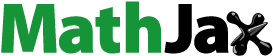
ABSTRACT
This study investigates spatial autocorrelation and the formation of spatial export clusters of Brazilian mesoregions based on technological intensity. It also analyzes how regional knowledge and agglomeration economies explain regional exports, using spatial econometric techniques. Brazilian exports exhibit a stronger spatial autocorrelation. Non-manufacturing sectors, which are less technology-intensive, exhibit lower spatial dependence than manufacturing sectors. The results also show an autocorrelation between exports and R&D expenditure, identifying the formation of clusters in the southeastern and southern regions. R&D expenditure fosters the growth of regional exports and the spatial lag effects of exports and R&D expenditure are significant in the high technology-intensive manufacturing sector.
1. Introduction
Agglomeration economies and knowledge spillovers have been used as a theoretical framework to explain the process of economic growth based on their impact on firm productivity (Audretsch & Feldman, Citation2004; Döring & Schnellenbach, Citation2006). They are based on the geographical proximity of economic activities and on the possibility of interaction and knowledge transfer among agents, as well as on other locational advantages called externalities (Fugita et al., Citation2002; Krugman, Citation1991; Marshall, Citation1961). Moreover, knowledge exchange between workers and firms, especially through interactive learning, enables technological innovation (Lundvall, Citation1992).
Thus, one of the determinants of technological innovation is proximity. Firms which are closer to sources of knowledge are able to innovate faster than firms that are further away as they can absorb the knowledge that is shared through the informal exchange of ideas between economic agents (Lambooy, Citation2010; Storper & Venables, Citation2004). Externalities that promote the adoption of new technologies are also more important at the regional level than at the national level and depend positively on the proximity of firms (Baptista, Citation2000). Moreover, social and relational proximity are important channels of knowledge dissemination and generate synergies that drive regional growth (Basile et al., Citation2012). A distinctive feature of relationships between economic agents is that they are not limited by regional administrative boundaries. As for the agglomeration economies resulting from the link between R&D and production, including exports, they are also likely to cross these borders (Coe & Helpman, Citation1995; Guastella & van Oort, Citation2015).
By exploring the spatial dimension of exports and R&D, this paper first examines spatial autocorrelation and formation of spatial export clusters of Brazilian mesoregions based on technological intensity. It also examines how regional knowledge and agglomeration economies explain regional exports, using spatial econometric techniques. Regions with higher export concentration are expected to have a greater capacity to form spatial clusters, which may vary depending on the technological intensity of the sectors. Furthermore, a positive spatial autocorrelation between exports and R&D expenditure is expected, with knowledge spillovers crossing administrative boundaries and spreading to neighboring regions, leading to different dynamics between regions.
While many studies have tried to establish a link between innovation and exports at the country level, little is known about this relationship (1) across location of exports within a country (i.e., at regional level), (2) by technological intensity of the export structure, (3) especially in developing countries. By analyzing the location of exports and R&D expenditure by technological intensity sector to characterize the pattern of regional concentration and whether there is a relationship between this pattern and R&D expenditure in 137 Brazilian mesoregions, this study contributes to expanding the range of empirical regional studies and international trade in this issue. In recent years, the structure of Brazilian exports has changed requiring new studies on the determinants of these exports with updated data, since the advantages acquired by firms/regions in international trade are always temporary in terms of technological issues.
Using data on 137 Brazilian mesoregions between 2008 and 2021 and the recent OECD taxonomy of economic activities based on R&D intensity (Galindo-Rueda & Verger, Citation2016), which includes not only manufacturing but also services, we found that Brazilian exports exhibit spatial autocorrelation. Non-manufacturing sectors (less technologically intensive) exhibit lower spatial dependence than manufacturing. Mesoregions with higher exports of high-manufacturing goods are surrounded by neighboring mesoregions with high R&D expenditure, hinting at the existence of a cluster in the southeastern and southern regions. Finally, using spatial econometric techniques, we found spatial spillover effects of exports (i.e., knowledge diffusion about foreign markets) and R&D expenditure between neighboring regions in the high manufacturing sector. Naturally, these results must be interpreted with caution due to limitations of the study, as there are few variables at the mesoregion level that allow for the best proxy of stock of knowledge and the choice of spatial weighting matrix.
The remainder of the paper is organized as follows. Section 2 provides a brief discussion on agglomeration economies, highlighting the externalities that may arise and the potential knowledge spillover and innovation. Section 3 describes the data source and empirical methodology employed, namely exploratory spatial data analysis and spatial panel data econometric techniques. Section 4 analyzes and discusses the results. The patterns of spatial distribution and clustering of Brazilian exports by technological intensity as well as their spatial relationship with R&D expenditure are highlighted. Finally, Section 5 underscores the main conclusions and possible policy implications.
2. Agglomeration economies, regional knowledge and exports
The literature on agglomeration economies generally highlights the advantages of closely located firms that lead to external economies, in particular productivity growth at the firm level, production growth at the sectoral and regional level, which ultimately foster regional economic growth (van Oort et al., Citation2012). The benefits can result, first, from internal economies of scale at the firm level, and second, from the scale of geographical concentration of industries, which not only favors internal economies of firms, but also enables external economies (Fugita et al., Citation2002; Galinari & Lemos, Citation2007; Hoover, Citation1937; Jones & Jordan, Citation2019). As a result, agglomeration economies have become an important element in studies on the location of economic activity (Krugman, Citation1991). Moreover, the space (within and between geographic areas) seems to determine the performance of firms/regions (Duschl et al., Citation2015; Tsvetkova et al., Citation2020).
Agglomeration economies may have origin in the spatial concentration of firms belonging to the same industry, specialization or localization economies, or from the variety and diversity of local economic activities, urbanization or diversity economies. Formal or informal contacts between firms and their workers belonging to the same industry foster productivity gains that are likely to be higher in regions where the industry is more concentrated, the so-called specialization economies (Hoover, Citation1937). At the local level, supplier-user link occurs due to the specialization in similar or complementary segments of the production process supplying raw materials, goods, and intermediate services.
Geographic proximity also reinforces intensive knowledge spillovers through the exchange of technical and organizational information between firms (Marshall, Citation1961). Externalities can also arise from the diversity of economic activities, called urbanization or diversity economies (Hoover, Citation1937). Diversification allows for more creativity and the exchange of information and experience between local industries (Bracalente et al., Citation2008; Galinari & Lemos, Citation2007; Góis Sobrinho & Azzoni, Citation2014).
