better in economy and the governance ability improves, the country could flatten the income inequality in many delicate ways. Why the opposite nexus is found in the EMEs? Although the prior studies have found the similar results, no theoretical explanation have been suggested.
Second, we find the population aging is a strictly robust negative determinant of income inequality in both the EMEs and the MEs. Prior literature has probed the effects of population aging on income inequality mainly using empirical methods in the EMEs, such as Zhong (Citation2011), Dong et al. (Citation2018). Intuitively, the increase of population aging indicates the productivity heterogeneity between economic agents decreases, which means that the wage disparity will decrease and income distribution tends to be equalized. However, except the productivity effect, population aging many produce many economic effects. How about these channels affect the income inequality? We need more delicate empirical works and build theoretical model to assist the analysis.
Third, we find the female labor force participation is a robust determinant of income inequality in the EMEs. This is an interesting finding, although prior studies have investigated the nexus between female labor force participation and income inequality (Değirmenci, Citation2018). As female labor force participation increases, the gender wage gap decreases, suggesting that income tends to be equal. Given the importance of female labor force participation on income inequality, we need more delicate studies, such as checking the nexus using the firm-level data.
Fourth, we find financial development is not a robust determinant of income inequality in the EMEs, but a key determinant in the MEs. The idea that financial development reduces the income inequality by easing financial market constraints remains being suspected in the EMEs. Lack of diverse financial systems may pose an obstacle to financial access to the poor (Claessens & Perotti, Citation2007). Thus, we need a detailed comparative analysis about the different roles of financial development in income inequality between the EMEs and the MEs. In practice, what kind of financial system could improve income distribution?
7. Conclusion
We use the BMA strategy to overcome the model uncertainty and identify the robust determinants of income inequality in the EMEs by employing the panel data over a period from 1990 to 2019. First, differently, we find a U-shaped Kuznets curve in emerging market economies rather than the widely accepted inverted U-shaped curve. Besides, we find four strictly robust determinants of income inequality in emerging market economies, including the population aging, the female labor force participation, the unemployment level and the share of labor compensation in output. These results remain stable through a series of robustness checks, including excluding the recent crisis period, alternating the model and coefficient priors, dropping the variable with many missing data, reducing the business cycle, using the extreme bound analysis and the Stochastic Search Variable Selection, adapting two machine learning strategies (Lasso and Random forest). In most cases, the government expenditure and real exchange rate could also robustly drive the dynamics of income inequality in emerging market economies.
Further, we build a comparison analysis on the drivers of income inequality between the EMEs and the MEs and find that financial development, inflation, human capital condition, total factor productivity and urbanization are distinct determinants of income inequality in the market economies.
Finally, we reflect our main findings and argue that both in theory and in empirics, we need more delicate studies. For example, the Kuznets effect of economic growth on income inequality, the roles of population aging and female labor force participation and a design of financial development beneficial to the income equality.
Acknowledgments
This work is supported by the Natural Science Foundation of China (72003205), the Humanities and Social Sciences Foundation of Chinese Ministry of Education (20YJC790142) and the General Project of Social Science Planning in Guangdong Province (GD22CYJ12).
Disclosure statement
No potential conflict of interest was reported by the author(s).
Additional information
Funding
Notes on contributors
Shengquan Wang
Shengquan Wang is a distinguished research associate fellow at the Institute of Advanced Studies in Humanities and Social Sciences, Beijing Normal University. His research interests are income inequality and macro-finance.
Yuan Gao
Yuan Gao is an undergraduate at Bay Area International Business School, Beijing Normal University. His research interests are income inequality and marketing.
Mingjin Luo
Mingjin Luo is an assistant research fellow at the International Development Cooperation Academy, Shanghai University of International Business and Economics. Her research interests are corporate finance and international finance.
Notes
1 See Steel (Citation2020) on the application of model averaging in economics.
2 Random effect estimation results are presented in .
3 This practice is intuitive and counterfactual. If the results still hold when using a low prior probability for the selected robust variables in the baseline and a high prior probability for others, it implies that the results are robust.
