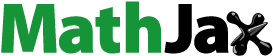
Abstract
We study the intraday relationship between novel Euro-specific news and the EURUSD exchange rate with over 100 million news articles with millisecond precision. We show a predictive but not contemporaneous relationship between these news and their sentiment and EURUSD returns. This lends support to the slow information diffusion hypothesis as well as rational inattention to new news theories. Furthermore, we identify a regime change in the relationship between news and the EURUSD returns since the Global Financial Crisis (GFC). The positive relationship observed since the GFC between news sentiment and the EURUSD was particularly prominent during the Eurozone debt crisis.
Is there fast or slow information diffusion into FX prices in the high-frequency space?
Do news releases get fully reflected in the price as soon as they are released, or does it take some time for market participants to process them? With the constant increase of high-frequency trading (HFT) in global markets over the past two decades, one can imagine that at least some market participants process and potentially act immediately on novel news. However, to which extent are markets efficient in the high-frequency space? And, in particular, foreign exchange markets, which are highly exposed and driven by macroeconomic, monetary policy, and geo-political news. Most high-frequency studies have been in the equities space, and the proportional market share of high-frequency traders by asset class is the highest in equity markets.Footnote1 In the most recent Euromoney FX survey, the current ranking reveals some high-frequency traders have made their way up to the top 10, only second to a few big banks.Footnote2
It has been widely debated whether high-frequency traders are in fact a potential cause of market-moving events, and, in particular, to flash crashes, or liquidity providers, which are essential to have during market turmoils. In such a case, high-frequency traders would act as a stabilizing force in the market microstructure. The latter appears to be the case, as Kirilenko et al. (Citation2017) note in their extensive study on the Flash Crash, which occurred on May 6, 2010 in the S&P 500 E-mini futures markets. The authors find that” the trading pattern of the most active nondesignated intraday intermediaries (classified as High-Frequency Traders) did not change when prices fell during the Flash Crash.” So for equity markets, it could well be the case that novel news (or no news in the case of the Flash Crash) can trigger significant price actions, which are exacerbated by high-frequency traders.
Does the same market microstructure apply to the FX markets, which are typically seen as the most volatile across global investable asset classes? Are FX markets similarly influenced as equity markets? Do novel news get immediately reflected into FX prices in a similar fashion as in equity prices? shows the EURUSD movement on the day when S&P downgraded Greek sovereign debt to non-investment grade. The risk therefore significantly increased that Greece could default on its debt, which in turn triggered a debate about a potential exit of Greece from the Eurozone (a.k.a. Grexit). This sparked a debate about the viability of the Euro as a common currency in the Eurozone with major monetary policy and geo-political consequences. This uncertainty caused the Euro to depreciate days and weeks after the rating announcement of S&P. Since the depreciation occurred even weeks after the downgrade announcement, it implied that investors needed time to put the news in perspective and fully understand their magnitude because such event had not happened in the past before. In such events it is helpful to think about the marginal investor. After the rating downgrade, the marginal investor constantly decided to sell euros and buy US dollars for two reasons: first, the euro was significantly weakened because this was the first time in history that it was even debated among policy makers that a country could exit the Eurozone, and, second, the US was once more seen as a safe haven.
Figure 1. EURUSD sample of minute time series from 2010-04-21 to 2010-06-12. The red line indicates the time when S&P announced the downgrade of Greek debt to non-investment grade. The straight connecting lines indicate missing data over weekends.

Similar events can be identified when news can affect exchange rate developments with many studies showing the significance of the effect of macroeconomic announcements on the EURUSD exchange rate and on other financial asset prices.Footnote3 There have also been studies looking at sentiment of news showing that it takes time to influence equity, bond and foreign exchange prices.Footnote4 All of these studies have been undertaken with low frequency time series (typically daily or monthly). However, to the best of our knowledge, no study has considered the high frequency effect of news sentiment on exchange rates.Footnote5 How do in particular novel news affect foreign exchange rates? Does the price fully absorb new information instantaneously, or does it take some time for novel news to get incorporated into prices? How long is this effect and would it be possible to exploit it with a trading strategy?
We attempt to find answers to all of the above questions in this paper. In order to tackle this empirically, we use a global news database containing 16 years of news and their sentiment scores amounting to 100 million news articles in microsecond precision, ranging from 2003 to 2018. We filter and process the data to generate a high-frequency time series that reflects the novel news and their sentiment toward Europe and the EURUSD currency pair. We then show that this particular news sentiment time series predicts EURUSD returns 1 hour and beyond into the future. However, we do not find any significant relationship between EURUSD returns and novel Euro-specific news on a contemporaneous basis.
This proves that it in fact does take time for market participants to process novel news and that the entire information surprise is not immediately incorporated into FX prices when looked at on an intraday basis. Our results are in line with the findings in the current literature on low frequencies, showing that also in the low-frequency space it takes days and even weeks for the news sentiment effect to materialize.Footnote6 Additionally, our findings can even be linked to the rational inattention literature, as we only consider novel news. In order to test the relevance of such data in a real-life setting, we develop and backtest a trading strategy based on the high-frequency news sentiment data. We identify some inefficiencies in markets, but these inefficiencies disappear once we take into account transaction costs.
The remainder of the paper is structured as follows: the next section reviews the existing literature followed by a section on the data used for the analysis. The next sections discuss the empirical results, robustness checks and backtesting results, while the last section concludes.
