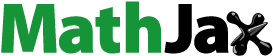
ABSTRACT
The purpose of this paper is to estimate the relationship among a primary set of economic variables, including two types of monetary aggregates: simple sum M2 and credit-card-augmented Divisia inside money services. The importance of that comparison has grown as the use of credit cards in purchase transactions has expanded. The data period includes the Great Recession, which was heavily associated with finance and thereby especially relevant to this study. The basic methodology in this paper is VAR-Sign Restrictions estimation. VAR is a well-known method to analyze inter-dependency among economic variables. By applying VAR-Sign Restrictions, we analyze how economic variables behave, positively or negatively, toward differently defined shocks. Imposing signs on the direction of economic variable responses to shocks is based on economic prior beliefs, using Bayesian estimation. Our results provide deeper insights into the relative merits of the two types of monetary aggregates as indicators.
1. Introduction
The purpose of this paper is to estimate the relationship among a set of primary economic variables, including two types of monetary aggregates: simple sum M2 and credit-card-augmented Divisia monetary aggregate M2 inside money. Since credit card transactions are becoming a main driving force for deferred payment monetary services, we are focusing on a credit-card-augmented Divisia monetary aggregate (hereafter often called credit-augmented Divisia), including credit card transaction volumes as a deferred payment liquidity service. The data period includes the Great Recession, which is attributed to the collapse of the credit sector. Therefore, we expect the use of credit-augmented Divisia to provide new economic insights into that period.
The basic methodology in this paper is VAR sign-restrictions estimation (Uhlig, Citation2005). VAR is a powerful and intuitive method to analyze inter-dependency among economic variables. By applying VAR sign restrictions, we analyze how economic variables behave (positively or negatively) toward differently defined shocks. Imposing signs to the direction of economic variables is based on economic prior belief. For example, we empirically accept that if the Federal Reserve implements contractionary monetary policy, then output, price, and money supply will be decreasing. Therefore, we can assign a negative sign to the change in those variables. In the same manner, we can impose several types of shocks on the variables and determine the variables’ directions based on empirical belief and the data. The use of Bayesian estimation helps to build the model structure. We expect that imposing prior beliefs toward various shocks can help to clarify the behavior of credit-augmented Divisia relative to the corresponding accounting simple sum monetary aggregates. Using VAR sign restrictions and a sample period focused on the Great Recession is intended to provide deeper insights into the indicator merits of the credit-augmented Divisia than available from prior empirical studies of those new aggregates.
Sometimes a mismatch in data frequency can be an obstacle to economic analysis. Most of the data used in this study are from the St. Louis Federal Reserve Bank’s FRED database. The credit-augmented Divisia are published monthly by the Center for Financial Stability (CFS) in New York City. Among the data, real GDP data are available officially only quarterly from FRED and the Commerce Department, while the other data are available monthly. There are many approaches to resolve this mismatch in data frequencies. We could adopt monthly GDP from private sources, such as IHS-Markit. Alternatively, we can internalize this problem into the model by adopting a mixed-data sampling approach (MIDAS, Andreou et al. (Citation2010), Ghysels (Citation2016)) or a mixed-frequency VAR (MFVAR, Scorfheide and Song (Citation2015)). In this paper, we build monthly frequency real GDP data by using mixed-frequency VAR.
This paper includes a primary contribution. In the current situation where credit utilization has been increasing, credit-augmented Divisia can be a good tool to meet the needs of economic analysis requiring the consideration of credit factors. This paper is the first study to justify this argument by using Bayesian VAR sign restrictions. We have three key findings: first, by using credit-augmented Divisia as a measure of monetary services, instead of the traditional simple sum monetary aggregate, we can expect a smaller value of mean squared forecasting error (MSFE) for monthly frequency real GDP built by Mixed-Frequency VAR backcasting. Second, the empirical results of impulse response to the credit supply shock show that the liquidity services provided by the credit transaction and the simple sum monetary aggregate have a substitute relationship. Third, the empirical results of historical decomposition show a stronger co-movement between the historical behavior of credit-augmented Divisia and credit supply shocks than co-movement between simple sum money and credit supply shocks.
The structure of this paper is as follows: Section 2 introduces Divisia monetary aggregates, Section 3 introduces VAR-sign-restrictions estimation, and Section 4 discusses the result. We conclude in Section 5.
2. Divisia monetary aggregation
2.1. The conventional divisia monetary aggregates
Barnett (Citation1978, Citation1980) derived the user-cost price formula of monetary services, needed to apply index number and aggregation theory to monetary aggregation, and proposed use of the discrete-time Divisia index to measure demand-side monetary services. While the simple sum accounting aggregates, published by the Federal Reserve, impute the same weight to each component monetary asset, Divisia weights the growth rates of the services of each monetary asset in accordance with the methodology of microeconomic aggregation and index number theory.
Many previous empirical publications have found that Divisia monetary aggregates are superior to the simple sum. For example, by using VAR estimation, Schunk (Citation2001) found that forecasts of U.S. real GDP are most accurate when a Divisia monetary aggregate is included. Serletis (Citation2009) found that estimation of autoregressive causality models with Divisia monetary aggregates is useful in anticipating future movements in macroeconomic activity. Using simple sum and Divisia monetary aggregates, Serletis and Rahman (Citation2013) investigate the relationship between money growth uncertainty and the level of economic activity in the United States in a New Keynesian context. By using bivariate VARMA, GARCH-in-mean, and asymmetric BEKK models, Serletis and Rahman (Citation2013) find that increased Divisia money growth volatility is associated with lower average growth rate of real economic activity and conclude that targeting Divisia monetary aggregate growth rates would contribute to higher overall economic growth. Serletis and Gogas (Citation2014) re-visit a cointegration test in the spirit of King et al. (Citation1991) by using Divisia monetary aggregates. The test finds that using simple sum monetary aggregates induces incoherence, as emphasized in the Barnett Critique defined by Chrystal and MacDonald (Citation1994). To resolve the Barnett critique problem, internally consistent nesting of aggregation theoretic monetary aggregator functions within integrable money demand systems was advocated and implemented by Barnett (Citation1983). Reprints of many published journal articles providing theoretical and empirical results with the Divisia monetary aggregates are collected together in the books, Barnett and Serletis (Citation2000) and Barnett and Chauvet (Citation2011).
