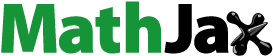
ABSTRACT
This study utilizes micro-level farm panel data from 2007 to 2012 to measure the technical efficiency of grain production among farms using a stochastic frontier analysis model. Additionally, it employs a two-way fixed effects model to empirically investigate the impact of fiscal support for agriculture on grain production technical efficiency and its underlying mechanisms. The results reveal significant room for improvement in the technical efficiency of grain production among Chinese farms, with increased fiscal support for agriculture demonstrating a substantial enhancement of their efficiency. This promoting effect only exists in the major grain-producing areas and increases with the increase of farm size. The augmentation of fiscal support for agriculture achieves this goal by augmenting modern agricultural input factors, alleviating financing constraints faced by farms, and optimizing agricultural production infrastructure. Our findings provide guidance for optimizing fiscal policy to support agriculture, promoting agricultural modernization, and achieving food security.
1. Introduction
Food security is a critical issue with significant implications for human survival. The United Nations (UN) has identified “eradicating hunger and achieving food security” as one of the seventeen Sustainable Development Goals (SDGs). Ensuring consistent and stable growth in grain yield is of significance for the economic development and social stability of developing countries. China is the largest developing country and one of the major agricultural countries, studying China’s food security issues holds valuable insights for other developing countries worldwide. Nowadays China’s food security is confronted with several challenges including the rising demands for grain and food consumption, the non-agricultural transfer of crucial input factors such as labour and land, and the mounting constraints imposed by limited resources and environmental concerns. Therefore, ensuring food security primarily hinges upon enhancing the grain production efficiency, rather than increasing input factors for grain production (Zhang et al., Citation2021). There exists an extensive body of literature that has quantitatively assessed grain production technical efficiency at macro levels, including provincial and county scales (Odeck, Citation2007; Zheng et al., Citation2023), and some of the literature used small-scale micro-level data for empirical analysis (Gong et al., Citation2019; Z. Liu & Zhuang, Citation2000). However, there is a lack of research based on national data on the technical efficiency of grain production on farms, which are the main body of grain production. Chari et al. (Citation2021) studied the impact of land property reform on farmer productivity by using the national fixed point (NFP) survey data at the farmer level. Although they used data on farmers at the national level, they did not examine the impact of fiscal support for agriculture on technical efficiency. Compared to their study, the measurement of technical efficiency in this study is further accurate to the farmer-crop level. On the other hand, considering the public attributes and externalities associated with agriculture and its low profitability as a vulnerable industry, government fiscal support plays a pivotal role in agricultural development. Agricultural protection and subsidies are prevalent practices globally (Byerlee & Sain, Citation1986; Mendelsohn, Citation2003). In China, the government places great importance on fiscal support and subsidies for agriculture. From 2016 to 2019, China’s general public budget expenditure allocated a cumulative expenditure of 6.07 trillion yuan for agricultural and rural purposes, with an average annual growth rate of 8.8%, surpassing the average growth rate of the general public budget expenditure.Footnote1 The government aims to improve grain production technical efficiency by fostering the enthusiasm of farmers through increasing fiscal support for agriculture, so as to ensure a stable grain supply and enhance the international competitiveness of grain products. Therefore, examining grain production technical efficiency at the farm level and investigating the impact and mechanism of fiscal support for agriculture on China’s grain production technical efficiency hold significant theoretical and policy implications for ensuring food security and promoting sustainable agricultural development in China and other developing countries.
Research on the economic impacts of fiscal support for agriculture primarily focused on aspects such as farmer income (Gao et al., Citation2013), agricultural economic growth (Stads & Beintema, Citation2015), and farmer consumption (Dercon et al., Citation2009; Fan et al., Citation2000). Existing studies have found that fiscal support for agriculture can effectively improve the agricultural production environment and conditions, enhance agricultural production capacity, and consequently promote income growth for farmers (Gao et al., Citation2013). Fiscal support for agriculture plays a significant role in stimulating agricultural economic growth in low- and middle-income countries (Idoko & Jatto, Citation2018). Fiscal support for agriculture elevates the consumption level of farmers, although there exist temporal and spatial heterogeneities (Blancard et al., Citation2006). On the other hand, extensive research examined the factors influencing grain production technical efficiency from both micro and macro perspectives. At the micro level, factors such as land input (Wu et al., Citation2005), fertilizer input (Liefert et al., Citation2003), machinery input (Monchuk et al., Citation2010; Wu et al., Citation2021), effective irrigation area (Hassan et al., Citation2000), labour input, and characteristics of farmers (Li & Sicular, Citation2013; Tian & Wan, Citation2000) have been found to affect grain production technical efficiency. At the macro level, existing research mainly focused on regional economic development levels (Kuang et al., Citation2021), environmental pollution (Hoang & Coelli, Citation2011; Li et al., Citation2022), climate conditions (Chen & Gong, Citation2021), technological changes (Jin et al., Citation2002, Citation2010), and agricultural infrastructure (Chen & Ding, Citation2007; Teruel & Kuroda, Citation2005) as factors influencing grain production efficiency. For instance, S. Chen and Gong (Citation2021) utilized 35 years of county-level panel data in China to assess the impact of global warming on China’s agricultural total factor productivity (TFP) and found that extremely high temperatures reduce China’s agricultural TFP, while climate adaptation can mitigate this negative impact. Although some studies have attempted to establish a linkage between fiscal support for agriculture and macro-level grain production efficiency (Zhang et al., Citation2021), they have overlooked the investigation of micro-level farms’ grain production technical efficiency and the exploration of underlying mechanisms.
Based on the literature above, despite a considerable number of empirical studies that have extensively examined fiscal support for agriculture and grain production technical efficiency, there are several shortcomings in the existing research. Firstly, previous studies on grain production technical efficiency primarily focused on macro-level measurements at the provincial and county levels, lacking investigation of grain production technology efficiency at the farm level of a national sample. Secondly, the examinations of the effects of fiscal support for agriculture in existing research are still not comprehensive, mainly concentrating on farmer income, consumption, and agricultural economic growth. There is a lack of research evaluating the economic consequences of fiscal support for agriculture from the perspective of grain production technical efficiency. Thirdly, although some studies explored the relationship between fiscal support for agriculture and grain production technical efficiency, there is still a lack of research that empirically examines the mechanisms, such as input factors, financing constraints, and infrastructure, through which fiscal support for agriculture affects grain production technical efficiency.
This study presents an empirical analysis of the effect of fiscal support for agriculture on farm-level grain production technical efficiency in China. By constructing a panel dataset at the farm level from 2007 to 2012, and employing the stochastic frontier analysis model, we measure the Chinese farm’s grain production technical efficiency. Furthermore, we investigate the effects and mechanisms through which fiscal support for agriculture affects farm-level grain production technical efficiency by utilizing a two-way fixed effects model. This study makes several significant contributions to the literature. Firstly, this study computes grain production technical efficiency using micro-level data, allowing us to examine the effect of city-level fiscal support for agriculture on farm-level grain production technical efficiency, thus addressing the limitations of existing macro-level studies. Secondly, this study enriches and expands the empirical research on the impacts of fiscal support for agriculture, providing valuable insights for a comprehensive assessment of the policy design of fiscal support for agriculture. These findings bear strong policy implications for government efforts in promoting agricultural modernization and sustainable growth in grain production. Lastly, this study elucidates the mechanisms through which fiscal support for agriculture affects grain production technical efficiency, thus contributing to the existing literature on the impact of public policies on grain production efficiency and offering valuable evidence from a developing country context regarding the relationship between fiscal support for agriculture and grain production technical efficiency.
The remainder of the article is organized as follows. Section 2 presents the conceptual framework, outlining the theoretical foundation of this study. Section 3 describes the empirical strategy and econometric model. Section 4 provides a comprehensive description of the data and variables in this study. Section 5 presents the results of baseline estimations, robustness checks, heterogeneity analyses, mechanism tests, and our discussion. Section 6 is our conclusion.