Literature review
One strain of research in the behavioral finance literature is concerned with the influence of news on asset prices. Evans and Lyons (Citation2008) find that the arrival of macro news can account for more than 30% of daily price variance on the DM/USD exchange rate. In addition, they find that order flow is higher after macroeconomic news releases. This is contrary to Fama (Citation1970) who claims that new knowledge is instantly impounded in the price.
In terms of empirical papers quantitatively assessing the tone of news articles, Tetlock (Citation2007) was one of the first to apply textual analysis for predicting equity prices in the time series. In this study, Tetlock used a simple bag-of-words algorithmFootnote7 on news from the Wall Street Journal to show that pessimistic news predict falling stock prices. In addition, Tetlock, Saar-Tsechansky, and Macskassy (Citation2008) find that the fraction of negative words in news related to a specific firm predict low earnings of that firm in the cross-section.
While the above studies were certainly ground-breaking in terms of applying textual analysis to predict stock returns or earnings, the way how the sentiment of text was generated has since been developed further. Academics and professional vendors alike have worked on sentiment analysis with machine-readable news (MRN)Footnote8 as well as finance-specific dictionaries, such as the one from Loughran and Mcdonald (Citation2011). Loughran and Mcdonald (Citation2011) analyze 10 K filings and find that if word lists developed for other disciplines to define negative sentiment are used, there will be 73.8% of the words misclassified as negative that might not be considered negative in a financial context.Footnote9 The Loughran and Mcdonald (Citation2011) dictionary has become one of the standard word lists used in the textual analysis of financial datasets, as used by Glasserman and Mamaysky (Citation2019). Most recently, Mamaysky (Citation2019) has applied the Loughran and Mcdonald (Citation2011) dictionary in combination with a topic search on COVID-19 in order to assess financial market response to sentiment about COVID-19 related news.
Within the realm of the interaction of foreign exchange and news-induced price moves, the more established and most extensive approach in the literature is to consider scheduled macroeconomic announcements for non-farm payrolls, ISM manufacturing, unemployment rate, inflation, GDP, or others, to explain the movements in currency pairs. Galati and Ho (Citation2003), for instance, use rolling regressions to show that there is a significant time variation of macroeconomic news to foreign exchange rate moves, which lead to parameter instabilities. Parameter instabilities occur when good news sometimes lead to positive returns, sometimes to negative returns, while at other times the market ignores the news. Therefore, the coefficient of their rolling regressions is unstable in the time-domain. They find that the news impact was strongest when news switched from positive to negative. They also find that looking at the exchange rate from Euro-area news and US-area news does not yield symmetric results. The hypothesis would be that the EURUSD exchange rate reacts positively to good news from the Euro area, and negatively to positive news from the US. However, the authors find that this is not always the case. One potential explanation for this price behavior could be the changing central bank policies and the subsequent interpretation of the marginal investor.
When considering alternative measures for news-induced shocks on foreign exchange prices, S. Feuerriegel, Wolff, and Neumann (Citation2016) show that news sentiment can explain the exchange rate forecasting error variance. He also finds statistical evidence that a shock in news sentiment might result in an overshooting of the exchange rate. Uhl (Citation2017) constructs news sentiment momentum specifically for the EURUSD exchange rate using kernel smoothing techniques with various harmonic functions. He identifies news sentiment momentum as an additional factor for explaining daily moves in the EURUSD exchange rate. He finds that news sentiment momentum is uncorrelated to price momentum.
Most of the existing news sentiment studies have considered aggregated data in the lower frequency space (i.e. using daily, weekly or monthly data). However, since news get released in real-time and this is also how most market participants consume these news, it makes sense to look at higher-frequency effects of news and their sentiment on asset prices and foreign exchange rates in particular, as we do in this study. The foreign exchange rate market is typically highly receptive of immediate macroeconomic news, but also of any other news related to monetary policy, geo-politics and the like, which makes this market ideal to study in relation to real-time news announcement (both for scheduled and unscheduled news).
Groß-Klußmann and Hautsch (Citation2009) and Groß-Klußmann and Hautsch (Citation2011) were probably the first to do an intraday study using data from an automated news engine called Reuters NewsScope Sentiment Engine.Footnote10 They look at intraday effects on stocks, using sentiment from 35 companies listed on the London Stock Exchange. They find that trading volume and volatility react to news sentiment, but only when news items are classified as highly relevant. Evans (Citation2011) shows that jumps in EURUSD, S&P 500 futures and bond futures are related to macroeconomic announcements. The jumps following the news are significantly higher than the jumps that do not follow a news announcement, and the informational surprise of the news announcement can explain large proportions of the following jump. Conrad and Lamla (Citation2010) extract sentiment from ECB press releases to predict the EURUSD exchange rate in a high-frequency setup (5-minute intervals). They fit an AR-FIGARCH model to the exchange rate and show that the tone in ECB communication can explain currency movements. Their research focuses mostly on explaining the volatility around ECB communication.
In this study, we consider all novel news (both ad-hoc and scheduled) and their sentiment relating to the EURUSD exchange rate in a high-frequency setting in order to test for predictability of sentiment on the one hand, and, if present, for the duration of this effect. Our study contributes to the behavioral finance literature in general and to the news sentiment literature in particular as this is the first study testing the effect of sentiment generated from both scheduled and unscheduled novel news on the EURUSD in a high-frequency setting.