As derived by Barnett (Citation1978, Citation1980), the real user cost (equivalent rental price) of a monetary asset among such assets is
where is the own rate of return on asset
during period
, and
is the “benchmark” rate of return on pure capital during period
. That benchmark rate is the risk-free rate of return on a completely illiquid asset. The growth rate of the Divisia quantity index in continuous time is
where is the expenditure share on monetary asset
and
is real balances of monetary asset
during period
, with
and
.
2.2. Credit-card-augmented divisia monetary aggregates
Credit-card-augmented Divisia monetary aggregates measure money services, including credit-card deferred-payment transaction services. Barnett et al. (Citation2023) and Barnett and Su (Citation2016, Citation2018, Citation2019) introduce this state-of-the-art monetary aggregate. These new Divisia aggregates are provided to the public by the Center for Financial Services (CFS) along with the original Divisia monetary aggregates, which do not include credit card transaction services. Although credit cards provide transaction services, those increasingly important services had never before been included in measures of money services. The reason is that the simple sum, based upon the traditional accounting conventions, does not allow adding liabilities to assets. But by using economic theory instead of accounting, aggregation over services does not depend upon whether the source of the service flow is an asset or a liability. Credit cards provide deferred payment services not available from monetary assets. The theory permits measurement of the joint services of credit cards and money in accordance with aggregation and index number theory. In general, economic index number theory measures service flows and is based on microeconomic aggregation theory, not accounting conventions.
By using a multivariate state space model, Barnett et al. (Citation2023) found that nowcasting with credit-augmented Divisia yields substantially smaller mean squared error than with the original Divisia monetary aggregates, which do not include credit card services. Dery and Serletis (Citation2019) show that the inference abilities of the Divisia monetary aggregates is strongest, when the broad Divisia monetary aggregates are used. Using Hamilton’s filter and Granger causality tests, Liu et al. (Citation2020) investigate the cyclical properties of the credit-augmented Divisia and find that both the narrow and broad credit-augmented Divisia are superior to the original Divisia monetary aggregates. By using an autoregressive distributed lag (ADL) model and Bayesian VAR, Barnett and Park (Citation2022) found that credit-augmented Divisia is the better indicator for forecasting inflation and output. Further relevant empirical research includes Liu and Serletis (Citation2020).
2.2.1. Demand side formula
The real user cost of credit cards services, derived in Barnett and Su (Citation2016), is
where is the benchmark rate of return on pure capital during period
, and
is the interest rate charged on credit card
balances.Footnote1 There are two categories of consumers using credit cards. The first category is consumers who maintain rotating balances and thereby pay interest to the credit cards company both on rotating balances and on credit card volumes on new purchases. The other category is consumers who do not pay interest, since they always repay their credit card debt before interest becomes due. Assuming the existence of a representative consumer aggregated over consumers, the credit card interest rate,
, is averaged over both those consumers who maintain rotating balances, while thereby paying interest on contemporaneous credit card purchases, and those consumers who pay off such credit card transactions before the end of the period, while thereby not paying explicit interest on the credit card transactions. The representative consumer’s utility function for the current period does not include rotating balances for transactions in prior periods, to avoid double counting of transactions services. The CFS data include credit card transaction services, measured by their current period reported “volumes,” for the four
credit cards companies, Visa, MasterCard, Discover, and American Express.
The credit-augmented Divisia aggregates are a function of monetary asset services and current period credit card deferred payment services. The aggregation-theoretic approach produces the credit-card-augmented aggregator function, , derived from optimizing behavior of consumers, where
is the vector of transaction volumes on the representative consumer’s credit cards from Visa, MasterCard, Discover, and American Express. The growth rate of the Divisia index,
, is the share-weighted average of the growth rates of the components. The credit card quantities included in the augmented Divisia index formula are the monthly credit card transaction volumes, not the credit cards balances, which also include rotating balances from previous period transactions.
The credit-augmented Divisia index, which exactly tracks the aggregator function in continuous time, is the following:
The share, , is the expenditure share of monetary asset
in the total services of monetary assets and credit cards, while the share,
, is the expenditure share of credit card
services in the total services of monetary assets and credit cards. In the computation of
, the relevant user cost price in the numerator of the share is
, while in the computation of
, the relevant user cost price in the numerator of the share is
. The user cost prices in the denominators of the shares include both the user costs of monetary asset services and of credit card volume services.
2.2.2. Supply side
While demand-side economic aggregation theory is based on the microeconomic theory of rational consumer behavior, supply-side economic aggregation theory is based on rational firm behavior. Barnett and Su (Citation2018) derived supply-side monetary aggregation theory for banks, supplying monetary account services along with credit card deferred payment services. The theory is also relevant to shadow banking production of such services.
The existence of non-interest-bearing required reserves imposes an implicit tax on banks. This tax has existed during most of US Federal Reserve history, although currently required reserves are set at zero in the United States. Barnett (Citation1987) derived the user cost price received by banks for servicing monetary asset type to be
where is the required reserve ratio on monetary asset
. The implicit tax on banks is the foregone interest on un-invested required reserves.
3. Empirical studies
3.1. Basic VAR estimation
The general reduced form VAR model (Sims, Citation1980) is:
where is the number of lags and
is assumed to be serially uncorrelated with covariance matrix, E
. The reduced form VAR disturbances are related to the underlying economic shocks,
, by
where has the identity matrix as its covariance matrix, and
is lower triangular. Substituting equation (7) into the VAR equation (6) and multiplying both sides by
, we get:
Now we have the structural VAR model and can uniquely determine the relationship by using Cholesky decomposition.
The order of the variables becomes important when we are imposing zero restrictions with Cholesky decomposition. There is no fixed rule for the variable ordering, but conventionally the most exogenous variable is in the first row of the vector , and the least exogenous variable is in the last row of the vector. However, since the main purpose of this paper is to estimate interdependencies among the endogenous variables by using sign restrictions, this method is relatively free from the conventional ordering rules.
3.2. Build monthly real GDP by using mixed-frequency VAR
A mismatch in data frequency could be an obstacle to economic analysis. For example, FRED and the Department of Commerce provide GDP data only with quarterly frequency. However, the CFS provides Divisia monetary data with monthly frequency. Other variables used in this study (price, policy rate, spread, and credit) are provided by FRED with monthly frequency. This frequency mismatch can be a critical problem for VAR estimation, but there are several solutions to unify the frequency of data. In this paper, we adopt mixed-frequency VAR.
