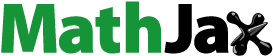
ABSTRACT
This paper uses the Bayesian model averaging approach to investigate the effects of trade openness on environmental quality in 64 selected developing countries for the period of 2003–2017. We find no evidence of a statistically significant effect of trade openness on environmental pollution in our sampled developing countries; however, our results show weak support for the pollution haven hypothesis. Besides trade openness, our findings indicate that financial openness, renewable energy consumption, and capital abundance are essential drivers of environmental quality, which appear to reduce carbon dioxide (CO2) emissions. In contrast, inward foreign direct investment and income harm environmental quality in the context of the developing countries studied. Based on our overall results, this paper suggests some strong policy recommendations for improving the environmental quality to achieve sustainable development under the background of a low-carbon economy in these nations.
1. Introduction
International trade could be one of the most crucial factors explaining environmental quality (Antweiler et al., Citation2001; Liddle, Citation2001; Atici, Citation2009, Citation2012; Shahbaz, Nasreen, et al., Citation2016; Mutascu, Citation2018; etc.). Trade increases the size of the economy leading to an increase in pollution, and hence trade, ceteris paribus, is the principal cause of environmental degradation (A. Harrison, Citation1995; Rock, Citation1996; Tobey, Citation1990). However, Birdsall and Wheeler (Citation1993), Jones and Manuelli (Citation1995), and Lee and Roland-Holst (Citation1997) suggest that environmental damage is linked to trade, but is not caused by trade. Due to the scale effect (through an increase in the size of the economy originating from increased trade volume), environmental quality could decline. However, trade could enhance the environmental quality via the technique effect and/or composition effect (i.e., as trade increases, income leading to environmental regulation and supervision is tightened). The production of pollution-intensive goods in one nation causes environmental pollution reductions while increasing pollution in other nations through international trade. This composition effect is attributed to two related hypotheses: the displacement hypothesis and the pollution haven hypothesis. The displacement hypothesis anticipates that openness or trade liberalisation may lead to the faster growth of pollution-intensive goods industries in poor countries as rich countries enforce stringent environmental regulation and supervision (A. Harrison, Citation1995; Rock, Citation1996; Tobey, Citation1990). The pollution haven hypothesis refers to the possibility that multinational enterprises with highly polluting operations migrate to nations with weaker environmental standards (Antweiler et al., Citation2001; Cole & Elliott, Citation2003). Fundamentally, there is no difference between these two hypotheses regarding comparative advantage in international trade. Given that trade relates one country with international communities, an underdeveloped economy could rely on technology transfer via foreign direct investment (FDI), which may reduce environmental pollution. Therefore, free trade has contradictory influences on environmental quality, both positive and negative.
So does trade liberalisation harm or benefit the environment? More specifically, does trade liberalisation increase or reduce carbon dioxide (CO2) emissions in the context of developing countries? Is the pollution haven hypothesis valid in these nations? The main objective of this paper is therefore to examine whether environmental pollution arising from trade openness in developing nations over the period 2003–2017. The contribution of this paper is the relevant literature in several aspects: first, differing from Antweiler et al. (Citation2001), Cole and Elliott (Citation2003), and Managi et al. (Citation2009), we extend their works on control variables by considering financial openness (Aydin & Turan, Citation2020; Koengkan et al., Citation2019; You et al., Citation2015), renewable energy consumption (Bilgili et al., Citation2016; Jebli et al., Citation2016; Sebri & Ben-Salha, Citation2014; Shafiei & Salim, Citation2014; Zafar et al., Citation2020), legal origins (Kock & Min, Citation2016), and regional dummy variables. Second, there is a persistent scarcity of relevant studies by the inclusion of such control variables, especially in developing countries. Including these variables in our analysis allows us to critically examine the effects of trade openness on environmental quality with a special focus on these countries. Finally, very few studies use approaches based on Bayesian statistics and ignore model uncertainty, leading to over-confident inferences, “all-or-nothing” constraint, and the presence of omitted variable bias that generalise poorly (Chipman et al., Citation2001; Fragoso et al., Citation2018; Hinne et al., Citation2020; Hoeting et al., Citation1999a; Raftery, Citation1993; Raftery et al., Citation1997, Citation2005). To our best knowledge, this is the first attempt that employs a Bayesian Model Averaging (BMA) approach to consider model uncertainty to investigate the effects of trade openness on environmental quality for a sample of developing countries. Several seminal studies proposed this approach because of the advantages of using the BMA mentioned above, such as Raftery (Citation1993), Raftery et al. (Citation1997, Citation2005), and Hoeting et al. (Citation1999b), etc. Therefore, this paper aims to fill this gap in the econometrics literature.
In this paper, we examine the effects of trade openness on CO2 emissions in 64 selected developing countries over the period 2003–2017 using the BMA approach to account for model uncertainty issues with 21 different potential regressors. Our main findings point out that although the relationship between trade openness and CO2 emissions is not found to be statistically significant, the interaction between trade openness and relative capital abundance has a significant and positive effect on CO2 emissions. Interestingly, in contrast to trade openness, empirical evidence indicates the importance of financial openness, renewable energy consumption, and capital abundance in improving environmental quality, whereas income and inward FDI stock to the domestic capital stock have negative effects on CO2 emissions in our sampled developing countries. Furthermore, our results show weak support for the pollution haven hypothesis but do not confirm the existence of an EKC in the case of these nations.
The remainder of the paper is structured as follows. Section 2 includes a literature review. Section 3 explains the variables, methodology, and data sources used. Section 4 reports the empirical findings and their discussions. Finally, Section 5 presents the conclusions and some policy suggestions.
2. Literature review
In the past few decades, several empirical studies used different statistical and econometrics models to investigate the relationship between trade openness and environmental indicators (CO2 emissions, sulfur dioxide (SO2) emissions, fossil fuels, greenhouse gases, etc.). Nevertheless, their findings are inconsistent.
On the one hand, trade openness is a vital factor that could positively influence environmental quality. For instance, Antweiler et al. (Citation2001) extend their work on 43 countries over the period 1971–1996 and follow the scale, technique, and composition effects of Grossman and Krueger (Citation1991) using SO2 emissions as a proxy variable for environmental degradation. For an average country in their sample, they find that the full effect of trade openness reduces SO2 concentrations. Atici (Citation2009) investigates the influences of GDP, energy use, and trade openness on CO2 emissions utilising the extended environmental Kuznets curve (EKC) with panel data from 1980 to 2002 in the Central and Eastern European countries. The findings provide some evidence for the existence of an EKC. When GDP increases, CO2 emissions decrease over time. The author indicates that globalisation does not increase CO2 emission levels through the trade openness variable in the region. Frankel and Rose (Citation2005) investigate the endogeneity of trade employing instrumental variables with exogenous geographic determinants of trade. The results show that trade tends to reduce air pollution statistically significantly via SO2 emissions concentrations and moderate NO2 emissions, but lacking for particulate matter. However, they suggest that there is little evidence to support that trade hurts the environment.
