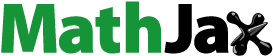
ABSTRACT
Stormwater management systems rely on an acyclic network structure, making the network more vulnerable to failure. Existing stormwater resilience studies have predominantly focused on hydraulic and hydrological characteristics of the system with no robust frameworks to evaluate the resilience of the stormwater piped network in terms of network connectivity. This paper introduces a new index-based quantitative framework to characterise the resilience of acyclic stormwater network structure using graph theory and resilience theories. This includes an index developed to evaluate the overall independency of a pipe within an acyclic piped network. The framework was applied to various simple network structures, and an existing stormwater network demonstrating the influence of network structure, pipeline connectivity and number of outlets on the network resilience. This framework can be used to test different network layouts and structures in urban design and pipeline renewal projects to maximise the resilience of the structure of stormwater networks.
1. Introduction
Urban network infrastructure comprises of components for transferring electricity, water, telecommunication services, industrial products, and passengers. This transfer can be referred to as load between two points or nodes (Lewis Citation2014). In these types of networks, load is transferred from an origin to a destination, or is transferred from one point to another point in the minimum possible time to improve service quality. Most infrastructure networks are cyclic systems with alternative paths to transfer the load from one point to another.
Network-based infrastructure can be more reliable when there are alternative pathways between nodes, providing redundancy when a portion of the network fails (Garbin and Shortle Citation2007). Urban network infrastructure has been the core of a number of past studies, with a focus on transportation network structure (Masucci, Stanilov, and Batty Citation2014; Porta, Crucitti, and Latora Citation2006), water supply systems (Nardo Di and Di Natale Citation2011; Nardo Di et al. Citation2013; Ostfeld Citation2005; Porse and Lund Citation2015; Yazdani and Jeffrey Citation2011, Citation2012), telecommunication networks (Aggarwal Citation1985; Alves et al. Citation2017; Lahby and Sekkaki Citation2018), electric power network structure (Bajpai, Chanda, and Srivastava Citation2016; Haynes et al. Citation2002; Holmgren Citation2006) and network interdependencies (Candelieri, Soldi, and Archetti Citation2015; Ip and Wang Citation2011; Lam and Tai Citation2012; Pant et al. Citation2018; Zorn et al. Citation2020).
The network structures associated with water supply are mainly a cyclic system, comprising a series of loops that provide alternative pathways for supply within the network. The characteristics of water related urban network infrastructure have been analysed in relation to network structure (Chartrand and Zhang Citation2012); cost effectiveness (Deuerlein Citation2008; Nardo Di and Di Natale Citation2011; Nardo Di et al. Citation2013; Ostfeld Citation2005); resilience of the network in terms of connectivity (Ostfeld Citation2005; Porse and Lund Citation2015) and the resilience of the system in terms of redundancy and robustness (Yazdani and Jeffrey Citation2011, Citation2012). Studies related to the structure of wastewater networks have mainly focused on topological characteristics (Bakhshipour et al. Citation2018; Ghosh, Hellweger, and Fritch Citation2006; Möderl, Butler, and Rauch Citation2009; Zischg et al. Citation2017), optimisation of storage capacities (Vazquez et al. Citation1997), optimisation of construction of the gravity piped network to minimise depth (Rodrigues et al. Citation2019), critical elements in the network (Meijer et al. Citation2018), reliability in terms of failure probability (Ermolin Citation2001) and centralising drainage systems (Alves et al. Citation2017; Reyes-Silva et al. Citation2020).
The main objective of a stormwater management system is to control excess runoff using various parts of the hydrologic cycle (Wanielista and Yousef Citation1993). However, the majority of stormwater management systems are still dependent on pipes and streams to collect and convey stormwater runoff from sub-catchments to outlets such as rivers, streams, lakes, and seas. Drainage pipes are mainly connected by manholes and joints that collect stormwater runoff generated in the catchments within the stormwater management system and then transfer it to outlets.
The urban stormwater drainage network is the most significant and vulnerable part of the wider stormwater infrastructure system, acting as an urban flood control system for frequent rainfall events. This drainage network is typically a gravity-based system, a key difference compared to other network infrastructures as this means the networks are predominately acyclic. These networks are also generally dependent on road layout and topographic constraints when they transfer load from sources to an outlet, as they are constructed under the road system to minimise the potential disturbance to surrounding properties and structures. The outlet of a stormwater network can either be a waterbody as the final discharge point or be a temporary storage area to reduce the impact of urban flooding. This type of network structure typically provides a minimum level of connectivity, without any alternative pathways between source nodes and outlet nodes, making the system particularly vulnerable to component failure.
In a drainage network system, the travel time of the load from the source to the final outlet is not as important as reducing the impact of any flooding on urban areas. Therefore, a temporary storage area to retain the runoff volume and reduce the flood risk downstream is an alternative approach that can be used. Currently, many stormwater management strategies have been developed using Best Management Practice (BMPs), Low Impact Development (LID), and Water Sensitive Design (WSD) for improving stormwater quantity and quality control, typically comprising structural and non-structural measures (Ahiablame, Engel, and Chaubey Citation2012; Birgani, Yaser, and Moghadam Citation2013; Chang and Liou Citation2010; Jia, Yuwen Lu, and Chen Citation2012). These approaches are able to improve the adaptability and robustness of the network in rainfall events by reducing the impact of a lack of redundancy in the network, thereby improving the network structure resilience. However, contemporary sustainable stormwater management strategies do not change the inherent acyclic nature of drainage-based stormwater management systems (Brown, Keath, and Wong Citation2009).