2. Conceptual framework
Agricultural production serves as the foundation for a nation’s sustenance and development (Ellis, Citation2008). Nevertheless, agriculture is inherently characterized by externalities and dual risk attributes, making it a sector with weakened competitiveness (Reardon et al., Citation1994). Smith (Citation1776) argued that agriculture cannot function under a strictly specialized system. Nurkse (Citation1952) identified the existence of a poverty-driven cycle in underdeveloped regions. The Lewis dualistic economic model presupposes agriculture as a low-quality sector (Lewis, Citation1954). Schultz (Citation1964), in contrast to prior scholars, proposed that modern agriculture could promote economic growth, contingent upon the modernization of the traditional “poor and inefficient” agricultural sector. However, the profit-driven dynamics of markets suggest that the market mechanism alone cannot facilitate the transition from traditional to modern agriculture (David et al., Citation2000; Timmer, Citation1995). Therefore, increasing government regulation in the agricultural sector to compensate for the inherent deficiencies of the market mechanism has become an important area of study in modern public fiscal theory (Kelly et al., Citation2003; Rubenstein et al., Citation2003). Although agricultural development can bring substantial social benefits, due to the non-rival and non-excludable characteristics of the public goods it provides, the private sector will lack the incentive to invest in it (Fan & Zhang, Citation2008). Consequently, government support through fiscal measures in agriculture has emerged as a critical approach to ensuring national food security, particularly in the face of increasing food demand and resource and environmental constraints (Fan et al., Citation2021; Qaim, Citation2020). The aforementioned theories of public fiscal and agricultural externalities provide theoretical support for fiscal policies aimed at improving agricultural productivity and reinforcing international food competitiveness.
Fiscal support for agriculture can affect the grain production technical efficiency through various channels. To start with, it contributes to enhancing modern input factors utilized in grain production. Against the backdrop of industrialization and urbanization, due to the higher returns of non-agricultural industries, there has been an overall outflow of agricultural labor. The substitution of modern input factors represented by agricultural machinery for traditional factors has become an inevitable trend in agricultural production (Huang et al., Citation2012). The process of agricultural development in China since the reform and opening up also reflects the above-mentioned pattern (Gong, Citation2018a). Firstly, fiscal support for agriculture can alleviate problems such as insufficient factor investment due to farmers’ financial constraints and risk expectations (Karlan et al., Citation2014), thus effectively augmenting the modern input factors in agricultural production processes. For instance, subsidies for the purchase of agricultural machinery can guide farmers in engaging specialized service providers to deliver mechanized operations essential for the entire grain production process, enabling farmers to efficiently accomplish their tasks (Labarthe & Laurent, Citation2013; Ma et al., Citation2018). Secondly, fiscal expenditures, serving as investments in technological research and development, can stimulate technological advancements, thereby enhancing the quality of modern agricultural input factors like machinery and fertilizers (McArthur & McCord, Citation2017). Thirdly, fiscal support for agriculture can alter the relative prices of agricultural input factors through various subsidy methods, further enhancing the allocation ratio of modern agricultural input factors (Adamopoulos & Restuccia, Citation2014; Deaton & Deaton, Citation2020), thereby optimizing the combination among machinery, land, labour, and other different factors. Previous studies suggest that modern input factors such as agricultural mechanization can improve the efficiency of resource allocation, reduce the average grain production costs, enhance specialized production practices, ultimately fostering improvements in both technological levels and productivity in grain production (Ma et al., Citation2018; Wang et al., Citation2020). Consequently, fiscal support for agriculture can facilitate improved grain production technical efficiency by elevating modern agricultural input factors.
Furthermore, fiscal support for agriculture contributes to increasing household income levels and facilitates asset accumulation among farmers, thereby reducing their financial constraints and improving accessibility to loans (Islam & Luo, Citation2018; Kassouri & Kacou, Citation2022). Due to the vulnerability of agricultural production and agricultural operators themselves, the profit-seeking nature of financial institutions, and the imbalance in the allocation of funds between urban and rural areas, agriculture faces serious financing constraints, hindering agricultural development and transformation (Guirkinger & Boucher, Citation2008). Agricultural credit subsidies within fiscal support for agriculture provide financial information for various stages such as production, procurement, warehousing, and transportation of grains. By offering timely and effective financing channels for grain producers, agricultural credit subsidies can reduce the financing costs associated with grain production. Numerous empirical studies indicate that agricultural credit plays a facilitating role in enhancing the grain production technical efficiency (Ali et al., Citation2014; Balana et al., Citation2022; Z. Liu & Zhuang, Citation2000). For instance, Liu and Zhuang (Citation2000) explained the importance of rural credit to farmers’ technical efficiency by relaxing liquidity constraints. Consequently, fiscal support for agriculture can enhance the grain production technical efficiency by alleviating credit constraints.
Finally, as a fundamental tool for national regulation for agricultural production, fiscal support for agriculture can effectively address the issue of inadequate supply of public goods in the agricultural production process (Hazell & Varangis, Citation2020). Compared to the needs of agricultural and rural modernization, there is insufficient investment in agricultural infrastructure, and issues of misallocation exist. The incidence of agricultural water and drought disasters remains relatively high (Pinstrup-Andersen & Shimokawa, Citation2006). Fiscal support for agriculture implies the flow of financial and fiscal resources from urban and non-agricultural sectors towards agriculture sector. One crucial direction of this support involves amplifying investments in agricultural infrastructure such as farmland and irrigation. This serves to enhance the agricultural production environment and elevate the level of agricultural infrastructure construction (Zhong et al., Citation2019). Agricultural infrastructure has both scale expansion effects, increasing the quantity and quality of arable land, and technological progress effects, optimizing the input structure of grain production to achieve higher crop yields with fewer expensive inputs (Finger et al., Citation2019; Pan et al., Citation2021). Infrastructure plays a crucial role in economic growth, and the agricultural sector, which heavily relies on external financial support, particularly benefits from agricultural infrastructure construction as a precursor to development (Donaldson, Citation2018; Shamdasani, Citation2021). Several empirical studies suggest that improving agricultural infrastructure construction effectively lowers agricultural production costs, promotes an increase in grain production efficiency, subsequently raising grain yield and competitiveness (Teruel & Kuroda, Citation2005; Yuan et al., Citation2021). Therefore, fiscal support for agriculture can enhance grain production technical efficiency by elevating the level of agricultural infrastructure (as shown in ).
Figure 1. Conceptual framework.
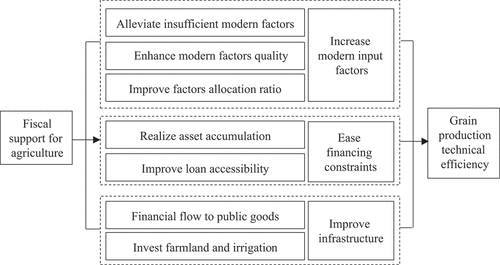
In summary, fiscal support for agriculture is crucial for agricultural development. Based on the conceptual framework above, fiscal support for agriculture might enhance input factors, alleviate financial constraints, and strengthen infrastructure, thereby fostering an improvement in grain production technical efficiency. However, whether fiscal support for agriculture inevitably enhances grain production technical efficiency, and whether the corresponding increase in agricultural fiscal expenditure inevitably promotes the improvement of grain production technical efficiency through the enhancement of these three channels, requires further empirical verification through quantitative analytical tools.
3. Empirical strategy and econometric model
3.1. Stochastic frontier analysis
Stochastic frontier analysis (SFA) and data envelopment analysis (DEA), are the most popular statistical tools in the line of efficiency analysis (Coelli & Rao, Citation2005; Ruttan, Citation2002). Some studies (Rezitis, Citation2010; Suhariyanto & Thirtle, Citation2008) have applied the DEA to estimate agricultural efficiency. However, some scholars (Headey et al., Citation2010; Nin et al., Citation2003) also claimed that DEA-based efficiency measures always draw anomalous results as compared with those from other measures of agricultural development, since DEA cannot distinguish efficiency from white noise and measurement error. Yuan et al. (Citation2021) stated that white noise and measurement error are significant challenges and problems in agricultural efficiency analysis. Nevertheless, neither of the two issues can be fully addressed by DEA. Compared with DEA, SFA imposes assumptions of parametric functional forms and can carry out statistical tests on the results (Nguyen et al., Citation2016), which means that SFA is able to deal with both problems and rule them out. Headey et al. (Citation2010) also pointed out that SFA estimations are significantly more stable and plausible than those derived by DEA. In summary, SFA model is more suitable for this study to estimate grain production technical efficiency.