On the other hand, trade openness may also have a negative effect on environmental quality. For example, Managi (Citation2004) examines the relationship between trade liberalisation and environmental quality using a panel dataset of 63 developed and developing countries over the period 1960–1999. The results show that trade liberalisation has detrimental effects on the environment. Moreover, income expansion may attenuate the degradation of the environment. Ang (Citation2009) suggests that CO2 emissions are negatively associated with technology transfer, research intensity, and the absorptive capacity to assimilate foreign technology in the Chinese economy by utilising the autoregressive distributed lag (ARDL) estimator. They also provide evidence that energy use, income, and trade openness positively affect CO2 emissions. Using cointegration techniques, Chebbi et al. (Citation2011) investigate short-run and long-run relationships between trade openness, capita real GDP, and CO2 emissions between 1961 and 2004 in Tunisia. They argue that trade openness has a positive direct effect on CO2 emissions both in the short-run and the long-run, but a negative indirect effect occurs at least in the long run. Nevertheless, the overall effect is positive both in the short-run and the long-run, which suggests that trade openness contributes to environmental pollution in Tunisia. This highlights the importance of trade reforms along with strong environmental policies. Employing cross-country panel data, Le et al. (Citation2016) investigate the link between trade openness and the environment by utilising particulate matter emissions as a proxy variable for environmental quality. According to the panel cointegration test, they provide significant evidence of a long-run link between particulate matter emissions and trade openness. In particular, increased trade openness might lead to environmental degradation across the global sample, but this depends on a country’s income level. Trade openness harms the environment in middle-income and low-income countries but has a benign impact in high-income countries. Applying the panel cointegration tests from Pedroni (Citation1999) and Westerlund (Citation2005), Shahbaz, Nasreen, et al. (Citation2016) investigate the link between trade openness and CO2 emissions using data from 105 countries where they find that trade openness reduces environmental quality among countries, but the impact varies widely according to each group of countries.
Furthermore, several studies argue that trade openness has an effect, either positive or negative, on environmental quality depending on developed and less-developed economies or different environmental indicators. For example, by adopting the same methodology as Antweiler et al. (Citation2001), Cole and Elliott (Citation2003) use data on emissions and pollution intensities instead of air and water pollutants data. The results provide clear evidence supporting both the pollution haven and factor endowment hypotheses in CO2 emissions and SO2 emissions but not in biochemical oxygen demand (BOD) emissions and nitrogen oxides (NOx) emissions. For emissions data, these authors argue that trade liberalisation may reduce emissions per capita in BOD, but the outcome is uncertain regarding SO2 emissions by adding up all the effects. However, trade liberalisation and emissions have a positive relationship in NOx emissions and CO2 emissions. On the other hand, for pollution intensities data, they suggest that trade liberalisation may reduce the pollution intensity of the output of all four pollutants. Considering trade and income variables as endogenous, Managi et al. (Citation2008) estimate the overall effect of trade openness on the quality of the environment utilising the instrumental variables technique. After the dynamic adjustment process, the authors find that the effect is large in the long term but small in the short term. Specifically, trade openness may benefit the environment in OECD countries. However, in selected non-OECD countries, it negatively affects SO2 and CO2 emissions while reducing BOD emissions. Besides, trade openness affects emissions via environmental regulation and capital-labour effects. By employing a different estimation technique, Managi et al. (Citation2009) re-investigate the trade – environment link for the OECD and non-OECD countries with SO2 emissions and CO2 emissions. The results are similar to the findings of Cole and Elliott (Citation2003) in that trade openness may benefit the environment in OECD countries. Baek et al. (Citation2009) argue that trade and income positively affect environmental quality in developed economies. However, the results provide clear evidence that trade harms environmental quality in most developing economies. Dirty industry migration is causing serious concern for poor and less developed economies, which are growingly bearing the pollution burdens of consumption in rich and developed economies. Employing time series data over the period 1971–2006 from China, Korea, and Japan, Choi et al. (Citation2010) consider the existence of the EKC by CO2 emissions and its causal links with openness and economic growth. The environmental consequences and the estimated EKC are inconsistent depending on the national characteristics. Japan shows a U-shaped curve, while China has an N-shaped curve. Such dissimilarities are also found in the relationship between CO2 emissions and openness. In contrast, regarding the link between CO2 emissions and openness, China presents a U-shaped curve, while Korea and Japan show an inverted U-shaped curve. Using a vector autoregression and a vector error correction model, their findings provide evidence for large heterogeneity among variables and countries. Nasir and Rehman (Citation2011) examine the links among CO2 emissions, energy consumption, income, and foreign trade in Pakistan from 1972 to 2008 by using the Johansen’s cointegration method. The authors indicate that CO2 emissions and income have a quadratic long-run relationship, supporting the existence of the EKC hypothesis in Pakistan. In addition, both foreign trade and energy consumption can positively affect emissions. However, they find no evidence to support the existence of the EKC hypothesis in the short run. Atici (Citation2012) analyses the interaction between the environment and trade in terms of CO2 emissions for ASEAN countries by adopting panel data over the period 1970–2006. The findings illustrate that CO2 emissions exhibit an inverted S-shaped curve in this region. In particular, exports play a crucial role in CO2 emissions in ASEAN countries, showing no evidence that trade positively affects environmental quality. In addition, the imports of Japan from the region probably do not cause pollution, while the imports of China can encourage pollution per capita.
Lastly, a few studies show that trade openness is not associated with environmental quality. For instance, Frankel (Citation2009) investigates whether globalisation has been deteriorating environmental quality. Empirical evidence from cross-country data generally determines that trade has no damaging effects on some indicators of environmental degradation, such as SO2 air pollution, after controlling for income. Hence, globalisation and environmental quality do not necessarily lead to conflict. Using time-series data of 1975–2005 and the autoregressive distributed lag (ARDL) methodology, Jalil and Mahmud (Citation2009) analyse the long-run relationships among CO2 emissions, energy consumption, real GDP, and foreign trade in China. The results show a quadratic relationship between CO2 emissions and real GDP, thus supporting the EKC hypothesis. Interestingly, there is one-way causality running from economic growth to CO2 emissions via Granger causality tests. The findings suggest that real GDP and energy consumption largely determine CO2 emissions in the long-run trends. Foreign trade has a positive and statistically insignificant influence on CO2 emissions. Kearsley and Riddel (Citation2010) examine the EKC literature that focuses primarily on the role of international trade in shaping this relationship. Specifically, they test the pollution haven hypothesis, which argues that emission reductions observed among developed countries are partly the consequence of shifting dirty good production to developing countries with relatively lax environmental standards and regulations. These authors find little empirical evidence that pollution havens play a crucial role in shaping the EKC. This finding does not support the view that economic growth improves environmental quality. Using the wavelet tool, Mutascu (Citation2018) examines the comovement between CO2 emissions and trade openness in France over the period 1960–2013. The main findings reveal that there is no comovement between trade openness and gas emissions at high frequency, supporting the neutral hypothesis in the short term. However, the business cycle, in the long term, drives the interaction between CO2 emissions and trade openness.
Generally, most of the existing literature on this issue investigates the effects of free trade on environmental pollution while controlling other relevant variables by using classical approaches based on traditional or frequentist statistics (Antweiler et al., Citation2001; Cole & Elliott, Citation2003; Frankel & Rose, Citation2005; Le et al., Citation2016). However, there is a lack of empirical studies which employ methods based on Bayesian statistics. Moreover, to our knowledge, very little attention has been paid to this topic to address the issue of model uncertainty, especially in a wide variety of competing theories and several possible determinants. Thus, BMA may provide a better framework to deal with such problems (Leamer, Citation1978; Madigan & Raftery, Citation1994; Raftery, Citation1988). Additionally, the obvious rationale for selecting developing countries for our analysis is that the increasing greenhouse gases emissions are a key threat of global warming. The ongoing climate change has been a crucial concern to all societies over the last two decades, especially in developing countries facing serious challenges of environmental pollution (see Appendix A).
