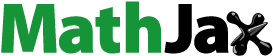
ABSTRACT
This study investigates the impact of uncertainty on income inequality in developing countries. Unlike prior studies, we analyse the role that the fiscal policy could play in the relationship. Using a sample of 66 developing countries over the period 2000–2020, we find at several levels of robustness that, uncertainty increases income inequality in developing countries, especially those with fiscal deficits and less fiscally disciplined. Additionally, we find that fiscal surpluses mitigate the negative effects of uncertainty on wealth distribution which in turn reduces income inequality. This result reveals the socio-economic stabilizing role that fiscal policy management could play during periods of uncertainty. Fiscal policy can maintain and enhance economic stability when countries face certain uncertainty.
1. Introduction
The macroeconomic volatility that accompanied the recession of 2010 has stimulated new literature on how uncertainty impacts economic activity and especially decision-making (Bloom, Citation2014). In recent years, major challenges have emerged, causing global political and economic uncertainty. Successive events in the aftermath of the financial crisis of 2007 such as the “Arab Spring”, Brexit, the trade war between the U.S. and China under the Trump Administration, the COVID-19 pandemic and now the Russia-Ukraine war, have raised global uncertainty. The level of uncertainty is now higher and more important than ever before ().
The role of uncertainty in economic fluctuations is at the core of economic decisions. However, its distributional effects have received little attention. Despite the growing attention of both Economists and Policymakers on the topic, little effort has been devoted to studying the effects of uncertainty on public choices, and its socio-economic implications, especially in developing countries. The negative effectsFootnote1 of uncertainty with the COVID-19 pandemic and the conflict between Russia and Ukraine are some examples of the importance of considering uncertainty in economic analyses and decision-making. Indeed, the analysis of the effects of uncertainty could allow decision-makers to make some reforms to reduce or avoid the economic effects of uncertainty shocks (Bonfiglioli et al., Citation2022).
According to the literature (Bloom, Citation2014), uncertainty impacts economic activity through three main channels: (1) real-options effects, (2) risk-premium effects and (3) precautionary-savings effects. Regarding the real-options effects, firms or companies become more cautious when decisions are costly to revert, delaying hiring and investments while they wait for more information, which as a consequence, leads to a decline in the productivity and a rise in the unemployment rate (Bernanke, Citation1983; Dixit et al., Citation1994). Regarding risk-premium effects, an increase in uncertainty raises the probability of default and, consequently, the risk in the financial market (Christiano et al., Citation2014). Indeed, foreign investors may be particularly sensitive to the level of risk in the financial market. When the risk increases, foreign investors may withdraw their capital from the market, which can lead to capital outflow and depreciation of the national currency. This may have implications for macroeconomic stability and the country’s ability to import goods and services. Like firms, households that are unsure about future income, postpone consumption particularly of durable goods, as a precautionary measure (Romer, Citation1990). In fact, when households delay purchases or reduce spending, aggregate demand for goods and services declines. This can lead to a decline in the production and sale of goods and services, which in turn can slow economic growth.
Uncertainty can affect income inequality. Indeed, as uncertainty affects asset prices, its impact on wealthy and poor households is not homogenous. Wealthy households are often more resilient to shocks triggered by uncertainty than poorer households. This reality contributes to increasing income inequality if measures are not taken by policymakers to support poor households. Despite the fact the literature on the effect of uncertainty on income inequality is not abundant, it has been proven that this effect can depend on factors such as education for example (Brueckner & Vespignani, Citation2017). In this paper, we assume that this effect could depend on an economic resilience factor: the fiscal position. Indeed, the fiscal position of a country reflects its ability to self-finance targeted spending for mitigating as soon as possible, the adverse effects of uncertainty or a crisis. When a developing country presents a fiscal surplus, it is more reactive in the adoption of measures and precisely countercyclical policies to mitigate the adverse effects of uncertainty (Bénassy-Quéré et al., Citation2016). It is not the case for a country which presents fiscal deficits during periods when negative effects of uncertainty occur. In this case, the country becomes less reactive and then adopts cyclical policies which can amplify the negative effects of uncertainty.
The current study is relevant because the reduction of income inequality is a crucial goal of sustainable development (SDG 10). Indeed, income inequality is considered as a factor that slows economic growth (Alesina & Perotti, Citation1996; Stiglitz, Citation2015), increases unemployment, especially youth unemployment (Adjor & Kebalo, Citation2018), can cause insecurity and political instability and reduce the pace at which growth translates into poverty reduction (Batuo et al., Citation2022; Bourguignon, Citation2004). In the last decade, developing countries have achieved more progress in growth, but income inequality didn’t fall (Batuo et al., Citation2022; Bicaba et al., Citation2017; Kebalo et al., Citation2022). The difficult operation of income inequality reduction in developing countries, despite the multiple traditional – economic, institutional, and social – policies, calls for more caution about how specialists and researchers analyse the determinants of income inequality. Moreover, developing countries are most of the time more exposed to the negative effects of global uncertainty. Thus, during periods of high uncertainty, most of them are often obliged to suspend their economic development programs that are intended to help to reduce inequalities and poverty. In addition, by exploring the fiscal position channel as a way to reduce income inequality in times of uncertainty, the paper highlights the need for developing countries to improve their fiscal policies and, thereby, foster economic development of the States concerned.
The contribution of this paper is twofold. First, we use an innovative measure of uncertainty. Indeed, uncertainty is a subjective feeling about the economy and is therefore not directly observable. Therefore, the main challenge for Economists is to find an appropriate indicator to measure it. In most cases, proxies which are indices, are used. These indices, either don’t varyFootnote2 across countries or are just availableFootnote3 for a group of emerging and developed countries. In this study, we use an innovative measure of uncertainty developed by a team of experts from the International Monetary Fund (Ahir et al., Citation2022): the World Uncertainty Index. This innovative index is superior in quality to other measures of uncertainty because it is the first comparable uncertainty index across developing, developed, and emerging countries, and it includes both political and economic uncertainties.
Second, we analyse the effect of uncertainty on income inequality in developing countries by considering their fiscal position as explained above. Indeed, the literature in this area has explored other factors that can help mitigate the impact of uncertainty on income inequality, such as human capital, the degree of openness of economies, the higher employment rate and the share of employees working in the manufacturing sector (Fawaz et al., Citation2012; Fischer et al., Citation2021; Thye et al., Citation2021). In this paper, we think that fiscal policy management can also play a role in the relationship between uncertainty and income inequality. The objective of doing this is to perform a robustness analysis. In addition, we want to propose according to the obtained results, targeted policies that could help countries to become more resilient during periods of uncertainty.
Given data availability, the analysis is performed on a panel set of 66 developing countries and covers the period 2000–2020. To obtain reliable results, the generalized method of moments (GMM) is used to address, amongst others, problems of simultaneity and endogeneity. Results show that uncertainty, no matter the measure used, increases income inequality. Having a fiscal deficit (respectively fiscal surplus) contributes to exacerbate (respectively to reduce) income inequality in developing countries. Our analysis shows that during periods of uncertainty, fiscal surpluses help countries to mitigate the negative effects of uncertainty on wealth distribution, and therefore, contribute to reducing income inequality. However, for countries with fiscal deficits, less fiscally disciplined, uncertainty contributes to increasing income inequality.