Knowledge spillovers arise from non-market interactions (Fujita & Thisse, Citation2013). Therefore, geographical proximity can also be a decisive factor for knowledge exchange and collaborative innovative activities. In this context, the concept of milieu innovateur—i.e., a complex network of social and productive relationships in a limited geographical area that intensifies local innovation capacity and productivity growth through collective learning processes – is more meaningful (Camagni, Citation1991; Capello et al., Citation2011). Studies show that regions with greater concentration of economic activities and with institutional assets, such as universities, research laboratories and other knowledge organizations, even if not directly linked, are more likely to accelerate knowledge and innovation transfer not only within the region but also to neighboring regions (Kauffeld-Monz & Fritsch, Citation2013; Zhou et al., Citation2019). Capello and Lenzi (Citation2013, Citation2015) have shown that innovation impacts the economic performance not only of those regions with a higher intensity of scientific knowledge creation but also neighboring regions.
It is reasonable to expect that the effects of agglomeration economies and knowledge spillovers also holds for exports. Since exports are key determinants of economic growth, countries adopt economic policies to promote them (Herzer & Nowak-Lehmann, Citation2006; Neves et al., Citation2016; Ribeiro et al., Citation2016). Therefore, it is important to study the spatial location pattern of exports and the formation of clusters, as well as the effects of agglomeration economies and knowledge spillover effects on these activities.
In the process of economic growth, more technology-intensive activities have a greater capacity for innovation and knowledge spillovers. Moreover, the higher the technological intensity content of exports, the larger the positive externalities for other sectors (Herzer & Nowak-Lehmann, Citation2006). de Almeida et al. (Citation2022) also point out that industries’ location patterns and their tendency to cluster vary according to their technological intensity. We have therefore our first hypothesis:
Hypothesis 1:
The regional exports of high technology-intensive activities are spatially autocorrelated and form clusters, while the less technology-intensive activities are more randomly distributed.
Regions with an export agenda based on the regional innovative environment are better placed to maintain and expand their international competitiveness (Gama et al., Citation2018) and consequently achieve higher growth (Neves et al., Citation2016). Since innovation efforts are often identified as determinants of exports (Neves et al., Citation2016) and the formation of spatial agglomerations due to patterns of regional concentration and spillovers (Audretsch & Feldman, Citation2004; Fagerberg, Citation1996; López-Bazo & Motellón, Citation2018), our second hypothesis is as follows:
Hypothesis 2:
There is a spatial autocorrelation between exports and R&D expenditure in Brazilian mesoregions, forming clusters of export regions with high innovation environment.
As we have seen, innovation impacts the economic performance not only of those regions with a higher intensity of scientific knowledge creation, but also neighboring regions (Capello & Lenzi, Citation2013, Citation2015). This brings us to our third hypothesis:
Hypothesis 3:
In the high technology-intensive activities, R&D investments benefit not only the exports of own region, but also neighboring regions.
3. Empirical methodology
3.1. Database
The Ministry of Industry, Foreign Trade, and Services of the Brazilian Federal Government is our source of data on exports across 137 mesoregions in Brazil between 2008 and 2021.Footnote1 Such data are provided in accordance with the Mercosur Common Nomenclature (NCM), which is based on the Harmonized Commodity Description and Coding System (HS). A correspondence table between the National Classification of Economic Activities (CNAE) and the NCM was used to match the data by technological intensity sectors (High, Medium-High, Medium and Medium-Low Manufacturing sectors and also Medium-Low and Low Non-Manufacturing sectors), as proposed by Galindo-Rueda and Verger (Citation2016).Footnote2 Export values are originally reported in U.S. dollars. They were converted into Brazilian real using annual average exchange rates (IPEA-DATA) and deflated by the IGP-DI.Footnote3
We also used data on R&D expenditure provided by the National Indicators of Science, Technology and Innovation, Ministry of Science, Technology, Innovation and Communications, also covering the period 2008–2021.Footnote4 They are in millions of Brazilian real and were deflated by the IGP-DI. However, the data are only available for the 27 Brazilian states (26 states plus the Federal District). To address this shortcoming, we used the mesoregion share of number of researchers and teachers in higher education in each of the 27 Brazilian states to allocate R&D expenditure proportionally among mesoregions.Footnote5 The Frascati Manual (OECD, Citation2015) identifies research institutes and the higher education sector as critical elements in the innovation efforts of countries and regions. Moreover, the assumption of a high correlation between R&D expenditure and the number of R&D employees is supported by several research works (e.g., Rehman et al., Citation2020).
3.2. Exploratory spatial data analysis
Regional economic research has emphasized the critical role of spatial proximity by using the econometric technique of Exploratory Spatial Data Analysis (ESDA) to identify spatial autocorrelation and spatial heterogeneity (Anselin, Citation1988; Anselin et al., Citation2008; Florax & Nijkamp, Citation2004; Florax et al., Citation2003; Raiher et al., Citation2017; Vogel & Azevedo, Citation2015). Global Moran’s I is the most commonly used test statistic for estimating global spatial autocorrelation of a variable (Anselin, Citation1996; Moran, Citation1950). The bivariate Moran’s I statistic, in turn, tests the spatial relationship of a variable in a particular region with other variables in surrounding regions.
The Local Indicator of Spatial Association (LISA), also known as Local Moran’s I, is used to calculate the individual contribution of each region to the overall Moran statistic. This disaggregated spatial indicator captures both spatial associations and heterogeneities (Miller, Citation2004). Local Moran’s I can be interpreted as a measure of local spatial agglomeration and can be used to identify local clusters (where adjacent regions have similar attribute values) or spatial outliers (areas distinct from their neighbors) using the Moran scatter plot (Anselin, Citation1995).
Following the same methodology of previous studies (e.g., de Campos et al., Citation2023; Kopczewska et al., Citation2017), we have selected for each sector the spatial weight matrix (W) with the best Moran’s I scores. Accordingly, we selected the spatial contiguity weight matrix of the Queen type for all technological intensity groups.Footnote6
3.3. Empirical model and estimation strategy
To estimate the determinants of regional export growth at sector level, we assume that they can be explained by regional R&D expenditure and by two additional sources of knowledge attributable to agglomeration economies: (i) localization economies or intra-industry specialization economies (SPE), resulting from knowledge related to agglomeration of workers in the same sector in the same regionFootnote7; and (ii) diversity economies (DIV), related to knowledge of workers in other sectors in the same regionFootnote8; that is:
where yirt is the exports of sector i, region r, at time t; rdrt is the (log) R&D expenditure, speirt and divirt are the (log) specialization and diversity economies, respectivelyFootnote9; Zrt is a set of control variables that includes size of region (proxied by region’s real GDP in log form) and year dummiesFootnote10; and is the error term. ( in the Appendix shows the descriptive statistics of variables.)