3. Methodology and data
This section briefly gives some motivations for BMA and provides an intuitive overview of its central ideas. We then discuss views of trade openness and its possible impact on environmental quality, while controlling other relevant variables. Finally, we provide a summary of the data sources included in our analysis.
3.1. Estimation strategy
According to Fragoso et al. (Citation2018), the BMA methodology has been developed as an extension to Thomas Bayes’s Bayesian inference approach. BMA can be used not only for parameter uncertainty estimation via the prior distribution but also for model uncertainty estimation via the posterior distribution. Therefore, it is undeniable that BMA is now widely accepted and increasingly being used by different fields of study as a principled way to take into account model uncertainty (Chipman et al., Citation2001; Fragoso et al., Citation2018; Hoeting et al., Citation1999b; Raftery et al., Citation1997). In addition, as argued convincingly by Hinne et al. (Citation2020), there are several advantages of the BMA framework compared to the selection of a single model: (i) reducing the overconfidence regarding the emerging issue of model uncertainty ignorance; (ii) predicting optimal results under several loss functions (e.g., squared error, logarithmic) that these errors are alleviated by BMA; (iii) avoiding the “all-or-nothing” mentality relating to classical hypothesis testing, that whole model is either accepted or rejected; (iv) gracefully reducing the variance of the estimated parameter across experiments; (v) being relatively robust to model misspecification; (vi) substantially reducing the possibility of omitted variable bias.
The BMA methodology is conducted in the context of linear regression, e.g., in Hoeting et al. (Citation1999a), Fernandez et al. (Citation2001a,Citation2001b), Feldkircher and Zeugner (Citation2009), and Ley and Steel (Citation2009), among others. We start from a linear regression model:
where denotes the CO2 emissions (metric tons per capita) over a particular period as a proxy measure for environmental quality;
is the constant term in the regression;
is a set of trade variables;
is a set of additional control variables; and
is the error term, assumed to have homoscedastic, normally distributed disturbances. In our model, we have
developing countries and a set of
potential explanatory variables.
Consider a set of K possible different models that can be denoted by , where M stands for the model space. One of its regular elements (
) can be described in the following manner:
where and
denote the vectors of unknown population parameters and sample observations for Y, respectively;
is the joint probability density function of
given y in jth-order model,
;
is the likelihood function of model
.
As suggested by Wasserman (Citation2000), we use model averaging techniques that estimate the posterior distributions of the quantity of interest under each possible model, a weighted average of their posterior model probabilities. According to BMA methodology, inference on any parameteracross the model space takes the form:
wheredenotes posterior distributions under models
;
is posterior distributions under the assumption that
is the true model conditional on the observed data;
is the posterior probability of model
.
Based on Bayes’ rule, the posterior probability of modelis given by:
wheredenotes the integrated likelihood of model
;
is the model prior; and
is the parameter prior.
Next, BMA methodology obtains the posterior inclusion probability (PIP) of each explanatory variable by summing the posterior model probabilities of all models across all models that include this particular variable. PIP can be written as:
with presents that the variable k is contained in the model.
This paper uses Markov Chain Monte Carlo Model Composition (MC3) algorithms to examine the model space stochastically, as suggested by Madigan et al. (Citation1995). We run the estimation with 1,000,000 burn-ins and 3,000,000 draws of the MC3 sampler. In such cases, three criteria for parameter prior are utilised to select the best-fit model: (i) unit information prior (UIP) (Fernandez et al., Citation2001a, Citation2001b; Kass & Wasserman, Citation1995); (ii) benchmark prior (BRIC) (Fernandez et al., Citation2001a, Citation2001b); (iii) local empirical Bayes approach (LEB) prior (George & Foster, Citation2000; Liang et al., Citation2008). Additionally, the priors over the models are assumed to be uninformative in the context of its application (here are the effects of trade openness on environmental quality). Further details on BMA, in general, can be found elsewhere, for example, Raftery et al. (Citation1997).
As mentioned previously, the BMA approach uses all possible models to examine the robustness of a large set of possible determinants of environmental quality. We briefly outline the theories linking trade openness to environmental quality and 21 potential explanatory variables in what follows.
Trade openness: More openness to trade is likely the result of more competition, leading to the exit from the market of the less energy-efficient and less productive firms. However, several studies postulate that the influence of trade liberalisation on environmental quality can decompose into three effects: scale (size of the economy), composition (specialisation), and technique (production methods) (Antweiler et al., Citation2001; Cole & Elliott, Citation2003). The technique effect likely brings beneficial environmental outcomes, while the scale effect might generate greater pollution. The composition effect is dependent on the comparative advantage of a country. Therefore, the net effect of trade openness on environmental quality depends on each opposing force’s relative strength, ultimately leading to an empirical issue. As for trade openness indicator, we use the sum of imports and exports over GDP to measure the degree of trade openness (Ang, Citation2009; Antweiler et al., Citation2001; Atici, Citation2009; Baek et al., Citation2009; Chebbi et al., Citation2011; Choi et al., Citation2010; Cole & Elliott, Citation2003; Frankel, Citation2009; Jalil & Mahmud, Citation2009; Kearsley & Riddel, Citation2010; Le et al., Citation2016; Managi, Citation2004, Citation2008; Managi et al., Citation2009; Mutascu, Citation2018; Nasir & Rehman, Citation2011; Shahbaz, Nasreen, et al., Citation2016).
Environmental quality: The effects of trade openness on environmental quality depend on different methods of pollution measurement in terms of concentrations or emissions. Both concentrations and emissions data have advantages and disadvantages (Cole & Elliott, Citation2003). In this paper, we use the most abundant greenhouse gas, CO2, to measure environmental quality (Ang, Citation2009; Atici, Citation2009, Citation2012; Chebbi et al., Citation2011; Choi et al., Citation2010; Frankel, Citation2009; Jalil & Mahmud, Citation2009; Le et al., Citation2016; Managi et al., Citation2009; Mutascu, Citation2018; Nasir & Rehman, Citation2011; Shahbaz, Nasreen, et al., Citation2016). The authors choose this emissions type as a proxy for environmental quality because of its influence on global warming and overall climate change (Nordhaus, Citation1991) and its sufficient data in the context of developing countries. According to the Intergovernmental Panel on Climate Change (IPCC), “carbon dioxide (CO2) is the most important anthropogenic [greenhouse gas]” (IPCC, Citation2007, pp. 36). Fossil fuel combustion and emissions from industrial production are the primary sources of anthropogenic CO2 emissions (EPA, Citation2019).