Since Zadrozny and Chambers (Citation1988) proposed mixed-frequency VAR, many publications have supported that approach. Scorfheide and Song (Citation2015) and Scorfheide and Song (Citation2023) provide a methodology for real-time forecasting under mixed-frequency VAR. Kuzin and Schumacher (Kuzin et al., Citation2011) compare the performance in forecasting Euro area GDP growth by MIDAS (mixed-data sampling) and mixed-frequency VAR.
We employ data representing each economic sector. We use quarterly real GDP, and the monthly variables, industrial production, consumption expenditure, CPI, capacity utilization, shadow federal fund rate, unemployment rate, and credit-augmented Divisia M2 inside money (M2I), as endogenous variables. There are several well-known works that support the performance of M2 in economic analysis. Hallman et al. (Citation1991), by modeling the mean-reverting series, provide empirical evidence that M2 and its velocity have a long-run link with the price level. Assuming M2 is controllable, Dotsey and Otrok (Citation1994) found that the use of M2 as an intermediate target of monetary policy could reduce the variance of nominal GDP. In addition and most relevantly, simple sum M2 level is the broadest monetary aggregate that is officially available from Federal Reserve. For direct comparison with the simple sum monetary aggregate, we chose the broadest available monetary aggregate, M2.
It is not our intent or belief to advocate Divisia M2 or credit-card augmented Divisia M2 as preferable to the broader Divisia aggregates available from the CFS, but the CFS does not provide broader simple sum monetary aggregates. Inferiority of simple sum M3 and M4 is well known and hence not available either from the Federal Reserve or from the CFS. For the CFS to provide those inferior broader simple sum monetary aggregates, the CFS would need the ability to consolidate the components to avoid double counting. The CFS has neither the available data nor the motivation to provide consolidated simple sum M3 or M4. But consolidation is not relevant to Divisia aggregation, which measures service flows, not accounting stocks. As a result, it is no longer possible to compare Divisia M3 or Divisia M4 with simple sum M3 or simple sum M4.
Finally, we choose inside money because in our sign-restriction model, we introduced a credit supply shock measured by the lending rate. On the supply side, the private banking sector provides the monetary-services value added to the economy as inside money.
After the Great Recession, the economy experienced a zero-lower bound interest rate for almost 6 years. As a result, we use the shadow federal fund rate as an alternative to the policy rate. The shadow federal fund rate has been found to provide accurate results for the monetary policy stance (Wu & Xia, Citation2016). Information on the data sources is provided in in Appendix A. Since this study focuses on the post-Great Recession episode, we exclude the more recent COVID-19 pandemic era. Our data period begins in July 2006 and ends in December 2019.
Meanwhile, Barnett et al. (Citation2023) employ a mixed frequency dynamic factor model to “nowcast” nominal GDP. Nowcasting methodology in economics permits prediction of the present, the very near future, and the very recent past state of an economic indicator, when not available at the frequency needed for direct measurement. In general, nowcasting takes into account the time lag of data release. For example, GDP is released with relatively long delays and only with quarterly frequency. Barnett et al. (Citation2023) find that the inclusion of the credit-augmented Divisia in their nowcasting model yields substantially smaller mean squared errors than inclusion of the traditional Divisia monetary aggregate excluding credit card services.
Nowcasting primarily aims to predict the near future behavior of variables by using a state-space model. “Backcasting” aims to estimate the behavior of past unobserved components by using the same model with Kalman smoothing. The mixed-frequency VAR allows backcasting variables that have an arbitrary pattern of missing observations. We use the method of constructing monthly real GDP data with the VAR model from Scorfheide and Song (Citation2015).Footnote2 By using the Gibbs sampler, we generate a draw from the posterior distribution of the reduced VAR parameters, conditional on observables and states. By using this draw and the Kalman smoother method, we estimate the unobserved states and thus implicitly recover the values of the missing observations.
By this procedure, we acquire the backcast monthly real GDP data. illustrates the behavior of monthly real GDP and the growth rate of monthly real GDP. We observe the big drop in real GDP in the Great Recession period in both graphs. The right figure shows that the average growth rate of quarterly real GDP is approximately 0.5%. In Appendix B, we iterate the same procedure, but with credit-augmented Divisia M2I replaced by simple sum M2. displays the result.
Figure 1. Log of real GDP (left) and growth rate of real GDP (right) with mixed-frequency VAR using credit-augmented divisia.

By estimating the missing observations, we obtain two datasets with each having 162 observations on 8 variables. The first dataset includes credit-augmented Divisia M2I and the other includes simple sum M2. As an initial comparison of the two, we conduct VAR forecasting. shows the unconditional forecasts of each variable using Minnesota priors. We use the algorithm introduced by Del Negro and Scorfheide (Citation2013) for Bayesian VAR forecasting. plots the forecasts of the year-over-year growth rates of key variables. However, the plots of the shadow federal fund rate and the unemployment rate forecasts are of levels, not year-over-year growth rates. The forecast starts are for the 10 periods from March 2019 to December 2019. In each figure, the thick-dark blue line is the actual data flow, while the thin-red line is the forecasted value. We also display the 68% and 90% highest credible posterior bands.
in the Appendix displays the results with the same procedure as , but using simple sum M2 instead of credit-augmented Divisia M2I. The behavior of forecasting is somewhat similar in each case, but the mean squared forecasting error (MSFE) for the growth rate of real GDP reveals substantial differences. With credit-augmented Divisia M2I, the MSFE of the forecasted growth rate of real GDP is 0.2994, while with simple sum M2 that MSFE is 0.3700. We find that credit-augmented Divisia M2I is a better indicator in forecasting real GDP growth.
3.3. Sign restrictions
VAR-sign restricted estimation (Uhlig, Citation2005) is the methodology adopted in this paper to produce deeper insights into the relative merits of the two monetary indicators. VAR is a widely used approach to investigate inter-dependency among economic variables. By applying sign restrictions, we can more deeply analyze how economic variables respond towards differently defined shocks. The Bayesian procedure assesses prior economic beliefs on plus/minus signs about the direction of changes in economic variables in response to shocks.