Studies related to stormwater network structure are very limited and typically refer to sewer drainage systems. Research related to stormwater network structure has been associated with reliability of the stormwater collection system (Kwietniewski and Lesniewski Citation2006) and optimisation of urban drainage systems (Bakhshipour et al. Citation2018, Citation2019; Hesarkazzazi et al. Citation2022; Rodrigues et al. Citation2019). Kwietniewski and Lesniewski (Citation2006) developed a model for reliability assessment of a Y-types sewerage network and assessed the effect of system failure using a probabistic model. The approach relies on unfit pipeline influence in network by considering a level of service. Bakhshipour et al. (Citation2018) introduced an algorithm based on graph theory to optimise the decentralisation of urban drainage layout based on collection systems and treatment devices. Studies focusing on the resilience of Urban Drainage Systems (UDSs) are mainly related to flooding and the hydraulic characteristics of the system (Guptha et al. Citation2021; Mugume et al. Citation2015). Mugume et al. (Citation2015) developed an approach to evaluate urban drainage system resilience using Global Resilience Analysis (GRA), system performance and resilience theory approach by considering the influence of link failure scenarios and flood volumes. Hesarkazzazi et al. (Citation2022) developed a graph theory approach to introduce redundancy in Urban Stormwater Networks (USNs) by considering the effective flow redistribution throughout the network and assessing the effect of redundancy on resilience for a decentralised network. The study considered the undirected graphs and cyclic networks with existing loops within the network. Wang et al. (Citation2023a) presents an assessment framework to optimise the hydrological performance of a coupled drainage system of grey and green infrastructure based on graph theory and genetic algorithms (GA), demonstrating the higher performance of coupled grey-green infrastructure and decentralised grey-green infrastructure. Wang et al. (Citation2023) identified that a decentralised drainage system exhibits economic advantages. In low intensity urban areas, decentralised drainage systems likely have a wider range of options, while high-density areas typically rely on a centralised system associated with high cost.
Previous studies related to stormwater network structure analysis considered the hydrological characteristics, hydraulic characteristics and the effect of discretisation of the network. Some of these studies also focused on sewer system whose characteristics differ from those of a separated stormwater network in terms of the transferring of the load within the system. In addition, the characteristics of acyclic networks was not highlighted in the previous studies. The evaluation of the resilience of acyclic piped network structures in terms of the connectivity of the network is a research gap.
The aim of this study is to develop a new quantitative framework to characterise the resilience of the structure of an acyclic piped stormwater network based on network connectivity, system adaptability and redundancy. This approach is able to increase the resilience of acyclic stormwater network structures, resulting in the improvement of overall stormwater management systems resilience. Resilience is defined in this study as the ability of the network to reduce the impacts of a shock event based on three main properties of resilience, namely the robustness, adaptability and redundancy (Vugrin et al. Citation2010). In addition, a new index is defined to evaluate the connectivity of the drainage network as an acyclic system. The details of the framework are first presented. A set of simple network structures are presented to demonstrate the application of the framework and understand how the outputs vary in relation to network connectivity. The framework is then applied to a real-world case study using two possible modifications of the network structure to evaluate how they change the resilience and connectivity of the network. Results from this case study application are discussed and key conclusions drawn.
2. Methodology
This study focuses on the characteristics of acyclic stormwater piped network and connectivity of sources with the outlet via node connectivity. Analysis of stormwater network structure can be used to determine the resilience of the system and the parameters that may affect the adaptability and robustness of the network (Lam and Tai Citation2012). Key factors to consider are: (1) the characteristics of acyclic networks; (2) the connectivity of the sources with the outlets instead of node-to-node connectivity; and (3) the effect of network properties on minimising stormwater accumulation impacts.
The structure of a stormwater network is associated with catchment topography, urban layout, street networks and planning strategies. The urban layout is related to a wide range of fields of studies such as urban design, architecture, archaeology, geography, history, environmental psychology (Rashid Citation2017a, Citation2017b). Rashid (Citation2017a) developed a set of indicators to indicate the characteristics of a city network, such as integrity, intelligibility, and connectivity of the city, and represented this using an axial map. An axial map demonstrates the relationship between city layout and network structures. The dependency of urban layouts and network structures shows the potential influence of urban design and specifically urban street patterns on the resilience of stormwater network structure. With the layout and structure fixed in this way, the resilience of the network structure can be influenced by applying WSD and LID approaches as part of urban design.
In this study, graph theory was used to represent the relationship between the pipes and the stormwater network structure. A network structure can be represented by a graph network that is based on a set of nodes (
) and
edges (
) (Godsil and Royle Citation2013). Each edge (pipe) connects a pair of nodes (manholes and/or pipe connections) to construct a graph
. In most network infrastructure, the edges of graphs are undirected, meaning that a load can transfer between two nodes without consideration of the direction of the edges. In a stormwater network the direction of water flow is from upstream, where runoff is collected in the catchment, to the outlets at the downstream end of the network. As such, an edge between nodes
and
is directed, representing a directed graph known as a digraph network
(Chartrand and Zhang Citation2012). Edges and nodes of the graph representing a stormwater network can be potentially weighted to indicate their physical and operational properties, which is important when the adaptability of the system is taken into consideration and the properties of the components are an important input. In general, in a stormwater network the nodes are classified into source nodes and outlets. The source nodes collect runoff from their contributing catchment and then pass the accumulated runoff through edges towards the outlet nodes. The outlet nodes discharge the runoff from the total catchment of the network to a water body or temporary storage.