The rest of the paper is organized as follows. Section 2 presents a literature review. Section 3 presents the data and discusses the estimation strategy. Section 4 presents and discusses the results. Section 5 concludes the analysis with economic policy recommendations.
2. Literature review
With the multiplicity of economic policies available to address global challenges and the negative effects of shocks, the consideration of uncertainty in economic decision-making and modelling tends to be minimized, especially in developing countries. The importance of uncertainty in economic decision-making is higher than ever in today’s interconnected world; and uncertainty, whether economic, financial, or political, has important implications for economies and economic policies. It has a significant effect on economic growth Balcilar et al. (Citation2017)), consumption and investment (Bernanke, Citation1983), employment (Caggiano et al., Citation2017), commodity prices (Fang et al., Citation2018), bank returns (Pastor & Veronesi, Citation2012), reforms (Bonfiglioli et al., Citation2022), and income inequality (Theophilopoulou, Citation2022). This literature review is focused on three main stands such as the effect of uncertainty on (1) economic activity in general, (2) development policies and reforms, and (3) income inequality.
2.1. Uncertainty and economic activity
According to Bernanke (Citation1983), uncertainty can indeed affect household consumption and investment decisions. It impacts the whole economy by slowing economic growth and delaying consumer spending when uncertainty is high (Bloom, Citation2009). In times of high economic uncertainty, investment and spending become less attractive to the average household (Pastor & Veronesi, Citation2012). Therefore, the average household postpones investment because of the reduction in personal income and/or corporate profitability. Political uncertainty also affects investment. Indeed, companies reduce their investments when there is some uncertainty about political reforms in developing countries.
Regarding the labour market, Bloom (Citation2014) shows that fluctuations in uncertainty often contribute to slow hiring, as companies are generally reluctant to make essential costly decisions in unpredictable regulatory environments. Caggiano et al. (Citation2017) show that the effect of uncertainty on unemployment is significant and even more than what some previous studies (Caggiano et al., Citation2014) have found. Indeed, uncertainty contributes to increasing unemployment volatility, particularly during recessions and periods of high uncertainty. Uncertainty shocks may be more important than monetary policy shocks for understanding the rise in unemployment during recessions. Whether the impact of uncertainty on unemployment is justified, Pierce and Schott (Citation2016) show that the impact on unemployment depends on industry exposure to both economic policies and international trade.
Since the publication of John Kenneth Galbraith’s book “The Age of Uncertainty” in 1977 (Galbraith, Citation1977), it is evident that the financial sector is sensitive to uncertainty. Uncertainty (policy uncertaintyFootnote4) mays significantly increase the risk premiums in various financial markets, thereby increasing borrowing costs, and undercutting productivity, ultimately resulting in poor economic prospects (Gilchrist et al., Citation2014). Uncertainty can also affect bank valuations, where values decline with the level of uncertainty. During periods of high uncertainty, returns are lower than in other periods (Pastor & Veronesi, Citation2012). Commodity prices are also correlated to uncertainty. As uncertainty increases, it leads to movements in commodity prices (Fang et al., Citation2018).
2.2. Uncertainty, development policies and reforms
The negative effects of uncertainty can be a reason to suspend reforms and/or development programs in a country. It can become a barrier to the adoption of reforms. Indeed, according to Fernandez and Rodrik (Citation1991) and Alesina and Drazen (Citation1991), uncertainty can lead to a break in the progress of economic reforms and programs in countries that do not have fiscal surpluses and effective economic instruments to deal with its perverse effects. During periods of high uncertainty, some countries, especially developing countries, without budget surpluses and in a situation of indebtedness, are often forced to suspend momently their economic and social development programs. One such example is the suspension of the Togolese National Development Plan 2018–2022 due to the negative economic effects of the COVID-19 pandemic (reduction in investment for instance). This development plan, originally designed to transform Togo economically and socially, was severely modified with the advent of the COVID-19 pandemic, to be more focused on resilience and economic recovery.
If generally, uncertainty is a barrier to some reforms, it can at the same time encourage the development of new reforms. There is a consensus that reforms are more likely to occur during times of crisis and high uncertainty (Ranciire & Tornell, Citation2016). For Tommasi and Velasco (Citation1996), the uncertainty that follows economic recessions facilitates economic reforms. By using one of the most exhaustive cross-country panel datasets on reforms in six major areas (domestic financial sector, capital account, product markets, agriculture, trade, and current account transactions), Bonfiglioli et al. (Citation2022) show that uncertainty has a positive and significant effect on the adoption of reforms. This result is robust to the inclusion of several variables of control, such as political and economic variables, crisis indicators, and a host of country, reform, and time-fixed effects.
Reforms are performed with the goal to avoid the consequences of future crises. For instance, with the consequences of the COVID-19 pandemic and the conflict between Russia and Ukraine that raised uncertainty around the world, the World Trade Organization (WTO) and countries have committed to some reforms as espoused in the Geneva package. This package of agreements secured at the 12th Ministerial Conference (MC12) was the culmination of efforts by WTO members to provide concrete trade-related responses to important challenges and uncertainty facing the world today. The reforms proposed by the WTO in response to the COVID-19 pandemic, and the food insecurity triggered by the Russia-Ukraine war, will allow countries to better respond to some of the most pressing challenges and the current uncertainty of our time. In addition, to address the challenges of the COVID-19 pandemic, countries have performed reforms to improve their resilience. For example, in some developing countries in Africa, reforms have been made to better digitalize public administration services. The share of social spending (health, education, and social protection) has increased in the budget of many countries. In West Africa, military programming laws have been adopted to increase the share of spending on security defence to limit the negative effects of uncertainty associated with insecurity. This reform would help countries to maintain investors’ confidence and preserve investments. All these examples show the central role that uncertainty plays in the adoption of reforms.
2.3. Uncertainty and income inequality
Uncertainty appears to account significantly for the variation in income and consumption inequality (Theophilopoulou, Citation2022). But the literature on the effect of uncertainty on income inequality remains less abundant. The important question that arises is how income inequality is affected by uncertainty.
Households are not affected in the same way by uncertainty. Wealthy households are often more resilient to uncertainty than poor households. Uncertainty shocks affect income inequality depending on some factors. For Brueckner and Vespignani (Citation2017), the effect of uncertainty on income inequality depends on the level of education of the population, and therefore, on its ability to anticipate global events than can affect economies. Indeed, according to these authors, in a country where only a small share of the population is educated, an increase in uncertainty is associated with a significant increase in income inequality. Wealthy households (a small share of the population), which are most of the time more educated, are often more resilient than poor and vulnerable households to uncertainty shocks because they can anticipate events that could affect negatively economic activity. But for the large share of the population composed of poor, vulnerable, and middle-income households, and without a high level of education, it would be difficult to anticipate uncertain events that could affect them economically and socially. Then, during periods of uncertainty, the consequence will be the accentuation of income inequalities, especially when economic and social measures – such as cash transfers, subsidies for necessities, tax breaks and banking facilities for instance, – are not taken by policymakers in favour of the large share of the population. Therefore, according to Brueckner and Vespignani (Citation2017), as the education level of the population increases, the correlation between uncertainty and income inequality decreases.
