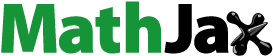
ABSTRACT
We investigate whether climate change affects the efficiency of monetary policy. We use temperature shocks, calculated as temperature deviations from historical average temperatures, to proxy climate change, and utilize a threshold vector autoregression model (TVAR) to estimate the impact of expansionary and tight monetary shocks on economic output under high and low regimes of temperature shocks. Our results characterize a climate change regime-dependent monetary policy. Expansionary monetary policy is less efficient and the negative impact of tight monetary policy is enhanced, when climate change is severe. The results can be explained by the climate-induced credit constraint of commercial banks. Higher temperature shocks lead to increases in banks’ non-performing loan ratios, which results in larger credit constraints of banks. Banks tend to be more prudent in credit expansion, and the bank credit channel of monetary policy transmissions is weakened.
1. Introduction
Does climate change affect the efficiency of monetary policy? This is an important question in climate finance. Monetary policy is regarded as one of the most important tools to mitigate economic inefficiency caused by climate change (Baranzini et al., Citation2017; Maestre-Andrés et al., Citation2019). Answering this question enhances the understanding of the economic and financial consequences of climate change and provides useful guidance for climate-based monetary policy formulations (L. P. Hansen, Citation2022).
Theoretically, expansionary monetary policy should be less efficient when climate change is severe. Climate change has been recognized worldwide as a new and nonnegligible source of economic and financial instability (Dafermos et al., Citation2018; Giglio et al., Citation2021). Climate change and its induced natural disasters have negative shocks to economic activities and lead to the devaluation of collaterals (Burke et al., Citation2015; Dell et al., Citation2009, Citation2012; Letta & Tol, Citation2019). Because climate change affects economic activities and the valuation of collaterals, the debt payments of corporations and households will deteriorate (Dafermos et al., Citation2018; Hosono et al., Citation2016; Klomp, Citation2014). The ratio of non-performing loans of commercial banks will increase, exposing banks to greater credit constraints and making banks more prudent to expand credit (Abbas et al., Citation2021; Abou-El-Sood, Citation2016; Pool et al., Citation2015). The credit channel of monetary policy transmissions is weakened by climate change, and thus, expansionary monetary policy is less influential when climate change is severe. Similarly, the negative impact of tight monetary policy on economic output should be exemplified when climate change is severe. Central bank should care for the climate change proactively by using the traditional monetary policy (C. Chen et al., Citation2021). Although these theories are well understood, the empirical evidence is still limited. Here are the research questions: Is the influence of monetary policy affected by climate change? How to describe the impact of climate change on the influence of monetary policy? How to explain the impact of climate change on the influence of monetary policy?
In this paper, we provide empirical evidence on the impact of climate change on monetary policy by examining the responses of economic output to monetary shocks under climate change regimes. We use temperature shocks, which are calculated as temperature deviations from historical average temperatures, to proxy climate change (Hong et al., Citation2019; Russell et al., Citation2014; Song & Fang, Citation2023). In the empirical analysis, we utilize a TVAR framework to reveal the impact of monetary policy on output, and the temperature shock is regarded as the threshold variable. Monetary policy (M2), output (GDP), and price level (CPI) are included as other endogenous variables. We perform one-standard-deviation unanticipated positive and negative monetary shocks separately and estimate the generalized impulse responses of output to shocks under high- and low-temperature regimes. Moreover, we explain our results through climate-induced credit constraints in the bank credit channel.
Our results are summarized as follows. First, we find that the influence of monetary policy is climate change regime dependent. Temperature shocks weaken the efficiency of monetary expansions and worsen the effects of monetary tightening. Second, previous literature shows that the effects of expansionary and tight monetary policies are asymmetric, and we show that asymmetries are more apparent under high-temperature regimes. Third, we provide empirical evidence to explain the path through which climate change affects the effectiveness of monetary policy by confirming the credit constraints of commercial banks. Temperature shocks worsen economic activities, which increases corporations’ and households’ loan default rates and banks’ non-performing loan ratios. Consequently, banks have greater credit constraints and are prudent in expanding credit under high-temperature regimes, suggesting that the credit channel of monetary policy is weakened.
Our study contributes to the literature on the role of climate change in monetary policy implementation. We provide empirical evidence that the efficiency of monetary policy is influenced by climate change, characterized as climate change regime dependency, which is meaningful to monetary policy in the context of climate change (C. Chen et al., Citation2021; George & Anastasios, Citation2018). Our results confirm the theoretical findings of C. Chen et al. (Citation2021) and suggest that policymakers should consider climate change in monetary policy formulations proactively. Expansionary monetary policy could be more aggressive when temperatures are higher, and tight monetary policy could be more conservative when climate change is severe.
Our paper is also related to the rich literature on the transmission of monetary policy shocks. We find that climate change dynamics affect monetary policy efficiency (Aastveit et al., Citation2017; Boivin & Giannoni, Citation2006; Caggiano et al., Citation2014). Specifically, we empirically confirm the climate-induced credit constraint of commercial banks, which has only been investigated in theory (Berg & Schrader, Citation2012; Hosono et al., Citation2016). Increases in temperature lead to higher bank non-performing loan ratios and greater credit constraints, which make banks more prudent in extending credit. The credit constraint weakens the bank credit channel of monetary policy and thus provides an explanation for the impact of climate change on the influences of monetary policies from a micro perspective.
The remainder of our paper is organized as follows. Section 2 presents a literature review. Section 3 describes the TVAR model and the data. Section 4 reports our main results and robustness checks. Section 5 explains our results, and Section 6 concludes.
2. Literature review and theoretical analysis
2.1. Literature review
Monetary policy plays an important role in adapting to climate-related risks, and central banks should incorporate climate risks into their policy frameworks to maintain economic and financial stability (Dikau & Volz, Citation2021). Most of the previous literature explores how central banks use monetary policy to support the transition to a low-carbon economy.