References
- Acemoglu, D. (1999). Changes in unemployment and wage inequality: An alternative theory and some evidence. American Economic Review, 89(5), 1259–34. https://doi.org/10.1257/aer.89.5.1259
- Acemoglu, D., Naidu, S., Restrepo, P., & Robinson, J. A. (2015). Democracy, redistribution and inequality. In Handbook of income distribution (pp. 1885–1966). Elsevier. https://doi.org/10.1016/B978-0-444-59429-7.00022-4
- Agnello, L., & Tavares, R. M. S. (2014). How does fiscal consolidation impact on income inequality? Review of Income and Wealth, 60(4), 702–726.
- Alesina, A., & Rodrik, D. (1994). Distributive politics and economic growth. The Quarterly Journal of Economics, 109(2), 465–490. https://doi.org/10.2307/2118470
- Bai, J. (2009). Panel data models with interactive fixed effects. Econometrica, 77(4), 1229–1279.
- Bainter, S. A., McCauley, T. G., & Wager, T. (2020). Improving practices for selecting a subset of important predictors in psychology: An application to predicting pain. Advances in Methods and Practices in Psychological Science, 3(1), 66–80. https://doi.org/10.1177/2515245919885617
- Baldi, G. (2013). Physical and human capital accumulation and the evolution of income and inequality. Journal of Economic Development, 38(3), 57–83. https://doi.org/10.35866/caujed.2013.38.3.003
- Bandyopadhyay, D., & Basu, P. (2005). What drives the cross-country growth and inequality correlation? Canadian Journal of Economics, 38(4), 1272–1297. https://doi.org/10.1111/j.0008-4085.2005.00325.x
- Banerjee, A. V., & Newman, A. F. (1993). Occupational choice and the process of development. Journal of political Economy, 101, 274–298. https://doi.org/10.1086/261876
- Barro, R. J. (2000). Inequality and growth in a panel of countries. Journal of Economic Growth, 5(1), 5–32. https://doi.org/10.1023/A:1009850119329
- Beck, T., Levine, R., & Levkov, A. (2010). Big bad banks? The winners and losers from bank deregulation in the United States. The Journal of Finance, 65(5), 1637–1667. https://doi.org/10.1111/j.1540-6261.2010.01589.x
- Berger, J. O., & Sellke, T. (1987). Testing a point null hypothesis: The irreconcilability of p values and evidence. Journal of the American Statistical Association, 82(397), 112–122. https://doi.org/10.1080/01621459.1987.10478397
- Bogliaccini, J. A., & Egan, P. J. W. (2017). Foreign direct investment and inequality in developing countries: Does sector matter? Economics & Politics, 29(3), 209–236. https://doi.org/10.1111/ecpo.12098
- Breiman, L. (2001). Random forests. Machine Learning, 45(1), 5–32. https://doi.org/10.1023/A:1010933404324
- Brock, W. A., & Durlauf, S. N. (2001). What have we learned from a decade of empirical research on growth? Growth empirics and reality. World Bank Economic Review, 15(2), 229–272. https://doi.org/10.1093/wber/15.2.229
- Cancian, M., & Schoeni, R. F. (1998). Wives’ earnings and the level and distribution of married couples’ earnings in developed countries. Journal of Income Distribution, 8(1), 45–61. https://doi.org/10.1016/S0926-6437(99)80003-1
- Cevik, S., & Correacaro, C. (2015). Growing (un) equal: Fiscal policy and income inequality in China and BRIC. IMF Working Paper.