The original SFA model was initially proposed by Aigner et al. (Citation1977) and Meeusen and van Den Broeck (Citation1977), then developed by Schmidt and Sickles (Citation1984) under a panel data setting:
Where means the output value of farm i at time t in logarithms; and
measures the kth input of farm i at time t in logarithms;
is the vector of all K types of inputs, including Labour (number of days of labour required), Fertilizer (total amount of pure fertilizer used), Machinery (cost of mechanical operation), and Other intermediate input (includes management cost, financial cost, insurance cost, seed cost, pesticide cost, and irrigation cost).
is the non-negative random term that indicates the technical inefficiency;
is the typical disturbance;
.
is the production function, which describes the production frontier.
The Transcendental Logarithmic (T-L) production function and the Cobb Douglas (C-D) production function are two widely used production functions. Hence, it is important to adopt scientific and rigorous tests to decide whether to choose the T-L production function or the C-D production function as the production function of this paper. Wallach and Goffinet (Citation1989) pointed out that the mean squared error (MSE) is a reasonable criterion of model quality and can be used to determine model selection. The MSE is defined by: , where
is the observed values of the variable, with
being the predicted values. Similar to the MSE, there are also the mean absolute error (
) and the mean absolute percentage error (
). The MSE, MAE and MAPE can be used to reflect the errors between the observed values and the predicted values of the model, and then reflect the accuracy of the model. Many studies have used these indicators to consider the selection of models (De Myttenaere et al., Citation2016; Köksoy, Citation2006; Willmott & Matsuura, Citation2005). Therefore, this paper also adopts the MSE, MAE and MAPE to determine the production function. By calculating and comparing these three indicators of the two functions’ estimation results, we find that the C-D production function has less errors and is more suitable for the data in this paper (See in Appendix).
EquationEquation (1)(1)
(1) shows the SFA model with the time-invariant technical inefficiency. However, it is not appropriate to assume that technical inefficiency is time-invariant when analysing the panel data with a long-time span. Hence, many scholars have developed various methods to estimate the time-variant SFA model (Battese & Coelli, Citation1992; Cornwell et al., Citation1990; Kumbhakar, Citation1990; Lee & Schmidt, Citation1993; Sickles, Citation2005). One of the most popular estimators is the BC92 estimator built by Battese and Coelli (Citation1992), it defines the technical inefficiency as:
Where is assumed to be independent and identically distributed non-negative truncations of the
distribution;
is an unknown scalar parameter; and
represents the whole time period length that the i-th farm is involved. The BC92 estimator has a monotonicity restriction on technical efficiency. But the assumption of time-invariant and individual-invariant growth rates in technical efficiency that is held by the BC92 estimator is unrealistic and the BC92 estimator fails to capture both the changing macro environment and individual-level factors due to its rigid assumption of the time-invariant and individual-invariant technical efficiency trend (Yuan et al., Citation2021). Hence, in order to capture the time-variant and individual-variant technical efficiency trend, the CSS estimator with more flexible assumption is applied in this paper. The CSS estimator established by Cornwell et al. (Citation1990) assumes that the technical inefficiency is a quadratic function that varies over time, which can be expressed as:
The CSS estimator sets the technical inefficiency as the quadratic equation of t, captures the non-linear trend of technical inefficiency over time, and captures the heterogeneity in the technical inefficiency trend across farms by the farm-specific parameters (). We have
,
, and rewrite EquationEquation (1)
(1)
(1) as:
We can obtain the estimate of
, then we have the
. We set the max function:
, then we have
. Technical efficiency can be derived by
.
3.2. Fixed effects model
In accordance with the research hypothesis, the objective of the empirical strategy design is to examine the impact of fiscal support for agriculture on grain production technical efficiency. The model should solve the endogeneity problems of farm-level panel data, which would lead to systematic bias in the estimation results, due to unobservable effects between different farms and between different years. We employ a panel two-way fixed effects model to obtain unbiased estimates of the impact of fiscal support for agriculture on grain production technical efficiency following Rockoff (Citation2004) and Lu (Citation2012). The regression equation is specified as follows:
In EquationEquation (4)(4)
(4) , the dependent variable
represents the grain production technical efficiency of farm i located in prefecture-level city j at time t. The independent variable
represents the fiscal support for agriculture of prefecture-level city j at time t, measured as the ratio of fiscal support for agriculture expenditure to the total budget expenditure. The coefficient β is of primary interest as it quantifies the impact of fiscal support for agriculture on grain production technical efficiency. The vector
represents a set of control variables of prefecture-level city j at time t that may affect grain production technical efficiency and fiscal support for agriculture. These control variables aim to eliminate potential confounding factors that could interfere with the estimation of the coefficient of core independent variable.
represents individual fixed effects, capturing unobserved factors that vary across farms but do not change over time.Footnote2
represents year fixed effects, capturing unobserved factors that vary across years but do not change over farms.Footnote3
denotes the random error term of the regression equation. This study adopts White’s heteroscedasticity-robust standard errors to account for possible heteroscedasticity problems.
4. Data
4.1. Data source
Based on the empirical strategy and econometric model described above, we employ the panel data that incorporated variables related to input-output of grain production, fiscal support for agriculture, and other relevant variables associated with agricultural production. By utilizing the panel data, we are able to capture both cross-sectional and time-series variations, thereby enhancing the robustness of our analyses and controlling for unobserved heterogeneity across different farms and time periods.
The data used in this study are from two databases. The first part of the data is at the farm level and from the annual Farm Production Costs and Returns Survey (2007–2012) conducted by China’s National Development and Reform Commission. This part of the data is mainly used in the SFA model to calculate the grain production technical efficiency. This study follows Liu et al. (Citation2016) and Gong (Citation2018b) in selecting output and inputs. Output is measured by output value per hectare and adjusted by provincial-level consumer price index. Inputs are measured by Labour (number of days of labour required per hectare), Fertilizer (total amount of pure fertilizer used per hectare), Machinery (cost of mechanical operation per hectare), and Other intermediate input (Other cost except mechanical cost per hectare, including management cost, financial cost, insurance cost, seed cost, pesticide cost, and irrigation cost). Machinery is adjusted by provincial-level agricultural machinery price index. Other intermediate input is adjusted by provincial-level agricultural means of production price index. Since output and input variables are all measured per hectare, we don’t have to take land into our input factors.
The second part of the data is from the China City Statistical Yearbook (2007–2012) conducted by China’s National Bureau of Statistics.Footnote4 This part of the data is mainly used in the fixed effects model to estimate the impact of fiscal support for agriculture on grain production technical efficiency. We choose the ratio of fiscal support for agriculture expenditure in the total budget expenditure as the fiscal support for agriculture. Following Brümmer et al. (Citation2006) and Chen et al. (Citation2008), we also choose per capita net income of rural residents (adjusted by provincial-level consumer price index), ratio of added value of primary industry in GDP, ratio of primary industry employees in total employment, cultivated area, and effective irrigation area as the control variables. These control variables can reflect the level of local rural economic development and agricultural development, and help to solve endogeneity problem.
We merge the two databases using prefecture-level city (the prefecture-level city where the farm is located) and year. Finally, an unbalanced farm-level panel for 188 prefecture-level cities from 2007 to 2012 in China with a total of 22,879 observations is collected from above two databases.