On the one hand, monetary policy acts as a complementary instrument for climate policy to cope with climate risks. It is not sufficient to realize the desired objectives by only implementing climate policies, and it is of great importance to consider additional policy instruments (Baranzini et al., Citation2017; Campiglio, Citation2016; Engle et al., Citation2018; Maestre-Andrés et al., Citation2019; Rozenberg et al., Citation2013). Monetary policy is regarded as an appropriate tool to address such problems (Benmir & Roman, Citation2020; Chan, Citation2020). Annicchiarico and Dio (Citation2017) utilize an extended environmental dynamic stochastic general equilibrium model to reveal that central banks should integrate climate change into monetary policy. Chan (Citation2020) investigates the interactions among climate change, monetary and fiscal policies using an environmental DSGE model and demonstrate that carbon taxation should complement monetary policy but not respond to fiscal policy, in which carbon emissions and household welfare can be maintained as dynamically stable. Moreover, because of market failures, Campiglio (Citation2016) finds that carbon pricing itself is not effective enough to enhance banking credit for low-carbon sectors, whereas monetary policy contributes to attenuating the constraints of bank lending, especially in emerging economies, for which central banks take powerful controls on credit allocation and use more monetary policy instruments.
On the other hand, some studies propose new climate-related monetary policy instruments that integrate climate objectives into monetary policy and evaluate their effects. Böser and Senni (Citation2020) propose climate-oriented monetary policy instruments, including green quantitative easing, green reserve requirements and a green collateral framework. They find that these instruments can make firms adopt cleaner technologies across the entire economy, reduce carbon dioxide emissions and mitigate climate damage. Boneva et al. (Citation2022) incorporate climate change objectives into monetary policy and discuss a couple of actions central banks can take to mitigate climate change. McConnell et al. (Citation2022) investigate several green monetary policy instruments and point out that it is the most promising conduit to incorporate brown collateral haircuts into the collateralized lending framework of central banks.
However, studies on the effects of climate change on monetary policy are currently quite limited. George and Anastasios (Citation2018) use an indirect method to assess the impacts of climate change on monetary policy. They incorporate total factor productivity (TFP) shocks derived from climate change uncertainty into the integrated assessment model (IAM) and then suggest that climate change uncertainty leads to larger and more persistent fluctuations in economic activity. By embodying more novel environmental features, such as the concealed emissions and potential penalties, and climate policy in the E-DSGE model, C. Chen et al. (Citation2021) find that climate policy is a factor affecting price level and welfare. McKibbin et al. (Citation2021) point out that supply shocks from climate change disrupt central banks’ ability to forecast and manage inflation and highlight that climate risks in the monetary framework will make monetary policy more effective.
In summary, one stream of literature mainly focuses on monetary policy as a complementary instrument for climate policy to mitigate climate risks and focuses on incorporating climate objectives into monetary policy. Another stream of literature highlights the role of monetary policy in dealing with climate risks and achieving sustainable development. However, there are several issues to be addressed. First, a large number of previous studies suggest that climate change can impair agricultural yields, industrial output, and economic growth, which are primary monetary policy concerns. However, few studies have simultaneously considered climate change, monetary policy, and output in a framework to discuss the effectiveness of monetary policy in the context of climate change (Carleton & Hsiang, Citation2016; Dell et al., Citation2012). Second, though many studies provide theories that relate climate change to monetary policy, the number of empirical examinations is quite limited. Evaluating the reactions of monetary policy to climate shocks is beneficial to more explicitly recognize climate risks, which is the foremost prerequisite of central banks to craft optimal monetary policy to attain objectives. These studies also provide evidence for how central banks should adjust monetary policy conditional on climate change and new perspectives for adapting to climate risks in monetary policy transmission. Third, previous studies have not shown empirical evidence on the influential mechanism that explains how climate change affects the efficiency of monetary policy. China has a bank-based financial system, which implies that the bank credit channel is important in monetary policy transmission. If climate change affects the bank credit channel, then it will definitely affect the influence of monetary policy. In the following analyses, we aim to address these issues using TVAR models and bank-level micro data.
2.2. Theoretical analysis and hypothesis development
The effective implementation of monetary policy depends not only on the efforts of the government, which formulates feasible monetary policy, but also, more importantly, on the transmission of monetary policy (Acharya et al., Citation2020; K. Chen et al., Citation2018). For example, when banks face strict regulatory and financial constraints or are undercapitalized, the transmission channel of monetary policy may be hampered, leading to less effective expansive monetary policy (Acharya et al., Citation2020). Meanwhile, the transmission channel mainly relies on financial institutions or the financial market, and climate change is regarded as a new source of financial instability (Giglio et al., Citation2021; Mishkin, Citation1996, Citation2001; Walsh, Citation2017). Therefore, it is conceivable for us to explore the path through which climate change affects monetary policy from the view of transmission channels.
We focus on evidence from China. Despite being the largest developing economy, its financial market is not well developed and its financial system primarily relies on the banking system (Hou et al., Citation2018; Klingelhöfer & Sun, Citation2019; H. Li et al., Citation2021). There are several other transmission channels of monetary policy, such as the interest rate channel, asset price channel, and exchange rate channel (Bernanke & Blinder, Citation1992; Kashyap & Stein, Citation1995; Mishkin, Citation1996, Citation2001; Walsh, Citation2017). In China, the (bank) credit channel dominates all other monetary policy transmission channels, whose contributions are relatively limited. In this regard, we analyze the role of banks in explaining the impact of climate change on the transmission of monetary policy.
According to the literature, temperature shocks have negative consequences for economic activities and human behavior. High temperatures lead to decreases in firm profitability through labor productivity, total factor productivity (TFP), and operation costs. First, temperature is negatively associated with labor productivity. High temperatures tend to harm human physiological functions, cognitive capacities, and psychological health, resulting in losses in labor productivity (S. Chen et al., Citation2018; Deschênes & Moretti, Citation2009; Hsiang, Citation2010; Seppänen et al., Citation2006; Somanathan et al., Citation2021; Zheng et al., Citation2019; Zivin et al., Citation2015). Second, high temperatures reduce TFP growth. Donadelli et al. (Citation2017) indicate that temperature risk has a long-lasting negative effect on TFP, which worsens the welfare cost. Third, temperature shocks increase enterprise operation costs. Pankratz et al. (Citation2023) document that administrative, selling, and general expenses increase when firms are exposed to high temperatures over prolonged periods of time, leading to rising operation costs.