On the other hand, Fawaz et al. (Citation2012) show strong evidence that business cycle fluctuations contribute to exacerbate income inequality in high income developing countries, while they help narrow the gap in low income developing countries. Moreover, they find that schooling helps reduce income inequality in both country groups, while investment increases income inequality. For their part, Fischer et al. (Citation2021) show that income inequality decreases in most states in the United States of America, with a pronounced degree of heterogeneity in terms of the dynamic responses. In contrast, some few states, mostly located in the Midwest, display increasing levels of income inequality over time. They explain that the difference in the reaction of household income inequality is affected by the composition of income and labour market fundamentals such as sectoral employment.
For Obiakor et al. (Citation2022), the short-run effects of economic size and uncertainty on income inequality is positive and insignificant. The effect in the long run remains positive and insignificant for economic size, but negative and significant for uncertainty. The effects of other variables like government expenditure and birth rate are negative and statistically significant. Thye et al. (Citation2021) indicate that the impact of uncertainties on income inequality on the ASEAN-5 countries was more significant in the long run than in the short run. Indeed, their results indicate that, in the long run, the income inequality of the ASEAN-5 countries was more sensitive to external uncertainty shocks than to internal uncertainty shocks. In particular, external uncertainty (respectively internal uncertainty) was a significant determinant of income inequality of all countries (respectively of Malaysia and Thailand). In addition, in the short run, while external uncertainty affected Thailand’s income inequality level significantly, internal uncertainty was found to matter for the income inequality level of Malaysia, Singapore and Thailand. Moreover, they demonstrate that real per capita income and inflation worsen income inequality, while a higher employment rate will have a corrective impact on the income inequality level.
Whether the effect of uncertainty on income inequality depends on education and the economic and social measures undertaken by countries, the important question is how to finance them. The management of fiscal policy is the answer. That’s why in this paper, we analyse the effect of uncertainty on income inequality in developing countries by considering the role that can play the fiscal policy.
3. Data and methodology
This section highlights the data used and describes the methodology mobilized. Some stylized facts are presented to explain more about the behaviour of our variables and their relationships.
3.1. Data and sources
The data used have been compiled from different sources that have been merged into an original and unique dataset. Due to data availability, we consider 66 developing countries, and the econometric analysis focuses on the period 2000–2020. All data are summarized in . Except for data collected on uncertainty which are quarterly and transformed into annual frequency, the remaining data collected are annual.
Table 1. Definition of variables.
3.1.1. Measure of inequality
Income inequality can be measured using various statistical indicators that look at the distribution of income within a population. Among these indicators, there is (i) the Gini coefficient, widely used in the literature (Brueckner & Vespignani, Citation2017; Fawaz et al., Citation2012; Fischer et al., Citation2021; Obiakor et al., Citation2022; Thye et al., Citation2021) to measure income inequality, (ii) the Palma index, which compares the income of the richest 10% of the population with that of the poorest 40%, (iii) the Theil index, measuring both inequality within each population group (intragroup) and inequality between groups (intergroup), (iv) the Lorenz curve, graphically representing the cumulative distribution of income against the ideal equal distribution, and (v) the ratio of P80/P20, which is the ratio of the income of the richest 20% to that of the bottom 20% poorest of the population.
In this study, the choice fell on the Gini coefficient as an indicator for measuring income inequality, particularly in connection with its simplicity. Indeed, it is calculated from a single mathematical formula which takes into account all individual incomes. In addition, the Gini coefficient makes it possible to compare inequalities between different regions, countries, and periods. This makes it a valuable tool for tracking changes in income inequality over time and in different geographic contexts. Moreover, it is sensitive to changes in income distribution. Even slight shifts in the income distribution can lead to significant shifts in the coefficient, making it a useful indicator for spotting trends even in situations where the differences seem subtle. Moreover, the value of the Gini coefficient is between 0 and 100, which makes it easily interpretable. A value close to 0 indicates a very equitable distribution of income, while a value close to 100 indicates maximum inequality. Finally, the Gini coefficient captures the entire income distribution in a single measure, making it possible to synthesize complex income distribution data into a simple and meaningful indicator.
Due to the fact that some countries do not have frequent data on income inequality, to deal with the missing values, we assume a five-year invariance of the Gini index (Ametoglo et al., Citation2018; Dout & Kebalo, Citation2021; Kebalo et al., Citation2022).
presents the overall evolution of income inequality in developing countries. It shows that income inequalities have decreased around the world in the first decade of the 2000s and then stabilized during the second decade. In addition, it highlights a disparity in the level of income inequalities in the different regions of the World. Indeed, developing countries in Latin America and the Caribbean (LAC) present the highest income inequalities level. They are followed by African and Asian developing countries. However, with a lower level of income inequality compared to the developing countries of other continents, European developing countries experienced a substantial decline in income inequality in the early 2000s.
3.1.2. Measure of uncertainty
Uncertainty is defined by Knight (Citation1921) as the inability to define the probability of events, i.e., a complete lack of knowledge about future events. Thus, uncertainty about the economy’s future is reflected in the inability to forecast it, as evidenced by differences in expert forecasts for economic variables. Discussions about the pervasiveness of uncertainty, regardless of its source or persistence, and its effects on the economy quickly lead to the following question: How do economists measure uncertainty? In most cases, proxies which are indices are used. Among the range of proxies used are:
the Economic Policy Uncertainty (EPU) index. It captures the risk in which government policies and regulatory frameworks are undefined for the near future. This phenomenon may lead businesses and individuals to delay spending and investments because of uncertainty in the market. Each EPU index reflects the relative frequency of own-country newspaper articles that contain a trio of terms: economy (E), policy (P) and uncertainty (U). At the moment this article is written, this index is only available for 22 developed and emerging countries.Footnote5 Some works have estimated the economic policy uncertainty and assessed their different economic effects (Arbatli et al., 2022; Armelius et al., Citation2017; Baker et al., Citation2016; Davis, Citation2016; Ghirelli et al., Citation2019; Huang & Luk, Citation2020; Zalla, Citation2017).
the Trade Policy Uncertainty (TPU) index. Defined as the risk of a tariff reversal, trade policy uncertainty has become an important source of economic uncertainty. It is developed by staff in the International Finance Division of the Federal Reserve Board and measures media attention to news related to trade policy uncertainty. The index reflects automated text-search results of the electronic archives of 7 leading newspapers discussing trade policy uncertainty: Boston Globe, Chicago Tribune, Guardian, Los Angeles Times, New York Times, Wall Street Journal, and Washington Post. The index is scaled so that 100 indicates that 1% of news articles contain references to TPU. The limitation of this index is that, as it is constructed today, it does not vary by country. Caldara et al. (Citation2020) and Handley and Limão (Citation2022) conducted the first analysis on the economic effects of trade policy uncertainty.
the World Uncertainty Index (WUI). It assesses the level of uncertainty in countries across the World. This index was proposed by Ahir et al. (Citation2018) who constructed quarterly indices of economic uncertainty for 143 developing, developed and emerging countries from 1996 onwards using frequency counts of “uncertainty” and its variants in the quarterly Economist Intelligence Unit (EIU) country reports. The EIU quarterly reports discuss key political and economic developments in each country, as well as analyses and forecasts of political, policy, and economic conditions.