Data
High-frequency foreign exchange data
The high-frequency EURUSD time series is obtained from the website with a web-scraping tool that we produced for this paper. The scraper downloads all monthly files of the EURUSD time series with 1-minute intervals from the website, concatenates the files into one single time series file while removing duplicates and buggy data (such as negative exchange rates or duplicate data for the same time interval). For the validation of the time series, we compare it with data from Bloomberg. We check minute-by-minute at randomly chosen samples for different months and years, and identify a discrepancy with the documentation. While the data documentation of HistData.com claims that the timezone of all data is Eastern Standard Time (EST) without day light savings adjustments, we note that it does have daylight savings adjustment, so that we correct for this while constructing our database. Additionally, we shift the time series from Eastern Standard Time (EST) to the standard Coordinated Universal Time (UTC), so that it matches the timezone used for the news sentiment time series, which is described in the next section. In total, we then have high-frequency data with 1-minute intervals for the EURUSD exchange rate from 1 January 2003 until 31 December 2018, totaling in over 6.6 million data points.
One caveat of the EURUSD dataset is that we have 1-minute data for the last best bid quotes, but not for the last ask quotes. Hence, we cannot calculate a time series of mid quotes as follows: Instead, we use the time series of the best bid quotes. Since the EURUSD is considered to be the most liquid FX pair, as Salmon (Citation2014) notes, the best bid quote is a good proxy for the current market price. We therefore assume that the difference between the bid and mid quote is not big enough to have any major impact on our results. Additionally, we assume that the best bid and best mid quote would move most of the time in tandem.
In terms of data cross-checks, we do further quality checks and note the following: naturally, we do not have data for weekends and on holidays when financial markets are closed. However, FX markets are open 24/5 with continuous global trading throughout the day, so that we typically have continuous data during the week. We classify missing data whenever we do not have any data points for at least one hour during any trading day (since we consider hourly news sentiment for the empirical setup as we describe in the following sections). The missing values that we identify represent less than 1% of the dataset. Even though the share of missing data is negligible, it is important when we run regressions later that need evenly spaced intervals without missing data. We can deal with this in two ways. In order not to introduce a bias to our dataset, we filter out all of the datapoints in the regression that have a missing EURUSD price when aligning the data later with the news sentiment data. A second possible solution would be to linearly interpolate the missing values. However, we would then introduce a bias to our analysis, as we would be trading on non-existent data. We choose the first option.
In , we check the average distribution of price data throughout the day. On average, we have a 17% difference between the most represented hour (13:00, 245’826 minute datapoints) and the least represented hour (21:00, 204’276 minute datapoints). The most represented hour (13:00) is typically the time when US markets open and European markets are in the middle of the trading session with Asian evening trading hours. The least represented hour (21:00) in terms of data points is intuitive as Europe, Asia and the US then experience night time hours.
Figure 2. Total count of 1-minute datapoints for each hour throught the day from 1 January 2003 to 31 December 2018.

In order to get further intuition for the data, we show a boxplot of EURUSD logreturns in for each hour. It might be possible that shows a lower dispersion of data for hours 3:00 and 21:00, because we have less datapoints for those hours, and hence we do not generate a comparable distribution. In order to cross-check, we randomly choose 200.000 points at each hourly interval from our datasets and generate the boxplot again. This time, we have equal sample sizes for every hour of the day. shows the results. The box plot looks almost the same as the unsampled one, which points to a higher dispersion of returns when Europe and the US are experiencing busy trading hours.
Figure 3. Boxplot of 1-minute EURUSD log returns, grouped by hour, average per day over full sample from 1 Jan 2003 - 31 Dec 2018.

Figure 4. Randomly sampled boxplot of 1-minute EURUSD log returns, grouped by hour, average per day over full sample from 1 Jan 2003 - 31 Dec 2018.

We run an Augmented Dickey–Fuller (ADF) test to check for a unit root in the EURUSD time series. We cannot reject the null hypothesis that the EURUSD exchange rate has a unit root. Therefore, we calculate EURUSD logreturns Rt as follows:
(1)
(1)
where Vt is the final value,
the initial value and t refers to a 1-minute interval. For log returns of the EURUSD, the t-value of the ADF test is −223, so that we can reject the null hypothesis that the process has a unit root. In all future references when we refer to the EURUSD time series, we refer to log returns of the EURUSD.
High-frequency news sentiment data
The news media has seen a strong increase in published articles over the last decade, in particular owing to the rise of the news generation by Natural Language Generation (NLG) algorithms. This has been the case for over a decade and is evolving, as Doerr (Citation2016) has described. While there are limitations to NLG, the use cases are already quite wide. In a more recent study, Tandoc, Yao, and Wu (Citation2020) argues that due to budget constraints, many traditional news providers are moving more and more toward generating automated news. Interestingly, they compare human- and machine-written news and find that there are no main differences in perceived source or message credibility between algorithm, human, and even a mixture of the two. This development in NLG has also put more focus on Natural Language Processing (NLP), which takes news texts and structurally analyzes them into tone, topic, company identifier, and other metadata classifiers. As one of the major global news providers, Thomson Reuters has a product called Thomson Reuters News Analytics (TRNA), which analyzes their entire news universe with a proprietary algorithm classifying each news article into tone (i.e. sentiment), topics, company identifiers, and over 40 other parameters of metadata. The NLP algorithm from TRNA is a combination of bag-of-words dictionaries and expert opinions combined in a neural network. Heston and Sinha (Citation2017) describe in detail how this algorithm is defined.
TRNA provides 40 parameters per individual news item. For this study, we focus on the following parameters:
Headline - The headline text of the news item. Note that the full text of the news article is not available in our dataset.
FeedTimestamp - UTC timestamp showing when the news item was released in the news feed with millisecond precision.