A simplified representation of a stormwater network structure is presented in . Stormwater manholes were defined as source nodes and the pipes connecting them are represented by edges. The most upstream sections of the network are comprised of small-sized pipes forming a sub-tree (representing part of the overall network), usually collecting stormwater runoff from private pipes, branched pipe connections, catch-pits and stormwater control devices. To simplify the network structure for this methodology, upstream sub-trees with small-diameter pipes were not directly represented within the network structure.
Figure 1. Simplified stormwater network graph with the upstream sub-tree for source nodes 1 and 4 represented. All source nodes have their own sub-tree, however those for source nodes 2 and 3 are not shown here.

An adjacency matrix provides fundamental graph information in terms of node connections, edge direction, the number of edges exiting a node (node out-degree), the number of edges entering a node (node in-degree), and all can be used in network structure analysis (Chartrand and Zhang Citation2012). An adjacency matrix of a graph of nodes is an
matrix
, where
is equal to 1 when there is a directed edge (pipe) from node
to node
, and 0 when there is no directed edge from node
to node
. In graph theory, the average node in-degree (
) is calculated by dividing the total node in-degree of the network by the total number of nodes. To represent the influence of the excluded sub-trees in the upstream parts of the network, the
was modified in this study by adding an in-degree value of 1.0 at the most upstream nodes in the network. Because of this, when the network structure changes, the value of
will not be constant. The maximum node in-degree (
) was defined as the maximum number of in-degree connections to a node within the network. As source nodes typically have one outlet pipe in a stormwater network structure, the out-degree value of the nodes will always be equal to 1. Therefore, the out-degree value was not used in this approach, with the node in-degree value (
) used to represent the sparseness of the network configuration.
Two indices were developed in this study to analyse stormwater network connectivity characteristics using graph theory. The first index used the concept of pipe failure probability to quantify the resilience of a stormwater network associated with the functionality and adaptability of the network. The pipe properties and effective contributing catchment area of each pipe were utilised in this approach. The second index represents the independency of the upstream catchments relative to the downstream piped network. The methodology used to develop these indices is summarised in the following sections.
2.1. resilience of stormwater network structure 

An approach was defined to evaluate the resilience of a stormwater network structure using graph theory and the reduction paradigm approach (Mata‐Montero Citation1991). In this approach the probability of the disconnection of a portion of the network from an outlet was considered. To evaluate the resilience of the stormwater piped network structure (Network Structure Resilience ) for urban catchments, the probability that a pipe was unable to convey flow and the proportion of the catchment that would be potentially affected by the failed pipe were calculated for each pipe. These values were used to evaluate the probability of disconnecting a portion of the catchment associated with part of the network (sub-tree) to the outlet. demonstrates the framework used to evaluate
. Each part of this framework, and how each part relates to one another, is summarised in the following sections.
2.2. Pipe failure probability
There are different types of drainage used in stormwater networks to convey flow, which are mainly classified as closed drains and open drains. The probability of failure of open drains, such as natural waterbodies and urban channels, to convey runoff within the catchment is low during rainfall events, which makes them a more resilient component of the network compared to closed drains. Even if a blockage or failure occurs in open drains, the system can still typically maintain the majority of its capacity to convey flow. Therefore, this paper only analyses the stormwater piped network structure as the main component of stormwater management system without including open drains. However, this approach could be modified to include open drains where these are present in the stormwater network.
The pipe failure probability () of a closed drain between two nodes
depends on external factors and pipe physical properties (Tran, Ng, and Perera Citation2007). The external factors are mainly associated with loads on the pipe or blockages within the pipe leading to disruption of functionality. The physical properties of the pipe such as section shape, length, roughness, material, and age can all influence the potential failure probability of the pipe (Najafi and Kulandaivel Citation2005).
2.3. Weight of nodes
In general, the runoff produced in each rainfall event is associated with the characteristics of the landcover in the catchment, which is represented by a runoff coefficient. The effective area for each catchment is equal to the catchment area multiplied by the runoff coefficient based on the rational method (Chow Citation1964). This parameter provides the coefficient of peak discharge of a catchment with a specific landcover.
Using a similar approach, the weight of each source node () was defined using EquationEquation 1
(1)
(1) .
where is the effective area of the catchment for source node
and
is the total effective catchment area for the network.
represents the proportion of the total effective catchment area that is collected by each source node
.
2.4. Sub-tree failure probability
Failure of a pipe (edge) in the stormwater network graph disconnects the upstream parts of the network from the outlet downstream, causing a disturbance in the flow from an upstream sub-tree to the outlet. This accumulates all the weight of the upstream source nodes to the failed pipe in each sub-tree. The probability of failure of an upstream sub-tree of the network () due to pipe failure was calculated for each pipe
using EquationEquation 2
(2)
(2) .
where is the upstream network that contains all source nodes associated with edges that either directly or indirectly lead to pipe
. As source nodes in the upstream parts of the network have smaller cumulative
values, the value of
for these locations is smaller than those for pipes in the downstream parts of the network.
2.5. Network failure factor
The Network Failure Factor () is the sum of all possible failure probabilities of a network based on the ‘OR’ probability function, representing the probability of network disturbance if a pipe
fails (EquationEquation 3
(3)
(3) ). The value of this factor is associated with the network structure, pipe failure probability and the number of available outlets within the system.
where is the total number of the pipes in the network.
2.6. Network structure resilience
To determine the resilience of a network, the network failure factor was normalised based on the maximum and minimum network failure factors. The minimum network failure factor () is defined by modifying the network of interest such that all source nodes are connected independently to an outlet or waterbody without any load being conveyed through other source nodes or pipes. In this case the flooding that develops when there is failure of an edge would be minimised for the network structure. A network structure with a maximum failure factor (
) is defined by modifying the network of interest into a series network where all the source nodes and pipes in the network are connected in series. In this modified network, the largest catchment is located furthest upstream within the network and the smallest catchment is nearest to the outlet. For this network structure the load accumulates moving from the upstream network towards the outlet without any redundant pathways, meaning the flooding that develops would be maximised.