Based on the relationship between temperature shocks and firm profitability, temperature shocks tend to exacerbate the credit channel. High temperatures weaken firm profitability, which deteriorates the firm balance sheet and firms’ ability to repay loans from banks. The non-performing loan ratios of banks correspondingly increase, causing banks to suffer capital losses and to reduce lending to maintain the regulatory capital ratio (Abbas et al., Citation2021; Abou-El-Sood, Citation2016; Sandra et al., Citation2016). In addition, increases in the non-performing loan ratios could signal an economic downturn, and commercial banks could become more prudent and conservative in lending to firms (Kollmann et al., Citation2010; Pool et al., Citation2015). Weakened firm profitability is also closely related to greater adverse selection and moral hazard, which hinders banks from lending to these firms.
Overall, high temperatures weaken firm profitability and increase the default probabilities of bank loans, thus resulting in higher bank non-performing loan ratios. These consequences make banks more risk averse and prudent in expanding credit and dampen the efficiency of monetary policies. This is the climate-induced credit constraint, as theoretically stated by Berg and Schrader (Citation2012) and Hosono et al. (Citation2016). Therefore, we propose the following hypotheses:
H1:
When climate change is severe, the positive impact of expansionary monetary policy is less efficient and the negative impact of tight monetary policy is enhanced.
H2:
Climate change affects the efficiency of monetary policy by weakening the bank credit channel of monetary policy transmission.
3. Econometric model and data descriptions
3.1. TVAR model specification
We employ a TVAR model to examine the regime-dependent nonlinear effects of monetary policy in the context of climate change (Lo & Zivot, Citation2001; Tsay, Citation1998). The TVAR model has the following characteristics and advantages. First, the TVAR model is a nonlinear multivariate system with regime switching. We can transform the model into several distinct linear VARs. These VARs correspond to several regimes based on the threshold variable (in this paper, the threshold is the climate change variable), and the coefficients of VAR models are unique to each regime. Second, the TVAR model allows the threshold variable to be endogenous. This indicates that the regime can switch after shocks occur (Afonso et al., Citation2018; Balke, Citation2000; Ferraresi et al., Citation2015; Jörg, Citation2020)
To investigate whether climate change affects the influence of monetary policy, we use temperature shocks as a proxy for climate change and as the threshold variable. The model with two regimes is specified as follows:
where is the vector of all endogenous variables, including temperature shocks, monetary policy, price level, and output. In this paper, and the sample period is from 2004Q2 to 2021Q4 (additional information on these variables can be found in Section 3.2).Footnote1 We set the ordering as temperature shocks, monetary policy, price level, and output in the baseline TVAR model. The ordering in the vector of endogenous variables reflects the way in which variables interact.Footnote2
is the threshold variable for temperature shocks at time
, and
is the lag length.
is an indicator function that equals 1 when
is more than threshold
and 0 otherwise. This setting means that regime switching occurs at time
if the threshold variable at time
exceeds
.
and
are the vectors of the constant term.
and
are lag polynomial matrices.
is the vector of structural shocks.
To reveal the responses of endogenous variables to shocks, we employ the generalized impulse response function (GIRF) to compute impulse responses (Koop et al., Citation1996). Moreover, the GIRF allows us to investigate the effects of shocks of distinct directions and sizes. The GIRF is described as follows:
where is the response variable,
is the horizon,
denotes historical information and
is the shock. The response of variable
at horizon
is that the expectation of variable
at period
imposing shock
conditional on history
deducts the expectation of variable
at period
without shock
conditional on history
, which is calculated under the framework of linear VAR. In regard to the TVAR model, it is necessary to calculate impulse responses for each regime.
3.2. Data descriptions
3.2.1. Temperature data
Following previous studies, temperature shocks are calculated as temperature deviations from historical average temperatures, which indicate the trend of global warming and unanticipated temperature changes (Hong et al., Citation2019; Song & Fang, Citation2023). The temperature data for China are obtained from the National Centers for Environmental Information (NCEI) of the National Oceanic and Atmospheric Administration (NOAA). The NCEI database of NOAA provides weather data monitored by every meteorological station around the world. To calculate temperature shocks in China, we follow three steps. First, we select stations located in China. Because missing data at each station are a challenge for calculating average temperatures, we remove stations that recorded data for fewer than 350 days per year, resulting in a remaining 260 stations. Next, we collect temperature data from these stations and take the quarterly average of the daily temperatures at each station. Finally, we compute the quarterly average temperatures of these stations () and remove the trend by subtracting the historical H-year averages from quarterly average temperatures:
The historical average temperatures are computed using H = 30/25/20-year moving averages (M. E. Kahn et al., Citation2021). plots the temperature shocks in China. We find that most temperature shocks are greater than zero, which reflects a trend of global warming. Temperature shocks have larger fluctuations in the first and fourth quarters, which is consistent with the findings of Dell et al. (Citation2012) and S. Kahn et al. (Citation2019). The sample period spans from 2004Q2 to 2021Q4.
3.2.2. Macroeconomic data
The money stock and interbank-offered rate are usually employed as proxies of monetary policy. The interbank-offered rate has the drawbacks of forward-looking expectations and inconsistent movement with monetary policy, while money stocks predominantly act as the intermediate target of monetary policy in China (K. Chen et al., Citation2018; Heryan & Tzeremes, Citation2017; R. Li & Tian, Citation2018).Footnote3 To proxy monetary policy, we use the monetary stock growth rate, which is calculated as the year-on-year growth rate of money stocks (M2). In the TVAR model, we include the consumer price index (CPI) and economic output (GDP), which is calculated as the year-on-year growth rate of gross domestic product. We utilize Census X-12 to seasonally adjust the quarterly time series. The data are all collected from the WIND database, and the sample period spans from 2004Q2 to 2021Q4, corresponding to the sample period for climate change.Footnote4
We perform the augmented Dickey–Fuller (ADF) test to check the variables’ stationarity, which is a vital prerequisite for applying a TVAR model (Banerjee et al., Citation1993; Said & Dickey, Citation1984). The null hypothesis of the ADF test is that the variable contains a unit root, and the alternative hypothesis is that the variable is generated by a stationary process. shows the results of the ADF test for all variables, including specifications. We find that all tests reject the null hypothesis at the 1% significance level. All the variables are stationary. The summary statistics for all the variables included in the TVAR models can also be found in .
Table 1. Summary statistics and unit root test.