In this study, we use the World Uncertainty Index (WUI) to appreciate the level of uncertainty in the world. Indeed, the WUI is superior to other uncertainty measures since it is the first uncertainty index constructed for a panel dataset of developed, emerging, and developing countries. In short, the novelty of the WUI is that it is the first uncertainty index, which is comparable across countries. To make the WUI comparable across countries, the raw counts are scaled by the total number of words in each EIU report. However, for the sake of robustness check, we use the Economic Policy Uncertainty (EPU) index and the Trade Policy Uncertainty (TPU) index as alternative measures of uncertainty.
shows the evolution of the World Uncertainty Index. It highlights the generalized rise in uncertainty in the World. It shows three main phases during which uncertainty has increased.
Figure 2. Evolution of world uncertainty index, developing countries.

The first phase, from 2000 to 2003, highlights the uncertainty that resulted from events such as the dot-com bubble in 2001, the terrorist attacks of September 11 of the same year, and the second Gulf War (2002–2003). The second phase goes from 2012 to 2013 and corresponds to the uncertainty arising from the Eurozone crisis (sovereign debt crisis) and the change of leadership in China. The third phase goes from the end of 2017 to 2020. This last phase highlights the global uncertainty that resulted from the trade war led by the Trump administration against the European Union and China (2018–2019), and from the health crisis related to the COVID-19 pandemic between (2020–2021). further shows a generalized increase in uncertainty in developing countries. Uncertainty was highest in developing countries in Latin America and the Caribbean in the early 2000s. However, since the 2008 recession, uncertainty has increased more in developing countries in Europe.
3.1.3. Uncertainty and income inequality
shows a positive relationship between world uncertainty and income inequality in developing countries. In general, income inequalities increase as uncertainty increases. Uncertainty therefore appears to be a potential factor that can contribute to the increase of income inequality in developing countries and the rest of the World (Theophilopoulou, Citation2022).
However, the nature and especially the intensity of the relationship between uncertainty and income inequality varies according to the fiscal situation (fiscal deficit and fiscal surplus) of countries ().
3.1.4. Choice of variables
While the analysis of the different figures reveals that the effect of uncertainty on income inequality varies according to the geographical area of belonging and the fiscal situation of the country, for the same level of uncertainty in two countries, income inequalities (Gini coefficients) are different. This reveals that in addition to uncertainty, there are other factors (economic, social, and institutional) that could explain income inequality. Therefore, in this study, with reference to the economic literature, we consider a set of factors that may influence income inequality in developing countries.
Per capita income is the first variable considered. According to the literature, this variable can influence income inequality. However, the impact is mixed. For example, Anyanwu et al. (Citation2016) and Kebalo et al. (Citation2022) show that an increase in per capita income leads to a reduction in income inequality in developing countries, while Thye et al. (Citation2021) demonstrate that an increase in per capita income worsen income inequality. This is not surprising. If the increase in per capita income is distributed equitably across all segments of the population, it can help reduce income inequality. By contrast, if the increase in per capita income mainly benefits high-income groups, inequalities could remain stable or even increase.
The second variable is the official development assistance (ODA) received by countries (Batuo et al., Citation2022). Indeed, ODA is generally intended to support the economic, social, and human development of developing countries. By strengthening essential social services, it can reduce income inequality by reducing poverty and improving the living conditions of low-income people or vulnerable population.
The education level is the third variable. The literature shows that the improvement of the level of education or educational policies is an important driver of the reduction of income inequality (Dout & Kebalo, Citation2021; Kebalo et al., Citation2022). In fact, people with a higher level of education generally have a better chance of finding a stable and well-paid job. Education enhances their skills and employability, reducing the risk of unemployment and precarious work. summarizes variables and provides details on their definitions, measures, and sources.
3.2. Methodology
To estimate the effect of uncertainty on income inequality considering the fiscal position (fiscal deficit and fiscal surplus) of countries, we consider the following equation:
where ,
,
, and
represent respectively the income inequality, the World Uncertainty index, the vector of control variables and the error term.
and
are the parameters.
refers to countries and
to time.
and
are binary variables allowing to capture the effect of fiscal position on income inequality. From Equationequation 1
(1)
(1) ,
and
are expected to be positive. Thus, we can conclude that uncertainty increases income inequalities.
Regarding the fiscal position of countries, two cases are considered. For the case 1 on fiscal deficit, takes the value 1 at
when country
presents a fiscal deficit, and 0 otherwise. For the case 2 on fiscal surplus,
takes the value 1 at
when country
presents a fiscal surplus, and 0 otherwise.
The equation is estimated by the generalized method of moments (GMM). Indeed, the GMM is suitable when the individual dimension is superior to the temporal dimension (). Our study includes 66 developing countries and covers the period 2000–2020. Therefore,
. Moreover, the GMM makes it possible to provide solutions to problems of simultaneity bias, reverse causality and omitted variables. We use a dynamic model because the lagged dependent variable of order 1 is among the explanatory variables. In fact, the literature supports the persistence of income inequality in developing countries (Dout & Kebalo, Citation2021; Kebalo et al., Citation2022). Unlike dynamic panel GMM, standard econometric techniques such as ordinary least squares (OLS) do not make it possible to obtain efficient estimates of such a model, because of the presence of the lagged dependent variable on the right of the equation (Kinda & Thiombiano, Citation2021).
As a reminder, there are two types of GMM estimator: (i) the first difference GMM estimator and (ii) the system GMM estimator (which is preferred). Indeed, Blundell and Bond (Citation1998) show, using Monte Carlo simulations, that the system GMM estimator performs better than the first difference GMM estimator, the latter gives biased results in finite samples when the instruments are weak. The system GMM estimator combines the first difference equations with the level equations in which the variables are instrumented by their first differences (Blundell & Bond, Citation1998). The instrumentation method differs according to the nature of the explanatory variables: (a) for the purely exogenous variables, current variables are used as instruments; (b) for weakly exogenous variables, values lagged by at least one period are used as instruments; (c) for endogenous variables, values lagged by two periods and more can be used as valid instruments.