To estimate the empirical model (1), considering that regional knowledge transmission is not limited by regional boundaries, we adopt an estimation approach that includes spatial interaction effects: endogenous interactions among the dependent variable (Wy), exogenous interactions among the explanatory variables (WX), and interactions among the error terms (Wµ). We have considered the following specifications derived from the general nested spatial model (Carreira & Lopes, Citation2020; Elhorst, Citation2014; O’Connor et al., Citation2018; Posada et al., Citation2018):
i) the Spatial Durbin Model (SDM):
ii) the Spatial Autoregressive Model (SAR):
iii) the Spatial Error Model (SEM):
The model selected is the one that best fits the data.
4. Empirical results and discussion
4.1. Spatial autocorrelation and export clustering
We used Moran’s I statistics to examine the spatial autocorrelation of Brazilian exports by manufacturing and non-manufacturing technological intensity sectors. Overall, Global Moran’s I is statistically significant and positive for all technological intensity sectors and for total exports, except in 2008, indicating spatial autocorrelation of exports across Brazilian mesoregions ().
Table 1. Global Moran’s I for exports by technological intensity, 2008–2021.
The breakdown of exports by technology intensity shows that the High Manufacturing (HM) sector has the highest Global Moran’s I value (except in 2016, 2018, 2020, and 2021), indicating a greater spatial dependence of exports in this sector than in other sectors. On the other hand, the lowest spatial autocorrelation values are observed in the Medium-Low Non-Manufacturing (MLNM) and Low Non-Manufacturing (LNM) sectors, meaning that export activities with lower technological content are more geographically spread across Brazilian mesoregions.
Thus, our results also confirm that the relationship between the location of exports and the level of technological intensity varies significantly. The OECD taxonomy (Galindo-Rueda & Verger, Citation2016), which provides a division between manufacturing and non-manufacturing sectors and then a subdivision within manufacturing, is therefore relevant for this type of study, as it allows a better identification and characterization of sectoral differences. Compared to other studies, we obtained Moran’s I values in line with those of Raiher et al. (Citation2017), who used a different classification, with a relatively high similarity in the technology-intensive sectors and the non-manufacturing sectors. The left graph in shows the Moran scatterplot and the right map the points of the regions on the scatterplot for exports from 2008 to 2021.
Figure 1. Dispersion diagram of Global Moran’s I and LISA cluster map of exports, 2008–2021.

The spatial distribution pattern of Brazilian exports of the High-High type was observed in the southern regions of Brazil, in the state of Rio Grande do Sul with four mesoregions and in the southeastern region, particularly in the states of São Paulo with nine mesoregions and Minas Gerais with three mesoregions (see , and , in the Appendix for a list of mesoregions). Thus, two major agglomerations of exports were identified, showing that mesoregions with high exports are surrounded by neighbors with also high exports, confirming the existence of spatial autocorrelation.
The formation of spatial clusters of the Low-Low type was observed in a much larger number of mesoregions, but they are also grouped in two large clusters. The first is located in the northern region of the country, in the states of Roraima with two mesoregions, Amazonas with two mesoregions, and Acre also with two mesoregions. The other agglomeration is located in the northeast, in the states of Rio Grande do Norte, Paraíba, Piauí and Bahia, each with one mesoregion, in the states of Ceará and Alagoas, each with two mesoregions, and in the states of Pernambuco and Sergipe, each with three mesoregions.
Besides the spatial autocorrelation patterns of the High-High and Low-Low types, the patterns of the High-Low type were found in the northern region, in the state of Amazonas with two mesoregions, and in the northeastern region, in the state of Alagoas with one mesoregion. The Low-High autocorrelation pattern was found in the south-eastern region, in the states of Minas Gerais and São Paulo, each with one mesoregion, and in the southern region, in the states of Paraná and Santa Catarina each also with one mesoregion.
Similar results were reported by Schettini (Citation2019), showing that the northern and central-western regions of Brazil have little or no industrial agglomeration, while the southern and southeastern regions have the most agglomeration in the country. ( in the Appendix presents the dispersion diagram of Global Moran’s I and LISA cluster map of exports by technological intensities). Overall, our results therefore show the formation of two types of spatial clusters: a High-High type cluster in the southeastern and southern regions of the country and a Low-Low type cluster in the northern and part of the northeastern regions. The remaining mesoregions of the country show spatial randomness in their exports and thus no spatial interdependence.
The formation of spatial clusters can also be observed by technological intensity sectors. We only highlight High Manufacturing (HM) in the High-High pattern due to the scale of the task. The High Manufacturing (HM) sector is particularly important because it consists of technology-intensive activities with relatively high levels of knowledge, which generate more regional dynamics and greater knowledge spillovers. We found clusters with the High-High pattern in the southern states Rio Grande do Sul with one mesoregion, Santa Catarina with four mesoregions, and Paraná with three mesoregions. Clustering was also observed in the Southeast region, in the states of São Paulo with 12 mesoregions, Rio de Janeiro with one mesoregion, and Minas Gerais with two mesoregions.
Our results for exports by technological intensity seem to confirm the spatial pattern of innovative activities (i.e., North-South polarization) observed by Gonçalves (Citation2007). The author reported a spatial autocorrelation showing spatial heterogeneity with High-High patterns for the southern region and Low-Low patterns for the northern region.
In short, spatial autocorrelation is associated with manufacturing activities, while non-manufacturing activities, which are less technologically intensive, are more randomly distributed. Thus, the first hypothesis of the study was confirmed, as the formation of a spatial cluster was observed for the southeastern and southern regions, led by the regions of São Paulo, Rio de Janeiro, Minas Gerais and Rio Grande do Sul, which definitely have more technology-intensive export activities.
4.2. Autocorrelation and spatial cluster formation in exports and R&D expenditure
In this section, we use the univariate and bivariate Global Moran’s I and LISA to identify the formation of spatial clusters and to test the occurrence of spatial autocorrelation first in R&D expenditure and then in exports. Several studies have shown that high technology-intensive exports are associated with higher levels of innovation (Audretsch & Feldman, Citation2004; Fagerberg, Citation1988; Guarascio et al., Citation2016; López-Bazo & Motellón, Citation2018).
First, we looked into the existence of spatial autocorrelation of R&D expenditure between Brazilian mesoregions (univariate Moran’s I). As can be seen in , the values are statistically significant throughout the period of analysis, rejecting the hypothesis that there is no spatial autocorrelation. confirms that R&D expenditure shows spatial interdependence between mesoregions, with the formation of spatial clusters in both the southeastern region (states of São Paulo, Rio de Janeiro and Minas Gerais) and the southern region (state of Santa Catarina). These results confirm previous studies that have found a concentration of patents and innovative activities in Brazil (Araújo & Garcia, Citation2019; Gonçalves et al., Citation2019).