There are advantages of sign restrictions estimation. An and Wang (Citation2012) identify several advantages to the sign restrictions approach. First, in the traditional structural VAR model, sign restrictions from conventional views are often used implicitly as criteria to check the validity of identifying assumptions. In the sign restrictions approach, those restrictions are made more explicit by being imposed directly on impulse responses. Second, in estimating impulse responses, the sign restrictions approach takes into account both data and identification uncertainty by simulation. Third, sign restrictions are weak prior information, since they do not lead to exact identifications of the reduced form VAR. This advantage is particularly important, because it circumvents excessively strong zero restrictions on the contemporaneous and long-run impacts of shocks. Finally, the sign restrictions method uses the Bayesian Monte Carlo procedure, which, according to Sims (Citation1988), does not require differencing. Thus, the sign restrictions approach can avoid many of the subtle specification issues for observationally equivalent trend and difference stationary variables in VAR.
We use the sign restrictions methodology developed by Rubio-Ramirez et al. (Citation2010), which involves QR decomposition. The algorithm is as follows:
Take a random draw
.
Compute the lower-triangular Cholesky decomposition
.
For
consider
random draws of the rotation matrix
. For each
combination , compute the set of implied structural impulse responses
.
(4) If
satisfies prior beliefs, store its value. Otherwise discard it.
(5) Repeat this
times.
Here is the vectorized coefficient matrix, and
is the orthonormal matrix satisfying
, where the randomness and the signs are imposed in this matrix. The algorithm assumes
follows the inverse-Wishart prior distribution.
We keep the median value of the impulse responses for each replication of this algorithm. The prior beliefs on the sign of response-changes play an important role in this procedure.
3.4. Data sources and transformations
The monthly frequency variables in the model are often related to credit. The source of monthly GDP data is described in Section 3.2. We also use data on CPI, shadow federal funds rate, credit-augmented Divisia M2I, total consumer credit, and spread. To overcome the intrinsic problems of the effective federal funds rate at the lower bound, we adopt the shadow federal funds rate. Following Mian et al. (Citation2017), we normalize total consumer credit by real GDP to reflect the growth in debt relative to the size of the economy. Conventionally, the difference between the Baa bond rate and the 10-year Treasury bill is a measure of “spread.” However, we adopt Littlejohn (Citation2020)’s definition of spread as the difference between the bank prime loan rate and the 3-month Treasury Bill, as a measure of credit price, reflecting willingness to bear private sector prime rate risk. Banks use that measure as a benchmark for charging households or firms. The level data are converted to the logarithm form. AIC and BIC criteria both conclude that the optimal lag length is 4 with constant. The data sources are described in in Appendix A.
We introduce four types of shocks in the model. We impose demand, supply, monetary policy, and credit supply shocks on endogenous variables. Most of them are universally utilized in sign-restriction literature. Our sign restrictions estimation follows the Matlab toolbox developed in 2020 by Ambrogio Cesa-Bianchi.Footnote3
3.5. Identification
The restrictions are assumed to be imposed contemporaneously on impact for one period. A widely held prior belief is that a contractionary monetary policy shock induces a decrease in price and real output and an increase in the federal funds rate (Uhlig, Citation2005). The convention is to call such a contractionary monetary policy shock a “positive” monetary policy shock. We can apply similar conventional prior beliefs to different types of shocks. explains how different types of shocks are expected to affect the direction of endogenous variables. All types of shocks are assumed to be implemented positively.
Table 1. Sign restrictions with use of credit-augmented divisia M2I.
For a positive demand shock, we would expect real GDP, price level, shadow federal funds rate, and any monetary aggregate to move in the same direction. For a positive supply shock, we would expect real GDP and the price level to move in the opposite directions. Following Littlejohn (Citation2020), a credit supply shock is typically measured by the lending rate or by the spread relative to the risk-free government rate. A positive credit supply shock is assumed to move in the same direction as credit-augmented Divisia M2I, which includes credit card transaction service volume. Some cells are left blank when the effects of the shocks are ambiguous.
We repeat this process by switching the monetary aggregate, credit-augmented Divisia M2I, to be simple sum M2. explains the characteristic of the simple sum M2. For example, when a contractionary monetary policy shock is implemented, the conventional simple sum M2 is expected to decrease, while there is no prior expectation regarding the response of credit-augmented Divisia M2I. The effect of a credit supply shock on simple sum M2 is ambiguous, since it depends on the relationship between credit transactions and the traditional monetary aggregate.
4. Results
Initially we focus on the impulse responses of the variables to shocks. The impulse responses are used to describe how the economy reacts over time to exogenous impulses. Since we draw 1,000 values of the matrix, we observe 1,000 impulse responses. displays the 1,000 impulse responses. Among them, we display the median value of impulse responses at each period.
Figure 3. 1,000-rotations of impulse responses to demand shock (upper-left), supply shock (upper-right), monetary policy shock (down-left), credit supply shock (down-right) with data including credit-augmented divisia M2I.

4.1. Impulse response
The solid dark-blue line in is the median impulse responses to each shock with a 95% credible interval from the 1,000 draws. The upper left panel shows the impulse response to the demand shock. The impulse responses of real GDP, Consumer Price Index (CPI), and prime spread show the fluctuation from the beginning of the period. The impulse response of the shadow federal funds rate has a humped shape, increasing to 0.1%, and then converging to the origin. The credit ratio experiences major change. It declines to −0.05% in the short term, but the duration of its negative effect lasts only 1 year. It shows a positive effect in the long run. This shock causes significant increases in credit-augmented Divisia M2I, with the effect not dissipating in the long run. The upper-right panel shows the impulse responses to the supply shock. Most of the impulse responses converge to 0 with the responses being statistically insignificant. The impulse response of real GDP experiences fluctuation in the early 3 years.
Figure 4. Impulse responses to demand shock (upper-left), supply shock (upper-right), monetary policy shock (down-left), and credit supply shock (down-right) with data set including credit-augmented divisia M2I.

The down-left panel shows the impulse responses to the monetary policy shock. Interpreting the impulse responses to monetary policy shocks is a main task of our VAR research. The price level declines to −0.2% and then converges to the origin, while showing statistical significance during a very short period. The shadow federal funds rate has a humped shape reaching its peak while increasing by more than 0.1%. Their behaviors are similar to the results of Uhlig (Citation2005). The down-right panel displays the impulse response to the credit supply shock. Injecting the credit volume into the economy clearly bolsters the economy through the credit transmission channel. Real GDP increases to 0.15% before it converges to a positive level. The responses of credit-augmented Divisia M2I show positive but statistically insignificant behavior. Other variables converge to 0 in the long run after experiencing fluctuations.