4. Empirical results
4.1. Nonlinearity test
Before estimating the TVAR model, we apply the nonlinearity test to examine whether it is reasonable to employ the TVAR model in this paper and determine the number of regimes to set (B. E. Hansen, Citation1999; Lo & Zivot, Citation2001). To answer these two questions, we perform three nonlinearity tests, in which the null hypotheses include the “linear VAR versus two-regime TVAR model (1vs2)”, “linear VAR versus three-regime TVAR model (1vs3)” and “two-regime TVAR model versus three-regime TVAR model (2vs3)”. We take the value of 1 for the lag order based on the AIC and BIC. reports the results of the nonlinearity tests. According to the “1vs2” and “1vs3” tests, all the statistics reject the null hypothesis of linear VAR at the 1% or 5% significance levels, indicating the presence of multiple regimes. In this respect, employing the TVAR model is preferable. Comparing the two-regime TVAR model with the three-regime TVAR model, the results show that we should not reject the null hypothesis of a two-regime TVAR model. Therefore, we employ a TVAR model with two regimes for temperature shocks and evaluate the efficiency of monetary policy under high- and low-temperature shocks.Footnote5
Table 2. Nonlinearity test.
4.2. Results from the TVAR model
To explore the impact of climate change on the influence of monetary policy, we use the GIRFs to analyze the output responses to positive and negative policy shocks. We separately perform one-standard-deviation unanticipated positive and negative monetary policy shocks to the TVAR models. shows the impulse responses for TVAR models using temperature shocks as threshold variables for the first 20 quarters. The dashed and solid lines denote the impulse responses under the high regime and low regime of temperature shocks, respectively.
Figure 2. Impulse responses for TVAR models with Y=[tempv_H, M2, CPI, GDP].
![Figure 2. Impulse responses for TVAR models with Y=[tempv_H, M2, CPI, GDP].](/cms/asset/24767244-e4ce-4968-bdc9-590181ba33a3/recs_a_2329840_f0002_b.gif)
The first column shows the responses of the output to one-standard-deviation positive monetary policy shocks. The output positively responds to monetary policy shocks, which aligns with conventional economic theory. We mainly focus on the differences in impulse responses under high and low regimes of temperature shocks. The influences of monetary policy under high and low regimes of temperature shocks are apparently distinctive. The responses of output are always positive under low regimes, and GDP increases by at most approximately 0.4%. The effect of monetary shocks on output during hotter periods is slightly negative for the first two-quarters and becomes positive for the following quarters. Such lagged effects of monetary policy shocks can occur due to insufficient information, as stated by Kerssenfischer (Citation2019). Moreover, the output only increases by approximately 0.1% following the monetary shock when tempv_30 and tempv_20 are used as threshold variables under high regimes. More importantly, the responses of output under high regimes are lower than those under low regimes, implying that the effect of monetary expansion is less efficient when temperatures are higher than historical averages.
The second column displays impulse responses to one-standard-deviation negative monetary policy shocks, which correspond to tight monetary policies. The output negatively responds to negative shocks. Under low regimes of temperature shocks, the output falls by at most approximately 0.5%, and under high regimes, it falls by at most approximately 0.9%. Output is more sensitive to negative monetary shocks under high-temperature regimes. In addition, the effects of tight monetary shocks are stronger than the effects of expansionary shocks, and such asymmetric effects are quite similar to the results in Cover (Citation1992) and Florio (Citation2004).
indicates that temperature shocks weaken the efficiency of monetary expansions and worsen the effects of monetary tightening, which corresponds to a temperature-regime dependency in monetary policy transmission. The patterns of regime-dependent impulse responses are consistent with the theoretical results of Sandra et al. (Citation2016) and George and Anastasios (Citation2018). We also empirically confirm the results of George and Anastasios (Citation2018), who argue that climate change leads to larger fluctuations in economic outputs and lengthens the duration of divergence to steady states. Overall, Hypothesis H1 is confirmed.
4.3. Robustness checks
4.3.1. Alternative orders of endogenous variables
We consider alternative orderings of endogenous variables in TVAR models. In the TVAR model in Section 4.2, we order these variables based on the assumption that temperature shocks have an immediate impact on the other variables but do not contemporaneously respond to other shocks, and that they are the same as monetary policy. However, economic growth also leads to an increase in carbon dioxide emissions and climate fluctuations. The assumption for the model in Section 4.2 may not be appropriate, and the impulse responses may change (Copeland & Taylor, Citation2004). To determine if and to what extent the assumption could affect our results, we employ four possible alternative orders of the endogenous variable vectors to see if our results remain the same.
show that the impulse responses of output to monetary shocks under high and low regimes of temperature shocks are the same as those shown in . Our empirical findings are not sensitive to the order of the endogenous variables, confirming their robustness.
Figure 3. Impulse responses for the TVAR model with Y=[M2, tempv_H, CPI, GDP].
![Figure 3. Impulse responses for the TVAR model with Y=[M2, tempv_H, CPI, GDP].](/cms/asset/0d2a16e5-7525-424d-983c-cbd1321095b0/recs_a_2329840_f0003_b.gif)
Figure 4. Impulse responses for the TVAR model with Y=[M2, CPI, GDP, tempv_H].
![Figure 4. Impulse responses for the TVAR model with Y=[M2, CPI, GDP, tempv_H].](/cms/asset/8f954510-03eb-41d8-b856-42af5b43b9c5/recs_a_2329840_f0004_b.gif)
Figure 5. Impulse responses for the TVAR model with Y=[CPI, GDP, tempv_H, M2].
![Figure 5. Impulse responses for the TVAR model with Y=[CPI, GDP, tempv_H, M2].](/cms/asset/4a87b4d8-26ef-411e-a20d-6d1c51a8c427/recs_a_2329840_f0005_b.gif)
Figure 6. Impulse responses for the TVAR model with Y=[CPI, GDP, M2, tempv_H].