4.2. Data description
presents the definitions and summary statistics of the main variables used in our empirical analysis.Footnote5 Within our study sample, the average output value is 11.419 thousand yuan/hectare, with a standard deviation of 3.284. The average number of days of labour required is 124 days/hectare, with a standard deviation of 50. The average amount of fertilizer used is 315 kg/hectare, with a standard deviation of 100. The average cost of mechanical operation is 1,033 yuan/hectare, with a standard deviation of 653. The average cost of other intermediate input is 2,669 yuan/hectare, with a standard deviation of 928. Different farms face varying production costs and output returns. The average fiscal support ratio is 11.6%, with a standard deviation of 0.037. Overall, the sample’s fiscal support ratio is low, and there are variations across different prefecture-level cities. The highest prefecture-level city has a fiscal support ratio as high as 44.5%, while the lowest prefecture-level city accounts for only 3.0%. The independent variable exhibits significant variation, enabling us to effectively evaluate the impact of the fiscal support ratio on grain production technical efficiency. Examining other variables related to agricultural production, the mean income is 5.142 thousand yuan per capita. The average primary industry ratio is 17.5%, and the average employee ratio is 43.8%. This indicates a relatively higher employee ratio to primary industry ratio. The average size of cultivated area in the prefecture-level city is 360 thousand hectares, and the average size of effective irrigation area is 225 thousand hectares. Overall, the sample covers prefecture-level cities with different levels of economic and agricultural development, as well as farms with different agricultural production characteristics. Additionally, the variables used in the empirical analysis exhibit significant statistical differences within our sample.
Table 1. Summary statistics.
A description of the various waves of the farm-level data is provided in in Appendix. In a total of 6 waves of samples, the number of farms per year ranges from 1,969 to 5,444, and there is no extreme gap between the number of farms in different years. Besides, the average output value increased from 10,109 yuan/hectare in 2007 to 13,570 yuan/hectare in 2012. Interestingly, the average labour input decreased from 130 days/hectare in 2007 to 110 days/hectare in 2012, and the average fertilizer input decreased from 323 kg/hectare in 2007 to 293 kg/hectare in 2012, while the average machinery input increased from 856 yuan/hectare in 2007 to 1,333 yuan/hectare in 2012. This indicates that farms used more machinery input and reduced labour and fertilizer inputs in agricultural production. This shows that farms may increase the use of machinery to replace labour and fertilizer inputs.
5. Results
5.1. Efficiency estimates
Based on the unbalanced panel data of 22,879 farms, we use the SFA model to estimate the grain production technical efficiency at the farm level in China from 2007 to 2012. reports the estimated results of the SFA model. Where means the variance of technical inefficiency,
means the typical disturbance variance. Hence,
means the proportion of the productivity gap between farms and the production frontier attributed to technical inefficiency. It can be observed from the table that
is 0.8177 indicating that 81.77% of the gap between farms and the production frontier is attributed to technical inefficiency. Additionally, the average technical efficiency is 0.545, which is considerably lower than the maximum value of 1. This suggests that there is significant room for improvement in the grain production technical efficiency among Chinese farms.
Table 2. Estimation results of the production function.
In , we compare the average fiscal support ratio and the average grain production technical efficiency across the prefecture-level cities during the period of 2007–2012. illustrates that prefecture-level cities with higher average fiscal support ratio tend to have higher average grain production technical efficiency, while prefecture-level cities with lower average fiscal support ratio exhibit lower average grain production technical efficiency. We present the time trend of grain production technical efficiency in prefecture-level cities categorized by high and low fiscal support ratios in . We find that grain production technical efficiency among farmers in prefecture-level cities with higher fiscal support ratios is notably superior to that of farmers in prefecture-level cities with lower fiscal support ratios. Therefore, it is likely that fiscal support for agriculture has a positive impact on grain production technical efficiency. Furthermore, we also provide a distribution histogram of the fiscal support ratio and the grain production technical efficiency in .
Figure 2. Fiscal support ratio and technical efficiency in China (2007–2012).
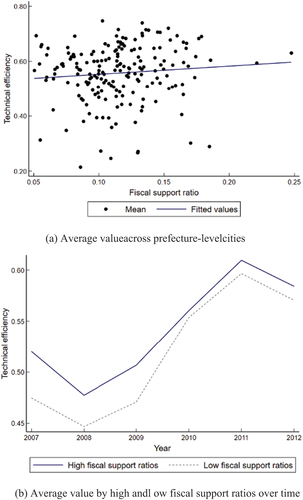
5.2. Baseline results
presents the OLS estimation results of the impact of fiscal support for agriculture on grain production technical efficiency. In column (1), only the fiscal support ratio is included as the independent variable, while column (2) also includes time fixed effects and individual fixed effects. In columns (3)-(5), we remove time fixed effects and individual fixed effects, then gradually introduce control variables that reflect farmers’ economic conditions, the importance of agriculture, and the level of agricultural development. In columns (6) and (7), we re-add time fixed effects and individual fixed effects, respectively. When controlling fiscal support ratio alone, the coefficient of fiscal support ratio is significantly positive. After gradually adding control variables and fixed effects, the coefficient remains to be significantly positive. This indicates that the impact of fiscal support for agriculture on technical efficiency is robust.
Table 3. Main regression results.
We prefer the coefficient in column (7) because the regression result in column (7) has the most comprehensive control variables. Specifically, a 1% increase in the fiscal support ratio results in a 0.2573% increase in grain production technical efficiency. In our study sample, the mean grain production technical efficiency is 0.545, suggesting that a 10% increase in the fiscal support ratio leads to a 0.014 increase in grain production technical efficiency. Our findings are consistent with B. Gong’s (Citation2018a) estimates based on provincial-level data, where he suggests that a 1% increase in agricultural public expenditure could lead to a 0.006% improvement in agricultural productivity. Moreover, by utilizing farm-level data, we effectively control for individual fixed effects, thereby mitigating the underestimation issue caused by omitted variables.
Furthermore, the R-squared of the regression in column (7) is 0.8715, indicating that the regression model can explain 87.15% of the variation in grain production technical efficiency and highlighting the accuracy of the regression model.
5.3. Robustness checks
In this section, we conduct a series of robustness checks to validate our baseline results and mitigate concerns regarding potential biases. presents the results of our robustness checks.
Table 4. Robustness checks.
Firstly, in our baseline regression, we use the ratio of fiscal support for agriculture expenditure in total budget expenditure (Fiscal support ratio) as the independent variable to measure fiscal support for agriculture. However, different ways of measuring fiscal support for agriculture may lead to different estimation results. To verify whether our estimation results are affected by the measurement of the independent variable, we use the logarithm of fiscal support for agriculture expenditure (Ln(Fiscal support)) and the ratio of fiscal support for agriculture expenditure in the added value of the primary industry (Fiscal support ratio’) as alternative proxies for fiscal support for agriculture in columns (1) and (2) of . The results in columns (1) and (2) indicate that both the logarithm of fiscal support for agriculture expenditure and the ratio of fiscal support for agriculture expenditure in the added value of the primary industry still have a significantly positive impact on grain production technical efficiency. This suggests that our baseline results are robust and not driven by the measurement of the independent variable.
Secondly, in our baseline regression, heteroscedasticity-robust standard errors are adopted to determine the significance of the estimated coefficients, as these standard errors do not rely on any specific assumptions under large sample sizes. To check the robustness of coefficient significance, we make different assumptions about the variance distribution of the error term of the regression model in columns (3) and (4) of . In column (3), we assume that the variance of the error term is heteroscedastic across counties and use clustered standard errors at county level. In column (4), we assume that the variance of the error term is heteroscedastic across prefecture-level cities and use clustered standard errors at the prefecture-city level. The results in columns (3) and (4) indicate that the impact of fiscal support for agriculture on the grain production technical efficiency is still significantly positive at 5% level. This suggests that our baseline results are robust and not driven by the assumptions about the variance distribution of the error term of the regression model.
Lastly, we use the SFA model based on the C-D production function to estimate the grain production technical efficiency in our baseline regression. Hence, in column (5) of , we use the SFA model based on the T-L production function to estimate the new grain production technical efficiency and replace the original dependent variable with the new T-L technical efficiency. Furthermore, in our baseline regression, we employ the CSS estimator to estimate the SFA model based on the C-D production function. To examine whether our empirical results are affected by the choice of estimator, we employ the BC95 estimator (Battese & Coelli, Citation1995) and adopt size (the acreage of the farm) and variety (the dummy variable of crop varieties) to define the technical inefficiency effects. Then we use the BC95 estimator to estimate the SFA model based on the C-D production function and obtain the new grain production technical efficiency. In column (6) of , we replace the original dependent variable with the new BC95 technical efficiency. The results in columns (5) and (6) confirm our baseline results once again. After using new technical efficiency, the impact of fiscal support for agriculture on the grain production technical efficiency is still significantly positive. This indicates that our baseline results are robust and not driven by the measurement of the dependent variable.