In addition to providing solutions to the problems cited above, the GMM method provides the results of Hansen’s over-identification test and the autocorrelation of errors by Arellano and Bond (Citation1991) allowing the validity of lagged variables such as instruments and the null hypothesis of no second-order autocorrelation of the errors of the difference equation. Finally, in this study, we retained the GMM estimator estimated in two steps,Footnote6 which would be more efficient than the GMM estimator estimated in one step (Roodman, Citation2009). Moreover, we base ourselves in the writing of the commands relating to our estimates on the recommendations of Roodman (Citation2009) and Newey and Windmeijer (Citation2009), including the application of the correction of Windmeijer (Citation2005), in order to obtain deviations – asymptotic types, therefore robust and eliminate, thus, the potential bias that could arise from the two-step estimation.Footnote7
4. Results
This section presents and discusses results from the estimations of baseline equation. Then, robustness tests are conducted to consolidate the different results obtained.
4.1. Overall results
The results presented in are obtained by the GMM estimator. These results are valid from both an economic and an econometric perspective. Indeed, the use of GMM requires some conditions. The outcomes of the diagnostic tests that allow for the checking of the consistency of the two-step system GMM approach are reported at the bottom of the table.
Table 2. Baseline results.
The Arellano and Bond tests are designed to assess the presence of serial autocorrelation in dynamic panel regression errors. They are based on the empirical moments of the errors and the theoretical moments expected in the case where there is no autocorrelation. The idea is to compare these moments in other to detect any significant serial correlation. These tests are important because the autocorrelation of errors can bias the parameter estimates and affect the statistical properties of the estimates. If autocorrelation is present, it may be necessary to adjust the model specification or take these correlations into account in the estimation. In practice, it is expected, for the validity of the model, a presence (respectively an absence) of the autocorrelation of order 1 (AR (1)) (respectively of order 2 (AR(2)). This is in line with the results present in which indicate that, no matter the column (1 to 2), the null hypothesis associated with the AR(1) test is rejected (p-value 0.05) and that of AR(2) cannot be rejected (p- value
0.10).
In addition, results of the Sargan’s test indicate that the instruments are valid, it means that the conditions for the validity of the instrumental moments are satisfied and that the estimates obtained using the GMM model are efficient and consistent. In other words, since the null hypothesis is not rejected (p-value 0.10), then the instruments used are exogenous and have no systematic bias. Moreover, the results of the Hansen test underline that the additional instruments are valid and are not redundant in the model. Indeed, the null hypothesis not being rejected (p-value
0.10), then the instruments added to the GMM model are not correlated with the errors of the model and provide useful information for the estimation of parameters of the model. The validity of aforementioned tests ensures the robustness of results obtained from the GMM.
Moreover, the results presented in show that no matter the estimate (1 or 2), an improvement in the lagged dependent variable of order 1 is associated, at the 1% threshold, with an increase in the level of income inequality. This result attests to the relevance of the use of a dynamic model in our study and confirm the persistent problem of income inequality often noted in developing countries (Kebalo et al., Citation2022). In addition, the results show that uncertainty increases income inequality in developing countries. Thus, a 1-point improvement in World Uncertainty is associated, at the 1% threshold, with an increase in Gini index (income inequality) by 3.163-points (column 1) and 3.231-points (column 2). Indeed, households are not similarly affected by uncertainty. Wealthy households, most of the time are more resilient than poor and vulnerable households to uncertainty shocks. During periods of uncertainty and when negative effects occur, poor, vulnerable and middle-income households have difficulty in the mobilization of resources required to mitigate the effects on their welfare. The same is not necessarily true for wealthy households. The direct consequence will be the increase in income inequality, especially when social measures such as cash transfers, subsidies for necessities, tax breaks and banking facilities for example, are not taken by policymakers in favour of poor and vulnerable households. Furthermore, the results show that developing countries with a fiscal deficit have, at the 5% threshold, a higher level of income inequality (+0.312-point) than countries without a fiscal deficit (column 1). Other results reveal that no matter the estimate (1 or 2), an improvement in per capita income (respectively education level, official development assistance) contributes, at the 1% threshold, to increasing the level of income inequality (respectively to reduce the level of income inequality). More precisely, a 1% improvement in per capita income is associated, at the 1% threshold, with an increase in Gini index by 0.039-point (column 1) and 0.015-point (column 2). A 1-point improvement in official development assistance (ODA) is associated, at the 1% threshold, with a decrease in Gini index by 0.0004-point (column 1) and 0.0003-point (column 2). Also, a 1-point improvement in tertiary education is associated, at the 1% threshold, with a decrease in Gini index by 0.0008-point (column 1) and 0.0004-point (column 2).
These results are in line with the literature. Indeed, Thye et al. (Citation2021) demonstrate that an increase in per capita income worsen income inequality, when the increase in per capita income mainly benefits high-income groups. Batuo et al. (Citation2022) show that an official development assistance, by strengthening essential social services, can help to reduce poverty and improve the living conditions of low-income people. Dout and Kebalo (Citation2021) indicate that the improvement of the level of education or educational policies is an important driver of the reduction of income inequality.
In addressing the challenge of income inequality, education and development assistance have an important role to play. Public authorities must put more emphasis on the expansion of education, educational policies, and the matching of the education system to labour market needs (Abdullah et al., Citation2015; Kebalo et al., Citation2022; Tchamyou et al., Citation2019). Indeed, when a large portion of the population is better educated, they can undertake private initiatives of wealth creation, have expectations about events that may generate more uncertainty, and then be more resilient to national and world disturbances. This could contribute to reducing the existing income gap between wealthy households and the rest of the population. In addition, actions must be carried out within the framework of strengthening the effectiveness of development assistance. Improving effectiveness means ensuring that the assistance provided to developing countries actually helps them to improve the living conditions of the poorest populations. To do this, aid must be truly targeted on the development priorities set by the countries themselves.
shows that developing countries with a fiscal surplus have, at the 1% threshold, a low level of income inequality (-0.163-point) than countries without a fiscal surplus (column 2). Moreover, the results indicate that the fiscal surplus limits the impact of uncertainty on income inequality. But more important, when the uncertainty increases by 1-point, the countries presenting a fiscal surplus have, at the 1% threshold, a low level of income inequality (-2.241-points) than countries without a fiscal surplus (column 2).
Overall, we think that our results are consistent with the realities in developing countries. Indeed, our results highlight one of the realities in developing countries. However, robustness tests are conducted to consolidate the different results obtained.
4.2. Alternative measures of uncertainty
In this section, we replace successively the World Uncertainty index by the Economic Policy Uncertainty (EPU) index and the Trade Policy Uncertainty (TPU) index in other to show that, no matter the uncertainty measure is used, fiscal surplus contributes to mitigate the positive effects of uncertainty on income inequality.