![Figure 6. Impulse responses for the TVAR model with Y=[CPI, GDP, M2, tempv_H].](/cms/asset/5407a964-6d56-499e-bb40-5aa09d3fe0a5/recs_a_2329840_f0006_b.gif)
4.3.2. Controlling for fiscal policy
Fiscal policy is also an important factor that influences economic growth, and our results may be biased in the absence of fiscal policy. To address this potential issue, we include the fiscal policy variable (fp) in the endogenous variable vector. Fiscal policy is proxied by the year-on-year growth rate of public expenditure, which is collected from the National Bureau of Statistics of China. The endogenous variable vector becomes .
shows the impulse responses of monetary policy controlling the effects of fiscal policy. Unsurprisingly, the responses of output to monetary shocks become weaker. For example, the output increases by at most approximately 0.3% under the low regime, and the response is 0.2% under the high regime of temperature shocks with 20-year historical averages after a one-standard-deviation positive monetary shock. The efficiency of monetary policy is still lower when the weather is hotter.
Figure 7. Impulse responses for the TVAR model with Y = [tempv_H, M2, fp, CPI, GDP].
![Figure 7. Impulse responses for the TVAR model with Y = [tempv_H, M2, fp, CPI, GDP].](/cms/asset/6675d79a-d103-485e-9352-0f49dbecf74c/recs_a_2329840_f0007_b.gif)
4.3.3. Alternative variables for output
We use an alternative indicator of output. We replace the growth rate of GDP with the growth rate of industrial production and re-estimate the TVAR model. The results in show that using an alternative proxy of output does not affect our empirical results. In addition, we use monthly data for another robustness check to alleviate the bias from using a relatively small sample size of quarterly data. The impulse response results are shown in . With a larger sample size, the results are consistent with the baseline results.
Figure 8. Impulse responses for the TVAR model with Y=[tempv_H, M2, CPI, GDP_ind].
![Figure 8. Impulse responses for the TVAR model with Y=[tempv_H, M2, CPI, GDP_ind].](/cms/asset/db52881b-64b0-4594-afe2-5da15aa3ac21/recs_a_2329840_f0008_b.gif)
Figure 9. Impulse responses for the TVAR model with Y=[tempv_H, M2, CPI, IGDP].
![Figure 9. Impulse responses for the TVAR model with Y=[tempv_H, M2, CPI, IGDP].](/cms/asset/f274f45e-2b54-4048-b4a5-13d4affdd352/recs_a_2329840_f0009_b.gif)
5. Influential mechanism: the climate-induced credit constraint
Hypothesis H2 indicates that high temperatures weaken firm profitability, increase the default probabilities of bank loans, and result in higher bank non-performing loan ratios. Commercial banks will be more risk averse and prudent in expanding credit, which weakens the efficiency of monetary policies. This climate-induced credit constraint is used to explain the impact of climate change on monetary policy efficiency (Berg & Schrader, Citation2012; Hosono et al., Citation2016). To empirically examine the climate-induced credit constraint, we employ Equations (4)–(6).
where denotes the growth rate of the bank loan scale calculated by the first difference of log total loans,
denotes the temperature shocks as mentioned above,
denotes the log non-performing loan ratio of banks, and
denotes bank-level variables, which include bank size (lnsize), log total assets of banks, the capital ratio (cap), the liquidity ratio (liq), the ratio of liquid assets to total assets, the ratio of owners’ equity to total assets. We also include macroeconomic variables, such as shadow banking (sb), the growth rate of the social entrusted loan scale, the money stock (M2), and economic growth (GDP).
denotes the bank fixed effect. Considering the accessibility of bank data, we select 343 banks, containing 6 state-owned banks, 12 joint-stock commercial banks, 111 city commercial banks, and 214 rural commercial banks, spanning from 2004Q2 to 2021Q4. We obtain bank characteristics and economic data from the WIND database.
displays the estimation results for EquationEquations (3)(3)
(3) –(Equation5
(5)
(5) ). The standard errors are clustered at the bank level. In Columns (1), (4), and (7), the coefficient estimates for temperature shocks in EquationEquation (3)
(3)
(3) are significantly negative at the 10% or 5% level, indicating that increases in temperature shocks impede bank lending. For example, the estimated
for
is −0.007 with a robust t-statistic of −1.906, which is significant at the 10% level. In Columns (2), (5), and (8), the estimates for temperature shocks in EquationEquation (4)
(4)
(4) are significantly positive at the 1% level, suggesting that temperature shocks increase the non-performing loan ratio of commercial banks. In Columns (3), (6), and (9), the estimated coefficients for temperature shocks in EquationEquation (5)
(5)
(5) are all insignificant, while the coefficients
for the non-performing loan ratio are significantly negative at the 1% level. The significant
s and
s and the insignificant
s indicate that the non-performing loan ratio of banks has a complete mediating effect in explaining the influence of temperature shocks on monetary policies. Hypothesis H2 is confirmed.
Table 3. Mechanism test for credit channel.
6. Concluding remarks
In this paper, we investigate whether climate change affects the efficiency of monetary policy. We use temperature shocks, calculated as temperature deviations from historical average temperatures, to proxy climate change, and utilize TVAR models and GIRFs to reveal the effects of expansionary and tight monetary policies on economic output under high- and low-temperature regimes.
Our results suggest that there is a climate change regime dependency in monetary policy. Increases in temperature weaken the efficiency of monetary expansions and worsen the negative effects of monetary tightening. Consistent with previous findings, we also find that there are asymmetric effects for expansionary and tight monetary policy, in which tight monetary policy has stronger effects in both high- and low-temperature regimes. The asymmetries are more obvious under high-temperature regimes. We explain our results by empirically confirming the climate-induced credit constraint of commercial banks argued by previous theoretical studies. Temperature shocks can decrease labor productivity and TFP growth and increase firm operation costs, which impairs firm profitability associated with loan repayment, corresponding to an increase in the non-performing loan ratio of banks. Increases in non-performing loan ratios lead to credit constraints induced by climate change and thus weaken the credit channel of monetary policy transmissions.
In the era of global warming, our study has meaningful implications for climate risk management and mitigation for central banks and financial institutions. First, central banks should incorporate climate change into their monetary policy framework to formulate optimal monetary policies. For example, central banks should implement more aggressive expansionary monetary policy and weaker tight monetary policy when climate change is severe. Second, it is critical for banks to manage climate risk. As climate conditions deteriorate, commercial banks should take precautions to increase their risk-taking ability to avoid capital losses and perform climate stress tests to frequently evaluate climate change risk.