5.4. Heterogeneity analyses
In this section, we conduct heterogeneity analyses on the results of baseline regression by introducing interaction terms. The results are shown in .
Table 5. Heterogeneity results by major grain-producing areas and by farm size.
On the basis of considering the differences in resource endowments and the development of grain production among provinces, the Chinese government divided each province into major grain-producing areas (MPGA) or non-major grain-producing areas. The major grain-producing areas undertake the main grain production tasks and play a core role in ensuring national food security. Hence, the impact of fiscal support for agriculture on the grain production technical efficiency may be different between major grain-producing areas and non-major grain-producing areas. Results in column (1) of show that the coefficient of the interaction term of fiscal support ratio and MGPA is significantly positive, while the coefficient of fiscal support ratio is not significant. These results suggest that the promotion effect of fiscal support for agriculture on grain production technical efficiency is only present in major grain-producing areas. In major grain-producing areas, where grain production is the primary focus, government fiscal support for agriculture can effectively implement measures that enhance grain production, thereby improving grain production technical efficiency. These findings underscore the importance of targeted policies that consider regional heterogeneity in promoting sustainable and efficient grain production in China’s agricultural sector.
The relationship between farm size and agricultural production technical efficiency has been a focal point in the field of agricultural economics, but a consensus has not been reached. Small-scale farms have the advantage of providing more family labour and the ability to adjust agricultural production strategies, thereby improving agricultural production technical efficiency (Bevis & Barrett, Citation2020; Rada & Fuglie, Citation2019). However, large-scale farms have greater scale-related budget capabilities and the ability to invest in advanced machinery, allowing them to better benefit from technological advancements (Sheng & Chancellor, Citation2019; Tan et al., Citation2010; Wang et al., Citation2015). Overall, the improvement of technical efficiency growth that farms benefit from fiscal support for agriculture may vary with their farm size. Results in column (2) of show that the coefficient of the interaction term of fiscal support ratio and size is significantly positive. This indicates that larger farms benefit more from the promotional effect of fiscal support for agriculture on grain production technical efficiency.
Moreover, we take the MGPA and Size into the same regression in column (3) and find that the coefficients of interaction terms don’t change much. This indicates that the heterogeneity in MGPA and farm size of the impact of fiscal support for agriculture on technical efficiency is robust.
5.5. Mechanisms
We have already identified the positive impact of government fiscal support for agriculture on grain production technical efficiency in our baseline regression. In this section, we will further explore the specific mechanisms through which fiscal support for agriculture affects grain production technical efficiency. presents the results of our mechanisms tests.
Table 6. Mechanism tests.
Firstly, an increase in the proportion of modern agricultural production input factors contributes to the improvement of grain production technical efficiency (Y. Zhang & Brümmer, Citation2011). We measure the modern agricultural production input factors using the natural logarithm of total agricultural machinery power. The results reported in column (1) of indicate that for every 1% increase in the fiscal support ratio, there is a corresponding 0.2459% increase in the agricultural machinery power. It indicates that increased government fiscal support for agriculture enhances grain production technical efficiency by facilitating the allocation of modern agricultural input factors and optimizing the allocation of agricultural production resources. We did not include modern agricultural production factors as control variables in our baseline regression, because in this study, fiscal support for agriculture can affect grain production technical efficiency by influencing modern agricultural production factors. Including the mechanism variables in the regression would lead to an underestimation of our baseline estimation results.
Furthermore, due to the high risk and low liquidity of agricultural activities, as well as the lack of high-quality collateral for agricultural credit, many farmers often face difficulties in obtaining sufficient credit loans, which hinders their ability to engage in optimal agricultural production and improve agricultural production technical efficiency (Ali et al., Citation2014; Balana et al., Citation2022). We measure the financing constraint faced by farmers using the agricultural loan rate, which is calculated as the ratio of agricultural loans to the total loan amount. The results reported in column (2) of demonstrate that a 1% increase in the fiscal support ratio leads to a 0.0232% increase in the agricultural loan ratio. The increase in government fiscal support for agriculture alleviates financing constraints faced by farmers, enabling them to make grain production decisions without being limited by financial constraints. Consequently, this enhances grain production technical efficiency.
Lastly, the improvement in agricultural infrastructure contributes to enhancing agricultural production conditions, reducing production costs, and further improving grain production technical efficiency (Teruel & Kuroda, Citation2005; Yuan et al., Citation2021). We employ two variables, rural electric power consumption and per capita highway mileage, to measure the level of infrastructure construction in the respective regions, which are logarithmically transformed prior to regression analysis. The results in columns (3) and (4) of demonstrate that a 1% increase in the fiscal support ratio leads to a 0.7521% increase in rural electricity power consumption and a 0.1893% increase in per capita highway mileage. The increase in government fiscal support for agriculture promotes the development of agriculture-related infrastructure, thereby enhancing grain production technical efficiency.
6. Conclusion
Based on the panel data of farms and prefecture-level cities from 2007 to 2012, we employ the SFA model to estimate the grain production technical efficiency at the farm level. Additionally, we use a two-way fixed effects model to empirically examine the impact of government fiscal support for agriculture on grain production technical efficiency. Furthermore, a series of robustness checks are conducted to ensure the stability of the baseline estimates. We also do heterogeneity analyses by major grain-producing areas and farm size. Finally, following the conceptual framework, we explore the mechanisms through which government fiscal support for agriculture affects grain production technical efficiency, focusing on three aspects: modern agricultural input factors, financing constraints, and agricultural production infrastructure.
We find that during the period of 2007–2012, the average grain production technical efficiency of Chinese farms was 0.545 significantly below the maximum value of 1. This indicates that there is substantial room for improvement in the grain production technical efficiency of Chinese farms. Furthermore, the results of the baseline regression show that government fiscal support for agriculture can significantly enhance grain production technical efficiency. For 1% increase in the ratio of fiscal support for agriculture expenditure to the total budget expenditure, the grain production technical efficiency is observed to improve by 0.2573%. Moreover, the robustness checks demonstrate that the baseline regression results remain robust under different measures of fiscal support for agriculture, variance assumptions, and technical efficiency estimation methods. Additionally, the heterogeneity analyses reveal that the promotion effect of government fiscal support on grain production technical efficiency is only significant in major grain-producing areas. Furthermore, larger-scale farms experience a greater promotion effect. Lastly, the mechanism analysis indicates that government fiscal support for agriculture can enhance grain production technical efficiency by promoting modern agricultural production input factors, alleviating financing constraints faced by households, and improving agricultural production infrastructure.
This study addresses the limitations of existing literature by utilizing unique farm-level data to estimate grain production technical efficiency, thereby providing a more comprehensive understanding of agricultural production technical efficiency at the micro level. In addition to measuring grain production technical efficiency at the farm level, this study empirically examines the impact and mechanisms of government fiscal support for agriculture on grain production technical efficiency. It complements the existing literature on the economic effects of government fiscal support for agriculture and the factors influencing grain production technical efficiency. Furthermore, this study deepens our understanding of the relationship between government fiscal support for agriculture and grain production technical efficiency. The findings highlight the significance of fiscal support in grain production and offer valuable insights for other developing countries seeking to enhance grain production technical efficiency and formulate agricultural fiscal support policies.
Author contributions
All authors contributed to the study conception and design. Material preparation, data collection and analysis were performed by Zu Wang. The first draft of the manuscript was written by Zhihao Wu and all authors commented on previous versions of the manuscript. All authors read and approved the final manuscript.
Availability of data and materials
The first dataset is available from the first author on reasonable request. The second dataset analysed during the current study is available in the China City Statistical Yearbook repository, https://data.stats.gov.cn/easyquery.htm?cn=C01.