Results presented in show that, no matter the measure used (EPU index or TPU index), uncertainty contributes to increasing income inequality. Indeed, according to , a 1-point improvement in EPU index is associated, at the 1% threshold, with an increase in Gini index by 0.002-point (column 1) and 0.006-point (column 2). According to , a 1-point improvement in TPU index is associated, at the 1% threshold, with an increase in Gini index by 0.002-point (column 1) and 0.003-point (column 2). In addition, indicates that developing countries with a fiscal deficit have, at the 5% threshold, a higher level of income inequality (+0.141-point) than countries without a fiscal deficit (column 1). Moreover, the results show that the fiscal deficit exacerbate the impact of uncertainty on income inequality. In fact, when the EPU index increases by 1-point, countries presenting a fiscal deficit have, at the 1% threshold, a higher level of income inequality (+0.004-point) than countries without a fiscal deficit. Furthermore, shows that developing countries with a fiscal surplus have, at the 1% threshold, a low level of income inequality (-0.174-point) than countries without a fiscal surplus (column 2).
Table 3. Robustness test: baseline results considering economic policy uncertainty.
Table 4. Robustness test: baseline results considering trade policy uncertainty.
Also, results indicate that when the EPU index increases by 1-point, countries presenting a fiscal surplus have, at the 1% threshold, a low level of income inequality (-0.003 point) than countries without a fiscal surplus (column 2). The sign of the coefficients of the other explanatory variables are in line with theoretical expectations.
indicates that developing countries with a fiscal deficit have, at the 1% threshold, a higher level of income inequality (+0.162-point) than countries without a fiscal deficit (column 1). Moreover, the results show that when the TPU index increases by 1-point, countries presenting a fiscal deficit have, at the 1% threshold, a higher level of income inequality (+0.005-point) than countries without a fiscal deficit. Furthermore, shows that developing countries with a fiscal surplus have, at the 1% threshold, a low level of income inequality (-point) than countries without a fiscal surplus (column 2). Finally, results show that when the TPU index increases by 1-point, countries presenting a fiscal surplus have, at the 1% threshold, a low level of income inequality (
-point) than countries without a fiscal surplus (column 2). The sign of coefficients of other explanatory variables are in line with theoretical expectations.
Thus, no matter the measure of uncertainty, fiscal deficit (respectively fiscal surplus) contributes to increasing (respectively to mitigate) the positive effects of uncertainty on the income inequality.
These results are not surprising insofar as a country’s fiscal position reflects its ability to self-finance, as soon as possible, targeted expenditures to mitigate the negative socio-economic effects of uncertainty or crises. As developed above, households are not impacted in the same way by uncertainty shocks and the following economic consequences. Thus, to avoid the increase in the income gap between the wealthy and poor people, countries with fiscal surpluses take economic and social measures as soon as possible to support the poor and vulnerable households. In response to the adverse effects of uncertainty, these countries use fiscal surpluses to make social transfers to poor and vulnerable households, to subsidize prices of essential goods, and to support Small and Medium-sized Enterprises (SMEs) through tax reductions and banking facilities (guarantees) for instance.
Moreover, developing countries with fiscal deficits will be less reactive in implementing the socio-economic policies mentioned above to support poor and vulnerable households. They will be therefore tempted to adopt cyclical fiscal policies which often result in a reduction of public expenditures and/or an increase in tax rates during periods of uncertainty and crisis (Alesina et al., Citation2008; Bénassy-Quéré et al., Citation2016). This difficulty in financing the required policies would reduce the resilience of poor and vulnerable households vis-à-vis wealthy households and then will increase income inequality.
The above factors therefore explain why, despite the presence of uncertainty, developing countries with a fiscal surplus have lower income inequality than developing countries with a fiscal deficit. These interesting results reveal the important socio-economic stabilizing role that fiscal policy management plays during periods of uncertainty.
5. Conclusion and policy recommendations
Given warnings of international development institutions about a potential increase in income inequality in the World due to uncertainty arising from the COVID-19 pandemic and the Russia-Ukraine war, we have conducted an empirical investigation on the effect of uncertainty on income inequality in developing countries over the period 2000–2020. To have reliable results, the generalized method of moments was used. Results show that uncertainty, no matter the measure used, increases income inequality. In addition, the results indicate that, in the presence of uncertainty, fiscal deficit (respectively fiscal surplus) contributes to increasing (respectively to mitigate) the positive effects of uncertainty on the income inequality. These results reveal the important socio-economic stabilizing role that fiscal policy management plays during periods of uncertainty. Moreover, we find that an improvement in per capita income (respectively education level, official development assistance) contribute to increasing (respectively to reducing) the level of income inequality. From the above, it appears that:
Improving fiscal discipline in developing countries is crucial for fostering sustainable economic growth, reducing poverty, enhancing financial stability and, thereby, reducing income inequality. For that, developing countries should focus their spending on key sectors such as education, health, basic infrastructure and rural development. This can increase productivity and improve people’s living conditions, which is essential for reducing income inequality. Furthermore, developing countries must avoid excessive accumulation of public debt. It is important to maintain a sustainable level of debt to avoid the risk of financial crisis and excessive interest payments. In addition, developing countries need to develop efficient and fair tax systems to increase their internal revenues. This may include broadening the tax base, narrowing tax loopholes and improving tax collection mechanisms. Similarly, government subsidies should be precisely targeted to help vulnerable populations without wasting resources. Reforms to reduce inefficient subsidies can free up funds for other priorities. Finally, developing countries should invest in building the skills and capacities of government institutions responsible for budget management. This can lead to better planning, implementation and evaluation of fiscal policies.
Increasing the level of education in developing countries is key to reducing income inequality, by promoting economic, social and human development. In doing so, developing countries must allocate adequate resources to the education sector, increasing budgets for building schools, training teachers, purchasing teaching materials and implementing quality educational programs. In addition, countries should strive to provide equitable educational access to all, regardless of gender, ethnicity, economic status or geography. This may involve the construction of schools in rural and remote areas, as well as initiatives to encourage the schooling of girls and disadvantaged children. Furthermore, educational programs must be adapted to local and cultural needs, while including essential skills such as digital skills. Also, information and communication technologies can be used to improve access to education and to provide interactive educational resources, especially in regions where educational infrastructure is limited. Finally, developing countries must remove the cultural, social and economic barriers that prevent girls from accessing education.
Strengthening the effectiveness of official development assistance is important for maximizing the benefits of aid and fostering sustainable development in recipient countries and, therefore, reducing income inequalities. To achieve this, donors should align their aid programs with the development priorities of recipient countries. This implies better coordination with national plans and strategies. Furthermore, official development assistance (ODA) should be directed towards the achievement of specific and measurable development objectives. Projects should be designed with clear indicators to assess their impact. In addition, donors and recipient countries should be transparent in their actions and expenditures. Mutual accountability can help ensure effective use of funds. Finally, ODA-funded projects should aim to strengthen local systems, develop skills and create sustainable infrastructure, rather than simply providing temporary relief.
Disclaimer
The views expressed in this paper are those of the authors and do not necessarily reflect those of their institutions.
Acknowledgments
We thank anonymous referees for their valuable comments and suggestions. We thank Kimonique Powell, Mosuru Olukayodé Alli, and Sylvie Sanou for the proofreading service.
Disclosure statement
No potential conflict of interest was reported by the author(s).