AssetId - Primary identifier for the asset. PermID for companies and topic codes for commodities.
Relevance - A decimal number indicating the relevance of the news item to the asset ranging from 0 to 1.
SentimentClass - This field indicates the predominant sentiment class for each news item with respect to the asset for each news article. The indicated sentiment class is the one with the highest probability value among the three for each article: positive (1), neutral (0), and negative (-1).
Subjects - Topic codes and company identifiers that relate to this news item.
Urgency - Differentiates story types. For this study, we focus on alerts only, as we are interested in novel and not stale or old news.
The available TRNA data is high-frequency tick data ranging from 1 January 2003 to 31 December 2018. We build a tick-by-tick database filtering for all messages with subject codes related to foreign exchange news in combination with EURUSD-specific currency news (N2:EUR and N2:FRX). We also only filter for alerts, as we are interested in novel news only, which are supposed to impact currency markets immediately.
As a robustness check whether we have filtered for the correct news content, we validate the TRNA dataset on a random sample of news. We search online for headlines of the original news which match the headlines of news from our database.Footnote11 We then compare the time stamps of the news article found online and in the TRNA database. After converting to the appropriate timezone (UTC), we find that the timestamps for the randomly sampled news items from the website and the one from the database match. This confirms that the timestamps from the news in the database are accurate.Footnote12
In order to do a similar cross-check as for the high-frequency EURUSD data, we construct a bar chart with the average amount of news for each hour during UTC timezone over the full sample, shown in . We note that in the early and very late hours of the day, the news volume is significantly lower than during normal business hours of Europe, which is intuitive and as expected, as we are selecting news related to Euro-specific events.
Figure 5. Plot of news, grouped by hour, average per day over full sample from 1 Jan 2003 - 31 Dec 2018.

Since the news occur in irregular time intervals when considered in a high-frequency setting, we need to transform the time series and align with the high-frequency EURUSD time series as described in the previous section.Footnote13 We opt for the most simple de-noising option by constructing a simple moving average on a rolling basis with 1-hour time intervals. With this transformation we obtain a time series with evenly spaced time intervals, enabling us to run high-frequency regressions in order to test the data further.
In , we show the empirical distributions of the hourly rolling average news sentiment time series at every hour of the day. We can clearly see that the news sentiment is mostly skewed toward negative values with all hourly means below zero.
Figure 6. Boxplot of hourly rolling moving averages of Euro-specific news sentiment from 1 Jan 2003 to 31 Dec 2018.

Since we would like to have a balanced predictor ideally with a mean around zero, we first difference the news sentiment time series. depicts the boxplot for this new time series, showing that the intraday dependency disappears. An ADF test shows that this time series does not have a unit root. For the empirical analysis discussed in the next section, we will apply differenced news sentiment going forward.
Figure 7. Boxplot of differenced hourly rolling moving averages of Euro-specific news sentiment from 1 Jan 2003 to 31 Dec 2018.

In order to get more intuition for the available news data, we show the time series of hourly rolling moving averages of the Euro-specific new sentiment in and plot the EURUSD exchange rate next to it before and after Greek debt got downgraded. The vertical line indicates when S&P announced the downgrade of Greek sovereign debt.Footnote14 While the news sentiment time series is quite noisy, we can nevertheless observe that the sentiment turned negative well before Greek debt got downgraded. The EURUSD reacted shortly thereafter with a sudden drop. In the next section, we assess the interaction between news sentiment and EURUSD returns more broadly.
Empirical analysis
Regression analysis
We want to assess the relationship between the EURUSD exchange rate and Euro-specific news sentiment in a high-frequency framework. As a start, we run simple ordinary least squares (OLS) regressions in the following contemporaneous and predictive manner:
(2)
(2)
Where:
= EURUSD log return from time i to time i + 1.
β0 = intercept,
1-hour rolling moving average of Euro-specific news sentiment that is published between time i − 1 and time i. Note that for the contemporaneous regression we use same period EURUSD log returns and differenced sentiment, whereas for the predictive regressions we lag sentiment by 1 hour,
= one period lagged EURUSD log return,
- denotes the time, ranging from 1 Jan 2003 to 31 Dec 2018. The interval between i and i + 1 is 1 hour in the regression.
The contemporaneous regression results are displayed in in column 1. We do not find a statistically significant relationship between news sentiment and EURUSD returns in the same 1-hour time interval. Column 2 in shows the result of the predictive regression, in which we regress 1-hour lagged news sentiment on EURUSD log returns, while controlling for autocorrelation in prices from the previous time interval. In this regression, the news sentiment coefficient is positive and statistically significant at the 1%-level. These findings bear interesting implications. First, it appears that Euro-specific news and their sentiment have a positive relationship with EURUSD returns, although with a delay of at least one hour. This means that if the sentiment of the news are positive (negative) during one hour, this typically causes the EURUSD to go up (down) in the next hour. Second, since we do not find statistically significant evidence of a relationship between news sentiment and EURUSD returns in the same hour, we postulate that investors need at least one hour to incorporate novel news into prices. Therefore, there is some delay in the information processing of new information.
Table 1. This Table shows a contemporaneous and predictive regression between differenced sentiment and EURUSD log returns of 1-hour periods, controlling for price momentum using only alert type news (i.e. news that are new content and not commentaries on previously published news).
In , we show longer-period time intervals for the regressions, namely for 1-day and 2-day intervals. Both sentiment regression coefficients are statistically significant and positive. This is in line with previous research done in the lower frequency space, and as in Uhl (Citation2017).