The factors and
provide an index of minimum and maximum network failure factor based on the source node weights and pipe failure probabilities of the network. By considering
,
and the network failure factor (
), the Network Structure Resilience (
) is calculated using:
By increasing the number of pipelines in the network, the distribution of the network failure factor skews towards the , which reduces the differences in the
values between scenarios. As a result, the inverse of the failure factor has been considered in
to provide a clearer distinction between the different scenarios.
2.7. independency factor 

In an acyclic network, conveyance of upstream flow is directly dependent on the pipes downstream of the catchment. However, the drainage network pattern can vary depending on the urban pattern, catchment topography and the location of natural waterbodies. This can change the dependency of the upstream source nodes on different numbers of pipes to convey flow downstream.
To represent the overall independency of the source nodes within a network, an independency index () was defined:
where is the number of source nodes for a network and
is the number of pipes that lead directly from a source node
to an outlet. The maximum value of
is 1.0, representing the ideal network structure where each source node discharges independently to a waterbody without being influenced by any other pipes.
As stormwater piped networks are acyclic there are generally no alternative pathways to transfer the runoff from sources to outlets. Splitting up the piped network into smaller sections, each with their own outlet, reduces the dependency of upstream pipes on the downstream network. This increases the index toward 1.0, representing a more independent acyclic network.
Increasing the connectivity of the network structure potentially reduces the dependency on a single pipe, which can reduce the impact of pipe failure in the network. Based on the resilience definition for urban infrastructure, it increases the absorption capacity (robustness) of the system, leading to an increase in system resilience (Vugrin et al. Citation2010). The adaptive capacity refers to the system ability to change in order to recover the system performance (Vugrin et al. Citation2010). The addition of temporary storage volume capacity can also reduce the load on pipes in failure events. Based on objective of stormwater piped networks, it fulfils the definition of adaptive capacity as part of the resilience concept.
3. Application to simple network structures
presents eight different possible stormwater network graphs with an identical number of source nodes () and pipe edges (
). Each network represents different connectivity conditions between the nodes to convey the load to the outlet. The weights of the nodes are assumed identical for these eight networks. These are used to demonstrate the application of the framework described in the previous section.
Figure 3. Different schematic stormwater acyclic networks: (D1) series network; (D2, D3, D4, D5, D6 and D7) partially series network; (D8) decentralised network.

For simplicity the failure probability of all pipes was assumed to be equal to 0.01. Therefore, any changes in network resilience are directly dependent on the connectivity of the network and the number of individual outlets.
summarises the network properties and the indices for each of the network graphs. Here the number and properties of the source nodes and pipes of all eight graphs are identical, with the only difference being the structure of the graphs. The maximum node in-degree of the networks ranges from 1 to 4 depending on the structure of the networks. The average node in-degree varies from 1 in the series network (D1) to 1.6 in D8, providing a basic measure of the connectivity of the directed graphs. As the pipes are connected predominately in a series type structure, the value of the average node in-degree in stormwater networks is generally between 1 and 2.
Table 1. Network properties and metrics for each of the schematic networks in .
The independency factor ranges between 0.52 for D1 and 1.00 for D8, with the independency of graphs increasing when there is increased connectivity between source nodes and an increased number of outlet points. Although the maximum node in-degree and average node in-degree factors are the same for D2, D3 and D5, the independency coefficient varies between 0.54 and 0.71. This demonstrates that the connection pattern between the nodes has an influence on the network independency. The network resilience of graph D1 is 0.00 as the network is entirely in series and the stormwater is accumulated in each downstream catchment, increasing the impact of failure. The dependency of the upstream pipes on the downstream piped network structure and the average network in-degree changes when the structure of the graph is changed. This changes the absorption capacity of the network structure and eventually the resilience of the network structure. The source nodes of graph D8 are discharged from the system without being affected by the piped network, with the failure of a pipe not influencing the functionality of the rest of system, making the network more resilient compared to the other network patterns illustrated in . By discretising the network and increasing the number of source nodes connected to an outlet independently, the impact of pipe failure on the functionality of the other parts of the network reduces, which increases the adaptive capacity of the network (Vugrin et al. Citation2010). In a stormwater system, the outlets can be temporary storage to accumulate the runoff volume in flood events or any potential disturbance of downstream stormwater network structure that affects its ability to convey the flow. These temporary storage locations can be either dual purpose dry ponds with urban social functionalities such as sport fields and park (Mah, Salehe, and Josep Putuhena Citation2014; Zorn et al. Citation2020) for less frequent events, or engineered structures and underground devices for frequent events. These temporary outlets are considered as an alternative approach to fulfil the objective of the stormwater network and increase the redundancy of the network structure.
compares the Network Structure Resilience and the average node in-degree, as a primary measure of the connectivity of the network structure, for all possible network structures with source nodes and pipe numbers between two and five. The minimum node in-degree of the graphs across all scenarios is equal to 1, representative of series type networks, and these all have a resilience degree of 0%. By changing the structure of the network, the connectivity between the source nodes changes, leading to a change of the average node in-degree in the network structure. The average node in-degree is shown to vary for network structures with different number of source nodes. The maximum average node in-degree for a network with two source nodes is 1.33, increasing to 1.65 for a network with four source nodes. In addition, the increase in the network connectivity increases the average node in-degree, resulting in an increase in the Network Structure Resilience .