- Chakrabarti, A. (2001). The determinants of foreign direct investments: Sensitivity analyses of cross-country regressions. Kyklos, 54(1), 89–114. https://doi.org/10.1111/1467-6435.00142
- Claessens, S., & Perotti, E. (2007). Finance and inequality: Channels and evidence. Journal of Comparative Economics, 35(4), 748–777. https://doi.org/10.1016/j.jce.2007.07.002
- Coibion, O., Gorodnichenko, Y., Kueng, L., & Silvia, J. (2017). Innocent Bystanders? Monetary policy and inequality. Journal of Monetary Economics, 88, 70–89. https://doi.org/10.1016/j.jmoneco.2017.05.005
- Costantini, M., & Paradiso, A. (2018). What do panel data say on inequality and GDP? New evidence at US state-level. Economics Letters, 168, 115–117. https://doi.org/10.1016/j.econlet.2018.04.019
- Cragg, M. I., & Epelbaum, M. (1996). Why has wage dispersion grown in Mexico? Is it the incidence of reforms or the growing demand for skills? Journal of Development Economics, 51(1), 99–116. https://doi.org/10.1016/S0304-3878(96)00427-0
- Dabla-Norris, M. E., Kochhar, M. K., Suphaphiphat, M. N., Ricka, M. F., & Tsounta, E. (2015). Causes and consequences of income inequality: A global perspective. IMF Working Papers.
- Değirmenci, S. (2018). Female labor force participation and income inequality: The case of Turkey. Institutions, National Identity, Power, and Governance in the 21st Century, 2017, June 28–30, Belgrade, Serbia.
- Dietzenbacher, E. (1989). The dynamics of population growth, differential fertility, and inequality: Comment. American Economic Review, 79(3), 584–587.
- Doepke, M., & Schneider, M. (2006). Inflation and the redistribution of nominal wealth. Journal of Political Economy, 114(6), 1069–1097. https://doi.org/10.1086/508379
- Doerrenberg, P., & Peichl, A. (2014). The impact of redistributive policies on inequality in OECD countries. Applied Economics, 46(17), 2066–2086. https://doi.org/10.1080/00036846.2014.892202
- Dong, Z., Tang, C., & Wei, X. (2018). Does population aging intensify income inequality? Evidence from China. Journal of the Asia Pacific Economy, 23(1), 1–12. https://doi.org/10.1080/13547860.2017.1354270
- Dorn, F., Fuest, C., & Potrafke, N. (2018). Globalization and income inequality revisited. SSRN Electronic Journal. https://doi.org/10.2139/ssrn.3143398
- Esping-Andersen, G. (2007). Sociological explanations of changing income distributions. American Behavioral Scientist, 50(5), 639–658. https://doi.org/10.1177/0002764206295011
- Espoir, D. K., & Ngepah, N. (2021). Income distribution and total factor productivity: A cross-country panel cointegration analysis. International Economics & Economic Policy, 18(4), 661–698. https://doi.org/10.1007/s10368-021-00494-6
- Feenstra, R. C., & Hanson, G. H. (1997). Foreign direct investment and relative wages: Evidence from Mexico’s maquiladoras. Journal of International Economics, 42(3–4), 371–393. https://doi.org/10.1016/S0022-1996(96)01475-4
- Feenstra, R. C., & Hanson, G. H. (1999). The impact of outsourcing and high-technology capital on wages: Estimates for the United States, 1979–1990. Quarterly Journal of Economics, 114(3), 907–940. https://doi.org/10.1162/003355399556179
- Furceri, D., & Ostry, J. D. (2019). Robust determinants of income inequality. Oxford Review of Economic Policy, 35(3), 490–517.
- Galor, O. (2000). Income distribution and the process of development. European Economic Review, 44(4–6), 706–712. https://doi.org/10.1016/S0014-2921(99)00039-2
- Galor, O., & Moav, O. (2004). From physical to human capital accumulation: Inequality and the process of development. Review of Economic Studies, 71(4), 1001–1026. https://doi.org/10.1111/0034-6527.00312
- Galor, O., & Tsiddon, D. (1997). Technical progress, mobility, and economic growth. American Economic Review, 87(3), 363–382.