Table 2. This Table shows predictive regressions between differenced sentiment and EURUSD log returns of 1 and 2 day periods, using only alert type news (i.e. news that are new content and not commentaries on previously published news).
Additionally, we run 1-year rolling predictive regressions with hourly news sentiment and EURUSD log returns in the same manner as specified above (including the relevant control variables). shows the results over time. We make several interesting observations. Up until the global financial crisis (GFC) in 2008, the regression coefficient of news sentiment was negative, meaning that there was an inverse relationship between news sentiment and EURUSD returns. It is difficult to identify why this was the case, but it is probably fair to say that the market dynamics before the GFC were different than afterwards. Since 2010, the regression coefficient of news sentiment has turned positive. This positive relationship was particularly the case after 2010 and the subsequent years during the Eurozone debt crisis. We can clearly see that EURUSD returns became very sensitive to news and their sentiment related to Euro-specific topics. From 2010 onwards, the majority of these news centered around the European debt crisis and massive policy intervention by the ECB. The value of the regression coefficient reached its peak in 2012. That year, the then president of the European Central Bank (ECB), Mario Draghi, made his famous statement on 26 July 2012 that” […] the ECB is ready to do whatever it takes […].”Footnote15 When the Eurozone crisis eased, the news sentiment coefficient also decreased, but stayed positive. Since 2015, the sentiment regression coefficient has gradually increased, but is most recently still not at the levels seen during the Eurozone crisis. Candelon, Sy, and Arezki (Citation2011) make similar findings as they show that investors interpret positive news on the Eurozone differently since the European debt crisis in 2010.
Figure 9. This figure shows 1-year rolling regression coefficients of differenced hourly Euro-specific news sentiment regressed on previous hour EURUSD log returns while controlling for momentum with a lagged hourly EURUSD log return. Standard errors are heteroskedasticity and autocorrelation consistent based on Newey-West (HAC).

In order to test for robustness of the obtained results, we check how the possible relationship between news sentiment and EURUSD returns dissipates through time. We run the above regressions for different time intervals as follows:
(3)
(3)
= EURUSD log return from time j to time j + 1.
β0 = intercept,
k-period rolling moving average of differenced Euro-specific news sentiment that is published between time k − 1 and time k,
= one period lagged EURUSD log return,
- denotes the time, ranging from 1 Jan 2003 to 31 Dec 2018. We vary the time interval between j and j + 1 from 1 minute to 2 days (2880 minutes).
- denotes the time, ranging from 1 Jan 2003 to 31 Dec 2018. We vary the time interval between k and k + 1 from 1 minute to 2 days (2880 minutes).
For ease of interpreting the results, we display the results of all regression coefficients of Euro-specific news sentiment from the above regression in a three dimensional surface plot. shows the overall results. On the y-axis, we display the regression coefficient of the independent variable news sentiment with the news sentiment time period k ranging from 1 minute to 2880 minutes (i.e. 2 days). The z-axis displays the respective EURUSD time period j considered for the dependent variable, also ranging from 1 to 2880 minutes.Footnote16 We can see that with an increase in the time interval both for the sentiment and EURUSD return window, we generally obtain a higher regression coefficient for news sentiment. Overall, we can only observe positive regression coefficients, indicating a positive relationship between news sentiment and subsequent EURUSD log returns. This is in line with the previous findings made for the time period considered, particularly after the GFC.
Figure 10. Surface plot showing news sentiment regression coefficients based on predictive regressions with different lookback windows for both the dependent and independent variables. The dependant variable is EURUSD log returns, and the independent variable is a rolling average of news sentiment with different rolling lookback lengths.

However, since all of the regressions coefficients are quite low, there is a possibility that we obtain this relationship by chance. We use the empirical results from Ferson, Sarkissian, and Simin (Citation1998) to make the p-values of our regressions more robust and set the lag in the Newey-West regression much larger than the autocorrelation window might be - we set it to 14 in all of our regressions, which is common standard. We then plot the surface again, but only show the significant coefficients this time. shows the results. Although the surface plot is somewhat decimated, we can nevertheless still observe a similar pattern as in the previous plot: we obtain higher regression coefficients for news sentiment with an increase in the lookback window for both news sentiment and for EURUSD log returns. We always observe a positive relationship between news sentiment and subsequent EURUSD log returns, making our initial findings more robust.
Figure 11. Surface plot showing news sentiment regression coefficients based on predictive regressions with different lookback windows for both the dependent and independent variables. The dependant variable is EURUSD log returns, and the independent variable is a rolling average of news sentiment with different rolling lookback lengths. We only display regression coefficients with

Our findings lend additional insights related to the slow information diffusion hypothesis, as outlined in Boguth et al. (Citation2016) since we identify a slow reaction to novel market information (relatively speaking in high-frequency terms). Our findings also lend support to rational inattention theory and portfolio decisions. As Huang and Liu (Citation2007) show, it is rational (and less costly) for investors to obtain important economic news with only limited frequency or limited accuracy. This might be one possible explanation for why we do not observe a contemporaneous relationship between novel Euro-specific news and EURUSD returns, as it takes time for investors to process new information because it is costly. However, this effect is short-lived as we can already identify a statistically significant and positive relationship between news and EURUSD returns one hour after the news have been published. Since earlier studies on rational inattention theory have looked at more historic data, our findings might lend support to a potential regime shift in how fast investors process novel news. As mentioned in the introduction, the growing market share and importance of high-frequency traders might have caused this development.