Control variables
Financial openness: Based on the pioneering contributions of Goldsmith (Citation1969), McKinnon (Citation1973), and Shaw (Citation1973), a relationship between financial liberalisation and economic growth was established. In addition, trade liberalisation often accompanies financial liberalisation by increased FDI capital and research and development investments, which in turn can promote economic growth (Frankel & Romer, Citation1999), influencing environmental quality (Tamazian & Rao, Citation2010; Tamazian et al., Citation2009). On the other hand, if trade openness and financial openness lead to financial development (Baltagi et al., Citation2007; Chinn & Ito, Citation2002; Rajan & Zingales, Citation2003), it can promote economic growth. Hence, it follows that trade and financial openness also affect environmental performance (Aydin & Turan, Citation2020; Koengkan et al., Citation2019; You et al., Citation2015). Therefore, assessing the effects of trade and financial openness on environmental quality will provide a more complete picture. We use the capital account openness index (KAOPEN) as the proxy for financial openness by Chinn and Ito (Citation2006) (David et al., Citation2014; Karimu & Marbuah, Citation2017; Law & Demetriades, Citation2006; Rajan & Zingales, Citation2003; Tamazian & Rao, Citation2010; Tamazian et al., Citation2009; Trabelsi & Cherif, Citation2017).
Inward FDI stock to domestic capital stock: Besides trade and financial openness, FDI as an economic openness indicator also plays a crucial role in determining environmental performance, and it is well-recognised in the existing literature (Atici, Citation2012; Cole et al., Citation2006; He, Citation2006). For instance, A. E. Harrison and Eskeland (Citation2002) provide evidence that foreign-owned plants in four developing countries use cleaner energy than domestic-owned plants. In this paper, FDI intensity is the percentage of inward FDI stock relative to a country’s entire capital stock. FDI intensity may be associated with cleaner production techniques (Antweiler et al., Citation2001; He, Citation2006).
Income: We use real gross national product (GNP) per capita as a proxy for income (Antweiler et al., Citation2001; Cole & Elliott, Citation2003). The income level may induce pollution; however, environmental degradation may decrease when income increases because of the higher level of environmental awareness and cleaner energy technologies (Antweiler et al., Citation2001; Cole & Elliott, Citation2003).
Renewable energy consumption: Energy is being recognised as an engine for economic growth and development. Renewable energy consumption is positively associated with living standards in most countries. On the other hand, renewable energy consumption is considered a sustainable solution for reducing greenhouse gases emissions, such as CO2, SO2, etc., while other forms of energy consumption influence the environment in various unfavourable ways. Thus, the relationship between renewable energy consumption and economic growth and the contributions of renewable energy consumption to environmental emissions have become popular in theoretical and empirical literature in recent years. Several works find that renewable energy consumption plays a crucial role in improving environmental quality (Sebri & Ben-Salha, Citation2014, Shafiei & Salim, Citation2014, Bilgili et al., Citation2016, Jebli et al., Citation2016, Khan et al., Citation2020, Zafar et al., Citation2020).
Legal origins: Since the seminal work of Porta et al. (Citation1998), the differences in legal origins and economic performance have received a great deal of attention in the literature (Beck, Citation2002; Glaeser & Shleifer, Citation2002; Porta et al., Citation2008). However, a few scholars have recently examined the effects of the legal origins on environmental performance (DiVita, Citation2009; Kock & Min, Citation2016). For instance, Kock and Min (Citation2016) provide evidence that the legal origins have a strong effect on CO2 emissions via the shaping of governance mechanisms, leading to the overall institutional logic that governs human behavior.
Other variables: Considering Antweiler et al. (Citation2001) and Cole and Elliott (Citation2003), we selected the following 13 control variables for environmental quality including capital – labour ratio (capital abundance) and its quadratic; the one-period lag of income and its quadratic; relative capital abundance; relative income; economic intensity and its quadratic; the interaction of trade openness with a country’s relative capital abundance; the interaction of trade openness with relative per capita income; the interaction of trade openness, relative capital abundance and relative income; the square of capital abundance; the interactive term between capital abundance and income, and time trend. In addition, this paper includes regional dummy variables to compare the levels of CO2 emissions across regions.
summarises the definitions of all variables.
Table 1. Information on variables.
3.2. Data sources
This paper employs panel data from 64 developing countries classified by the United Nations Development Programme (UNDP) between 2003 and 2017 (see Appendix B). Depending on data availability, data for the period 2003–2017 are the most complete for investigation of the effects of trade openness on environmental quality. The classification of the UNDP is based on the traditional multifaceted measure of the Human Development Index (HDI). For more details, provides the definitions and data sources of the variables employed in this paper.
4. Results and discussions
This section discusses the estimated findings employing the BMA approach as described above. BMA allows any subset of up to our 21 determinants of environmental quality to enter the regressions; hence, the model space consists of 221 (=2,097,152) different models. The results are generated from the Markov chain simulations of 20 million recorded draws after 10 million burn-ins, visiting 3,954,118 models. The posterior expected model size is 15.321 (i.e., the average number of included regressors). The BRIC, the UIP, and the LEB techniques are always utilised under the assumption of uninformative priors over the parameters within each model. Our estimates are based on a sample of 64 developing countries over the period of 2003 to 2017, where the dependent variable is CO2 emissions (metric tons per capita) as the proxy for environmental quality.
presents the results of the posterior estimates under uniform model priors, including posterior inclusion probability (PIP), posterior mean (Post mean), and posterior standard deviation (Post SD). As mentioned above, the prior probability of including a given variable in the true model is 0.5; hence, the variables are identified as robust determinants of environmental quality that have PIPs higher than 0.5. On the other hand, the variables have PIPs of less than 0.5 under all three alternative prior structures on parameters, implying that they have no significant effects on environmental quality in developing countries.
Table 2. The effects of trade openness on environmental quality: posterior estimates under uniform model priors.
demonstrates the posterior density of the coefficient on trade openness, confirming the positive but statistically insignificant effect of trade openness on environmental quality. The results suggest that the trade openness with a PIP of 0.033 exerts an insignificant effect on environmental quality. This figure presents the same PIP, marginal densities of trade openness from BRIC in response to environmental quality, and the averaged point estimates (the green solid vertical line) of the corresponding regressor, as shown in . The red solid vertical line and the red dotted lines indicate conditional expected values and the double conditional standard deviation, respectively.
This paper first considers the trade variables. As presented in , the coefficient attached to the average trade openness implies that a 1% increase in trade openness increases CO2 emissions by roughly 0% with a very low PIP (0.03). The results indicate that an increase in trade openness is not associated with increased CO2 emissions in developing countries, which is consistent with empirical work by Frankel (Citation2009), Jalil and Mahmud (Citation2009) and Kearsley and Riddel (Citation2010). This finding is inconsistent with Antweiler et al. (Citation2001), who find evidence that freer trade is good for the environment because of a consequence of higher demand for cleaner techniques of production. Next, we show that the interaction between trade openness and relative capital abundance is associated with the increase in the CO2 emissions. This interactive variable is not aligned with the prediction of Antweiler et al. (Citation2001), Managi et al. (Citation2009), and Cole and Elliott (Citation2003) for SO2 and CO2 emissions that a country with high relative capital abundance will find pollution falling in response to trade openness. However, this finding is consistent with Cole and Elliott (Citation2003) for NOx and BOD emissions. It means that a developing country with weak environmental regulation and supervision will find that freer trade leads to increased pollution because their comparative advantage in dirty production deepens. However, our results show weak support for the pollution haven hypothesis that rich countries with stringent regulation and supervision may lose the dirty industries while poor countries would get them all (Ang, Citation2009; Chebbi et al., Citation2011; Le et al., Citation2016; Managi, Citation2004; Managi et al., Citation2009; Nasir & Rehman, Citation2011). The paper shows a negative relationship between the square of relative capital abundance and CO2 emissions in response to trade openness, as Cole and Elliott (Citation2003) suggested for NOx and BOD emissions. As expected, this implies that an increase in relative capital abundance leads to a decrease in CO2 emissions with a diminishing marginal effect. The paper finds no evidence that an increase in relative income leads to an increase in CO2 emissions, and each additional increase in relative income has a diminishing impact, as Antweiler et al. (Citation2001), Cole and Elliott (Citation2003), and Managi et al. (Citation2009) argued. There is a negative relationship between CO2 emissions and trade openness interacting with the relative capital abundance and relative income, which is inconsistent with empirical work by Antweiler et al. (Citation2001) and Cole and Elliott (Citation2003). This finding is in line with Managi et al. (Citation2009), implying that the production of capital-intensive goods in developing countries with higher relative income increases, and the CO2 emissions decrease. In other words, a developing country with relatively strict environmental regulation is likely to have a more comparative advantage in relative capital accumulation (e.g., technological innovation, economies of scale) following increased trade openness, thus reducing CO2 emissions.