4. Case study network application
The proposed framework was tested using an existing stormwater network system from a case study area and two modified versions of the stormwater network structure. The study area is part of the Otara Creek and Flat Bush urban catchments in the Auckland Region of New Zealand (36°57′S,174°54′E), with total contributing catchment area of 89 ha (). The study area is located in the upstream area of the catchment, which was developed in early 2000 to form a residential area. The study area has a separated stormwater network structure collecting stormwater runoff and conveying it to an open channel in the middle of the catchment. The stormwater runoff eventually discharges into Otara Creek. The diameter of the stormwater pipes within the study area ranges between 225 mm and 1800 mm.
Figure 5. The location of the study area and the existing stormwater piped network in the Otara catchment, Auckland.

Three scenario analyses were taken into account in this study. Scenario 1 was based on the as-built stormwater network within the catchment, comprising four separate stormwater piped network sub-catchments within the total catchment, A total of 644 individual pipes formed the networks. The sub-catchment area of the four networks ranged between 2.1 ha and 42 ha, with an average area of 22.2 ha. A natural creek that crossed the catchment from the northeast to the southwest collected stormwater runoff from the piped network via detention ponds on the basis of Low Impact Design (LID) approach ().
Figure 6. Layout of stormwater network systems and contributing catchment area for Scenario 1. The color shading shows the extent of each of the sub-catchments.

Scenario 2 assumed that all the stormwater pipes within the catchment were a combined network system and discharged downstream via a single outlet node without any alternatives (). This provided a representation of the network structure with the lowest independency value. The existing natural channel within the study area provided an opportunity to further discretise the urban area based on a LID approach, meaning the stormwater networks could be divided into smaller sub-catchments with a larger number of outlets. Therefore, in Scenario 3 the piped network system was discretised into 15 smaller sub-catchments with contributing catchment areas ranging from 1.2 ha to 14.9 ha and an average catchment area of 6.3 ha (). This provided a representation of the network structure with a high independency value.
Figure 8. Layout of stormwater network systems and contributing catchment area for Scenario 3. The color shading shows the extent of each of the sub-catchments.

The characteristics of the stormwater piped network for the three scenarios are summarised in . The Network Structure Resilience of the catchment for Scenario 1 was 4.7%, indicating that a large portion of the pipes within the catchment are connected in series. In Scenario 2, the outlets of the four sub-catchments were connected in series and discharged from the total network to downstream via a single outlet. The Network Structure Resilience of this network reduced slightly as a result of an extension of the overall series structure of the network. As the downstream pipes collected the runoff from the majority of the urban catchment, the upstream piped network was considerably larger, increasing the network failure factor for the piped network, resulting in a reduction of the Network Structure Resilience. The large number of upstream pipes depends on the downstream pipes to convey the runoff to the outlet, which led to a slight reduction in the independency value for Scenario 2 compared with Scenario 1.
Table 2. Network properties and metrics for the case study application scenarios.
Dividing the catchment into 15 smaller sub-catchments with separate outlets into a waterbody increased the Network Structure Resilience to approximately 14% for Scenario 3, a three-fold increase compared to the other scenarios. In this case the robustness capacity of the wider network increases during pipe failure incidents, although this does not provide any alternate paths from source to outlet for any of the sub-catchments. In Scenario 3, the piped network adaptive capacity increases because of the discretisation of the network, with a pipe failure affecting a smaller number of upstream pipes. In this case a pipe failure incident results in a smaller proportion of the catchment being disconnected from an outlet. Discretisation of the stormwater piped network can be implemented by utilising waterbodies such as open channels or natural streams, which are considered resilient edges within the stormwater system with low failure probabilities.
Temporary storage areas can also discretise the network by providing enough volume to collect stormwater runoff and reduce flood hazard in urban areas. There are various design approaches that make use of temporary storage areas, and these can be implemented based on the catchment size. The temporary storage acts as an alternative means to achieve the stormwater network objective and maintain a level of serviceability. In addition, discretising the network reduces the impact of a pipe failure on other pipe conveyance, which is the main objective of the redundancy property of a network within the definition of resilience.
These results highlight the performance of the framework and its ability to quantify the resilience of different drainage network structures in terms of robustness and adaptability of the networks. By extending a series of pipes and source nodes within a network, the overall failure probability of the network increases, resulting in the reduction of the resilience of the network structure. In contrast, the resilience of the network can be increased by increasing the connectivity of the network, using a different piped network layout, adding more outlets into the network or connecting more source nodes to outlets and utilising resilient drainage paths to collect and convey the flow increases the overall resilience of the network structure. By considering the constraints on stormwater piped network location due to urban layout characteristics, geographical conditions of the area and stormwater design requirements, this framework can be used as a tool in urban design for new developments to optimise the resilience of the stormwater piped network structure, which eventually reduces flood hazards due to stormwater piped network failure.