Persistent predictor
As previously shown with the surface plots, we observe higher regression coefficients for larger lookback periods. This effect has been well documented in the literature, and is due to performing a regression with an autocorrelated predictor. Cochrane (Citation2000) discusses this effect in detail: when there is predictability of independent variables and we have an autocorrelated predictor, there will by definition be higher regression coefficients for larger intervals. For example, let the EURUSD price be unchanged between 5:00 and 10:00o’clock. If we now extend the time horizon from 5:00 to 15:00 o’clock, the 10-hour return will have a coefficient that is (on average) at least as high as the 5-hour return, since half of its time interval is shared with the 5-hour return. If we have a predictor that is both autocorrelated and also predicts the future return, we will ultimately get higher predicting power at longer intervals.
Let represent the EURUSD log returns from time t to t + 1, xt the differenced news sentiment at time t, and
a stochastic error term. The following equation shows how to model future returns by taking into account the current sentiment:
(4)
(4)
shows that news sentiment is auto correlated. We therefore model it with the following equation:
(5)
(5)
where ρ represents the autocorrelation factor in the news sentiment series. If we try to forecast returns for longer time horizons, we can rewrite the equation as the sum of future log returns:
(6)
(6)
Figure 12. Autocorrelation and partial autocorrelation plot of hourly (differenced) sentiment time series related to Euro-currency specific news from 1 Jan 2003 to 31 Dec 2018.

For a positive ρ the coefficient next to xt will increase with longer horizons. This phenomenon might explain the results by Heston and Sinha (Citation2017), as they find weekly sentiment can predict quarterly returns, whereas daily sentiment only predicts daily returns. Since news sentiment is autocorrelated, the prediction is bound to be stronger and more persistent for longer time horizons.
Backtesting exercise
In order to test the empirical results and the predictive power of news sentiment in a high-frequency setting further, we perform backtests with a simple trading strategy inspired by the results from the predictive regressions. At the end of each hourly interval when we have assessed the Euro-specific news and their sentiment, we either go long (short) the EURUSD based on positive (negative) sentiment from the previous hour. Since we consider Euro-specific news sentiment, we formulate the trading strategy for a Euro-based investor. The benchmark is therefore a simple buy and hold strategy, which is standard in the FX high-frequency literature, as Bekiros (Citation2015) shows. The risk-free rate for the benchmark strategy is the ECB deposit facility rate.
The backtest is calculated from 1 January 2003 to 31 December 2018, and the strategy achieves an annualized return of 12.7% with an annualized volatility of 9.5%.Footnote17 Compared to the benchmark, the trading strategy returns an Information Ratio of 1.16. shows the results of the backtests graphically over time. If we first consider the strategy results without transaction costs, we can see that the strategy did not generate alpha from the beginning of the considered time period until the GFC. However, after the GFC, the strategy performed very well. If we recall the findings from the rolling regressions shown in , this is again an indication of a paradigm shift during the onset of the European debt crisis in 2010 when investors started to consider Euro-specific news and their sentiment in relation to the EURUSD with a positive relationship.
Figure 13. Asset evolution of a simple trading strategy that goes 100% long EURUSD when the hourly sentiment is positive and 100% long USDEUR when the sentiment is negative, rebalancing every hour. Test sample ranges from 1 Jan 2003 to 31 Dec 2018.

If we consider transaction costs, the profitability of the trading strategy decreases significantly due to its high turnover. Since we operate in a high-frequency setting, the trading strategy switches positions approximately 3 times per day on average. If we set transaction costs for a one-way trade to 0.5 bps, the trading strategy returns an overall negative performance for the time period considered, as it detracts by 16.6%. If we set the one-way transaction costs to 1 bps, the trading strategy returns −89.8% over the entire time period.Footnote18
There are various possible ways how to turn the trading strategy profitable even after transaction costs. For example, one could apply smoothing techniques on the news sentiment values in order to rebalance less often. This would be in line with the findings made with the surface plots and the extended time horizons for the regressions.
Conclusion
This is the first study assessing an intraday relationship between Euro-specific news sentiment and foreign exchange rates systematically over an extensive time horizon of 16 years with over 100 million news articles in millisecond precision. We only evaluate novel news about Euro-specific topics and their sentiment, making the study exclusively dedicated to the EURUSD foreign exchange rate. We assess the data in a high-frequency manner, which makes the analysis more complex than the low-frequency studies that currently exist in the behavioral literature on news sentiment and FX.
In line with existing studies from the behavioral finance literature that identify longer-term cycles in news sentiment and their predictive power not only for equities, but also for FX, we make similar findings in the high-frequency space. We show a predictive relationship between novel news about the Euro and EURUSD returns 1-hour and more ahead into the future. However, we also show that there is no contemporaneous relationship in the same hour between novel news and EURUSD returns. This can be partly explained by slow information diffusion and potential rational inattention to new news. The predictive effect of news sentiment becomes stronger over longer time horizons, which can be related to the persistent predictor phenomenon.
We further identify a regime change in the relationship between novel news and the EURUSD after the Global Financial Crisis. From 2009/2010 onwards, we identify a strong positive and statistically significant relationship between Euro-specific news and EURUSD returns. This effect was particularly prominent during the Eurozone debt crisis from 2010 to 2012.
As additional robustness check, we construct an intraday trading strategy, which is able to exploit a signal based on news sentiment quite well with an information ratio above 1. However, once accounted for transaction costs, the strategy does not generate significant alpha anymore due to its high turnover. Future research could look into smoothing techniques of the high-frequency news sentiment signal in order to bring down transaction costs, and in order to make it a viable candidate for potential implementation in practice.