There are several limitations of our work. First, we highlight the channel through which banks engage in risk-taking in monetary policy transmission because the banking sector dominates the Chinese financial system. In developed economies, the capital market is more important than the banking sector, and new channels through which climate change affects the influence of monetary policy should also be investigated. Second, climate change variables of daily frequency may be available, but macroeconomic variables are usually quarterly. When including both daily and quarterly variables in a TVAR model, we must transform the frequency of climate change variables from daily to quarterly. This results in a loss of information of climate change fluctuations. To address this issue, we may rely on a mixed-frequency data sampling model that accommodates variables of different frequencies (Ghysels et al., Citation2006, Citation2007). Third, other proxies of climate change and natural disasters, such as precipitation, drought, and floods, may also affect the transmission of monetary policy. We may use the TVAR or other models to provide additional evidence. We leave them for future research.
Disclosure statement
No potential conflict of interest was reported by the author(s).
Data availability statement
Available upon request.
Additional information
Funding
Notes
1 Since the main objectives of People’s Bank of China implementing monetary policy are maintaining price stability and stimulating economic growth, we select three other variables, monetary policy, price level and output, in baseline TVAR model (H. Chen et al., Citation2017; K. Chen et al., Citation2018).
2 For example, temperature shocks are ordered first, indicating that temperature shocks do not contemporaneously react to all other variables. Output is ordered last, indicating that output contemporaneously reacts to all other variables. We also use other orderings in robustness checks.
3 The Central Economic Work Conference in China decides on the M2 growth target for the next coming year at the end of this year. In the next year, the government adjusts the actual M2 growth from quarter to quarter subject to the annual M2 growth target. M2 growth also serves mainly for the economic growth (K. Chen et al., Citation2018).
4 Due to the availability of monthly GDP data, we have to utilize quarterly data in the baseline TVAR model. Some readers may conjecture that the TVAR results can be biased by the small sample size. We address this concern by using monthly industrial production in Section 4.3.3. The results with a larger sample size remain robust.
5 The nonlinearity test and TVAR models are estimated using R software.
References
- Aastveit, K. A., Natvik, G. J., & Sola, S. (2017). Economic uncertainty and the influence of monetary policy. Journal of International Money and Finance, 76, 50–24. https://doi.org/10.1016/j.jimonfin.2017.05.003
- Abbas, F., Ali, S., & Rubbaniy, G. (2021). Economics of capital adjustment in the US commercial banks: Empirical analysis. Journal of Applied Economics, 24(1), 71–90. https://doi.org/10.1080/15140326.2021.1881877
- Abou-El-Sood, H. (2016). Are regulatory capital adequacy ratios good indicators of bank failure? Evidence from US banks. International Review of Financial Analysis, 48, 292–302. https://doi.org/10.1016/j.irfa.2015.11.011
- Acharya, V. V., Imbierowicz, B., Steffen, S., & Teichmann, D. (2020). Does the lack of financial stability impair the transmission of monetary policy? Journal of Financial Economics, 138(2), 342–365. https://doi.org/10.1016/j.jfineco.2020.06.011
- Afonso, A., Baxa, J., & Slavík, M. (2018). Fiscal developments and financial stress: A threshold VAR analysis. Empirical Economics, 54(2), 395–423. https://doi.org/10.1007/s00181-016-1210-5
- Annicchiarico, B., & Dio, F. D. (2017). GHG emissions control and monetary policy. Environmental and Resource Economics, 67(4), 823–851. https://doi.org/10.1007/s10640-016-0007-5
- Balke, N. S. (2000). Credit and economic activity: Credit regimes and nonlinear propagation of shocks. Review of Economics and Statistics, 82(2), 344–349. https://doi.org/10.1162/rest.2000.82.2.344
- Banerjee, A., Dolado, J. J., Galbraith, J. W., & Hendry, D. F. (1993). Co-integration, error correction, and the econometric analysis of non-stationary data. Oxford University Press.
- Baranzini, A., Bergh, J., Carattini, S., Howarth, R. B., Padilla, E., & Roca, J. (2017). Carbon pricing in climate policy: Seven reasons, complementary instruments, and political economy considerations. Wiley Interdisciplinary Reviews: Climate Change, 8(4), e462. https://doi.org/10.1002/wcc.462
- Benmir, G., & Roman, J. (2020). Policy interactions and the transition to clean technology. Grantham research institute on climate change and the environment working paper, 337.
- Berg, G., & Schrader, J. (2012). Access to credit, natural disasters, and relationship lending. Journal of Financial Intermediation, 21(4), 549–568. https://doi.org/10.1016/j.jfi.2012.05.003
- Bernanke, B. S., & Blinder, A. S. (1992). The federal funds rate and the channels of monetary transmission. American Economic Review, 82(4), 901–921.
- Boivin, J., & Giannoni, M. P. (2006). Has monetary policy become more effective? Review of Economics and Statistics, 88(3), 445–462. https://doi.org/10.1162/rest.88.3.445
- Boneva, L., Ferrucci, G., & Mongelli, F. P. (2022). Climate change and central banks: What role for monetary policy? Climate Policy, 22(6), 770–787. https://doi.org/10.1080/14693062.2022.2070119
- Böser, F., & Senni, C. (2020). Emission-based interest rates and the transition to a low-carbon economy. Economics Working Paper Series No. 20/337.
- Burke, M., Hsiang, S. M., & Miguel, E. (2015). Global non-linear effect of temperature on economic production. Nature, 527(7577), 235–239. https://doi.org/10.1038/nature15725
- Caggiano, G., Castelnuovo, E., & Groshenny, N. (2014). Uncertainty shocks and unemployment dynamics in U.S. recessions. Journal of Monetary Economics, 67, 78–92. https://doi.org/10.1016/j.jmoneco.2014.07.006
- Campiglio, E. (2016). Beyond carbon pricing: The role of banking and monetary policy in financing the transition to a low-carbon economy. Ecological Economics, 121, 220–230. https://doi.org/10.1016/j.ecolecon.2015.03.020
- Carleton, T. A., & Hsiang, S. M. (2016). Social and economic impacts of climate. Science, 353(6304). https://doi.org/10.1126/science.aad9837
- Chan, Y. T. (2020). Are macroeconomic policies better in curbing air pollution than environmental policies? A DSGE approach with carbon-dependent fiscal and monetary policies. Energy Policy, 141, 111454. https://doi.org/10.1016/j.enpol.2020.111454
- Chen, H., Chow, K., & Tillmann, P. (2017). The effectiveness of monetary policy in China: Evidence from a qual VAR. China Economic Review, 43, 216–231. https://doi.org/10.1016/j.chieco.2017.02.006
- Chen, K., Jue, R., & Tao, Z. (2018). The nexus of monetary policy and shadow banking in China. American Economic Review, 108(12), 3891–3936. https://doi.org/10.1257/aer.20170133
- Chen, S., Oliva, P., & Zhang, P. (2018). Air pollution and mental health: Evidence from China. NBER Working Papers No. 24686.