Figure 2. Dispersion diagram of Global Moran’s I and LISA cluster map of total R&D expenditure in Brazil, 2008–2021.

Table 2. Global Moran’s univariate I for R&D expenditure and Global Moran’s bivariate I for exports and R&D expenditure by technological intensity, 2008–2021.
In terms of spatial autocorrelation patterns, the High-High type prevailed, meaning that mesoregions with high R&D expenditure were surrounded by mesoregions with high R&D expenditure (). Twenty mesoregions with a High-High pattern shape a large region with the states of São Paulo with 15 mesoregions, Rio de Janeiro with three mesoregions, and Santa Catarina and Minas Gerais with one mesoregion each, forming a large spatial agglomeration of mesoregions with high R&D expenditure. Twelve mesoregions show a Low-Low pattern in the northern region (the states of Amazonas with two mesoregions and Roraima, Pará, Amapá, Acre, and Rondônia with one mesoregion each) and northeastern region (states of Sergipe with two mesoregions and Maranhão, Piauí, and Bahia with one mesoregion each). The rest of the country shows a random pattern (spatial heterogeneity). Moreover, only the state of Amazonas, in the northern region of Brazil, shows a High-Low pattern in one mesoregion, while the Low-High pattern was also only found in one mesoregion in the state of Paraná.
Bivariate analysis of the spatial autocorrelation between total exports and R&D expenditure shows that all Moran’s I values are positive and statistically significant (). Medium Low Manufacturing (MLM) intensity and R&D expenditure have the highest Moran’s I values, followed by Medium High Manufacturing (MHM) and R&D expenditure, suggesting the existence of spatial autocorrelation.
The most technology-intensive export activities (High Manufacturing, HM) are spatially correlated with R&D expenditure. This result supports previous studies that have shown that technological development strongly depends on spatial spillovers (e.g., Gonçalves et al., Citation2019). The importance of local knowledge spillovers has also been highlighted as an explanation for firms’ productivity (Carreira & Lopes, Citation2018).
Regions in Brazil that are more specialized in high-tech and invest more in R&D tend to internalize knowledge and extract gains from the innovation process (de Almeida et al., Citation2021). Thus, corroborating previous studies (Braja & Gemzik-Salwach, Citation2019; Neves et al., Citation2016), the relationship between exports and R&D is more intense in production activities with MLM and HM intensity ().
The spatial autocorrelation pattern is of High-High type for exports associated to R&D expenditure, forming a cluster of 22 mesoregions with high export values associated to high values of R&D in their neighboring mesoregions. There is also spatial autocorrelation in the Low-Low patterns in 11 mesoregions, although more geographically spread out. On the other hand, a large part of Brazil showed spatial randomness in exports and R&D expenditure (), consistent with previous studies (Araújo & Garcia, Citation2019; Gonçalves & Almeida, Citation2009).
Figure 3. Dispersion diagram of bivariate Global Moran’s I and LISA cluster map of exports and R&D expenditure, 2008–2021.

Overall, we found the existence of spatial autocorrelation of exports and R&D expenditure between Brazilian mesoregions, mainly for manufacturing activities, confirming the second hypothesis. The results also reveal the existence of a cluster with a High-High pattern for a large area of the country in the southeastern and southern regions. The mesoregions that comprise this cluster have nationally and internationally recognized teaching and research institutions, namely the University of São Paulo (USP), the University of Campinas (UNICAMP), the Federal University of Rio de Janeiro (UFRJ), and the Federal University of Paraná (UFPR), with relevant interaction and cooperation with the productive sector of the economy. In fact, enhancement of the university-industry relationship has been reported, particularly among the twelve largest universities located in the southern and southeastern regions of Brazil (Bastos & Britto, Citation2017; Fischer et al., Citation2019). Moreover, more university research is positively correlated with higher levels of innovation in the respective region (Araújo & Garcia, Citation2019).
4.3. Knowledge and agglomeration effects measurement
In this section, we estimate the determinants of regional exports by technological intensity, considering regional R&D expenditure and two sources of knowledge explained by agglomeration economies, specialization economies and diversity economies, assuming that there are spatial effects. We also consider regional real GDP to control for the region size and year dummies.
shows the estimates of model (1) without spatial interactions, using the fixed effects estimator with time fixed effects. We observe a positive impact of R&D on exports in the MM and ML sectors. Surprisingly, R&D does not seem to have any impact on the HM sector. Specialization economies have a positive effect in the MHM, MLM, MLNM and LNM sectors and a negative effect in the HM sector. In turn, the effect of diversification economies has only a significantly positive impact in the HM and MM sectors and a negative impact in the MLM sector.
Table 3. Regression results using fixed effect (without spatial interactions).
To account for spatial effects in our analysis, we first performed diagnostic tests to select the most appropriate spatial panel model to fit the data for each sector (). In all sectors, random effects were rejected according to the Hausman test and, consequently, fixed effects were used. The most appropriate spatial panel model that fits our data was selected according to the strategy used by LeSage and Pace (Citation2009, Citation2011), Elhorst (Citation2010), and Belotti et al. (Citation2016). As can also be seen in , the spatial model to be applied to the HM and LNM sectors is the Spatial Durbin Model (SDM), while the Spatial Autoregressive Model (SAR) is applied to the MHM and MM, and the Spatial Error Model (SEM) to the MLM and MLNM sectors.
Table 4. Diagnostic tests.
shows the regression results by sector using the selected spatial model. As expected, the estimated coefficients vary by sector. In what concerns non-spatially lagged variables, the estimated coefficients for R&D are positive and statistically significant in the MM, MLM and MLNM sectors, but not in the HM sector (the interpretation of this result is provided below). The effect of specialization economies is statistically significant and positive in the MHM, MLM, MLNM and LNM sectors, while it is negative in the HM sector. The negative sign in this sector could be explained by the congestion effect (i.e., negative externalities of agglomeration). In fact, Góis Sobrinho and Azzoni (Citation2014) found industrial agglomerations, namely in the motor vehicle industry (technology-intensive industry) in the southeast (São Paulo and Minas Gerais) and southern (Paraná and Rio Grande do Sul) regions. In contrast, the authors found that medium and medium-low technology-intensive sectors are more spatially dispersed. Finally, the effect of diversity economies is significantly positive in the MM sector and negative in the MLM sector.
Table 5. Regression results using spatial panel specification.