Conflict of interest
The authors declare that they have no conflict of interest.
Notes
1 See https://en.wikipedia.org/wiki/High−frequency_trading, last accessed 15 August 2020.
2 See https://www.euromoney.com/article/b1mqgmxfq42cfx/fx−survey−2020−jpmorgan−dominates−with−more−than−10−market−share, last accessed 15 August 2020.
3 See, for instance, Galati and Ho (Citation2003), Ehrmann and Fratzscher (Citation2005), Jansen and De Haan (Citation2005), Andersen et al. (Citation2007), and Evans and Lyons (Citation2008).
4 See, for instance, Uhl (Citation2014), Uhl (Citation2017), Heston and Sinha (Citation2013), Smales (Citation2014), and Glasserman and Mamaysky (Citation2019).
5 There have been some studies on high-frequency news effects on FX volatility, such as Conrad and Lamla (Citation2010), Kalev et al. (Citation2004), Evans and Lyons (Citation2008), but not on the directional response of intraday FX prices to novel news and their sentiment.
6 See for example Uhl (Citation2017), Uhl, Pedersen, and Malitius (Citation2015), and Heston and Sinha (Citation2013).
7 The bag-of-words sentiment generation is done by counting the words in a news article that belong to a positive or negative category (e.g. ’bad’, ’down’, ’low’, ’negative’ would be classified as negative). Based on this count, the percentage share of the document (either negative or positive) is determined by assigning an overall sentiment score to each specific article.
8 Thomson Reuters News Analytics (TRNA) is a MRN-specific sentiment generated from a proprietary neural network. Various studies have utilized this dataset, such as Heston and Sinha (Citation2013), Uhl (Citation2014), and Smales (2014).
9 Their benchmark is the Harvard IV dictionary. For example, they show in their analysis that words, such as tax, cost, capital, board, liability, foreign and vice are identified as negative by the Harvard Dictionary. However, in a financial context these words do not carry a negative connotation and are in most cases a common part of a 10 K filing.
10 The predecessor of Thomson Reuters News Analytics (TRNA).
11 The search was performed on google.com and reuters.com on 20 June 2020.
12 For a more intuitive understanding of the data, we provide in the appendix with headlines and sentiment of each individual news item released on 27 April 2010, the day when S&P downgraded Greek debt, and as illustrated previously in .
13 We take the timestamp of each individual news article into account when it was published. In the TRNA dataset, this information is provided with millisecond precision.
14 See Papadimas and Graham (Citation2010), last accessed 25 August 2020.
15 See ECB, https://www.ecb.europa.eu/press/key/date/2012/html/sp120726.en.html, last accessed 5 October 2020.
16 Note that we do not display the constant and the regression coefficient of the lagged EURUSD log returns.
17 The annualized volatility is calculated using hourly returns. As a robustness check, we also calculate it using monthly returns, resulting in a comparable volatility of 11.9%
18 Note that we have ignored the interest rate differential for the performance calculation in all backtests because the trading strategies are active in the high-frequency space and therefore the constantly changing yearly EURUSD interest rate differentials between 0% and 2% would not make a significant difference.
References
- Andersen, T. G., T. Bollerslev, F. X. Diebold, and C. Vega. 2007. “Real-Time Price Discovery in Global Stock, Bond and Foreign Exchange Markets.” Journal of International Economics 73 (2):251–77. doi:10.1016/j.jinteco.2007.02.004
- Bekiros, S. D. 2015. “Heuristic Learning in Intraday Trading under Uncertainty.” Journal of Empirical Finance 30:34–49. doi:10.1016/j.jempfin.2014.11.002
- Boguth, O., M. Carlson, A. Fisher, and M. Simutin. 2016. “Horizon Effects in Average Returns: The Role of Slow Information Diffusion.” Review of Financial Studies 29 (8):2241–81. doi:10.1093/rfs/hhw024
- Candelon, B., M. A. N. R. Sy, and M. R. Arezki. 2011. Sovereign Rating News and Financial Markets Spillovers: Evidence from the European Debt Crisis. Number 11-68. International Monetary Fund. doi:10.5089/9781455227112.001
- Cochrane, J. H. 2000. “Asset Pricing.” http://www-gsb.uchicago.edu/fac/john.cochrane/research/papers.
- Conrad, C., and M. J. Lamla. 2010. “The High-Frequency Response of the EUR-USD Exchange Rate to ECB Communication.” Journal of Money, Credit and Banking 42 (7):1391–417. doi:10.1111/j.1538-4616.2010.00346.x
- Doerr, K. 2016. “Mapping the Field of Algorithmic Journalism.” Digital Journalism 4 (6):700–22. doi:10.1080/21670811.2015.1096748.
- Ehrmann, M., and M. Fratzscher. 2005. “Exchange Rates and Fundamentals: New Evidence from Real-Time Data.” Journal of International Money and Finance 24 (2):317–41. doi:10.1016/j.jimonfin.2004.12.010
- Evans, K. P. 2011. “Intraday Jumps and US Macroeconomic News Announcements.” Journal of Banking & Finance 35 (10):2511–27. doi:10.1016/j.jbankfin.2011.02.018
- Evans, M. D., and R. K. Lyons. 2008. “How is Macro News Transmitted to Exchange Rates?” Journal of Financial Economics 88 (1):26–50. doi:10.1016/j.jfineco.2007.06.001
- Fama, E. F. 1970. “Efficient Capital Markets: A Review of Theory and Empirical Work.” The Journal of Finance 25 (2):383. doi:10.2307/2325486
- Ferson, W. E., S. Sarkissian, and T. Simin. 1998. “Is Stock Return Predictability Spurious?” Technical Report 3. www.joim.com.