5. Conclusions
This paper presented a framework to quantify the resilience of stormwater piped network structures using graph theory in terms of network connectivity, system adaptability and redundancy of the networks. The resilience in this approach is influenced by the failure probability of the pipes, the pipe network layout, and connectivity of the source nodes to outlets. The approach considers the absorption and adaptive capacity of the stormwater piped network structure to evaluate the resilience of the stormwater piped network structure based on the connectivity of the network structure. In addition, an index was introduced representing the independency of source nodes in acyclic networks to transfer loads from the source node to the outlets. Application of the approach to simple network structures showed that the connection pattern between the nodes influences the network independency factor. By discretising the network and increasing the number of source nodes connected to an outlet independently, the impact of pipe failure on the functionality of the other parts of the network reduces, which increases the adaptive capacity of the network. The approach was tested in an urban catchment within the Auckland Region, New Zealand, for three different network structure scenarios. The results provide a quantifiable representation of the influence of the number of outlets and discretisation of the network on the connectivity of the piped network and the Network Structure Resilience. By dividing the catchment into 15 smaller sub-catchments with individual outlets, the Network Structure Resilience almost tripled. In addition, the discretisation of the network increased the piped network adaptive capacity, with a pipe failure impacting a smaller number of upstream pipes. Further research will focus on developing an approach to evaluate the failure probability of pipes in terms of external load factors and pipe deterioration. Future studies will use the framework to analyse the effect of urban layouts and the use of Low Impact Development (LID) approaches on stormwater piped network resilience. The interaction of piped network structure and the overland flow path network can also be considered in future research. The study is based on the assumptions that the pipe failure probability is constant for all the pipelines to assess the resilience of the stormwater piped network structure. Further study can be implemented to determine the effect of pipe failure probability in the resilience of the network structure. The approach presented in this paper is limited to the network structure dimension, and this is part of a wider study focusing on the quantification of the resilience of stormwater management systems to flooding based on network, hydraulic and hydrology dimensions.
Acknowledgements
We acknowledge Auckand Council for providing the details of the case study catchment stormwater network. This research was funded by the Resilience to Nature’s Challenges National Science Challenge.
Disclosure statement
No potential conflict of interest was reported by the author(s).
Data availability statement
The data that support the findings of this study are openly available in Auckland Council GeoMaps mapping service at https://www.aucklandcouncil.govt.nz/geospatial/geomaps/Pages/default.aspx.
Additional information
Funding
References
- Aggarwal, K. K. 1985. “Integration of Reliability and Capacity in Performance Measure of a Telecommunication Network.” IEEE Transactions on Reliability 34 (2): 184–186. https://doi.org/10.1109/TR.1985.5221990.
- Ahiablame, Laurent M, Bernard A Engel, and Indrajeet Chaubey. 2012. “Effectiveness of Low Impact Development Practices: Literature Review and Suggestions for Future Research.” Water, Air, & Soil Pollution 223 (7): 4253–4273. https://doi.org/10.1007/s11270-012-1189-2.
- Alves, Rogerio J M, Marcos M Rodrigues, Marcia H M Paiva, and Marcelo E V Segatto. 2017. “A Note on Resilience of Telecommunication Networks.” In 2017 CHILEAN Conference on Electrical, Electronics Engineering, Information and Communication Technologies (CHILECON), Pucon, Chile, 1–6. IEEE.
- Bajpai, Prabodh, Sayonsom Chanda, and Anurag K Srivastava. 2016. “A Novel Metric to Quantify and Enable Resilient Distribution System Using Graph Theory and Choquet Integral.” IEEE Transactions on Smart Grid 9 (4): 2918–2929. https://doi.org/10.1109/TSG.2016.2623818.
- Bakhshipour, Amin E, Milad Bakhshizadeh, Ulrich Dittmer, Ali Haghighi, and Wolfgang Nowak. 2019. “Hanging Gardens Algorithm to Generate Decentralized Layouts for the Optimization of Urban Drainage Systems.” Journal of Water Resources Planning and Management 145 (9): 4019034. https://doi.org/10.1061/(ASCE)WR.1943-5452.0001103.
- Bakhshipour, Amin E, Milad Bakhshizadeh, Ulrich Dittmer, Wolfgang Nowak, and Ali Haghighi. 2018. “A Graph-Theory Based Algorithm to Generate Decentralized Urban Drainage Layouts.” In International Conference on Urban Drainage Modelling, 633–637. Springer. https://doi.org/10.1007/978-3-319-99867-1109.
- Birgani, Tahmasebi, Farhad Yazdandoost Yaser, and Mani Moghadam. 2013. “Role of Resilience in Sustainable Urban Stormwater Management.” Journal of Hydraulic Structures 1 (1): 44–53.
- Brown, R. R., Nina Keath, and T. H. F. Wong. 2009. “Urban Water Management in Cities: Historical, Current and Future Regimes.” Water Science & Technology 59 (5): 847–855. https://doi.org/10.2166/wst.2009.029.
- Candelieri, Antonio, Davide Soldi, and Francesco Archetti. 2015. “Network Analysis for Resilience Evaluation in Water Distribution Networks.” Environmental Engineering and Management Journal 14 (6): 1261–1270. https://doi.org/10.30638/eemj.2015.136.
- Chang, Chia-Ling, and Te-Yueh Liou. 2010. “The Placement Strategies of Structural Best Management Practices for Different Moving Rainstorms.” Environmental Monitoring and Assessment 166 (1–4): 495–502. https://doi.org/10.1007/s10661-009-1018-2.
- Chartrand, Gary, and Ping Zhang. 2012. A First Course in Graph Theory. Mineola, New York: Dover Publications, INC.
- Chow, VenTe. 1964. Handbook of Applied Hydrology: A Compendium of Water-Resources Technology. New York (USA): McGraw-Hill.
- Deuerlein, Jochen W. 2008. “Decomposition Model of a General Water Supply Network Graph.” Journal of Hydraulic Engineering 134 (6): 822–832. https://doi.org/10.1061/(ASCE)0733-9429(2008)134:6(822).
- Ermolin, Yuri A. 2001. “Estimation of Raw Sewage Discharge Resulting from Sewer Network Failures.” Urban Water 3 (4): 271–276. https://doi.org/10.1016/S1462-0758(01)00034-6.
- Garbin, D. A., and J. F. Shortle. 2007. “Measuring Resilience in Network-Based Infrastructures.” Critical Thinking: Moving from Infrastructure Protection to Infrastructure Resilience 73–85.