We calculate an additional set of impulse responses by replacing credit-augmented Divisia M2I by simple sum M2. in Appendix A explains the response signs imposed. The results are displayed in in Appendix B. In a broad sense, the behavior of impulse responses with the two different monetary aggregates is similar. However, some differences are observed. First, the contractionary monetary policy shock induces decrease in credit-augmented Divisia M2I, reaching its lowest point of −0.2% and converging to −0.1% in the long run. While the effect of positive monetary policy shock on simple sum M2 shows a similar behavior, the magnitude differs. It reaches the lowest point of −0.3% and converges to −0.2% in the long run. In addition, while the impulse response of credit-augmented Divisia M2I to monetary policy shocks is statistically insignificant, the response of simple sum M2 to monetary policy shocks is statistically significant.
Meanwhile, the impulse responses of credit ratio to monetary policy shocks in the two different data sets show similar behavior. However, we can track meaningful differences in statistical significance. In , the credit ratio decreases initially and converges to a negative value in the long run. But it shows statistical significance only in the short time period (8 to 22 period). In , the credit ratio shows similar behavior to . However, it is statistically significant in the long run.
Second, we focus on the noticeably different behavior of the variables to credit supply shocks. In (down-right), the real GDP increases to 0.15% and converges to a positive value in the long run, which is consistent with the prior belief. The result shows statistical significance for the first 15 periods. Meanwhile, the real GDP in (down-right) in Appendix B shows different movement. The real GDP increases to 0.1% and converges to a negative value in the long run. That result is not consistent with the prior belief. Except for the short initial six periods, the result is statistically insignificant. The impulse responses of credit ratio to the credit supply shock in and in show similar behaviors. However, the response converges to a positive value in , whereas it converges to a negative value in Considering the prior belief that we imposed on this model, the data including credit-Divisia M2I show more reliable results. In (down-right), we observe that the credit supply shock has a positive effect on the response of credit-augmented Divisia M2I, as is consistent with the imposed prior belief. After the shock, credit-augmented Divisia increases by 0.1% instantly, and after experiencing moderate fluctuation it converges to near-0 value. On the other hand, in (down-right), we find that the credit supply shock has a negative effect on the response of simple sum M2, even though we do not impose any prior belief. After the shock, simple sum M2 declines to −0.2% and converges to −0.1% in the long run.
We reach an important conclusion. For the same credit supply shock, simple sum money shows negative behavior and credit-augmented Divisia M2I shows positive behavior respectively. It therefore appears that the positive effect of the liquidity services provided by credit transactions offsets and dominates the negative effect of the simple sum money. We observe a potential substitution relationship between money and credit. A more formal analysis of Hicks-Allen substitutability in consumption could be the basis of future research requiring estimation of a system of consumer demand equations, while formal analysis of substitutability in production would require estimation of a system of bank output supply functions. That potential future research would best be accomplished using a conventional Divisia monetary aggregate and a Divisia index aggregating only over credit card services, rather than a simple sum monetary aggregate and a Divisia index aggregating jointly over monetary services and credit card services.
4.2. Limitations of sign restrictions
Fry and Pagan (Citation2011) and Kilian and Murphy (Citation2012) reveal limitations of sign restrictions. The response functions collected from the posterior median responses for each horizon are a composite of different structural response functions. Furthermore, the median for the forecasting error variance decomposition and the historical decomposition are difficult to interpret. They suggest using sign restrictions with zero restriction and Median Target (MT) method. Recently, Wolf (Citation2020) argues in the weak sign restrictions framework, that it is ambiguous to distinguish the effects on output of positive demand and supply shock and negative monetary policy shock. He concludes that the sign restrictions are vulnerable to expansionary demand and supply shocks “masquerading” as contractionary monetary policy shocks. He finds that the algorithm constructed by Haar prior tends to mis-characterize the sign of the aggregate output response resulting from the relatively low volatility of the policy shock.
To solve problems inherent in sign restrictions, we follow the second solution suggested by Fry and Pagan (Citation2011). They suggest selecting a single value of close to the median value of
, called
. In our model, among 20 times repeated 1,000 draws, we select the 14th trial, 108th
matrix as the “Median Target (MT)” closest to the
matrix. In and Appendix , we plot red-dashed lines for the median-target impulse responses from 1,000-times of the rotations (). In most of the cases, the median impulse responses and median-target impulse responses share common behavior with very small differences. By choosing single-valued
, we calculate the forecasting error variance decomposition and historical decomposition.
Using credit-augmented Divisia and simple sum M2 respectively, and Appendix explain the forecast error variance decomposition (FEVD) for each endogenous variable during the Great Recession and immediate post-Great Recession periods, including the zero-lower bound policy implementation. By comparing relative magnitudes, we can verify which shocks contributed more to the unexpected fluctuation of the endogenous variables. For all variables in this VAR model, we analyze forecast error variance and its contribution to structural disturbance terms as percentages summing to 1. The results are based on the median target and 1, 4, 12, 36, and 72-month horizons.
Table 2. Median target forecast error variance decomposition, credit-augmented divisia M2I.
During the output fluctuation in , supply and monetary policy shocks are leading factors in the initial period. Both shocks share more than 40% of output fluctuation during the whole period. Demand and credit supply shocks are not the main factors of output fluctuation. However, the contribution of demand and credit supply shock is gradually increasing and share more than 13% of each of the contributions. For the fluctuation of price, the supply shock is the main contributor in the initial period, but its influence is diminishing. Demand shock is also notable contributor to the fluctuation of prices. Its contribution remains nearly 30% in the long run. The contribution of the monetary policy shock is very small during initial periods but after one-year jumps to 20%. For the fluctuation of the policy rate, considered to be the shadow federal funds rate, the demand and monetary policy shocks are the main driving forces of its early period fluctuation. However, the effect of supply shock is gradually increasing.