- Galor, O., & Zeira, J. (1993). Income distribution and macroeconomics. The Review of economic studies, 60, 35–52. https://doi.org/10.2307/2297811
- Genuer, R., Poggi, J. M., & Tuleau-Malot, C. (2010). Variable selection using random forests. Pattern Recognition Letters, 31(14), 2225–2236. https://doi.org/10.1016/j.patrec.2010.03.014
- George, E. I., & McCulloch, R. E. (1993). Variable selection via Gibbs sampling. Journal of the American Statistical Association, 88(423), 881–889. https://doi.org/10.1080/01621459.1993.10476353
- George, E. I., & McCulloch, R. E. (1997). Approaches for Bayesian variable selection. Statistica Sinica, 7, 339–373. https://doi.org/10.1007/s004400050098
- Goda, T., Onaran, Ö., & Stockhammer, E. (2017). Income inequality and wealth concentration in the recent crisis. Development and Change, 48(1), 3–27. https://doi.org/10.1111/dech.12280
- Greenwood, J., & Jovanovic, B. (1990). Financial Development, Growth, and the Distribution of Income. Journal of political Economy, 98(5), 1076–1107. https://doi.org/10.1086/261720
- Gruber, J. (2013). Public finance and public policy (4th ed.). Worth Publishers.
- Hansen, M. H., & Yu, B. (2001). Model selection and the principle of minimum description length. Journal of the American Statistical Association, 96(454), 746–774. https://doi.org/10.1198/016214501753168398
- Heathcote, J., Perri, F., & Violante, G. L. (2010). Unequal we stand: An empirical analysis of economic inequality in the United States, 1967–2006. Review of Economic Dynamics, 13(1), 15–51. https://doi.org/10.1016/j.red.2009.10.010
- Hémous, D., & Olsen, M. (2022). The rise of the machines: Automation, horizontal innovation, and income inequality. American Economic Journal: Macroeconomics, 14(1), 179–223. https://doi.org/10.1257/mac.20160164
- Hidalgo-Hidalgo, M., & Iturbe-Ormaetxe, I. (2018). Long-run effects of public expenditure on poverty. Journal of Economic Inequality, 16(1), 1–22. https://doi.org/10.1007/s10888-017-9360-z
- Inagaki, K. (2010). Income inequality and the suicide rate in Japan: Evidence from cointegration and LA-VAR. Journal of Applied Economics, 13(1), 113–133. https://doi.org/10.1016/S1514-0326(10)60006-2
- Jaumotte, F., Lall, S., & Papageorgiou, C. (2013). Rising income inequality: Technology, or trade and financial globalization? IMF Economic Review, 61(2), 271–309. https://doi.org/10.1057/imfer.2013.7
- Jeanneney, S. G., & Hua, P. (2001). How does real exchange rate influence income inequality between urban and rural areas in China? Journal of Development Economics, 64(2), 529–545. https://doi.org/10.1016/S0304-3878(00)00149-8
- Jetter, M., Mahmood, R., Parmeter, C. F., & Ramírez-Hassan, A. (2022). Post-Cold War civil conflict and the role of history and religion: A stochastic search variable selection approach. Economic Modelling, 114, 105907. https://doi.org/10.1016/j.econmod.2022.105907
- Knight, J. B., & Sabot, R. H. (1983). Educational expansion and the Kuznets effect. American Economic Review, 73(5), 1132–1136.
- Kumhof, M., Rancière, R., & Winant, P. (2015). Inequality, leverage, and crises. American Economic Review, 105(3), 1217–1245. https://doi.org/10.1257/aer.20110683
- Kuznets, S. (1955). Economic growth and income inequality. American Economic Review, 45(1), 1–28.
- Leamer, E. E. (1985). Sensitivity analyses would help. American Economic Review, 75(3), 308–313.