Given the spatial autocorrelation of exports observed in Section 4.1, it is not surprising that there are significant and positive global spatial spillover effects of exports in the HM sector. That is, it seems that the regional knowledge on foreign markets spill over between regions. Andersson and Weiss (Citation2012) also found that the probability of a Swedish firm exporting is positively related to the local presence of exporters, with the effect being more important in contract-intensive industries – the contract-intensive industries are essentially skill intensive (Nunn, Citation2007), whose knowledge on foreign markets tends to be more important due to high entry costs. The knowledge on foreign market tends to spill over to local firms, lowering their entry costs (Andersson & Weiss, Citation2012). Conversely, in the case of the MM sector, exporting of surrounding regions will tend to reduce the export activity in its own region.
Regarding the spatial interaction effects of R&D expenditure, we observe that R&D has a positive statistically significant effect in the cases of the HM and LNM sectors. This result is not surprising in Brazil, considering that, as we had seen in Section 4.2, innovative knowledge resulting from investments in R&D tends to be concentrated in a large export area in the southeastern and southern regions with a relevant number of nationally and internationally recognized universities and research institutions (). The university-industry relationship is more compelling in the high technology-intensive sector than in other sectors (Araújo & Garcia, Citation2019; Bastos & Britto, Citation2017; Fischer et al., Citation2019). Thus, it is more important for an export region of HM goods to be surrounded by areas with high R&D expenditure than for that region to make its own R&D investments, which is not the case for less technology-intensive goods – note that the R&D coefficient in the HM sector is not statically significant, which is not the case of medium and medium-low technology-intensive sectors where the coefficients are significantly positive. When we estimate the model (3) at the state level (i.e., at a more aggregate level), the R&D coefficient in the HM sector is now positive and statistically significant, which seems to confirm our conclusion (the result is available from the authors upon request).
We also found negative effects for spatially lagged specialization and diversity economies in the case of the HM sector. This could possibly be due to the mobility of workers between regions, which is relatively high in Brazil, as noted by Gonçalves et al. (Citation2016), who analyzed the impact of the migration of highly skilled workers between regions on innovation in the destination region. Ruesga et al. (Citation2014) and Lameira et al. (Citation2020) also observed high worker mobility in Brazil.
Finally, shows the spatial exogenous effects, namely the direct, indirect and total effects of each independent variable, as well as the feedback effect. In the case of the HM sector, the results seem to confirm the previous conclusion that R&D expenditure of neighbor regions is determinant for exports of the region (the indirect and total effect are positive and statically significant, while the direct effect is not statically significant), favoring the formation of clusters, as we observed in section 4.2.
Table 6. Direct, spatial spillover and feedback effects.
The effects of the remaining independent variables and sectors are consistent with the previous results. In the MM sector, for example, we found significant positive direct effects of R&D and diversity economies, which are moderated by the indirect negative effects of neighboring regions. The feedback effect is also positive and statically significant for the two variables. (The feedback effect measures the impact on the dependent variable of a given region as a result of a change in an independent variable of that region affecting the dependent variable of other regions, which in turn exerts a feedback effect on the dependent variable of the region where the first change occurred.)
5. Conclusions
This study investigated the spatial autocorrelation patterns of regional exports in Brazil and their determinants by technological intensity. It was argued that regions with higher export intensity and higher R&D expenditure are more likely to form spatial clusters, particularly of the High-High type, indicating a greater capacity for knowledge transfer between regions.
The results show that Brazilian exports exhibit spatial autocorrelation, particularly in the High Manufacturing (HM), Medium High Manufacturing (MHM), and Medium Manufacturing (MM) sectors. Non-manufacturing sectors, which are less technology-intensive, show lower spatial dependence than manufacturing sectors. The results also show the formation of mainly two types of spatial clusters: a High-High type cluster in the southeastern and southern regions of Brazil and a Low-Low type cluster in the northern and part of the northeastern regions. The remaining mesoregions of the country show spatial randomness in their exports and thus no spatial interdependence.
We also found a spatial autocorrelation of exports and R&D expenditure between Brazilian mesoregions, especially for manufacturing sectors. Mesoregions with high export values were surrounded by neighboring mesoregions with high R&D expenditure, revealing the existence of a cluster with a High-High pattern in the southeastern and southern regions.
Estimates of the determinants of regional exports by technological intensity using spatial econometric techniques confirm the existence of a spatial spillover effect of exports between neighboring regions in the HM sector. In addition, we also found a spatial spillover effect of R&D expenditure. That is, an increase in R&D expenditure in a neighboring region increases the region’s export of HM goods.
These results have helped broaden the range of empirical studies that have found a relationship between exports and R&D expenditure. As far as structural policies are concerned, our results recommend not only incentives for regional R&D investments but, more importantly, measures to improve the mechanisms of regional spillovers, especially through cooperation and productive complementarity between regions. In this sense, regional policies to strengthen partnerships through support networks, such as the Quadruple Helix (Carayannis & Campbell, Citation2009), seem to be the most promising way to improve the competitiveness of the Brazilian export sector.
The results of this study also reveal some limitations, namely the fact that more variables are not available at the mesoregion level to better represent the stock of knowledge. It should be noted that future studies should focus on the interactions between universities (and research laboratories) and industries and examine the correlation between exports and the level of technological intensity. In addition, the selection of the spatial weighting matrix provided new insights that could contribute to future studies using this econometric tool. However, further research on this topic is needed (Corrado & Fingleton, Citation2012). These limitations open opportunities for future research.
Disclosure statement
No potential conflict of interest was reported by the author(s).
Additional information
Funding
Notes on contributors
Antonio Carlos de Campos
Antonio Carlos de Campos is an Associate Professor in the Department of Economics, at the State University of Maringá and the Postgraduate Programme in Economic Sciences (PCE/UEM), Brazil. He holds a PhD from the Federal University of Paraná (UFPR), Brazil, and a Post-Doctorate from the Faculty of Economics of the University of Coimbra (FEUC), Portugal. His research interests lie in the areas of agglomeration economies, regional development, and technological innovation.
Luís Lopes
Luís Lopes is an Assistant Professor at the Faculty of Economics of the University of Coimbra, Portugal and holds a PhD in Economics at the same University. He is a researcher at the CeBER research centre. His current research activities include agglomeration economies, firm-level studies, productivity, input-output analysis, economic base models, international trade, global macroeconomic policy.
Carlos Carreira
Carlos Carreira is an Associate Professor of Economics at the Faculty of Economics of the University of Coimbra and a researcher at the Centre for Business and Economics Research (CeBER). He holds a PhD in Economics at the University of Coimbra and has been a visiting scholar at the Centre for European Economic Research (ZEW), Mannheim (2015), and Carnegie Mellon University (CMU), Pittsburgh (2011), among others. His primary research interests are applied microeconomics and industrial organisation. In particular, he has been analysing the firm dynamics and their relationship with productivity, innovation, financial constraints, and exports.