Monetary policy and credit supply shocks act as the main contributors to the fluctuations of the variables in the financial category: spread, credit ratio, and credit-augmented Divisia M2I. Monetary policy shocks provide a moderate contribution to the fluctuation of spread and credit ratio during the entire period. The credit supply shock is the main factor in the fluctuation of credit-augmented Divisia M2I, but its effect is decreasing rapidly. In the long run, the fluctuation of credit-augmented Divisia M2I is dominated by the monetary policy shock.
in the Appendix displays the result of forecast error variance decomposition by replacing monetary aggregate data with simple sum M2. For the real GDP fluctuation, it is a common finding for both data sets that the supply and monetary shocks are the main driving forces. However, they explain more than 50% of the fluctuations in the long run, greater than the result of . The price fluctuation is initially influenced by the supply shock. However, its impact is gradually diminishing. Compared to , the monetary policy shock contributes more to the price fluctuation. The demand shock shares the stable portion. The monetary policy shock is the sole main driving force for the fluctuation of policy rate. However, its status as a main contributor is replaced by the supply shock in the long run. The effect of the demand shock is decreasing.
It is a common result shared by both data sets that the credit supply shock provides a major contribution to the fluctuations of the financial sector variables. The fluctuation of spread can be explained by the monetary and credit supply shocks during the entire time period. The initial fluctuation of credit ratio is mainly influenced by the credit supply shock. However, the portion of the monetary policy shock is increasing, and it becomes a main contributor to the fluctuation in the long run. For the fluctuation of the simple sum aggregate, the credit supply shock is a main contributor during the whole period, while the monetary policy shock declines in importance over time.
In general, the monetary policy shock is the main contributor to the fluctuation of each variable. Littlejohn (Citation2020) also finds that monetary policy acts as the leading contributor to the fluctuation of each variable, but in our study the contribution of the monetary policy shock is noticeably larger than in his work.
and the Appendix report the historical decomposition of real GDP, CPI, and credit-augmented Divisia M2I or simple sum M2 respectively. Those figures measure the contribution of uncertainty shocks to the variables from their respective baseline projection and thereby quantify how much a given structural shock explains the historically observed fluctuations in the VAR variables. We identify the blue shaded area as the Great Recession and the gray shaded area as the three-time “Quantitative Easing.” In the left panels of , the height of each bar stands for the magnitude of each shock towards the fluctuation of endogenous variables, where blue designates the demand shock, red the supply shock, yellow the monetary policy shock, purple the credit supply shock, and green the residual.
By connecting historic events and the behavior of each structural shock, we can track the economic implication during a period. The right panels of display the decomposition of the stacked shocks. The first row of each right panel shows the quarter-to-quarter growth rate of real GDP, CPI, and credit-augmented Divisia. The other rows provide the cumulative effect of a demand shock, supply shock, monetary policy shock, credit supply shock, and residual. The common patterns would be captured between the growth rate of the endogenous variables and individual shocks.Footnote4
The first-row panels discuss the stacked and individual line-form historical decomposition of real GDP, on the left and right sides respectively. We observe the major change in the overlapped period of the Great Recession and the first quantitative easing. The historical decompositions of price and credit-augmented Divisia M2I also show this change. According to the individual line graph, the big drop in the quarter-to-quarter growth rate of real GDP was mainly attributed to the drop in supply and monetary policy shocks. That conclusion is consistent with the result from the forecasting error variance decomposition.
The second-row panels of show the historical decomposition of price. From the right panel, we find the historical behavior of the growth rate of price is roughly consistent with the cumulative effect of a flow supply shock. The first row of shows this result in detail. The left panel of is induced from the data set including credit-augmented Divisia M2I. The dark blue solid line shows the growth rate of price, and the red dashed line shows the cumulative effect of a flow supply shock. The graphs describe the co-movements between the growth rate of the price level and supply shock. The right panel of is from the data set including simple sum M2. After the three periods of quantitative easing, the price and the supply shock share a similar pattern in both panels. However, the left graph, using the data set including credit-augmented Divisia M2I, displays the stronger co-movement in detail.
Figure 6. The first row displays behavior of supply shock and growth of CPI, with credit-augmented divisia M2I on the left and simple sum M2 on the right. The second row displays the behavior of credit supply shock and growth of monetary aggregates, with credit-augmented divisia M2I on the left and simple sum M2 on the right.

The third-row panels of and the Appendix relate to the historical decomposition of credit-augmented Divisia M2I and simple sum M2, respectively. We also track the major change during the Great Recession and the first two-quantitative easing periods. From the right panel of , we focus on the cumulative effect of a flow credit supply shock and the behavior of credit-augmented Divisia M2I. The second row of displays the details: the dark blue solid line is the growth rate of each monetary variable, with credit-augmented Divisia M2I on the left and simple sum M2 on the right, while the red dashed line shows a cumulative effect of a flow credit supply shock. Observe that credit supply shock is a better indicator for capturing the behavior of credit-augmented Divisia M2I growth than of simple sum M2 growth. Comparing these two graphs in the second row in , we find stronger co-movements between the historic growth of credit-augmented Divisia M2I and credit supply shock.
5. Conclusion
The purpose of this paper is to investigate the relationship among primary economic variables and two monetary aggregates during the Great Recession period with particular emphasis on the role of credit. The two monetary aggregates are the state-of-the-art credit-augmented Divisia M2 aggregate, based on microeconomic aggregation and index number theory, and the widely used accounting based simple sum M2 aggregate. The Great Recession period is especially relevant to the comparison because of the relevance of credit and the credit crisis during that period. By using the back-casting method, we calculate monthly real GDP to synchronize the frequency of the endogenous variables. We use VAR sign-restrictions estimation to provide deeper insights than available from prior empirical methodologies used to investigate the credit-augmented Divisia.
In using VAR sign-restrictions estimation, we impose plus/minus signs on the direction of the response of variables to various shocks, based on prior economic belief. The use of that prior information is by Bayesian methodology. The result of the impulse responses, variance decomposition, and historical decomposition shed light on the role of credit-related variables including credit-augmented Divisia. In historical decomposition, strong co-movement between the growth rate of the credit-augmented Divisia and the credit supply shocks is clear. Based on these results, we find that considering credit-related variables and shocks helps to interpret recent economic phenomena, with the credit-augmented Divisia being especially informative.
As the utilization of credit in the world’s economies continues to increase, overlooking credit-related variables is not justifiable. The conventional simple sum monetary aggregates, unable to include credit, are especially inadequate. The accounting convention preventing aggregating jointly over assets and liabilities is not relevant to economic aggregation theory, which aggregates over service flows, regardless of whether those services are from assets, such as monetary assets, or liabilities, such as credit card volumes. The credit-augmented Divisia can provide important insights, as revealed by this paper’s empirical results.