We can now turn to the non-trade variables. Interestingly, this paper finds substantial evidence in favour of financial openness as one of the most important determinants of environmental quality by reducing CO2 emissions in developing countries. This result indicates that financial liberalisation might increase in both FDI capital and research and development investments, which in turn can promote economic growth and hence improve environmental quality, as suggested by Tamazian et al. (Citation2009) and Tamazian and Rao (Citation2010). Compared to the French legal origin, our findings do not provide evidence for the significant role of the British legal origin in improving environmental quality, whereas the Socialist legal origin dummy mitigates the positive effects of trade liberalisation on environmental quality. The developing countries in Africa and Latin America and the Caribbean have a lower degree of environmental pollution compared to Asian developing countries. The paper provides evidence that an increase in capital abundance interacted with income improves environmental quality, as Antweiler et al. (Citation2001), Cole and Elliott (Citation2003), and Managi et al. (Citation2009) pointed out. Regarding the capital abundance (adjusted), our findings demonstrate that increases in capital abundance decrease CO2 emissions to a certain point, after which it starts harming environmental quality. These results suggest that the capital accumulation in developing countries promotes the technological process, therefore adopting cleaner production technologies. According to the findings, there is a positive relationship between inward FDI stock to domestic capital stock and CO2 emissions (Antweiler et al., Citation2001). Notably, CO2 emissions have positive time trends. This finding is consistent with Managi et al. (Citation2009), but it is not in line with Cole and Elliott (Citation2003). Similar to financial openness, a decrease in CO2 emissions causes a higher share of renewable energy consumption (Bilgili et al., Citation2016; Jebli et al., Citation2016; Sebri & Ben-Salha, Citation2014; Shafiei & Salim, Citation2014; Zafar et al., Citation2020). In contrast, this paper finds no evidence to suggest that the economic intensity, the square of economic intensity, and the square of income are significant determinants of environmental pollution. Our findings are inconsistent with Antweiler et al. (Citation2001) and Cole and Elliott (Citation2003). Finally, the empirical results also reveal that an increase in income causes higher CO2 emissions. Our empirical results do not support for the EKC that an inverted-U-shaped relationship exists between per capita income and pollution. These results could be explained by the fact that the consequences of environmental pollution depend not only on the income level, but also on the underlying source of economic growth because of the differentiating characteristics of developing countries compared to developed countries.
5. Conclusions
The environmental influence of trade openness has been vigorously debated over the past two decades. This paper aims to investigate the effects of trade openness on environmental quality by using CO2 emissions (metric tons per capita) as an indicator of environmental pollution in developing countries. The main contribution of this paper is the adoption of the regression model based on the BMA approach proposed by Fernandez et al. (Citation2001b) to consider model uncertainty for capturing the environmental consequence of trade openness that previous studies have not addressed this. Another important contribution is the inclusions of financial openness, legal origins, and renewable energy consumption as key determinants of environmental quality, which are not considered by the seminal works of Antweiler et al. (Citation2001) and Cole and Elliott (Citation2003). Since this paper addressed this, we believe our empirical findings are more holistic than any other relevant studies conducted in the context of developing countries. By adopting the BMA approach and using data from only developing countries, we find some empirical results that are significantly different from those of Antweiler et al. (Citation2001), Cole and Elliott (Citation2003), and Managi et al. (Citation2009). Therefore, this paper contributes to a better understanding of the characteristics of developing economies in our findings.
The empirical results of this paper additionally suggest that trade openness is not the significant determinant of environmental degradation in our sampled developing countries. However, we find that the pollution haven hypothesis does not have strong validity. The results further show an increase in relative capital abundance leading to a lower environmental quality in response to trade openness and increasing relative capital abundance has a diminishing marginal effect on CO2 emissions in response to trade openness. The paper provides evidence that the interaction between trade openness, relative capital abundance, and relative income has a negative effect on CO2 emissions. Besides trade variables, the paper provides strong evidence that financial openness, renewable energy consumption, and capital abundance play a significant role in improving environmental quality by reducing CO2 emissions, whereas income and inward FDI stock to domestic capital stock have negative effects on environmental quality. The results show that the effect of capital abundance on environmental quality as an inverted U-shaped curve. Lastly, we find that Asian and socialist developing countries seem to face more serious challenges of CO2 emissions compared to developing countries in other regions and legal origins, respectively.
Based on the above-mentioned main findings, this paper suggests some policy implications for developing countries, especially in Asian and socialist developing countries with more severe challenges in improving environmental quality. As proposed by O. J. Blanchard et al. (Citation2022, Citation2023), we adopt a holistic approach to addressing a wide variety of challenges of policy-making for improving environmental quality in the context of developing countries. First, international trade policies should be redesigned to accommodate changes in environmental protection policies to alleviate environmental damage and climate change impacts, such as green trade and carbon trading (e.g., European Carbon Border Adjustment Mechanism implementations as argued convincingly by O. J. Blanchard et al. (Citation2022, Citation2023)). Second, financial liberalisation policies should be considered one of the central tasks for improving environmental quality in developing countries, such as removing financial repression. However, the sequencing and timing of financial liberalisation policies and their content should be customised to meet each developing country’s situation. Third, developing countries’ governments continue to actively encourage renewable energy consumption by designing different policy tools, such as (i) reducing fossil fuel consumption, (ii) establishing competitive electricity markets, (iii) addressing sustainable energy transformation strategies, (iv) promoting climate finance, (v) stimulating intense R&D effort, innovation, and adoption for clean energy technologies, (vi) promoting a low-carbon economy (e.g., carbon pricing, carbon taxation, carbon credits). Fourth, foreign direct investment policies might be redesigned to strengthen environmental protection through clean energy technology transfer and green foreign direct investments. Fifth, as suggested in the Paris Protocol, Kyoto Protocol and the UN Climate Change Conferences (or COPs), developed countries should provide financial assistance to support effective adaptation and mitigation actions to the adverse effects of climate change in developing countries. Sixth, developing countries’ governments should carefully design the implementation strategies of environmental regulations to ensure the achievement of their environmental protection goals, such as the choice between command-and-control and market-based policies. Seventh, developing countries’ governments need to seriously consider ways to enhance individual and public awareness of environmental issues and promote environmental responsibility. Eighth, developing countries need to improve the effectiveness of international cooperation and learn from international experience in sustainable energy transition. Last but not least, developing countries should seek an effective balance between environmental protection and economic growth to achieve sustainable development, especially in fossil-fuel-driven economies.