- Chen, C., Pan, D., Huang, Z., & Bleischwitz, R. (2021). Engaging central banks in climate change? The mix of monetary and climate policy. Energy Economics, 103, 105531. https://doi.org/10.1016/j.eneco.2021.105531
- Copeland, B. R., & Taylor, M. S. (2004). Trade, growth, and the environment. Journal of Economic Literature, 42(1), 7–71. https://doi.org/10.1257/.42.1.7
- Cover, J. P. (1992). Asymmetric effects of positive and negative money-supply shocks. The Quarterly Journal of Economics, 107(4), 1261–1282. https://doi.org/10.2307/2118388
- Dafermos, Y., Nikolaidi, M., & Galanis, G. (2018). Climate change, financial stability and monetary policy. Ecological Economics, 152, 219–234. https://doi.org/10.1016/j.ecolecon.2018.05.011
- Dell, M., Jones, B. F., & Olken, B. A. (2009). Temperature and income: Reconciling new cross-sectional and panel estimates. American Economic Review, 99(2), 198–204. https://doi.org/10.1257/aer.99.2.198
- Dell, M., Jones, B. F., & Olken, B. A. (2012). Temperature shocks and economic growth: Evidence from the last half century. American Economic Journal Macroeconomics, 4(3), 66–95. https://doi.org/10.1257/mac.4.3.66
- Deschênes, O., & Moretti, E. (2009). Extreme weather events, mortality, and migration. Review of Economics and Statistics, 91(4), 659–681. https://doi.org/10.1162/rest.91.4.659
- Dikau, S., & Volz, U. (2021). Central bank mandates, sustainability objectives and the promotion of green finance. Ecological Economics, 184, 107022. https://doi.org/10.1016/j.ecolecon.2021.107022
- Donadelli, M., Jüppner, M., Riedel, M., & Schlag, C. (2017). Temperature shocks and welfare costs. Journal of Economic Dynamics and Control, 82, 331–355. https://doi.org/10.1016/j.jedc.2017.07.003
- Engle, N., Brandon, C., Hallegatte, S., Hannam, P., & Heine, D. (2018). Strategic use of climate finance to maximize climate action: A guiding framework. World Bank.
- Ferraresi, T., Roventini, A., & Fagiolo, G. (2015). Fiscal policies and credit regimes: A TVAR approach. Journal of Applied Econometrics, 30(7), 1047–1072. https://doi.org/10.1002/jae.2420
- Florio, A. (2004). The asymmetric effects of monetary policy. Journal of Economic Surveys, 18(3), 409–426. https://doi.org/10.1111/j.0950-0804.2004.0226.x
- George, E., & Anastasios, X. (2018). Monetary policy under climate change. CESifo Working Paper, No. 7021.
- Ghysels, E., Santa-Clara, P., & Valkanov, R. (2006). Predicting volatility: Getting the most out of return data sampled at different frequencies. Journal of Econometrics, 131(1–2), 59–95. https://doi.org/10.1016/j.jeconom.2005.01.004
- Ghysels, E., Sinko, A., & Valkanov, R. (2007). MIDAS regressions: Further results and new directions. Econometric Reviews, 26(1), 53–90. https://doi.org/10.1080/07474930600972467
- Giglio, S., Kelly, B., & Stroebel, J. (2021). Climate finance. Annual Review of Financial Economics, 13(1), 15–36. https://doi.org/10.1146/annurev-financial-102620-103311
- Hansen, B. E. (1999). Testing for linearity. Journal of Economic Surveys, 13(5), 551–576. https://doi.org/10.1111/1467-6419.00098
- Hansen, L. P. (2022). Central banking challenges posed by uncertainty climate change and natural disasters. Journal of Monetary Economics, 125, 1–15. https://doi.org/10.1016/j.jmoneco.2021.09.010
- Heryan, T., & Tzeremes, P. G. (2017). The bank lending channel of monetary policy in EU countries during the global financial crisis. Economic Modelling, 67, 10–22. https://doi.org/10.1016/j.econmod.2016.07.017
- Hong, H., Li, F., & Xu, J. (2019). Climate risks and market efficiency. Journal of Econometrics, 208(1), 265–281. https://doi.org/10.1016/j.jeconom.2018.09.015
- Hosono, K., Miyakawa, D., Uchino, T., Hazama, M., Ono, A., Uchida, H., & Uesugi, L. (2016). Natural disasters, damage to banks, and firm investment. International Economic Review, 57(4), 1335–1370. https://doi.org/10.1111/iere.12200
- Hou, X. H., Li, S., & Wang, Q. (2018). Financial structure and income inequality: Evidence from China. Emerging Markets Finance and Trade, 54(2), 359–376. https://doi.org/10.1080/1540496X.2017.1347780
- Hsiang, S. M. (2010). Temperatures and cyclones strongly associated with economic production in the Caribbean and Central America. Proceedings of the National Academy of Sciences of the United States of America, 107(35), 15367–15372. https://doi.org/10.1073/pnas.1009510107
- Jörg, S. (2020). Risk, asset pricing and monetary policy transmission in Europe: Evidence from a threshold-VAR approach. Journal of International Money and Finance, 109(2), 102235. https://doi.org/10.1016/j.jimonfin.2020.102235
- Kahn, M. E., Mohaddes, K., Ng, R. N. C., Pesaran, M. H., Raissi, M., & Yang, J. (2021). Long-term macroeconomic effects of climate change: A cross-country analysis. Energy Economics, 104, 105624. https://doi.org/10.1016/j.eneco.2021.105624
- Kahn, S., Peng, Z., & Li, Y. (2019). Energy consumption, environmental degradation, economic growth and financial development in globe: Dynamic simultaneous equations panel analysis. Energy Reports, 5, 1089–1102. https://doi.org/10.1016/j.egyr.2019.08.004
- Kashyap, A. K., & Stein, J. C. (1995). The impact of monetary policy on bank balance sheets. Carnegie Rochester Conference Series on Public Policy, 42, 151–195. https://doi.org/10.1016/0167-2231(95)00032-U