Disclosure statement
No potential conflict of interest was reported by the authors.
Notes
1 Further extension to the case of risk with intertemporal nonseparability can be found in Barnett and Liu (Citation2019).
2 We used the Matlab code of Filippo Ferroni and Fabio Canova (2020).
4 In a similar manner, Kilian and Lee (Citation2014) focus on the historical behavior of the de-meaned real price of crude oil and individual shocks. They find that the increase in crude oil prices in the early millennium is attributed to the cumulative effect of a flow demand shock.
References
- Andreou, E., Ghysels, E., & Kourtellos, A. (2010). Regression models with mixed sampling frequencies. Journal of Econometrics, 158(2), 246–28. https://doi.org/10.1016/j.jeconom.2010.01.004
- An, L., & Wang, J. (2012). Exchange rate pass-through: Evidence based on vector autoregression with sign restrictions. Open Economies Review, 23(2), 359–380. https://doi.org/10.1007/s11079-010-9195-8
- Barnett, W. A. (1978). The user cost of money. Economics Letter, 1(2), 145–149. Reprinted in Barnett, W. A. and Serletis, A. (eds.) (2000), The Theory of Monetary Aggregation, North Holland, Amsterdam, pp. 6-10. https://doi.org/10.1016/0165-1765(78)90051-4
- Barnett, W. A. (1980). Economic monetary aggregates: An application of index number and aggregation theory. Journal of Econometrics, 14(1), 11–48. Reprinted in Barnett, W. A. and Serletis, A. (eds.) (2000), The Theory of Monetary Aggregation, North Holland, Amsterdam, 11–48. https://doi.org/10.1016/0304-4076(80)90070-6
- Barnett, W. A. (1983). New indices of money supply and the flexible laurent demand system. Journal of Business & Economic Statistics, 1(1), 7–23. Reprinted in Barnett, W. A. and Serletis, A. (eds.) (2000), The Theory of Monetary Aggregation, North Holland, Amsterdam, 325–359. https://doi.org/10.2307/1391766
- Barnett, W. A. (1987). The microeconomic theory of monetary aggregation. In W. A. Barnett & K. Singleton (Eds.), New approaches to monetary economics: Proceedings of the Second International Symposium in Economic Theory and econometrics, (pp. 156–168 doi:). Cambridge University Press. Reprinted in Barnett, W. A. and Serletis, A. (eds.) (2000), The Theory of Monetary Aggregation, North Holland, Amsterdam, pp. 49-99.
- Barnett, W. A., & Chauvet, M. (2011). Financial aggregation and index number theory. World Scientific.
- Barnett, W. A., Chauvet, M., Leiva-Leon, D., & Su, L. (2023, July 28). The credit-card-services augmented divisia monetary aggregates. Journal of Money, Credit, and Banking. https://doi.org/10.1111/jmcb.13088
- Barnett, W. A., & Liu, J. (2019). User cost of credit card services under risk with intertemporal nonseparability. Journal of Financial Stability, 42, 18–35. https://doi.org/10.1016/j.jfs.2019.05.005
- Barnett, W. A., & Park, S. (2022). Forecasting inflation and output growth with credit-card-augmented divisia monetary aggregates. Journal of Forecasting, 42(2), 1–16. https://doi.org/10.1002/for.2910
- Barnett, W. A., & Serletis, A. (Eds.). (2000). The theory of monetary aggregation. North Holland, Amsterdam.
- Barnett, W. A., & Su, L. (2016). Joint aggregation over money and credit card services under risk. Economics Bulletin, 36(4), A223–A234.
- Barnett, W. A., & Su, L. (2018). Financial firm production of inside monetary and credit card services: An aggregation theoretic approach. Macroeconomic Dynamics, 24(1), 1–31. https://doi.org/10.1017/S1365100518000767
- Barnett, W. A., & Su, L. (2019). Risk adjustment of the credit-card augmented divisia monetary aggregates. Macroeconomic Dynamics, 23(S1), 90–114. https://doi.org/10.1017/S1365100518000160
- Chrystal, K. A., & MacDonald, R. (1994). Empirical evidence on the recent behavior and usefulness of simple-sum and weighted measures of the money stock. Federal Reserve Bank of St Louis, March/april, 76(2), 73–109. https://doi.org/10.20955/r.76.73-109
- Del Negro, M., & Scorfheide, F. (2013). DSGE model-based forecasting. Handbook of Economic Forecasting, 2(Part A), 57–40. https://doi.org/10.1016/B978-0-444-53683-9.00002-5
- Dery, C., & Serletis, A. (2019). Interest rates, money, and economic activity. Macroeconomic Dynamics, 25(7), 1842–1891. https://doi.org/10.1017/S1365100519000890
- Dotsey, M., & Otrok, C. (1994). M2 and monetary policy: A critical review of the recent debate. Federal Reserve of Richmond Economic Quarterly, 80(1), 41–59.
- Fry, R., & Pagan, A. (2011). Sign restrictions in structural vector autoregressions: A critical review. Journal of Economic Literature, 49(4), 938–960. https://doi.org/10.1257/jel.49.4.938
- Ghysels, E. (2016). Macroeconomics and the reality of mixed frequency data. Journal of Econometrics, 193(2), 294–314. https://doi.org/10.1016/j.jeconom.2016.04.008
- Hallman, J. J., Porter, R. D., & Small, D. H. (1991). Is the price level tied to the M2 monetary aggregate in the Long Run? American Economic Review, 81(4), 841–858.
- Kilian, L., & Lee, T. K. (2014). Quantifying the speculative component in the real price of oil: The role of global oil inventories. Journal of International Money and Finance, 42, 71–87. https://doi.org/10.1016/j.jimonfin.2013.08.005
- Kilian, L., & Murphy, D. P. (2012). Why agnostic sign restrictions are not enough: Understanding the dynamics of oil market VAR models. Journal of the European Economic Association, 10(5), 1166–1188. https://doi.org/10.1111/j.1542-4774.2012.01080.x
- King, R. G., Plosser, C. I., Stock, J. H., & Watson, M. W. (1991). Stochastic trends and economic fluctuations. American Economic Review, 81(4), 819–840. https://doi.org/10.3386/w2229
- Kuzin, V., Marcellino, M., & Schumacher, C. (2011). MIDAS vs. Mixed-frequency VAR: Nowcasting GDP in the euro area. International Journal of Forecasting, 27(2), 529–542. https://doi.org/10.1016/j.ijforecast.2010.02.006
- Littlejohn, M. (2020). Sector-specific credit supply shocks and the U.S. Economy. Working paper. Else School of Management.