Table R3.docx
Download MS Word (36.1 KB)Acknowledgments
We gratefully acknowledge the constructive and insightful recommendations of anonymous reviewers and Inmaculada Martinez-Zarzoso (the editor). We also wish to thank Bryan Weber (City University of New York) for his helpful support. Any remaining errors are the authors’ own responsibility.
Disclosure statement
No potential conflict of interest was reported by the author(s).
Supplementary Material
Supplemental data for this article can be accessed online at https://doi.org/10.1080/15140326.2024.2339610
Additional information
Funding
Notes on contributors
Diem Thi Thuy Pham
Diem Thi Thuy Pham, PhD, is a lecturer at Ho Chi Minh University of Banking (HUB), Viet Nam and is also serving for the Asian Journal of Economics and Banking (AJEB), which is owned by HUB. Her primary research interests are in the areas of banking and financial economics, as well as development economics.
Hoai Trong Nguyen
Hoai Trong Nguyen is a professor in economics at the University of Economics Ho Chi Minh (UEH) and currently holds the position of Editor-in-Chief for the Journal of Asian Business and Economic Studies (JABES), which is owned by UEH. His research focuses on development studies.
References
- Ang, J. B. (2009). CO2 emissions, research and technology transfer in China. Ecological Economics, 68(10), 2658–22. https://doi.org/10.1016/j.ecolecon.2009.05.002
- Antweiler, W., Copeland, B. R., & Taylor, M. S. (2001). Is free trade good for the environment? American Economic Review, 91(4), 877–908. https://doi.org/10.1257/aer.91.4.877
- Atici, C. (2009). Carbon emissions in central and Eastern Europe: Environmental kuznets curve and implications for sustainable development. Sustainable Development, 17(3), 155–160. https://doi.org/10.1002/sd.372
- Atici, C. (2012). Carbon emissions, trade liberalization, and the Japan–ASEAN interaction: A group-wise examination. Journal of the Japanese and International Economies, 26(1), 167–178. https://doi.org/10.1016/j.jjie.2011.07.006
- Aydin, M., & Turan, Y. E. (2020). The influence of financial openness, trade openness, and energy intensity on ecological footprint: Revisiting the environmental kuznets curve hypothesis for BRICS countries. Environmental Science and Pollution Research, 27(34), 43233–43245. https://doi.org/10.1007/s11356-020-10238-9
- Baek, J., Cho, Y., & Koo, W. W. (2009). The environmental consequences of globalization: A country-specific time-series analysis. Ecological Economics, 68(8–9), 2255–2264. https://doi.org/10.1016/j.ecolecon.2009.02.021
- Baltagi, B. H., Demetriades, P. O., & Law, S. H. (2007). Financial development, openness and institutions: Evidence from panel data (No.0022). World Economy Finance Research Programme, World Economy Finance Research.
- Beck, T. (2002). Financial development and international trade: Is there a link? Journal of International Economics, 57(1), 107–131. https://doi.org/10.1016/S0022-1996(01)00131-3
- Bilgili, F., Koçak, E., & Bulut, Ü. (2016). The dynamic impact of renewable energy consumption on CO2 emissions: A revisited environmental kuznets curve approach. Renewable and Sustainable Energy Reviews, 54, 838–845. https://doi.org/10.1016/j.rser.2015.10.080
- Birdsall, N., & Wheeler, D. (1993). Trade policy and industrial pollution in Latin America: Where are the pollution havens? The Journal of Environment & Development, 2(1), 137–149. https://doi.org/10.1177/107049659300200107
- Blanchard, O., Gollier, C., & Tirole, J. (2023). The portfolio of economic policies needed to fight climate change. Annual Review of Economics, 15(1), 689–722. https://doi.org/10.1146/annurev-economics-051520-015113
- Blanchard, O. J., Gollier, C., & Tirole, J. (2022). Fighting the war against climate change (working paper no.1360). Toulouse School of Economics. https://www.tse-fr.eu/sites/default/files/TSE/documents/doc/wp/2022/wp_tse_1360.pdf
- Chebbi, H. E., Olarreaga, M., & Zitouna, H. (2011). Trade openness and CO2 emissions in Tunisia. Middle East Development Journal, 3(1), 29–53. https://doi.org/10.1142/S1793812011000314
- Chinn, M. D., & Ito, H. (2002). Capital account liberalization, institutions and financial development: Cross country evidence (no. w8967). National Bureau of Economic Research. https://www.nber.org/papers/w8967.
- Chinn, M. D., & Ito, H. (2006). What matters for financial development? Capital controls, institutions, and interactions. Journal of Development Economics, 81(1), 163–192. https://doi.org/10.1016/j.jdeveco.2005.05.010
- Chinn, M. D., & Ito, H. (2019). The chinn-ito index – a de Jure measure of financial openness. http://web.pdx.edu/~ito/Chinn-Ito_website.htm.
- Chipman, H., George, E. I., McCulloch, R. E., Clyde, M., Foster, D. P., & Stine, R. A. (2001). The practical implementation of Bayesian model selection. Lecture Notes-Monograph Series, 65–134. https://doi.org/10.1214/lnms/1215540964.
- Choi, E., Heshmati, A., & Cho, Y. (2010). An empirical study of the relationships between CO2 emissions, economic growth and openness (no. 5304). IZA Institute of Labor Economics. https://ssrn.com/abstract=1708750.
- Cole, M. A., & Elliott, R. (2003). Determining the trade–environment composition effect: The role of capital, labor and environmental regulations. Journal of Environmental Economics and Management, 46(3), 363–383. https://doi.org/10.1016/S0095-0696(03)00021-4
- Cole, M. A., Elliott, R. J., & Fredriksson, P. G. (2006). Endogenous pollution havens: Does FDI influence environmental regulations? The Scandinavian Journal of Economics, 108(1), 157–178. https://doi.org/10.1111/j.1467-9442.2006.00439.x
- David, M. A., Mlachila, M. M., & Moheeput, A. (2014). Does openness matter for financial development in Africa? (No.14/94). International Monetary Fund. https://doi.org/10.5089/9781498359290.001.
- DiVita, G. (2009). Legal families and environmental protection: Is there a causal relationship? Journal of Policy Modeling, 31(5), 694–707. https://doi.org/10.1016/j.jpolmod.2009.01.003
- EPA (U.S. Environmental Protection Agency). (2019). Greenhouse gas reporting program. https://www.epa.gov/ghgreporting
- Feldkircher, M., & Zeugner, S. (2009). Benchmark priors revisited: On adaptive shrinkage and the supermodel effect in Bayesian model averaging. International Monetary Fund.