Acknowledgments
The authors acknowledge Zhejiang University for its support of this study.
Disclosure statement
No potential conflict of interest was reported by the author(s).
Additional information
Funding
Notes on contributors
Zu Wang
Zu Wang is a Ph.D. candidate in Agricultural Economics and Management from Zhejiang University. His research interests include agricultural technical progress and food security.
Zhihao Wu
Zhihao Wu is a PhD candidate in Agricultural Economics and Management from Zhejiang University. Her research interests include agricultural trade, digital agriculture, and rural development.
Longbao Wei
Longbao Wei is a distinguished professor at Zhejiang University, whose research field mainly focuses on food economics, agribusiness management, and digital economy.
Notes
1 Information source: Portal of the Central People’s Government of the People’s Republic of China (https://www.gov.cn/xinwen/2020–12/23/content_5572857.htm).
2 Control the influence change over farms, such us gender of the owner of the farm.
3 Control the influence change over years, such us external environment.
4 The data can be downloaded at https://data.stats.gov.cn/easyquery.htm?cn=C01.
5 See in Appendix for the definitions and summary statistics of the other variables..
References
- Adamopoulos, T., & Restuccia, D. (2014). The size distribution of farms and international productivity differences. American Economic Review, 104(6), 1667–29. https://doi.org/10.1257/aer.104.6.1667
- Aigner, D., Lovell, C. K., & Schmidt, P. (1977). Formulation and estimation of stochastic frontier production function models. Journal of Econometrics, 6(1), 21–37. https://doi.org/10.1016/0304-4076(77)90052-5
- Ali, D. A., Deininger, K., & Duponchel, M. (2014). Credit constraints and agricultural productivity: Evidence from rural Rwanda. The Journal of Development Studies, 50(5), 649–665. https://doi.org/10.1080/00220388.2014.887687
- Balana, B. B., Mekonnen, D., Haile, B., Hagos, F., Yimam, S., & Ringler, C. (2022). Demand and supply constraints of credit in smallholder farming: Evidence from Ethiopia and Tanzania. World Development, 159, 106033. https://doi.org/10.1016/j.worlddev.2022.106033
- Battese, G. E., & Coelli, T. J. (1992). Frontier production functions, technical efficiency and panel data: With application to paddy farmers in India. Journal of Productivity Analysis, 3(1–2), 153–169. https://doi.org/10.1007/BF00158774
- Battese, G. E., & Coelli, T. J. (1995). A model for technical inefficiency effects in a stochastic frontier production function for panel data. Empirical Economics, 20(2), 325–332. https://doi.org/10.1007/BF01205442
- Bevis, L. E., & Barrett, C. B. (2020). Close to the edge: High productivity at plot peripheries and the Inverse size-productivity relationship. Journal of Development Economics, 143, 102377. https://doi.org/10.1016/j.jdeveco.2019.102377
- Blancard, S., Boussemart, J. P., Briec, W., & Kerstens, K. (2006). Short‐ and long‐run credit constraints in French agriculture: A directional distance function framework using expenditure–constrained profit functions. American Journal of Agricultural Economics, 88(2), 351–364. https://doi.org/10.1111/j.1467-8276.2006.00863.x
- Brümmer, B., Glauben, Y., & Lu, W. (2006). Policy reform and productivity change in Chinese agriculture: A distance function approach. Journal of Development Economics, 81(1), 61–79. https://doi.org/10.1016/j.jdeveco.2005.04.009
- Byerlee, D., & Sain, G. (1986). Food pricing policy in developing countries: Bias against agriculture or for urban consumers? American Journal of Agricultural Economics, 68(4), 961–969. https://doi.org/10.2307/1242142
- Chari, A., Liu, E. M., Wang, S. Y., Wang, Y., & Schoenberg, U. (2021). Property rights, land misallocation, and agricultural efficiency in China. The Review of Economic Studies, 88(4), 1831–1862. https://doi.org/10.1093/restud/rdaa072
- Chen, W., & Ding, Y. (2007). Total factor productivity in Chinese agriculture: The role of infrastructure. Frontiers of Economics in China, 2(2), 212–223. https://doi.org/10.1007/s11459-007-0011-3
- Chen, S., & Gong, B. (2021). Response and adaptation of agriculture to climate change: Evidence from China. Journal of Development Economics, 148, 102557. https://doi.org/10.1016/j.jdeveco.2020.102557
- Chen, P., Yu, M., Chang, C., & Hsu, S. (2008). Total factor productivity growth in China’s agricultural sector. China Economic Review, 19(4), 580–593. https://doi.org/10.1016/j.chieco.2008.07.001
- Coelli, T. J., & Rao, D. P. (2005). Total factor productivity growth in agriculture: A Malmquist index analysis of 93 countries, 1980–2000. Agricultural Economics, 32(s1), 115–134. https://doi.org/10.1111/j.0169-5150.2004.00018.x
- Cornwell, C., Schmidt, P., & Sickles, R. C. (1990). Production frontiers with cross-sectional and time-series variation in efficiency levels. Journal of Econometrics, 46(1–2), 185–200. https://doi.org/10.1016/0304-4076(90)90054-W
- David, M. B. D. A., Dirven, M., & Vogelgesang, F. (2000). The impact of the new economic model on Latin America’s agriculture. World Development, 28(9), 1673–1688. https://doi.org/10.1016/S0305-750X(00)00047-4
- Deaton, B. J., & Deaton, B. J. (2020). Food security and Canada’s agricultural system challenged by COVID‐19. Canadian Journal of Agricultural Economics/Revue Canadienne D’agroeconomie, 68(2), 143–149. https://doi.org/10.1111/cjag.12227
- De Myttenaere, A., Golden, B., Le Grand, B., & Rossi, F. (2016). Mean absolute percentage error for regression models. Neurocomputing, 192, 38–48. https://doi.org/10.1016/j.neucom.2015.12.114
- Dercon, S., Gilligan, D. O., Hoddinott, J., & Woldehanna, T. (2009). The impact of agricultural extension and roads on poverty and consumption growth in fifteen Ethiopian villages. American Journal of Agricultural Economics, 91(4), 1007–1021. https://doi.org/10.1111/j.1467-8276.2009.01325.x
- Donaldson, D. (2018). Railroads of the Raj: Estimating the Impact of Transportation Infrastructure. American Economic Review, 108(4–5), 899–934. https://doi.org/10.1257/aer.20101199
- Ellis, F. (2008). The determinants of rural livelihood diversification in developing countries. Journal of Agricultural Economics, 51(2), 289–302. https://doi.org/10.1111/j.1477-9552.2000.tb01229.x
- Fan, S., Hazell, P., & Thorat, S. (2000). Government spending, growth and poverty in Rural India. American Journal of Agricultural Economics, 82(4), 1038–1051. https://doi.org/10.1111/0002-9092.00101
- Fan, S., Teng, P., Chew, P., Smith, G., & Copeland, L. (2021). Food system resilience and COVID-19–lessons from the Asian Experience. Global Food Security, 28, 100501. https://doi.org/10.1016/j.gfs.2021.100501
- Fan, S., & Zhang, X. (2008). Public expenditure, growth and poverty reduction in Rural Uganda. African Development Review, 20(3), 466–496. https://doi.org/10.1111/j.1467-8268.2008.00194.x
- Finger, R., Swinton, S. M., El Benni, N., & Walter, A. (2019). Precision farming at the Nexus of agricultural production and the environment. Annual Review of Resource Economics, 11(1), 313–335. https://doi.org/10.1146/annurev-resource-100518-093929
- Gao, Y., Wen, T., Yi, W., & Wang, X. (2013). A spatial econometric study on effects of fiscal and financial supports for agriculture in China. Agricultural Economics, 59(7), 315–332. https://doi.org/10.17221/126/2012-agricecon
- Gong, B. (2018a). Agricultural reforms and production in China: Changes in provincial production function and productivity in 1978–2015. Journal of Development Economics, 132, 18–31. https://doi.org/10.1016/j.jdeveco.2017.12.005
- Gong, B. (2018b). The impact of public expenditure and international trade on agricultural productivity in China. Emerging Markets Finance and Trade, 54(15), 3438–3453. https://doi.org/10.1080/1540496X.2018.1437542
- Gong, T. C., Battese, G. E., & Villano, R. A. (2019). Family farms plus cooperatives in China: Technical efficiency in crop production. Journal of Asian Economics, 64, 101129. https://doi.org/10.1016/j.asieco.2019.07.002
- Guirkinger, C., & Boucher, S. R. (2008). Credit constraints and productivity in Peruvian agriculture. Agricultural Economics, 39(3), 295–308. https://doi.org/10.1111/j.1574-0862.2008.00334.x
- Hassan, R. M., Faki, H., & Byerlee, D. (2000). The Trade-off between economic efficiency and food self-sufficiency in using Sudan’s irrigated land resources. Food Policy, 25(1), 35–54. https://doi.org/10.1016/S0306-9192(99)00063-9
- Hazell, P., & Varangis, P. (2020). Best practices for subsidizing agricultural insurance. Global Food Security, 25, 100326. https://doi.org/10.1016/j.gfs.2019.100326
- Headey, D., Alauddin, M., & Rao, D. P. (2010). Explaining agricultural productivity growth: An international perspective. Agricultural Economics, 41(1), 1–14. https://doi.org/10.1111/j.1574-0862.2009.00420.x
- Hoang, V. N., & Coelli, T. (2011). Measurement of agricultural total factor productivity growth incorporating environmental factors: A nutrients balance approach. Journal of Environmental Economics and Management, 62(3), 462–474. https://doi.org/10.1016/j.jeem.2011.05.009
- Huang, J., Gao, L., & Rozelle, S. (2012). The effect of off‐farm employment on the decisions of households to rent out and rent in cultivated land in China. China Agricultural Economic Review, 4(1), 5–17. https://doi.org/10.1108/17561371211196748
- Idoko, C. U., & Jatto, S. M. (2018). Government expenditure on agriculture and economic growth in Nigeria (1985–2015). International Journal of Academic Research and Reflection, 6(4), 24–39.