- Kerssenfischer, M. (2019). The puzzling effects of monetary policy in VARs: Invalid identification or missing information? Journal of Applied Econometrics, 34(1), 18–25. https://doi.org/10.1002/jae.2647
- Klingelhöfer, J., & Sun, R. (2019). Macroprudential policy, central banks and financial stability: Evidence from China. Journal of International Money and Finance, 93, 19–41. https://doi.org/10.1016/j.jimonfin.2018.12.015
- Klomp, J. (2014). Financial fragility and natural disasters: An empirical analysis. Journal of Financial Stability, 13, 180–192. https://doi.org/10.1016/j.jfs.2014.06.001
- Kollmann, R., Enders, Z., & Müller, G. (2010). Global banking and international business cycles. European Economic Review, 55(3), 407–426. https://doi.org/10.1016/j.euroecorev.2010.12.005
- Koop, G., Pesaran, M., & Potter, S. (1996). Impulse response analysis in nonlinear multivariate models. Journal of Econometrics, 74(1), 119–147. https://doi.org/10.1016/0304-4076(95)01753-4
- Letta, M., & Tol, R. S. J. (2019). Weather, climate and total factor productivity. Environmental and Resource Economics, 73(1), 283–305. https://doi.org/10.1007/s10640-018-0262-8
- Li, H., Ni, J., Xu, Y., & Zhan, M. (2021). Monetary policy and its transmission channels: Evidence from China. Pacific-Basin Finance Journal, 68, 101621. https://doi.org/10.1016/j.pacfin.2021.101621
- Li, R., & Tian, X. (2018). Identifying the interdependence between monetary policy and financial stress: Evidence from China. Pacific Economic Review, 23(3), 411–425. https://doi.org/10.1111/1468-0106.12174
- Lo, M., & Zivot, E. (2001). Threshold cointegration and nonlinear adjustment to the law of one price. Macroeconomic Dynamics, 5(4), 533–576. https://doi.org/10.1017/S1365100501023057
- Maestre-Andrés, S., Drews, S., & Bergh, J. (2019). Perceived fairness and public acceptability of carbon pricing: A review of the literature. Climate Policy, 19(9), 1186–1204. https://doi.org/10.1080/14693062.2019.1639490
- McConnell, A., Yanovski, B., & Lessmann, K. (2022). Central bank collateral as a green monetary policy instrument. Climate Policy, 22(3), 339–355. https://doi.org/10.1080/14693062.2021.2012112
- McKibbin, W. J., Morris, A. C., Wilcoxen, P. J., & Panton, A. J. (2021). Climate change and monetary policy: Issues for policy design and modelling. Oxford Review of Economic Policy, 36(3), 579–603. https://doi.org/10.1093/oxrep/graa040
- Mishkin, F. S. (1996). The channels of monetary transmission: Lessons for monetary policy. NBER Working Paper No. 5464.
- Mishkin, F. S. (2001). The transmission mechanism and the role of asset prices in monetary policy. NBER Working Paper No. w8617.
- Pankratz, N., Bauer, R., & Derwall, J. (2023). Climate change, firm performance, and investor surprises. Management Science, 69(12), 7352–7398. https://doi.org/10.1287/mnsc.2023.4685
- Pool, S., Haan, L., & Jacobs, J. (2015). Loan loss provisioning, bank credit and the real economy. Journal of Macroeconomics, 45(2), 124–136. https://doi.org/10.1016/j.jmacro.2015.04.006
- Rozenberg, J., Hallegatte, S., Perrissin-Fabert, B., & Hourcade, J. C. (2013). Funding low-carbon investments in the absence of a carbon tax. Climate Policy, 13(1), 134–141. https://doi.org/10.1080/14693062.2012.691222
- Russell, S. V., Scott, A., Mike, S., Imke, D., Matthew, J. M., Claude, N. W. J., Chris, F., Karin, G., & Derek, A. (2014). Improved historical temperature and precipitation time series for U.S. climate divisions. Journal of Applied Meteorology and Climatology, 53(5), 1232–1251. https://doi.org/10.1175/JAMC-D-13-0248.1
- Said, S. E., & Dickey, D. A. (1984). Testing for unit roots in autoregressive-moving average models of unknown order. Biometrika, 71(3), 599–607. https://doi.org/10.1093/biomet/71.3.599
- Sandra, B., Rhiannon, S., & Misa, T. (2016). Let’s talk about the weather: The impact of climate change on central banks. Bank of England Working Paper No. 603.
- Seppänen, O., Fisk, W. J., & Lei, Q. H. (2006). Effect of temperature on task performance in office environment. Lawrence Berkeley National Laboratory.
- Somanathan, E., Somanathan, R., Sudarshan, A., & Tewari, M. (2021). The impact of temperature on productivity and labor supply: Evidence from Indian manufacturing. Journal of Political Economy, 129(6), 1797–1827. https://doi.org/10.1086/713733
- Song, X., & Fang, T. (2023). Temperature shocks and bank systemic risk: Evidence from China. Finance Research Letters, 51, 103447. https://doi.org/10.1016/j.frl.2022.103447
- Tsay, R. S. (1998). Testing and modeling multivariate threshold models. Journal of the American Statistical Association, 93(443), 1188–1202. https://doi.org/10.1080/01621459.1998.10473779
- Walsh, C. E. (2017). Monetary theory and policy (4th ed.). MIT Press Books.
- Zheng, S., Wang, J., Sun, C., Zhang, X., & Kahn, M. E. (2019). Air pollution lowers Chinese urbanites’ expressed happiness on social media. Nature Human Behaviour, 3(3), 237. https://doi.org/10.1038/s41562-018-0521-2
- Zivin, J., Hsiang, S. M., & Neidell, M. J. (2015). Temperature and human capital in the short- and long-run. NBER Working Papers.