- Fernandez, C., Ley, E., & Steel, M. F. (2001a). Benchmark priors for Bayesian model averaging. Journal of Applied Econometrics, 100(2), 381–427. https://doi.org/10.1016/S0304-4076(00)00076-2
- Fernandez, C., Ley, E., & Steel, M. F. (2001b). Model uncertainty in cross‐country growth regressions. Journal of Applied Econometrics, 16(5), 563–576. https://doi.org/10.1002/jae.623
- Fragoso, T. M., Bertoli, W., & Louzada, F. (2018). Bayesian model averaging: A systematic review and conceptual classification. International Statistical Review, 86(1), 1–28. https://doi.org/10.1111/insr.12243
- Frankel, J. A. (2009). Environmental effects of international trade (no. 31). Harvard Kennedy School, Harvard University.
- Frankel, J. A., & Romer, D. H. (1999). Does trade cause growth? American Economic Review, 89(3), 379–399. https://doi.org/10.1257/aer.89.3.379
- Frankel, J. A., & Rose, A. K. (2005). Is trade good or bad for the environment? Sorting out the causality. Review of Economics and Statistics, 87(1), 85–91. https://doi.org/10.1162/0034653053327577
- George, E., & Foster, D. P. (2000). Calibration and empirical Bayes variable selection. Biometrika, 87(4), 731–747. https://doi.org/10.1093/biomet/87.4.731.
- Glaeser, E. L., & Shleifer, A. (2002). Legal origins. The Quarterly Journal of Economics, 117(4), 1193–1229. https://doi.org/10.1162/003355302320935016
- Goldsmith, R. W. (1969). Financial structure and development. Yale University Press. https://doi.org/10.2307/2230134.
- Grossman, G. M., & Krueger, A. B. (1991). Environmental impacts of a North American free trade agreement (no. w3914). National Bureau of economic research. https://doi.org/10.3386/w3914.
- Harrison, A. (1995). Openness and growth: A time-series, cross-country analysis for developing countries. Journal of Development Economics, 48(2), 419–447. https://doi.org/10.1016/0304-3878(95)00042-9.
- Harrison, A. E., & Eskeland, G. (2002). Moving to greener pastures? Multinationals and the pollution-haven hypothesis National Bureau of Economic Research. https://doi.org/10.3386/w8888.
- He, J. (2006). Pollution haven hypothesis and environmental impacts of foreign direct investment: The case of industrial emission of sulfur dioxide (SO2) in Chinese provinces. Ecological Economics, 60(1), 228–245. https://doi.org/10.1016/j.ecolecon.2005.12.008
- Hinne, M., Gronau, Q. F., van den Bergh, D., & Wagenmakers, E. J. (2020). A conceptual introduction to Bayesian model averaging. Advances in Methods and Practices in Psychological Science, 3(2), 200–215. https://doi.org/10.1177/2515245919898657
- Hoeting, J. A., Madigan, D., Raftery, A. E., & Volinsky, C. T. (1999a). Bayesian model averaging: A tutorial. Statistical Science, 382–401. https://doi.org/10.1214/ss/1009212519.
- Hoeting, J. A., Madigan, D., Raftery, A. E., & Volinsky, C. T. (1999b). Bayesian model averaging: A tutorial (with comments by M. Clyde, David Draper and EI George, and a rejoinder by the authors). Statistical Science, 14(4), 382–417. https://doi.org/10.1214/ss/1009212519.
- IPCC (Intergovernmental Panel on Climate Change). (2007). Climate change 2007: synthesis report. Contribution of working groups I, II and III to the fourth assessment report of the intergovernmental panel on climate change, 104. https://www.ipcc.ch/site/assets/uploads/2018/02/ar4_syr_full_report.pdf
- Jalil, A., & Mahmud, S. F. (2009). Environment kuznets curve for CO2 emissions: A cointegration analysis for China. Energy Policy, 37(12), 5167–5172. https://doi.org/10.1016/j.enpol.2009.07.044
- Jebli, M. B., Youssef, S. B., & Ozturk, I. (2016). Testing environmental kuznets curve hypothesis: The role of renewable and non-renewable energy consumption and trade in OECD countries. Ecological Indicators, 60, 824–831. https://doi.org/10.1016/j.ecolind.2015.08.031
- Jones, L. E., & Manuelli, R. E. (1995). A positive model of growth and pollution controls (no. w5205). National Bureau of Economic Research. https://doi.org/10.3386/w5205.
- Karimu, A., & Marbuah, G. (2017). Re-examining the financial development-openness nexus: Nonparametric evidence for developing countries. Journal of Applied Economics, 20(2), 373–394. https://doi.org/10.1016/S1514-0326(17)30017-X
- Kass, R. E., & Wasserman, L. (1995). A reference Bayesian test for nested hypotheses and its relationship to the schwarz criterion. Journal of the American Statistical Association, 90(431), 928–934. https://doi.org/10.1080/01621459.1995.10476592
- Kearsley, A., & Riddel, M. (2010). A further inquiry into the pollution haven hypothesis and the environmental kuznets curve. Ecological Economics, 69(4), 905–919. https://doi.org/10.1016/j.ecolecon.2009.11.014
- Khan, S. A. R., Yu, Z., Belhadi, A., & Mardani, A. (2020). Investigating the effects of renewable energy on international trade and environmental quality. Journal of Environmental Management, 272, 111089. https://doi.org/10.1016/j.jenvman.2020.111089
- Kock, C. J., & Min, B. S. (2016). Legal origins, corporate governance, and environmental outcomes. Journal of Business Ethics, 138(3), 507–524. https://doi.org/10.1007/s10551-015-2617-1
- Koengkan, M., Santiago, R., Fuinhas, J. A., & Marques, A. C. (2019). Does financial openness cause the intensification of environmental degradation? New evidence from Latin American and Caribbean countries. Environmental Economics and Policy Studies, 21(4), 507–532. https://doi.org/10.1007/s10018-019-00240-y
- Law, S. H., & Demetriades, P. (2006). Openness, institutions and financial development (no. 0012). World Economy and Finance Research Programme, Birkbeck, University of London. https://doi.org/10.2139/ssrn.941387.
- Leamer, E. E. (1978). Specification searches: Ad Hoc inference with nonexperimental data. Wiley.
- Le, T. H., Chang, Y., & Park, D. (2016). Trade openness and environmental quality: International evidence. Energy Policy, 92, 45–55. https://doi.org/10.1016/j.enpol.2016.01.030
- Lee, H., & Roland-Holst, D. (1997). The environment and welfare implications of trade and tax policy. Journal of Development Economics, 52(1), 65–82. https://doi.org/10.1016/S0304-3878(96)00439-7
- Ley, E., & Steel, M. F. (2009). On the effect of prior assumptions in Bayesian model averaging with applications to growth regression. Journal of Applied Econometrics, 24(4), 651–674. https://doi.org/10.1002/jae.1057
- Liang, F., Paulo, R., Molina, G., Clyde, M. A., & Berger, J. O. (2008). Mixtures of g priors for Bayesian variable selection. Journal of the American Statistical Association, 103(481), 410–423. https://doi.org/10.1198/016214507000001337
- Liddle, B. (2001). Free trade and the environment-development system. Ecological Economics, 39(1), 21–36. https://doi.org/10.1016/S0921-8009(01)00215-4
- Madigan, D., & Raftery, A. E. (1994). Model selection and accounting for model uncertainty in graphical models using Occam’s window. Journal of the American Statistical Association, 89(428), 1535–1546. https://doi.org/10.1080/01621459.1994.10476894
- Madigan, D., York, J., & Allard, D. (1995). Bayesian graphical models for discrete data. International Statistical Review / Revue Internationale de Statistique, 63. https://doi.org/10.2307/1403615. (2) 215
- Managi, S. (2004). Trade liberalization and the environment: Carbon dioxide for 1960-1999. Economics Bulletin, 17(1), 1–5. http://www.accessecon.com/pubs/EB/2004/Volume17/EB-03Q20005A.pdf.