Additional information
Notes on contributors
Léleng Kebalo
Léleng Kebalo, PhD, corresponding author. Economist at the Central Bank of West African States (BCEAO). He was a Young Professional at the World Trade Organization (WTO) when the article was written. His research interests include macroeconomics, public finance, trade and development.
Stéphane Zouri
Stéphane Zouri, PhD, works at the Central Bank of West African States (BCEAO) as an Economist. Holder of a PhD in economics from the University of Paris 1 Pantheon Sorbonne and Paris School of Economics, his research interests include macroeconomic dynamics, international trade and regional integration.
Notes
1 (i) disruption in the supply chain affecting the price of goods and services, (ii) increase in the indebtedness of countries, (iii) disruption or suspension of development programmes, (iv) reduction in investment, (v) expected increase in inequality.
2 For example, we have the Trade Policy Uncertainty index developed by Caldara et al. (Citation2020) and Handley and Limão (Citation2022), and the VIX: US financial market volatility indicator.
3 For example, we have the Economic Policy Uncertainty index developed by Baker et al. (Citation2016).
4 Policy uncertainty is the economic risk associated with undefined future government policies and regulatory frameworks. It increases the risk that economic agents will delay their spending, investment, and consumption expenditures due to market uncertainty. Therefore, it can delay the recovery of an economy during a crisis.
5 Australia, Brazil, Canada, Chile, China, Colombia, France, Germany, Greece, India, Ireland, Italy, Japan, Mexico, the Netherlands, Russia, South Korea, Spain, Sweden, Singapore, the United Kingdom, and the USA.
6 A first estimate is made under the assumption of the absence of correlation of errors and their homoscedasticity. Then, the vector of residuals resulting from this first estimation is used to convergently estimate an error variance-covariance matrix, in a second estimation step. At this second stage, the hypothesis of the absence of correlation of errors and their homoscedasticity is verified. With the STATA software, the GMM system method is pre-programmed (“xtabond2” and “twostep” commands).
7 Through the use of the “collapse” command, the Stata software guarantees a small number of instruments used which does not exceed the number of observations, in order to be able to estimate the model in an unbiased way, which avoids potentially the problem of instrument proliferation (Roodman, Citation2009).Indeed, with too many instruments, which exceeds the number of observations, the endogenous variables can be over-presented by their instruments, hence the risk of persistence of the endogeneity problem.
References
- Abdullah, A., Doucouliagos, H., & Manning, E. (2015). Does education reduce income inequality? A meta‐regression analysis. Journal of Economic Surveys, 29(2), 301–23. https://doi.org/10.1111/joes.12056
- Adjor, D. M., & Kebalo, L. (2018). Does corruption matter for unemployment in SADC countries? Review of Economic and Business Studies, 11(2), 65–92. https://doi.org/10.1515/rebs-2018-0074
- Ahir, H., Bloom, N., & Furceri, D. (2022). The world uncertainty index. Working paper No. w29763. National bureau of economic research.
- Alesina, A., Campante, F. R., & Tabellini, G. (2008). Why is fiscal policy often procyclical? Journal of the European Economic Association, 6(5), 1006–1036. https://doi.org/10.1162/JEEA.2008.6.5.1006
- Alesina, A., & Drazen, A. (1991). Why are stabilizations delayed? The American Economic Review, 81(5) , 1170–1188. https://doi.org/10.3386/w3053
- Alesina, A., & Perotti, R. (1996). Income distribution, political instability, and investment. European Economic Review, 40(6), 1203–1228. https://doi.org/10.1016/0014-2921(95)00030-5
- Ametoglo, M. E. S., Guo, P., & Wonyra, K. O. (2018). Regional integration and income inequality in ECOWAS zone. Journal of Economic Integration, 33(3), 604–627. https://doi.org/10.11130/jei.2018.33.3.604
- Anyanwu, J. C., Erhijakpor, A. E., & Obi, E. (2016). Empirical analysis of the key drivers of income inequality in West Africa. African Development Review, 28(1), 18–38. https://doi.org/10.1111/1467-8268.12164
- Arellano, M., & Bond, S. (1991). Some tests of specification for panel data: Monte Carlo evidence and an application to employment equations. The Review of Economic Studies, 58(2), 277–297. https://doi.org/10.2307/2297968
- Armelius, H., Hull, I., & Köhler, H. S. (2017). The timing of uncertainty shocks in a small open economy. Economics Letters, 155, 31–34. https://doi.org/10.1016/j.econlet.2017.03.016
- Baker, S. R., Bloom, N., & Davis, S. J. (2016). Measuring economic policy uncertainty. Quarterly Journal of Economics, 131(4), 1593–1636. https://doi.org/10.1093/qje/qjw024
- Balcilar, M., Bekiros, S., & Gupta, R. (2017). The role of news-based uncertainty indices in predicting oil markets: A hybrid nonparametric quantile causality method. Empirical Economics, 53(3), 879–889. https://doi.org/10.1007/s00181-016-1150-0
- Batuo, M. E., Kararach, G., & Malki, I. (2022). The dynamics of income inequality in Africa: An empirical investigation on the role of macroeconomic and institutional forces. World Development, 157, 105939. https://doi.org/10.1016/j.worlddev.2022.105939
- Bénassy-Quéré, A., Ragot, X., & Wolff, G. (2016). Quelle union budgétaire pour la zone euro ? Notes Du Conseil D’analyse Économique, n° 29(2), 1–12. https://doi.org/10.3917/ncae.029.0001
- Bernanke, B. S. (1983). Irreversibility, uncertainty, and cyclical investment. Quarterly Journal of Economics, 98(1), 85–106. https://doi.org/10.2307/1885568
- Bicaba, Z., Brixiová, Z., & Ncube, M. (2017). Can extreme poverty in sub-saharan Africa be eliminated by 2030? Journal of African Development, 19(2), 93–110. https://doi.org/10.5325/jafrideve.19.2.0093
- Bloom, N. (2009). The impact of uncertainty shocks. Econometrica, 77(3), 623–685. https://doi.org/10.3982/ECTA6248
- Bloom, N. (2014). Fluctuations in uncertainty. Journal of Economic Perspectives, 28(2), 153–76. https://doi.org/10.1257/jep.28.2.153
- Blundell, R., & Bond, S. (1998). Initial conditions and moment restrictions in dynamic panel data models. Journal of Econometrics, 87(1), 115–143. https://doi.org/10.1016/S0304-4076(98)00009-8
- Bonfiglioli, A., Crinò, R., & Gancia, G. (2022). Economic uncertainty and structural reforms: Evidence from stock market volatility. Quantitative Economics, 13(2), 467–504. https://doi.org/10.3982/QE1551
- Bourguignon, F. (2004). The poverty-growth-inequality triangle. New Delhi: Indian council for Research on International economic relations (ICRIER). Working Paper No 125. https://www.econstor.eu/bitstream/10419/176147/1/icrier-wp-125.pdf
- Brueckner, M., & Vespignani, J., (2017, March 28). Trade uncertainty and income inequality. CAMA Working Paper No. 24/2017. https://doi.org/10.2139/ssrn.2942479
- Caggiano, G., Castelnuovo, E., & Figueres, J. M. (2017). Economic policy uncertainty and unemployment in the United States: A nonlinear approach. Economics Letters, 151, 31–34. https://doi.org/10.1016/j.econlet.2016.12.002
- Caggiano, G., Castelnuovo, E., & Groshenny, N. (2014). Uncertainty shocks and unemployment dynamics in US recessions. Journal of Monetary Economics, 67, 78–92. https://doi.org/10.1016/j.jmoneco.2014.07.006
- Caldara, D., Iacoviello, M., Molligo, P., Prestipino, A., & Raffo, A. (2020). The economic effects of trade policy uncertainty. Journal of Monetary Economics, 109, 38–59. https://doi.org/10.1016/j.jmoneco.2019.11.002
- Christiano, L. J., Motto, R., & Rostagno, M. (2014). Risk shocks. The American Economic Review, 104(1), 27–65. https://doi.org/10.1257/aer.104.1.27
- Davis, S. J. (2016). An index of global economic policy uncertainty. National Bureau of Economic Research, NBER Working Paper No. 22740.