Notes
1 In 2019 this Ministry was transformed into a Secretariat under the Ministry of Economy.
2 Correspondence tables can be found at: https://concla.ibge.gov.br.
3 IGP-DI – general – centred - end of period – index (Aug. 1994 = 100) Frequency: Annual from 1944 until 2021. Source: Fundação Getúlio Vargas, Conjuntura Econômica – IGP (FGV/Conj. Econ. - IGP), http://www.fgv.br.
4 The 2020 and 2021 values were obtained using autoregressive techniques of order 1. Source: https://www.mctic.gov.br/mctic/opencms/indicadores/indicadores_cti.html.
5 The source of this data was RAIS (Annual Social Information Report) from the Ministry of Labor and Employment, based on the Brazilian Classification of Occupations (COB), codes 203 and 234 for Researchers and Higher Education Professors, respectively.
6 Other nearest neighbor spatial weight matrices (3NN, 5NN, 7NN and 9NN) were also tested, but with less robust results than those obtained with chosen weight matrix (LeSage & Pace, Citation2014).
7 Formally: , where Lirt is the number of workers in sector i, located in region r, at time t.
8 Formally: , where i’≠i denotes other sectors.
9 To address the problem of zero-valued observations (less than 0.05% of the observations), we replaced all zero values by 0.1 before taking the logarithm. We obtained similar results when we eliminated all zero observations.
10 We also tested for region-specific effects, but these were found to be statistically insignificant in all specifications, which could be due to the fact that the effect is already controlled by the agglomeration and size variables (note that these variables, by definition, show little variation over time).
References
- Andersson, M., & Weiss, J. F. (2012). External trade and internal geography local export spillovers by industry characteristics and firm size. Spatial Economic Analysis, 7(4), 421–27. https://doi.org/10.1080/17421772.2012.722664
- Anselin, L. (1988). Spatial econometrics: Methods and models. Kluwer Academic.
- Anselin, L. (1995). Local Indicators of spatial Association – LISA. Geographical Analysis, 27(2), 93–115. https://doi.org/10.1111/j.1538-4632.1995.tb00338.x
- Anselin, L. (1996). The Moran scatterplot as an ESDA tool to assess local instability in spatial association. In M. M. Fischer, H. J. Scholten, & D. J. Unwin (Eds.), Spatial analytical perspectives on GIS (pp. 121–137). Taylor & Francis. https://doi.org/10.1201/9780203739051
- Anselin, L., Gallo, J. L., & Jayet, H. (2008). Spatial panel econometrics. In L. Mátyás & P. Sevestre (Eds.), The Econometrics of Panel Data. Advanced studies in theoretical and applied Econometrics (Vol. 46). Springer. https://doi.org/10.1007/978-3-540-75892-1_19
- Araújo, V. C., & Garcia, R. (2019). Determinants and spatial dependence of innovation in Brazilian regions: Evidence from a spatial tobit model. Nova Economia, 29(2), 375–400. https://doi.org/10.1590/0103-6351/4456
- Audretsch, D. B., & Feldman, M. P. (2004). Knowledge spillovers and the geography of innovation. In V. Henderson & J. F. Thisse (Eds.), Handbook of regional and urban economics (pp. 2713–2739). Elsevier.
- Baptista, R. (2000). Do innovations diffuse faster within geographical clusters? International Journal of Industrial Organization, 18(3), 515–535. https://doi.org/10.1016/S0167-7187(99)00045-4
- Basile, R., Capello, R., & Caragliu, A. (2012). Technological interdependence and regional growth in Europe: Proximity and synergy in knowledge spillovers. Papers in Regional Science, 91(4), 697–722. https://doi.org/10.1111/j.1435-5957.2012.00438.x
- Bastos, C. P., & Britto, J. (2017). Inovação e geração de conhecimento científico e tecnológico no Brasil: Uma análise dos dados de cooperação da pintec segundo porte e origem de capital. Revista Brasileira de Inovação, Campinas (SP), 16(1), 35–62. https://doi.org/10.20396/rbi.v16i1.8649139
- Belotti, F., Hughes, G., & Mortaria, A. P. (2016). Spatial-panel data models using Stata. Stata Journal, 17(1), 139–180. https://doi.org/10.1177/1536867X1701700109
- Bracalente, B., Perugini, C., & Pompei, F. (2008). What sorts of agglomerations really matter to productivity?: A regional analysis of Europe’s Manufacturing sector. Review of Regional Studies, 38(2), 145–172. https://doi.org/10.52324/001c.8261
- Braja, M., & Gemzik-Salwach, A. (2019). Competitiveness of high-tech sectors in the European Union: A comparative study. Journal of International Studies, 12(2), 213–227. https://doi.org/10.14254/2071-8330.2019/12-2/13
- Camagni, R. (1991). Innovation networks – spatial perspectives. Belhaven Press.