- Islam, A. R. M., & Luo, R. H. (2018). Financing constraints and investment efficiency: Evidence from a panel of Canadian forest firms. Applied Economics, 50(48), 5142–5154. https://doi.org/10.1080/00036846.2018.1478387
- Jin, S., Huang, J., Hu, R., & Rozelle, S. (2002). The creation and spread of technology and total factor productivity in China’s agriculture. American Journal of Agricultural Economics, 84(4), 916–930. https://doi.org/10.1111/1467-8276.00043
- Jin, S., Ma, H., Huang, J., Hu, R., & Rozelle, S. (2010). Productivity, efficiency and technical change: Measuring the performance of China’s transforming agriculture. Journal of Productivity Analysis, 33(3), 191–207. https://doi.org/10.1007/s11123-009-0145-7
- Karlan, D., Osei, R., Osei-Akoto, I., & Udry, C. (2014). Agricultural decisions after relaxing credit and risk constraints. The Quarterly Journal of Economics, 129(2), 597–652. https://doi.org/10.1093/qje/qju002
- Kassouri, Y., & Kacou, K. Y. T. (2022). Does the structure of credit markets affect agricultural development in west African countries? Economic Analysis and Policy, 73, 588–601. https://doi.org/10.1016/j.eap.2021.12.015
- Kelly, V., Adesina, A. A., & Gordon, A. (2003). Expanding access to agricultural inputs in Africa: A review of recent market development experience. Food Policy, 28(4), 379–404. https://doi.org/10.1016/j.foodpol.2003.08.006
- Köksoy, O. (2006). Multiresponse robust design: Mean square error (MSE) criterion. Applied Mathematics and Computation, 175(2), 1716–1729. https://doi.org/10.1016/j.amc.2005.09.016
- Kuang, Y., Yang, J., & Abate, M. C. (2021). Farmland transfer and agricultural economic growth nexus in China: Agricultural TFP intermediary effect perspective. China Agricultural Economic Review, 14(1), 184–201. https://doi.org/10.1108/CAER-05-2020-0076
- Kumbhakar, S. C. (1990). Production frontiers, panel data, and time-varying technical inefficiency. Journal of Econometrics, 46(1–2), 201–211. https://doi.org/10.1016/0304-4076(90)90055-X
- Labarthe, P., & Laurent, C. (2013). Privatization of agricultural extension services in the EU: Towards a lack of adequate knowledge for small-scale farms? Food Policy, 38, 240–252. https://doi.org/10.1016/j.foodpol.2012.10.005
- Lee, Y. H., & Schmidt, P. (1993). A production frontier model with flexible temporal variation in technical efficiency. In H. O. Fried, S. S. Schmidt, & C. K. Lovell (Eds.), The measurement of productive efficiency: Techniques and applications (pp. 237–255). Oxford University Press.
- Lewis, W. A. (1954). Economic development with unlimited supplies of labor. The Manchester School, 22(2), 139–191. https://doi.org/10.1111/j.1467-9957.1954.tb00021.x
- Liefert, W. M., Gardner, B., & Serova, E. (2003). Allocative efficiency in Russian agriculture: The case of fertilizer and grain. American Journal of Agricultural Economics, 85(5), 1228–1233. https://doi.org/10.1111/j.0092-5853.2003.00535.x
- Li, M., & Sicular, T. (2013). Aging of the labor force and technical efficiency in crop production: Evidence from Liaoning Province, China. China Agricultural Economic Review, 5(3), 342–359. https://doi.org/10.1108/CAER-01-2012-0001
- Li, H., Tang, M., Cao, A., & Guo, L. (2022). Assessing the relationship between air pollution, agricultural insurance, and agricultural green total factor productivity: Evidence from China. Environmental Science and Pollution Research, 29(52), 78381–78395. https://doi.org/10.1007/s11356-022-21287-7
- Liu, S., Zhang, S., He, X., & Li, J. (2016). Efficiency change in North-East China agricultural sector: A DEA approach. Agricultural Economics, 61(11), 522–532. https://doi.org/10.17221/233/2014-AGRICECON
- Liu, Z., & Zhuang, J. (2000). Determinants of technical efficiency in post-collective Chinese agriculture: Evidence from farm-level data. Journal of Comparative Economics, 28(3), 545–564. https://doi.org/10.1006/jcec.2000.1666
- Lu, Y. (2012). Education of children left behind in Rural China. Journal of Marriage & Family, 74(2), 328–341. https://doi.org/10.1111/j.1741-3737.2011.00951.x
- Ma, W., Renwick, A., & Grafton, Q. (2018). Farm machinery use, off-farm employment and farm performance in China. Australian Journal of Agricultural and Resource Economics, 62(2), 279–298. https://doi.org/10.1111/1467-8489.12249
- McArthur, J. W., & McCord, G. C. (2017). Fertilizing growth: Agricultural Inputs and their effects in economic development. Journal of Development Economics, 127, 133–152. https://doi.org/10.1016/j.jdeveco.2017.02.007
- Meeusen, W., & van Den Broeck, J. (1977). Efficiency estimation from Cobb-Douglas production functions with composed error. International Economic Review, 18(2), 435–444. https://doi.org/10.2307/2525757
- Mendelsohn, R. (2003). The challenge of conserving indigenous domesticated animals. Ecological Economics, 45(3), 501–510. https://doi.org/10.1016/S0921-8009(03)00100-9
- Monchuk, D. C., Chen, Z., & Bonaparte, Y. (2010). Explaining production inefficiency in China’s agriculture using data envelopment analysis and semi-parametric bootstrapping. China Economic Review, 21(2), 346–354. https://doi.org/10.1016/j.chieco.2010.02.004
- Nguyen, T. P. T., Nghiem, S. H., Roca, E., & Sharma, P. (2016). Bank reforms and efficiency in Vietnamese banks: Evidence based on SFA and DEA. Applied Economics, 48(30), 2822–2835. https://doi.org/10.1080/00036846.2015.1130788
- Nin, A., Arndt, C., & Preckel, P. V. (2003). Is agricultural productivity in developing countries really shrinking? New evidence using a modified nonparametric approach. Journal of Development Economics, 71(2), 395–415. https://doi.org/10.1016/S0304-3878(03)00034-8
- Nurkse, R. (1952). Some international aspects of the problem of economic development. American Economic Review, 42(2), 571–583.