- Dixit, R. K., Dixit, A. K., & Pindyck, R. S. (1994). Investment under uncertainty. Princeton university press.
- Dout, H., & Kebalo, L. (2021). Trade intensity, fiscal integration and income inequality in ECOWAS. Statistics, Politics, and Policy, 12(2), 375–394. https://doi.org/10.1515/spp-2021-0008
- Fang, L., Chen, B., Yu, H., & Qian, Y. (2018). The importance of global economic policy uncertainty in predicting gold futures market volatility: A GARCH‐MIDAS approach. Journal of Futures Markets, 38(3), 413–422. https://doi.org/10.1002/fut.21897
- Fawaz, F., Rahnamamoghadam, M., & Valcarcel, V. (2012). Fluctuations, uncertainty and income inequality in developing countries. Eastern Economic Journal, 38(4), 495–511. https://doi.org/10.1057/eej.2011.32
- Fernandez, R., & Rodrik, D. (1991). Resistance to reform: Status quo bias in the presence of individual-specific uncertainty. The American Economic Review, 81(5), 1146–1155. https://www.jstor.org/stable/2006910
- Fischer, M. M., Huber, F., & Pfarrhofer, M. (2021). The regional transmission of uncertainty shocks on income inequality in the United States. Journal of Economic Behavior and Organization, 183, 887–900. https://doi.org/10.1016/j.jebo.2019.03.004
- Galbraith, J. K. (1977). The age of uncertainty. Houghton Mifflin.
- Ghirelli, C., Pérez, J. J., & Urtasun, A. (2019). A new economic policy uncertainty index for Spain. Economics Letters, 182, 64–67. https://doi.org/10.1016/j.econlet.2019.05.021
- Gilchrist, S., Sim, J. W., & Zakrajšek, E. (2014). Uncertainty, financial frictions, and investment dynamics. NBER Working Paper No w20038. National Bureau of Economic Research.
- Handley, K., & Limão, N. (2022). Trade policy uncertainty. Annual Review of Economics, 14(1), 363–395. https://doi.org/10.1146/annurev-economics-021622-020416
- Huang, Y., & Luk, P. (2020). Measuring economic policy uncertainty in China. China Economic Review, 59, 101367. https://doi.org/10.1016/j.chieco.2019.101367
- Kebalo, L., Dout, H., Couchoro, M. K., & Zouri, S. (2022). Intégration–commerciale, budgétaire, financière–régionale et inégalités de revenu dans la Communauté Economique des Etats de l’Afrique de l’Ouest (CEDEAO). African Development Review, 34(S1), S102–S116. https://doi.org/10.1111/1467-8268.12647
- Kinda, H., & Thiombiano, N. (2021). The effects of extractive industries rent on deforestation in developing countries. Resources Policy, 73, 102203. https://doi.org/10.1016/j.resourpol.2021.102203
- Knight, F. H. (1921). Risk, uncertainty and profit (Vol. 31). Houghton Mifflin.
- Newey, W. K., & Windmeijer, F. (2009). Generalized method of moments with many weak moment conditions. Econometrica, 77(3), 687–719. https://doi.org/10.3982/ECTA6224
- Obiakor, R. T., Akpa, E. O., & Okwu, A. T. (2022). Economic size, uncertainty, and income inequality in Nigeria. Journal of Economics and Allied Research, 7(2), 250–264. https://ssrn.com/abstract=4151748
- Pastor, L., & Veronesi, P. (2012). Uncertainty about government policy and stock prices. The Journal of Finance, 67(4), 1219–1264. https://doi.org/10.1111/j.1540-6261.2012.01746.x
- Pierce, J. R., & Schott, P. K. (2016). The surprisingly swift decline of US manufacturing employment. The American Economic Review, 106(7), 1632–62. https://doi.org/10.1257/aer.20131578
- Ranciire, R. G., & Tornell, A. (2016). Why do reforms occur in crises times? SSRN Electronic Journal. https://doi.org/10.2139/ssrn.2796251
- Romer, C. D. (1990). The great crash and the onset of the great depression. Quarterly Journal of Economics, 105(3), 597–624. https://doi.org/10.2307/2937892
- Roodman, D. (2009). How to do Xtabond2: An introduction to difference and system GMM in stata. The Stata Journal, 9(1), 86–136. https://doi.org/10.1177/1536867X0900900106
- Stiglitz, J. (2015). The origins of inequality, and policies to contain it. National Tax Journal, 68(2), 425–448. https://doi.org/10.17310/ntj.2015.2.09
- Tchamyou, V. S., Asongu, S. A., & Odhiambo, N. M. (2019). The role of ICT in modulating the effect of education and lifelong learning on income inequality and economic growth in Africa. African Development Review, 31(3), 261–274. https://doi.org/10.1111/1467-8268.12388
- Theophilopoulou, A. (2022). The impact of macroeconomic uncertainty on inequality: An empirical study for the United Kingdom. Journal of Money, Credit, and Banking, 54(4), 859–884. https://doi.org/10.1111/jmcb.12852
- Thye, G. L., Kumari, S. S., Lu, Y. S., & Muhairah, S. S. (2021). The impacts of external and internal uncertainties on income inequality in the ASEAN 5 countries. International Journal of Economics & Management, 15(3). http://eprints.um.edu.my/id/eprint/36063
- Tommasi, M., & Velasco, A. (1996). Where are we in the political economy of reform? Journal of Policy Reform, 1(2), 187–238. https://doi.org/10.1080/13841289608523362
- Windmeijer, F. (2005). A finite sample correction for the variance of linear efficient two-step GMM estimators. Journal of Econometrics, 126(1), 25–51. https://doi.org/10.1016/j.jeconom.2004.02.005
- Zalla, R. (2017). Economic policy uncertainty in Ireland. Atlantic Economic Journal, 45(2), 269–271. https://doi.org/10.1007/s11293-017-9536-8