- Managi, S., Hibiki, A., & Tsurumi, T. (2008). Does trade liberalization reduce pollution emissions? (no. 08013). Research Institute of Economy, Trade and Industry (RIETI). https://www.rieti.go.jp/jp/publications/dp/08e013.pdf.
- Managi, S., Hibiki, A., & Tsurumi, T. (2009). Does trade openness improve environmental quality? Journal of Environmental Economics and Management, 58(3), 346–363. https://doi.org/10.1016/j.jeem.2009.04.008
- McKinnon, R. I. (1973). Money and capital in economic development. Brookings Institution Press.
- Mutascu, M. (2018). A time-frequency analysis of trade openness and CO2 emissions in France. Energy Policy, 115, 443–455. https://doi.org/10.1016/j.enpol.2018.01.034
- Nasir, M., & Rehman, F. U. (2011). Environmental kuznets curve for carbon emissions in Pakistan: An empirical investigation. Energy Policy, 39(3), 1857–1864. https://doi.org/10.1016/j.enpol.2011.01.025
- Nordhaus, W. D. (1991). To slow or not to slow: The economics of the greenhouse effect. The Economic Journal, 101(407), 920–937. https://doi.org/10.2307/2233864
- Pedroni, P. (1999). Critical values for cointegration tests in heterogeneous panels with multiple regressors. Oxford Bulletin of Economics and Statistics, 61(S1), 653–670. https://doi.org/10.1111/1468-0084.61.s1.14.
- Porta, R. L., Lopez de Silanes, F., & Shleifer, A. (2008). The economic consequences of legal origins. Journal of Economic Literature, 46(2), 285–332. https://doi.org/10.1257/jel.46.2.285
- Porta, R. L., Lopez de Silanes, F., Shleifer, A., & Vishny, R. W. (1998). Law and finance. Journal of Political Economy, 106(6), 1113–1155. https://doi.org/10.1086/250042
- Raftery, A. E. (1988). Approximate Bayes factors for generalized linear models (No. 121). The University of Washington, Department of Statistics. https://stat.uw.edu/sites/default/files/files/reports/1988/tr121.pdf.
- Raftery, A. E. (1993). Bayesian model selection in structural equation models. Sage Focus Editions, 154, 163–180. https://sites.stat.washington.edu/raftery/Research/PDF/bollen1993.pdf.
- Raftery, A. E., Gneiting, T., Balabdaoui, F., & Polakowski, M. (2005). Using Bayesian model averaging to calibrate forecast ensembles. Monthly Weather Review, 133(5), 1155–1174. https://doi.org/10.1175/MWR2906.1
- Raftery, A. E., Madigan, D., & Hoeting, J. A. (1997). Bayesian model averaging for linear regression models. Journal of the American Statistical Association, 92(437), 179–191. https://doi.org/10.1080/01621459.1997.10473615
- Rajan, R. G., & Zingales, L. (2003). The great reversals: The politics of financial development in the twentieth century. Journal of Financial Economics, 69(1), 5–50. https://doi.org/10.1016/S0304-405X(03)00125-9
- Rock, M. T. (1996). Pollution intensity of GDP and trade policy: Can the World bank be wrong? World Development, 24(3), 471–479. https://doi.org/10.1016/0305-750X(95)00152-3
- Sebri, M., & Ben-Salha, O. (2014). On the causal dynamics between economic growth, renewable energy consumption, CO2 emissions and trade openness: Fresh evidence from BRICS countries. Renewable and Sustainable Energy Reviews, 39, 14–23. https://doi.org/10.1016/j.rser.2014.07.033
- Shafiei, S., & Salim, R. A. (2014). Non-renewable and renewable energy consumption and CO2 emissions in OECD countries: A comparative analysis. Energy Policy, 66, 547–556. https://doi.org/10.1016/j.enpol.2013.10.064
- Shahbaz, M., Nasreen, S., Ahmed, K., & Hammoudeh, S. (2016). Trade openness–carbon emissions nexus: The importance of turning points of trade openness for country panels. Energy Economics, 61, 221–232. https://doi.org/10.1016/j.eneco.2016.11.008.
- Shaw, E. S. (1973). Financial deepening in economic development. Oxford University Press: Oxford. https://doi.org/10.2307/2978421.
- Tamazian, A., Chousa, J. P., & Vadlamannati, K. C. (2009). Does higher economic and financial development lead to environmental degradation: Evidence from BRIC countries. Energy Policy, 37(1), 246–253. https://doi.org/10.1016/j.enpol.2008.08.025
- Tamazian, A., & Rao, B. B. (2010). Do economic, financial and institutional developments matter for environmental degradation? Evidence from transitional economies. Energy Economics, 32(1), 137–145. https://doi.org/10.1016/j.eneco.2009.04.004
- Tobey, J. A. (1990). The effects of domestic environmental policies on patterns of world trade: An empirical test. Kyklos, 43(2), 191–209. https://doi.org/10.1111/j.1467-6435.1990.tb00207.x
- Trabelsi, M., & Cherif, M. (2017). Capital account liberalization and financial deepening: Does the private sector matter? The Quarterly Review of Economics and Finance, 64, 141–151. https://doi.org/10.1016/J.QREF.2016.08.001
- Wasserman, L. (2000). Bayesian model selection and model averaging. Journal of mathematical psychology, 44(1), 92–107. https://doi.org/10.1006/jmps.1999.1278
- Westerlund, J. (2005). A panel CUSUM test of the null of cointegration. Oxford Bulletin of Economics and Statistics, 67(2), 231–262. https://doi.org/10.1111/j.1468-0084.2004.00118.x
- You, W. H., Zhu, H. M., Yu, K., & Peng, C. (2015). Democracy, financial openness, and global carbon dioxide emissions: Heterogeneity across existing emission levels. World Development, 66, 189–207. https://doi.org/10.1016/j.worlddev.2014.08.013
- Zafar, M. W., Shahbaz, M., Sinha, A., Sengupta, T., & Qin, Q. (2020). How does renewable energy consumption contribute to environmental quality? The role of education in OECD countries. Journal of Cleaner Production, 268, 122149. https://doi.org/10.1016/j.jclepro.2020.122149.
Appendix A.
Environmental quality in developing countries over the period 2003-2017
Figure A1. Environmental quality in developing countries over the period 2003–2017.
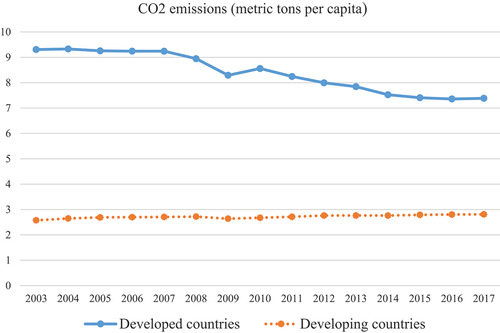
Appendix B.
The list of developing countries
Table A1. The list of selected developing countries.