- Leamer, E., & Leonard, H. (1983). Reporting the fragility of regression estimates. Review of Economics & Statistics, 65(2), 306–317. https://doi.org/10.2307/1924497
- Leòn-Gonzàlez, R., & Montolio, D. (2015). Endogeneity and panel data in growth regression: A Bayesian model averaging approach. Journal of Macroeconomics, 46, 23–39. https://doi.org/10.1016/j.jmacro.2015.07.003
- Ley, E., & Steel, M. F. (2012). Mixtures of g-priors for Bayesian model averaging with economic applications. Journal of Econometrics, 171(2), 251–266. https://doi.org/10.1016/j.jeconom.2012.06.009
- Liang, F., Paulo, R., Molina, G., Clyde, M. A., & Berger, J. O. (2008). Mixtures of g priors for Bayesian variable selection. Journal of the American Statistical Association, 103(481), 410–423. https://doi.org/10.1198/016214507000001337
- Lindner, T., Puck, J., & Verbeke, A. (2022). Beyond addressing multicollinearity: Robust quantitative analysis and machine learning in international business research. Journal of International Business Studies, 53(7), 1307–1314. https://doi.org/10.1057/s41267-022-00549-z
- Lin, S. C., Huang, H. C., Kim, D. H., & Yeh, C. C. (2009). Nonlinearity between inequality and growth. Studies in Nonlinear Dynamics & Econometrics, 13(2). https://doi.org/10.2202/1558-3708.1635
- Lin, Y. C., Huang, H. C., & Yeh, C. C. (2014). Inequality-growth nexus along the development process. Studies in Nonlinear Dynamics & Econometrics, 18(3), 237–252. https://doi.org/10.1515/snde-2012-0037
- List, J. A., & Gallet, C. A. (1999). The Kuznets curve: What happens after the inverted-U? Review of Development Economics, 3(2), 200–206. https://doi.org/10.1111/1467-9361.00061
- McAleer, M., & Veall, M. R. (1989). How fragile are fragile inferences? A re-evaluation of the deterrent effect of capital punishment. The Review of Economics and Statistics, 71(1), 99–106. https://doi.org/10.2307/1928056
- Menna, L., & Tirelli, P. (2017). Optimal inflation to reduce inequality. Review of Economic Dynamics, 24, 79–94. https://doi.org/10.1016/j.red.2017.01.004
- Min, H. G., Shin, S. O., & Mcdonald, J. A. (2015). Income inequality and the real exchange rate: Linkages and evidence. Annals of Economics and Finance, 16(1), 115–141.
- Miyazawa, K. (2006). Growth and inequality: A demographic explanation. Journal of Population Economics, 19(3), 559–578. https://doi.org/10.1007/s00148-005-0047-6
- Monnin, P. (2014). Inflation and income inequality in developed economies. CEP Working Paper Series.
- Moral-Benito, E. (2012). Determinants of economic growth: A Bayesian panel data approach. Review of Economics and Statistics, 94(2), 566–579. https://doi.org/10.1162/REST_a_00154
- Moral-Benito, E. (2015). Model averaging in economics: An overview. Journal of Economic Surveys, 29(1), 46–75. https://doi.org/10.1111/joes.12044
- OECD. (2012). Economic policy reforms 2012 going for growth (pp. 181–202). OECD Publishing. https://doi.org/10.1787/growth-2012-en
- Oyvat, C. (2016). Agrarian structures, urbanization, and inequality. World Development, 83, 207–230. https://doi.org/10.1016/j.worlddev.2016.01.019
- Piketty, T. (2014). Capital in the twenty-first century. Harvard University Press.
- Piketty, T., Saez, E., & Stantcheva, S. (2014). Optimal taxation of top labor incomes: A tale of three elasticities. American Economic Journal: Economic Policy, 6(1), 230–271. https://doi.org/10.1257/pol.6.1.230
- Raaum, O., Bratsberg, B., Røed, K., Österbacka, E., Eriksson, T., Jäntti, M., & Naylor, R. A. (2008). Marital sorting, household labor supply, and intergenerational earnings mobility across countries. The BE Journal of Economic Analysis & Policy, 7(2). https://doi.org/10.2202/1935-1682.1767
- Raftery, A. E. (1995). Bayesian model selection in social research. Sociological Methodology, 25, 111–163. https://doi.org/10.2307/271063
- Rajan, R. (2010). Fault lines: How hidden fractures still threaten the world economy. Princeton University Press. https://doi.org/10.1515/9781400839803
- Roine, J., Vlachos, J., & Daniel, W. (2009). The long-run determinants of inequality: What can we learn from top income data? Journal of Public Economics, 93(7–8), 974–988. https://doi.org/10.1016/j.jpubeco.2009.04.003
- Sala-I-Martin, X. (1997). I just ran four million regressions. NBER working paper.