- Ghosh, Indrani, Ferdi L Hellweger, and Todd G Fritch. 2006. “Fractal Generation of Artificial Sewer Networks for Hydrologic Simulations.” In Proceedings of the ESRI International User Conference, San Diego, California, August, 7–11.
- Godsil, Chris, and Gordon F Royle. 2013. Algebraic Graph Theory. Vol. 207. New York: Springer Science & Business Media.
- Guptha, Guru Chythanya, Sabyasachi Swain, Nadhir Al-Ansari, Ajay Kumar Taloor, and Deen Dayal. 2021. “Evaluation of an Urban Drainage System and Its Resilience Using Remote Sensing and GIS.” Remote Sensing Applications: Society & Environment 23:100601. https://doi.org/10.1016/j.rsase.2021.100601.
- Haynes, Teresa W, Sandra M Hedetniemi, Stephen T Hedetniemi, and Michael A Henning. 2002. “Domination in Graphs Applied to Electric Power Networks.” SIAM Journal on Discrete Mathematics 15 (4): 519–529. https://doi.org/10.1137/S0895480100375831.
- Hesarkazzazi, Sina, Amin E Bakhshipour, Mohsen Hajibabaei, Ulrich Dittmer, Ali Haghighi, and Robert Sitzenfrei. 2022. “Battle of Centralized and Decentralized Urban Stormwater Networks: From Redundancy Perspective.” Water Research 222:118910. https://doi.org/10.1016/j.watres.2022.118910.
- Holmgren, Åke J. 2006. “Using Graph Models to Analyze the Vulnerability of Electric Power Networks.” Risk Analysis 26 (4): 955–969. https://doi.org/10.1111/j.1539-6924.2006.00791.x.
- Ip, W. H., and Dingwei Wang. 2011. “Resilience and Friability of Transportation Networks: Evaluation, Analysis and Optimization.” Systems Journal, IEEE 5 (2): 189–198. https://doi.org/10.1109/JSYST.2010.2096670.
- Jia, Haifeng, L Yu Shaw Yuwen Lu, and Yurong Chen. 2012. “Planning of LID–BMPs for Urban Runoff Control: The Case of Beijing Olympic Village.” Separation and Purification Technology 84:112–119. https://doi.org/10.1016/j.seppur.2011.04.026.
- Kwietniewski, Marian, and Marcin Lesniewski. 2006. “The Reliability Assessment of a Typical Structure Fragment of a Stormwater Collection Network Including Uncertainty.” In Photonics Applications in Astronomy, Communications, Industry, and High-Energy Physics Experiments IV, pp. 61593A–61593A. International Society for Optics and Photonics. https://doi.org/10.1117/12.674878.
- Lahby, Mohamed, and Abderrahim Sekkaki. 2018. “A Graph Theory Based Network Selection Algorithm in Heterogeneous Wireless Networks.” In 2018 9th IFIP International Conference on New Technologies, Mobility and Security (NTMS), 1–4. IEEE. https://doi.org/10.1109/NTMS.2018.8328670.
- Lam, C. Y., and K. Tai. 2012. “Evaluating the Reliability of Infrastructure Networks by Resilience Analysis.” In Industrial Engineering and Engineering Management (IEEM), 2012 IEEE International Conference On, 1165–1169. Hong Kong, China: IEEE. https://doi.org/10.1109/IEEM.2012.6837926.
- Lewis, Ted G. 2014. Critical Infrastructure Protection in Homeland Security: Defending a Networked Nation. Hoboken, USA: John Wiley & Sons.
- Mah, Darrien Yau Seng, Afdal Haziq bin Mohamad Salehe, and Frederik Josep Putuhena. 2014. “Water Sensitive Urban Design in Existing Urban Settings: Case Study of Dry Detention Pond in Kuching City.” In InCIEC 2013: Proceedings of the International Civil and Infrastructure Engineering Conference 2013, Singapore, 315–322. Springer.
- Masucci, A Paolo, Kiril Stanilov, and Michael Batty. 2014. “Exploring the Evolution of London’s Street Network in the Information Space: A Dual Approach.” Physical Review E 89 (1): 12805. https://doi.org/10.1103/PhysRevE.89.012805.
- Mata‐Montero, Erick. 1991. “Resilience of Partial K -Tree Networks with Edge and Node Failures.” Networks 21 (3): 321–344. https://doi.org/10.1002/net.3230210305.
- Meijer, Didrik, Marco Van Bijnen, Jeroen Langeveld, Hans Korving, Johan Post, and François Clemens. 2018. “Identifying Critical Elements in Sewer Networks Using Graph-Theory.” Water 10 (2): 136. https://doi.org/10.3390/w10020136.
- Möderl, M., D. Butler, and W. Rauch. 2009. “A Stochastic Approach for Automatic Generation of Urban Drainage Systems.” Water Science and Technology 59 (6): 1137–1143. https://doi.org/10.2166/wst.2009.097.
- Mugume, Seith N, Diego E Gomez, Fu Guangtao, Raziyeh Farmani, and David Butler. 2015. “A Global Analysis Approach for Investigating Structural Resilience in Urban Drainage Systems.” Water Research 81:15–26. https://doi.org/10.1016/j.watres.2015.05.030.
- Najafi, Mohammad, and Guru Kulandaivel. 2005. “Pipeline Condition Prediction Using Neural Network Models.” Pipelines 2005: Optimizing Pipeline Design, Operations, and Maintenance in Today’s Economy 767–781. https://doi.org/10.1061/40800(180)61.