- Capello, R., Caragliu, A., & Nijkamp, P. (2011). Territorial capital and regional growth: Increasing returns in knowledge use. Tijdschrift Voor Economische En Sociale Geografie, 102(4), 385–405. https://doi.org/10.1111/j.1467-9663.2010.00613.x
- Capello, R., & Lenzi, C. (2013). Territorial patterns of innovation: A taxonomy of innovative regions in Europe. The Annals of Regional Science, 51(1), 119–154. https://doi.org/10.1007/s00168-012-0539-8
- Capello, R., & Lenzi, C. (2015). Knowledge, innovation and productivity gains across European regions. Regional Studies, 49(11), 1788–1804. https://doi.org/10.1080/00343404.2014.917167
- Carayannis, E. G., & Campbell, D. F. J. (2009). ‘Mode 3’ and ‘quadruple helix’: Toward a 21st century fractal innovation ecosystem. International Journal of Technology Management, 46(3–4), 201–234. https://doi.org/10.1504/IJTM.2009.023374
- Carreira, L., & Lopes, L. (2018). Regional knowledge spillovers: A firm-based analysis of non-linear effects. Regional Studies, 58(2), 948–958. https://doi.org/10.1080/00343404.2017.1360484
- Carreira, L., & Lopes, L. (2020). How are the potential gains from economic activity transmitted to the labour factor: More employment or more wages? Evidence from the Portuguese context. Regional Science Policy and Practice, 12(2), 319–348. https://doi.org/10.1111/rsp3.12269
- Coe, D. T., & Helpman, E. (1995). International R&D spillovers. European Economic Review, 39(5), 859–887. https://doi.org/10.1016/0014-2921(94)00100-E
- Corrado, L., & Fingleton, B. (2012). Where is the economics in spatial econometrics. Journal of Regional Science, 52(2), 210–239. https://doi.org/10.1111/j.1467-9787.2011.00726.x
- de Almeida, B. P., Gonçalves, E., da Silva, A. S., & Reis, R. C. (2021). Internalization of knowledge spillovers by regions: a measure based on self-citation patents. Annals of Regional Science 66, 309–330. https://doi.org/10.1007/s00168-020-01022-1
- de Almeida, E. T., Silveira Neto, R. M., & Rocha, R. M. (2022). Manufacturing location patterns in Brazil. Papers in Regional Science, 101(4), 839–873. https://doi.org/10.1111/pirs.12672
- de Campos, A. C., Lopes, L., & Carreira, C. (2023). Spatial autocorrelation of exports and R&D expenditures in Portugal. Journal of the Knowledge Economy, forthcoming. https://doi.org/10.1007/s13132-023-01425-3
- Döring, T., & Schnellenbach, J. (2006). What do we know about geographical knowledge spillovers and regional growth? A survey of the literature. Regional Studies, 40(3), 375–395. https://doi.org/10.1080/00343400600632739
- Duschl, M., Scholl, T., Brenner, T., Luxen, D., & Raschke, F. (2015). Industry-specific firm growth and agglomeration. Regional Studies, 49(11), 1822–1839. https://doi.org/10.1080/00343404.2013.861059
- Elhorst, J. P. (2010). Applied spatial econometrics: Raising the bar. Spatial Economic Analysis, 5(1), 9–28. https://doi.org/10.1080/17421770903541772
- Elhorst, J. P. (2014). Spatial econometrics. From cross-sectional data to spatial panels. Springer.
- Fagerberg, J. (1988). Why growth rates differ. In G. Dosi, C. Freeman, R. Nelson, G. Silverberg, & L. Soete (Eds.), Technical change and economic theory (pp. 432–527). Pinter Publishers.
- Fagerberg, J. (1996). Technology and competitiveness. Oxford Review of Economic Policy, 12(3), 39–51. https://doi.org/10.1093/oxrep/12.3.39
- Fischer, B. B., Schaeffer, P. R., & Vonortas, N. S. (2019). Evolution of university-industry collaboration in Brazil from a technology upgrading perspective. Technological Forecasting and Social Change, 145, 330–340. https://doi.org/10.1016/j.techfore.2018.05.001
- Florax, R. J. G. M., Folmer, H., & Rey, S. J. (2003). Specification searches in spatial econometrics: The relevance of Hendry’s methodology. Regional Science and Urban Economics, 33(5), 557–579. https://doi.org/10.1016/S0166-0462(03)00002-4
- Florax, R. J. G. M., & Nijkamp, P. (2004). Misspecification in linear spatial regression models. In K. Kempf-Leonard (Ed.), Encyclopedia of social measurement (pp. 695–707). Academic Press.
- Fugita, M., Krugman, P., & Venables, J. A. (2002). Economia espacial: Urbanização, prosperidade econômica e desenvolvimento humano no mundo. Futura.
- Fujita, M., & Thisse, J. F. (2013). Economics of agglomeration: Cities, industrial location and regional growth (2nd ed.). Cambridge University Press.
- Galinari, R., & Lemos, M. B. (2007). Economias de aglomeração no Brasil: Evidências a partir da concentração industrial paulista. ANPEC - Anais do XXXV Encontro Nacional de Economia [Proceedings of the 35th Brazilian Economics Meeting. Retrieved July , 2022, from http://www.anpec.org.br/encontro2007/artigos/A07A124.pdf
- Galindo-Rueda, F., & Verger, F. (2016). OECD taxonomy of economic activities based on R&D intensity. OECD Science, Technology and Industry. Working Papers nº. 04. OECD Publishing. https://doi.org/10.1787/18151965
- Gama, R., Barros, C., & Fernandes, R. (2018). Science Policy, R&D and knowledge in Portugal: An Application of Social network analysis. Journal Knowledge Economics, 9, 329–358. https://doi.org/10.1007/s13132-017-0447-3
- Góis Sobrinho, E. M., & Azzoni, C. R. (2014). Aglomerações industriais relevantes do Brasil. TD Nereus 07-2014 São Paulo. http://www.usp.br/nereus/wp-content/uploads/TD_Nereus_07_2014.pdf
- Gonçalves, E. (2007). O padrão espacial da atividade inovadora brasileira: Uma análise exploratória. Estudos Econômicos (São Paulo), 37(2), 405–433. https://doi.org/10.1590/S0101-41612007000200007
- Gonçalves, E., & Almeida, E. (2009). Innovation and spatial knowledge spillovers: Evidence from Brazilian patent data. Regional Studies, 43(4), 513–528. https://doi.org/10.1080/00343400701874131
- Gonçalves, E., Matos, C., & Araújo, I. F. (2019). Path-dependent dynamics and technological spillovers in the Brazilian regions. Applied Spatial Analysis and Policy, 12(3), 605–629. https://doi.org/10.1007/s12061-018-9259-5
- Gonçalves, E., Ribeiro, D. R. S., & Freguglia, R. S. (2016). Skilled labor mobility and innovation: A study of Brazilian microregions. Pesquisa E Planejamento Econômico, 46(2), 181–211. http://repositorio.ipea.gov.br/handle/11058/7294
- Guarascio, D., Pianta, M., & Bogliacino, F. (2016). Export, R&D and new products. A model and a test on European industries. Journal of Evolutionary Economics, 26(4), 869–905. https://doi.org/10.1007/s00191-016-0445-9
- Guastella, G., & van Oort, F. G. (2015). Regional heterogeneity and Interregional research spillovers in European innovation: Modelling and policy implications. Regional Studies, 49(11), 1772–1787. https://doi.org/10.1080/00343404.2015.1034668
- Herzer, D., & Nowak-Lehmann, D. (2006). What does export diversification do for growth? An econometric analysis. Applied Economics, 38(15), 1825–1838. https://doi.org/10.1080/00036840500426983
- Hoover, E. M., Jr. (1937). Location theory and the shoe and leather industries. Harvard University Press.