- Odeck, J. (2007). Measuring technical efficiency and productivity growth: A comparison of SFA and DEA on Norwegian grain production data. Applied Economics, 39(20), 2617–2630. https://doi.org/10.1080/00036840600722224
- Pan, W., Zhuang, M., Zhou, Y., & Yang, J. (2021). Research on sustainable development and efficiency of China’s E-Agriculture based on a data envelopment analysis-Malmquist model. Technological Forecasting and Social Change, 162, 120298. https://doi.org/10.1016/j.techfore.2020.120298
- Pinstrup-Andersen, P., & Shimokawa, S. (2006). Rural infrastructure and agricultural development. In Rethinking infrastructure for development (pp. 175–203). World Bank.
- Qaim, M. (2020). Role of new plant breeding technologies for food security and sustainable agricultural development. Applied Economic Perspectives and Policy, 42(2), 129–150. https://doi.org/10.1002/aepp.13044
- Rada, N. E., & Fuglie, K. O. (2019). New perspectives on farm size and productivity. Food Policy, 84, 147–152. https://doi.org/10.1016/j.foodpol.2018.03.015
- Reardon, T., Crawford, E., & Kelly, V. (1994). Links between nonfarm income and farm investment in African households: Adding the capital market perspective. American Journal of Agricultural Economics, 76(5), 1172–1176. https://doi.org/10.2307/1243412
- Rezitis, A. N. (2010). Agricultural productivity and convergence: Europe and the United States. Applied Economics, 42(8), 1029–1044. https://doi.org/10.1080/00036840701721026
- Rockoff, J. E. (2004). The impact of individual teachers on student achievement: Evidence from panel data. American Economic Review, 94(2), 247–252. https://doi.org/10.1257/0002828041302244
- Rubenstein, K. D., Heisey, P. W., Klotz-Ingram, C., & Frisvold, G. B. (2003). Competitive grants and the Funding of Agricultural Research in the United States. Applied Economic Perspectives and Policy, 25(2), 352–368. https://doi.org/10.1111/1467-9353.00143
- Ruttan, V. W. (2002). Productivity growth in World Agriculture: Sources and constraints. Journal of Economic Perspectives, 16(4), 161–184. https://doi.org/10.1257/089533002320951028
- Schmidt, P., & Sickles, R. C. (1984). Production frontiers and panel data. Journal of Business & Economic Statistics, 2(4), 367–374. https://doi.org/10.1080/07350015.1984.10509410
- Schultz, T. W. (1964). Transforming traditional agriculture. Yale University Press.
- Shamdasani, Y. (2021). Rural road infrastructure & agricultural production: Evidence from India. Journal of Development Economics, 152, 102686. https://doi.org/10.1016/j.jdeveco.2021.102686
- Sheng, Y., & Chancellor, W. (2019). Exploring the relationship between farm size and productivity: Evidence from the Australian grains industry. Food Policy, 84, 196–204. https://doi.org/10.1016/j.foodpol.2018.03.012
- Sickles, R. C. (2005). Panel estimators and the identification of firm-specific efficiency levels in Parametric, semiparametric and nonparametric settings. Journal of Econometrics, 126(2), 305–334. https://doi.org/10.1016/j.jeconom.2004.05.004
- Smith, A. (1776). An inquiry into the nature and causes of the wealth of nations. Strahan.
- Stads, G. J., & Beintema, N. (2015). Agricultural R&D expenditure in Africa: An analysis of growth and volatility. The European Journal of Development Research, 27(3), 391–406. https://doi.org/10.1057/ejdr.2015.25
- Suhariyanto, K., & Thirtle, C. (2008). Asian agricultural productivity and convergence. Journal of Agricultural Economics, 52(3), 96–110. https://doi.org/10.1111/j.1477-9552.2001.tb00941.x
- Tan, S., Heerink, N., Kuyvenhoven, A., & Qu, F. (2010). Impact of land fragmentation on rice producers’ technical efficiency in South-East China. NJAS–Wageningen Journal of Life Sciences, 57(2), 117–123. https://doi.org/10.1016/j.njas.2010.02.001
- Teruel, R. G., & Kuroda, Y. (2005). Public infrastructure and productivity growth in Philippine agriculture, 1974–2000. Journal of Asian Economics, 16(3), 555–576. https://doi.org/10.1016/j.asieco.2005.04.011
- Tian, W., & Wan, G. H. (2000). Technical efficiency and its determinants in China’s grain production. Journal of Productivity Analysis, 13(2), 159–174. https://doi.org/10.1023/A:1007805015716
- Timmer, C. P. (1995). Getting agriculture moving: Do markets provide the right signals? Food Policy, 20(5), 455–472. https://doi.org/10.1016/0306-9192(95)00038-G
- Wallach, D., & Goffinet, B. (1989). Mean squared error of prediction as a criterion for evaluating and comparing system models. Ecological Modelling, 44(3–4), 299–306. https://doi.org/10.1016/0304-3800(89)90035-5
- Wang, J., Chen, K. Z., Das Gupta, S., & Huang, Z. (2015). Is small still beautiful? A comparative study of rice farm size and productivity in China and India. China Agricultural Economic Review, 7(3), 484–509. https://doi.org/10.1108/CAER-01-2015-0005
- Wang, X., Yamauchi, F., Huang, J., & Rozelle, S. (2020). What constrains mechanization in Chinese agriculture? Role of farm size and fragmentation. China Economic Review, 62, 101221. https://doi.org/10.1016/j.chieco.2018.09.002
- Willmott, C., & Matsuura, K. (2005). Advantages of the Mean Absolute Error (MAE) over the Root Mean Square Error (RMSE) in assessing average model performance. Climate Research, 30, 79–82. https://doi.org/10.3354/cr030079
- Wu, Z., Dang, J., Pang, Y., & Xu, W. (2021). Threshold effect or spatial spillover? The impact of agricultural mechanization on grain production. Journal of Applied Economics, 24(1), 478–503. https://doi.org/10.1080/15140326.2021.1968218
- Wu, Z., Liu, M., & Davis, J. (2005). Land consolidation and productivity in Chinese household crop production. China Economic Review, 16(1), 28–49. https://doi.org/10.1016/j.chieco.2004.06.010
- Yuan, L., Zhang, S., Wang, S., Qian, Z., & Gong, B. (2021). World agricultural convergence. Journal of Productivity Analysis, 55(2), 135–153. https://doi.org/10.1007/s11123-021-00600-5
- Zhang, Y., & Brümmer, B. (2011). Productivity change and the effects of policy reform in China’s agriculture since 1979. Asian‐Pacific Economic Literature, 25(2), 131–150. https://doi.org/10.1111/j.1467-8411.2011.01307.x
- Zhang, D., Wang, H., Lou, S., Zhong, S., & Zúniga-González, C. A. (2021). Research on grain production efficiency in China’s main grain producing areas from the perspective of financial support. Public Library of Science ONE, 16(3), e0247610. https://doi.org/10.1371/journal.pone.0247610
- Zhang, Q., Zhang, F., Wu, G., & Mai, Q. (2021). Spatial spillover effects of grain production efficiency in China: Measurement and scope. Journal of Cleaner Production, 278, 121062. https://doi.org/10.1016/j.jclepro.2020.121062
- Zheng, Z., Cheng, S., & Henneberry, S. R. (2023). Total factor productivity change in China’s grain production sector: 1980–2018. Australian Journal of Agricultural and Resource Economics, 67(1), 38–55. https://doi.org/10.1111/1467-8489.12495
- Zhong, T., Zhang, X., Huang, X., & Liu, F. (2019). Blessing or curse? Impact of land finance on rural public infrastructure development. Land Use Policy, 85, 130–141. https://doi.org/10.1016/j.landusepol.2019.03.036
Appendix
Table A1. Statistical tests for production function selection.
Table A2. Summary statistics for the remaining variables.
Table A3. Summary statistics by waves.