- Liu, J., Dery, C., & Serletis, A. (2020). Recent monetary policy and the credit card-augmented divisia monetary aggregates. Journal of Macroeconomics, 64, 103203. https://doi.org/10.1016/j.jmacro.2020.103203
- Liu, J., & Serletis, A. (2020). Money growth variability and output: Evidence with credit-card-augmented divisia monetary aggregates. Studies in Nonlinear Dynamics and Econometrics, 24(5), 1–11. https://doi.org/10.1515/snde-2019-0106
- Mian, A. R., Sufi, A., & Verner, E. (2017). Household debt and business cycles worldwide. The Quarterly Journal of Economics, 132(4), 1755–1817. https://doi.org/10.1093/qje/qjx017
- Rubio-Ramirez, J. F., Waggoner, D. F., & Zha, T. (2010). Structural vector autoregressions: Theory of identification and algorithms for inference. The Review of Economic Studies, 77(2), 665–696. https://doi.org/10.1111/j.1467-937X.2009.00578.x
- Schunk, D. L. (2001). The relative forecasting performance of the divisia and simple sum monetary aggregates. Journal of Money, Credit, and Banking, 46(2), 272. https://doi.org/10.2307/2673885
- Scorfheide, F., & Song, D. (2015). Real-time forecasting with a mixed-frequency VAR. Journal of Business & Economic Statistics, 33(3), 366–380. https://doi.org/10.1080/07350015.2014.954707
- Scorfheide, F., & Song, D. (2023). Real-time forecasting with a (standard) mixed-frequency VAR during a pandemic. International Journal of Central Banking. (forthcoming).
- Serletis, A. (2009). A Bayesian classification approach to monetary aggregation. Macroeconomic Dynamics, 13(2), 200–219. https://doi.org/10.1017/S1365100508080024
- Serletis, A., & Gogas, P. (2014). Divisia monetary aggregates, the great ratios, and classical money demand functions. Journal of Money, Credit and Banking, 46(1), 229–241. https://doi.org/10.1111/jmcb.12103
- Serletis, A., & Rahman, S. (2013). The case for divisia money targeting. Macroeconomic Dynamics, 17(8), 1638–1658. https://doi.org/10.1017/S1365100512000247
- Sims, C. A. (1980). Macroeconomics and reality. Econometrica, 48(1), 1–49. https://doi.org/10.2307/1912017
- Sims, C. A. (1988). Bayesian skepticism on unit root econometrics. Journal of Economic Dynamics and Control, 12(2–3), 463–474. https://doi.org/10.1016/0165-1889(88)90050-4
- Uhlig, H. (2005). What are the effects of monetary policy on output? Results from an agnostic identification procedure. Journal of Monetary Economics, 52(2), 381–419. https://doi.org/10.1016/j.jmoneco.2004.05.007
- Wolf, C. K. (2020). SVAR (Mis)identification and the real effects of monetary policy shocks. American Economic Journal Macroeconomics, 12(4), 1–32. https://doi.org/10.1257/mac.20180328
- Wu, J. C., & Xia, F. D. (2016). Measuring the macroeconomic impact of monetary policy at the zero lower bound. Journal of Money, Credit and Banking, 48(2–3), 253–291. https://doi.org/10.1111/jmcb.12300
- Zadrozny, P. A., & Chambers, M. J. (1988). Econometric modelling with mixed frequency and temporally aggregated data. Journal of Time Series Analysis, 40(6), 869–871. https://doi.org/10.1111/jtsa.12510
Appendix A.
Tables
Table A1. Data information for back-casting.
Table A2. Data information for VAR-Sign restriction.
Table A3. Sign restriction using simple sum M2.
Table A4. Median target forecast error variance decomposition using simple sum M2.
Appendix B.
Figures using simple sum M2
Figure B1. Log of real GDP (left), growth rate of real GDP (right). Mixed-frequency VAR using simple sum M2.

Figure B3. 1,000-rotations of impulse responses to demand shock (up-left), supply shock (up-right), monetary policy shock (down-left), credit supply shock (down-right) with data set including SM2.

Figure B4. Impulse responses to demand shock (up-left), supply shock (up-right), monetary policy shock (down-left), and credit supply shock (down-right) with data including SM2.

Appendix C.
Robustness
In this appendix, we discuss robustness exercises to evaluate the model’s econometric performances. To test robustness, we adopt two alternative methods: (1) extending the lag length from 4 to 6 months, and (2) imposing the same restrictions in the first period after impact for the monthly frequency. Both of the robustness tests report the median value of impulse responses and follow the benchmark identification according to the table in chapter 3.5. Robustness to the choice of prior distribution is also important in Bayesian analysis. But we are leaving that formidable and potentially controversial analysis to future research.
C.1. Lag length
While the AIC and BIC suggest four lags as an ideal length for the benchmark model in Chapter 3, we adopt six lags (extended lag) estimation, which is a classical methodology for robustness check. displays the result. Generally, the impulse responses for six lags show similar behavior to the benchmark responses. However, we can track the specific discrepancy between the benchmark and robustness model in detail. In the robustness model, the impulse responses to all of the shocks for the consumer price index and that for credit-augmented Divisia show more fluctuations before they converge. Meanwhile, for the impulse responses to the monetary policy shock, the cycle of fluctuation tends to be shortened compared to the benchmark model. However, in the long run view, the responses in the robustness model show similar convergence to the benchmark model. In short, the change in lags induces a minor impact on the benchmark model robustness.
C.2. Imposing the restrictions in the first period after impact for the monthly frequency
This approach is a renowned and widely used methodology to check robustness. Since the impulse responses to demand, supply, and monetary policy shock in the robustness model do not show noticeable differences compared to the benchmark estimation, we focus only on the impulse responses to the credit supply shock (., down-right). For most of the variables, the impulse responses tend to converge to positive values in the long run, which are larger than the benchmark estimate. However, for the fluctuations before the convergence, the shapes and magnitudes are nearly identical to the benchmark estimations. Therefore, we conclude that the change in the timing of restriction imposition induces an insignificant impact on the benchmark results.