- Jones, C., & Jordan, D. (2019). Agglomeration, urbanization and competitive performance: The natural experiment of English football. Regional Studies, Regional Science, 6(1), 421–438. https://doi.org/10.1080/21681376.2019.1647794
- Kauffeld-Monz, M., & Fritsch, M. (2013). Who are the knowledge brokers in regional systems of innovation? A multi-actor network analysis. Regional Studies, 47(5), 669–685. https://doi.org/10.1080/00343401003713365
- Kopczewska, K., Kudła, J., & Walczyk, K. (2017). Strategy of spatial panel estimation: Spatial spillovers between taxation and economic growth. Applied Spatial Analysis and Policy, 10(1), 77–102. https://doi.org/10.1007/s12061-015-9170-2
- Krugman, P. (1991). Increasing returns and economic geography. Journal of Political Economy, 99(3), 483–499. https://doi.org/10.1086/261763
- Lambooy, J. G. (2010). Knowledge transfers, spillovers and actors: The role of context and Social capital. European Planning Studies, 18(6), 873–891. https://doi.org/10.1080/09654311003701407
- Lameira, V. C., Gonçalves, E., & Freguglia, R. (2020). Regional absorption capacity and migration decisions in Brazil. Area Development and Policy, 5(4), 351–375. https://doi.org/10.1080/23792949.2020.1750304
- LeSage, J. P., & Pace, R. K. (2009). Introduction to spatial econometrics. CRC Press. https://doi.org/10.1201/9781420064254
- LeSage, J. P., & Pace, R. K. (2011). Pitfalls in higher order model extensions of basic spatial regression methodology. Review of Regional Studies, 41(1), 13–26. https://doi.org/10.52324/001c.8141
- LeSage, J., & Pace, R. K. (2014). The biggest myth in spatial econometrics. Econometrics, 2(4), 217–249. https://doi.org/10.3390/econometrics2040217
- López-Bazo, E., & Motellón, E. (2018). Firm exports, innovation and the regional dimension in Spain. Regional Studies, 52(4), 490–502. https://doi.org/10.1080/00343404.2017.1332406
- Lundvall, B. A. (1992). National systems of innovation: Towards a theory of innovation and interactive learning. Pinter Publishers.
- Marshall, A. (1961). Principles of economics (9th ed.). Macmillan.
- Miller, H. J. (2004). ‘Tobler’s first law and spatial analysis. Annals of the Association of American Geographers, 94(2), 284–289. https://doi.org/10.1111/j.1467-8306.2004.09402005.x
- Moran, P. A. P. (1950). Notes on continuous stochastic phenomena. Biometrika, 37(1–2), 17–23. https://doi.org/10.2307/2332142
- Neves, A., Teixeira, A. A. C., & Silva, S. T. (2016). Exports-R&D investment complementarity and economic performance of firms located in Portugal. Investigación Económica, LXXV(295), 125–156. https://doi.org/10.1016/j.inveco.2016.03.004
- Nunn, N. (2007). Relationship-specificity, incomplete contracts and the pattern of trade. Quarterly Journal of Economics, 122(2), 569–600. https://doi.org/10.1162/qjec.122.2.569
- O’Connor, S., Doyle, E., & Doran, J. (2018). Diversity, employment growth and spatial spillovers amongst Irish regions. Regional Science and Urban Economics, 68, 260–267. https://doi.org/10.1016/j.regsciurbeco.2017.11.002
- OECD. (2015). Frascati manual 2015: Guidelines for collecting and reporting data on research and experimental Development, the measurement of scientific, technological and innovation activities. OECD Publishing. https://doi.org/10.1787/9789264239012-en
- Posada, D. G., Morollón, F. R., & Viñuela, A. (2018). The determinants of local employment growth in Spain. Applied Spatial Analysis and Policy, 11(3), 511–533. https://doi.org/10.1007/s12061-017-9226-6
- Raiher, A. P., Carmo, A. S. S., & Stege, A. L. (2017). The effect of technological intensity of exports on the economic growth of Brazilian microregions: A spatial analysis with panel data. EconomiA, 18(3), 310–327. https://doi.org/10.1016/j.econ.2017.03.001
- Rehman, N. U., Hysa, E., & Mao, X. (2020). Does public R&D complement or crowd-out private R&D in pre and post economic crisis of 2008? Journal of Applied Economics, 23(1), 349–371. https://doi.org/10.1080/15140326.2020.1762341
- Ribeiro, A. P., Carvalho, V., & Santos, P. (2016). Export-led growth in the EU: Where and What to export? The International Trade Journal, 30(4), 319–344. https://doi.org/10.1080/08853908.2016.1197806
- Ruesga, S., Bichara, J. S., & Monsueto, S. E. (2014). Labor mobility, informality and wage inequality in Brazil. Investigación Económica, 73(288), 59–80. https://doi.org/10.1016/S0185-1667(14)70919-1
- Schettini, D. (2019). As exportações industriais regionais brasileiras: Análise espacial da primeira década de 2000 | the Brazilian regional industrial exports: Spatial analysis of the first decade of the 2000s. Revista Brasileira de Estudos Urbanos E Regionais, 21(2), 298–311. https://doi.org/10.22296/2317-1529.2019v21n2p298
- Storper, M., & Venables, A. J. (2004). Buzz: Face-to-face contact and the urban economy. Journal of Economic Geography, 4(4), 351–370. https://doi.org/10.1093/jnlecg/lbh027
- Tsvetkova, A., Ahrend, R., Martins, J. O., Lembcke, A., Knutsson, P., Jong, D., & Terzidis, N. (2020). The spatial dimension of productivity: Connecting the dots across industries, firms and places. OECD Regional Development Working Papers, No. 2020/01, OECD Publishing. https://doi.org/10.1787/ba5edb47-en.
- van Oort, F. G., Burger, M. J., Knoben, J., & Raspe, O. (2012). Multilevel approaches and the firm-agglomeration ambiguity in economic growth studies. Journal of Economic Surveys, 26(3), 468–491. https://doi.org/10.1111/j.1467-6419.2012.00723.x
- Vogel, G., & Azevedo, A. F. Z. (2015). Intensidade Tecnológica das exportações do Brasil e de Estados Selecionados (2000-2010). Revista De Administração Da UFSM, 8(1), 26–41. https://doi.org/10.5902/198346596708
- Zhou, Y., Zhu, S., & He, C. (2019). Learning from yourself or learning from neighbours: Knowledge spillovers, institutional context and firm upgrading. Regional Studies, 53(10), 1397–1409. https://doi.org/10.1080/00343404.2019.1566705
Appendix
Table A1. Descriptive statistics, 2008–2021.
Table A2. Spatial auto-correlation pattern (High-High and Low-Low) of total exports, 2008–2021.
Table A3. Spatial auto-correlation pattern of total exports, of the technological intensity of High Manufacturing (HM), for the High-High pattern, 2008–2021.
Table A4. Spatial auto-correlation pattern (High-High and Low-Low) of R&D expenditures, 2008–2021.