- Sampson, T. (2014). Selection into trade and wage inequality. American Economic Journal: Microeconomics, 6(3), 157–202. https://doi.org/10.1257/mic.6.3.157
- Santelli, J. S., Song, X., Garbers, S., Sharma, V., & Viner, R. M. (2017). Global trends in adolescent fertility, 1990–2012, in relation to national wealth, income inequalities, and educational expenditures. Journal of Adolescent Health, 60(2), 161–168. https://doi.org/10.1016/j.jadohealth.2016.08.026
- Sequeira, T. N., Santos, M., & Ferreira-Lopes, A. (2017). Income inequality, TFP, and human capital. Economic Record. Economic Record, 93(300), 89–111. https://doi.org/10.1111/1475-4932.12316
- Solt, F. (2015). On the assessment and use of cross-national income inequality datasets. Journal of Economic Inequality, 13(4), 683–691. https://doi.org/10.1007/s10888-015-9308-0
- Steel, M. F. J. (2020). Model averaging and its use in economics. Journal of Economic Literature, 58(3), 644–719. https://doi.org/10.1257/jel.20191385
- Stiglitz, J. (2013). The price of inequality. W. W. Norton & Company.
- Stolper, W. F., & Samuelson, P. A. (1941). Protection and real wages. The Review of Economic Studies, 9(1), 58–73. https://doi.org/10.2307/2967638
- Sudo, N. (2017). The effects of women’s labor force participation: An explanation of changes in household income inequality. Social Forces, 95(4), 1427–1450. https://doi.org/10.1093/sf/sox011
- Székely, M. (2003). The 1990s in Latin America: Another decade of persistent inequality, but with somewhat lower poverty. Journal of Applied Economics, 6(2), 317–339. https://doi.org/10.1080/15140326.2003.12040597
- Thorbecke, E., & Charumilind, C. (2002). Economic inequality and its socioeconomic impact. World Development, 30(9), 1477–1495. https://doi.org/10.1016/S0305-750X(02)00052-9
- Tibshirani, R. (2011). Regression shrinkage and selection via the lasso: A retrospective. Journal of the Royal Statistical Society: Series B (Statistical Methodology), 73(3), 273–282. https://doi.org/10.1111/j.1467-9868.2011.00771.x
- Tsount, E., & Osueke, A. (2014). What is behind Latin America’s declining income inequality? IMF Working Paper.
- Varian, H. R. (2014). Big data New tricks for econometrics. Journal of Economic Perspectives, 28(2), 3–28. https://doi.org/10.1257/jep.28.2.3
- Walsh, J. P., & Yu, J. (2012). Inflation and income inequality: Is food inflation different? IMF Working Paper.
- Wang, S., & Luo, R. (2023). Income distribution, financial liberalisations and banking stability: Theory and international evidence. International Journal of Finance & Economics. https://doi.org/10.1002/ijfe.2809
- Yang, J., & Qiu, M. (2016). The impact of education on income inequality and intergenerational mobility. China Economic Review, 37, 110–125. https://doi.org/10.1016/j.chieco.2015.12.009
- Yeaple, S. R. (2005). A simple model of firm heterogeneity, international trade, and wages. Journal of International Economics, 65(1), 1–20. https://doi.org/10.1016/j.jinteco.2004.01.001
- Zhong, H. (2011). The impact of population aging on income inequality in developing countries: Evidence from rural China. China Economic Review, 22(1), 98–107. https://doi.org/10.1016/j.chieco.2010.09.003
Appendix 1
List of variables, countries, descriptive statistics
Table A1.1 List of variables.
Table A1.2 List of EMEs.
Table A1.3 List of MEs.
Table A1.4 Descriptive statistics of variables.
Table A1.5 Pearson’s correlation matrix.
Appendix 2
Random effects results
Table A2.1 Determinants of income inequality in the EMEs: RE results.
Appendix 3
Dynamics of Gini coefficients in the EMEs