- Nardo Di, Armando, and Michele Di Natale. 2011. “A Heuristic Design Support Methodology Based on Graph Theory for District Metering of Water Supply Networks.” Engineering Optimization 43 (2): 193–211. https://doi.org/10.1080/03052151003789858.
- Nardo Di, Armando, Michele Di Natale, Giovanni F Santonastaso, Velitchko G Tzatchkov, and Victor H Alcocer-Yamanaka. 2013. “Water Network Sectorization Based on Graph Theory and Energy Performance Indices.” Journal of Water Resources Planning and Management 140 (5): 620–629. https://doi.org/10.1061/(ASCE)WR.1943-5452.0000364.
- Ostfeld, Avi. 2005. “Water Distribution Systems Connectivity Analysis.” Journal of Water Resources Planning and Management 131 (1): 58–66. https://doi.org/10.1061/(ASCE)0733-9496(2005)131:1(58).
- Pant, R. A. G. H. A. V., C. O. N. R. A. D. Zorn, S. C. O. T. T. Thacker, and J. W. Hall. 2018. “Systemic Resilience Metrics for Interdependent Infrastructure Networks.” In 6th International Symposium on Reliability Engineering and Risk Management (6ISRERM), Singapore, 37–42. Singapore.
- Porse, Erik, and Jay Lund. 2015. “Network Analysis and Visualizations of Water Resources Infrastructure in California: Linking Connectivity and Resilience.” Journal of Water Resources Planning and Management 142 (1): 4015041. https://doi.org/10.1061/(ASCE)WR.1943-5452.0000556.
- Porta, Sergio, Paolo Crucitti, and Vito Latora. 2006. “The Network Analysis of Urban Streets: A Dual Approach.” Physica A: Statistical Mechanics and Its Applications 369 (2): 853–866. https://doi.org/10.1016/j.physa.2005.12.063.
- Rashid, M. 2017a. “Urban Layout and Its Significance.” In The Geometry of Urban Layouts, 13–18. Switzerland: Springer.
- Rashid, Mahbub. 2017b. The Geometry of Urban Layouts. Switzerland: Springer.
- Reyes-Silva, Julian D, Jonatan Zischg, Christopher Klinkhamer, P. Suresh, C. Rao, Robert Sitzenfrei, and Peter Krebs. 2020. “Centrality and Shortest Path Length Measures for the Functional Analysis of Urban Drainage Networks.” Applied Network Science 5 (1): 1–14. https://doi.org/10.1007/s41109-019-0247-8.
- Rodrigues, Gustavo Paiva Weyne, Luis Henrique Magalhães Costa, Guilherme Marques Farias, and Marco Aurélio Holanda de Castro. 2019. “A Depth-First Search Algorithm for Optimizing the Gravity Pipe Networks Layout.” Water Resources Management 33 (13): 4583–4598. https://doi.org/10.1007/s11269-019-02373-x.
- Tran, Dung H, A. W. M. Ng, and B. J. C. Perera. 2007. “Neural Networks Deterioration Models for Serviceability Condition of Buried Stormwater Pipes.” Engineering Applications of Artificial Intelligence 20 (8): 1144–1151. https://doi.org/10.1016/j.engappai.2007.02.005.
- Vazquez, J., D. Bellefleur, D. Gilbert, and B. Grandjean. 1997. “Real Time Control of a Combined Sewer Network Using Graph Theory.” Water Science & Technology 36 (5): 301–308. https://doi.org/10.2166/wst.1997.0223.
- Vugrin, Eric D, Drake E Warren, Mark A Ehlen, and R. Chris Camphouse. 2010. “A Framework for Assessing the Resilience of Infrastructure and Economic Systems.” In Sustainable and Resilient Critical Infrastructure Systems, pp. 77–116. Springer. https://doi.org/10.1007/978-3-642-11405-2_3.
- Wang, Mo, Ming Liu, Dongqing Zhang, Qi Jinda, Fu Weicong, Yu Zhang, Qiuyi Rao, Amin E Bakhshipour, and Soon Keat Tan. 2023. “Assessing and Optimizing the Hydrological Performance of Grey-Green Infrastructure Systems in Response to Climate Change and Non-Stationary Time Series.” Water Research 232:119720. https://doi.org/10.1016/j.watres.2023.119720.
- Wanielista, Martin P, and Yousef A Yousef. 1993. Stormwater Management. New York: John Wiley & Sons.
- Yazdani, Alireza, and Paul Jeffrey. 2011. “Applying Network Theory to Quantify the Redundancy and Structural Robustness of Water Distribution Systems.” Journal of Water Resources Planning and Management 138 (2): 153–161. https://doi.org/10.1061/(ASCE)WR.1943-5452.0000159.
- Yazdani, Alireza, and Paul Jeffrey. 2012. “Water Distribution System Vulnerability Analysis Using Weighted and Directed Network Models.” Water Resources Research 48 (6). https://doi.org/10.1029/2012WR011897.
- Zischg, J., C. Klinkhamer, X. Zhan, E. Krueger, S. Ukkusuri, P. S. C. Rao, W. Rauch, and R. Sitzenfrei. 2017. “Evolution of Complex Network Topologies in Urban Water Infrastructure.” World Environmental and Water Resources Congress 2017 648–659. https://doi.org/10.1061/9780784480625.061.
- Zorn, Conrad, Raghav Pant, Scott Thacker, and Asaad Y Shamseldin. 2020. “Evaluating the Magnitude and Spatial Extent of Disruptions Across Interdependent National Infrastructure Networks.” ASCE-ASME Journal of Risk and Uncertainty in Engineering Systems, Part B: Mechanical Engineering 6 (2): 20904. https://doi.org/10.1115/1.4046327.