- Feuerriegel, Stefan, Georg Wolff, and Dirk Neumann. 2016. “News Sentiment and Overshooting of Exchange Rates.” Applied Economics 48 (44):4238–50. 1 doi:10.1080/00036846.2016.1153796
- Galati, G., and C. Ho. 2003. “Macroeconomic News and the Euro/Dollar Exchange Rate.” Economic Notes 32 (3):371–98. doi:10.1111/1468-0300.00118
- Glasserman, P., and H. Mamaysky. 2019. “Does Unusual News Forecast Market Stress?” Journal of Financial and Quantitative Analysis 54 (5):1937–74. 10 doi:10.1017/S0022109019000127
- Groß-Klußmann, A., and N. Hautsch. 2011. “When Machines Read the News: Using Automated Text Analytics to Quantify High Frequency News-Implied Market Reactions.” Journal of Empirical Finance 18 (2):321–40. doi:10.1016/j.jempfin.2010.11.009
- Groß-Klußmann, A., and N. Hautsch. 2009. “Quantifying High-Frequency Market Reactions to Real-Time News Sentiment Announcements.” http://sfb649.wiwi.hu-berlin.de.
- Heston, S. L., and N. R. Sinha. 2013. “News versus Sentiment: Comparing Textual Processing Approaches for Predicting Stock Returns.” SSRN Electronic Journal 8:1–38. https://papers.ssrn.com/abstract=2311310. doi:10.2139/ssrn.2311310
- Heston, S. L., and N. Sinha. 2017. “News vs. Sentiment: Predicting Stock Returns from News Stories.” Financial Analysts Journal 73 (3):67–83. doi:10.2469/faj.v73.n3.3
- Huang, L., and H. Liu. 2007. “Rational Inattention and Portfolio Selection.” The Journal of Finance 62 (4):1999–2040.
- Jansen, D. J., and J. De Haan. 2005. “Talking Heads: The Effects of ECB Statements on the Euro-Dollar Exchange Rate.” Journal of International Money and Finance 24 (2):343–61. doi:10.1016/j.jimonfin.2004.12.009
- Kalev, P. S., W. M. Liu, P. K. Pham, and E. Jarnecic. 2004. “Public Information Arrival and Volatility of Intraday Stock Returns.” Journal of Banking & Finance 28 (6):1441–67. doi:10.1016/S0378-4266(03)00126-2
- Kirilenko, A., A. S. Kyle, M. Samadi, and T. Tuzun. 2017. “The Flash Crash: High-Frequency Trading in an Electronic Market.” Journal of Finance 72 (3):967–98. doi:10.1111/jofi.12498
- Loughran, T., and B. Mcdonald. 2011. “When is a Liability Not a Liability? Textual Analysis, Dictionaries, and 10-Ks.” The Journal of Finance 66 (1):35–65. doi:10.1111/j.1540-6261.2010.01625.x
- Mamaysky, H. 2019. “Financial Markets and News about the Coronavirus.” SSRN Working Paper:1–60. doi:10.2139/ssrn.3565597.
- Papadimas, L., and D. Graham. 2010. “S&P Cuts Greek Debt to Junk, Downgrades Portugal.” http://www.reuters.com/article/2010/04/27/us-greece-idUSLDE63P0LU20100427.
- Salmon, M. 2014. High Frequency Trading and Limit Order Book Dynamics. Milton Park: Routledge.
- Smales, L. A. 2014. “News Sentiment in the Gold Futures Market.” Journal of Banking & Finance 49:275–86. doi:10.1016/j.jbankfin.2014.09.006
- Tandoc Jr, Edson C., Lim Jia Yao, and Shangyuan Wu. 2020. “Man vs. Machine? The Impact of Algorithm Authorship on News Credibility.” Digital Journalism 8 (4):548–62. doi:10.1080/21670811.2020.1762102
- Tetlock, P. C. 2007. “Giving Content to Investor Sentiment: The Role of Media in the Stock Market.” The Journal of Finance 62 (3):1139–68. doi:10.1111/j.1540-6261.2007.01232.x
- Tetlock, P. C., M. Saar-Tsechansky, and S. Macskassy. 2008. “More than Words: Quantifying Language to Measure Firms’ Fundamentals.” The Journal of Finance 63 (3):1437–67. doi:10.1111/j.1540-6261.2008.01362.x
- Uhl, M. W. 2014. “Reuters Sentiment and Stock Returns.” Journal of Behavioral Finance 15 (4):287–98. doi:10.1080/15427560.2014.967852
- Uhl, M. W. 2017. “Emotions Matter: Sentiment and Momentum in Foreign Exchange.” Journal of Behavioral Finance 18 (3):249–57. 1332061. doi:10.1080/15427560.2017
- Uhl, M. W., M. Pedersen, and O. Malitius. 2015. “What’s in the News? Using News Sentiment Momentum for Tactical Asset Allocation.” The Journal of Portfolio Management 41 (2):100–12. https://jpm.pm-research.com/content/41/2/100. https://jpm.pm-research.com/content/41/2/100.abstract. doi:10.3905/jpm.2015.41.2.100
Appendix A
Table A1. Dataset example of headlines and the sentiment of the full news texts on 27 April 2010. A sentimentClass of -1 (1) indicates negative (positive) sentiment, 0 neutral or non-definable sentiment.