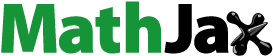
ABSTRACT
In this paper, we present a general formulation for the production inventory optimal control problem to a class of fractional fuzzy differential systems with reliability-dependent imperfect production. we investigate this model under the uncertain environment of fuzzy numbers with the rate of change of stock level expressed by granular Caputo fractional fuzzy derivative of order . The demands depend on the selling price, product quality, and stock level of the product displayed in the store. The unit production cost depends on development cost due to reliability, raw material cost, and wear tear cost. Here state, co-state variables and parameters are taken as uncertain variables with triangular fuzzy numbers and using granular dierentiability the existence and uniqueness of solution to the fractional dierential system with fuzzy variables and parameters. The production inventory optimal control problem for the fractional system is proposed and a necessary condition for optimality is obtained. Finally, this optimal control problem was solved by using the Pontryagin Maximum principle and for numerical results and graphical representation.
1. Introduction
Reliability of a system is a function of its component that is reliability is the capability of a device to work without any breakdown of the system components. In a complex system, smooth functioning of the components is not only required but also depends upon its design as well as on various interconnections. Environmental conditions like humidity, pressure and vibrations also affect on reliability of a system. Reliability is compactly connected with the quality of a product and is repeatedly deliberated as a subset of quality. To improve the quality of products and decrease defective rates, reliability plays an important role in the production process. Quality can be defined qualitatively as the amount by which the product satisfies the customer's demands. Reliability is worried about how long the product is uninterrupted to function once becomes operational. An impoverished quality product will alike have poor reliability and a nobel quality product will alike have a nobel reliability. Reliability deals with decreased failures over a time interval. All items will be of proper quality in the production inventory system, this is an unrealistic assumption. So in the long-run production process production of deteriorative units is a natural phenomenon. In the present emulative market, in the long-run business system, the demand faces competition and sales decayed by depreciation. All manufacture company wants to produce perfect quality but in real life, this is impossible during the production run-time due to raw material problem, machine breakdown, labour problems, etc., the system produces defective items. To make imperfect quality items into perfect items used and reworked at a cost and the perfect quality items are ready for sale. In the competition market, different manufacture companies produced many types of the item for the same product. The customers have to select the items with the best quality, highest reliability and lowest cost. Some researchers developed some models in this direction. Panda et al. [Citation1] developed an inventory model with stochastic demand and imperfect production under uncertain and chance constraints. Panda and Maiti [Citation2] developed a multi-item inventory model with price-dependent demand under reliability and flexibility consideration with imprecise space constraints. Sarkar [Citation3] gives an inventory model with reliability in an imperfect production process. Hazari et al. [Citation4] presented a reliability-dependent imperfect production inventory control problem in a bi-fuzzy environment. Roul et al. [Citation5] developed a multi-item reliability-dependent imperfect production inventory optimal control model. Khara et al. [Citation6] developed an inventory model under reliability-dependent demand and development cost-dependent imperfect production. In this paper, we deliberated a production inventory reliability-dependent manufacturing system. To decrease the production of imperfect items and increase the production of perfect items, the system has to be more reliable. To make the products more reliable, we consider that the material cost, the development cost and the unit production cost depend on the reliability parameter.
In traditional model formulation, all the data or variables or parameters in the form of input or output are deterministic. Normally production inventory optimal control models are presented with crisp demand. In real life demand for an item in a competitive market varies and fluctuates. Due to fluctuating demand, we cannot fit exact demand, for this, the demand can be expressed as a fuzzy number. Then it is called fuzzy demand. The traditional models are presented with deterministic variables, parameters and constraints whereas fuzzy models take on imprecise/vague variables, parameters and constraints which are more realistic and practical. In the case of crisp models, the imprecise data is replaced by average data. Normally conventional production inventory optimal control models consider ordinary differential equations as subjective conditions, but first, we consider the production inventory optimal control model with a fractional differential equation.
Now, in the real-world complexity increases day to day which is the main cause of facing some ambiguity/imprecision in various configurations. Ambiguity is inborn in most real-world systems. Besides randomness, fuzziness is also an important ambiguity, which acts as an indispensable part of the real actual situation [Citation7–9]. The fuzzy set theory has been presented by the scientist on Cybernetics Zadeh [Citation10] in 1965. In the last some decades, so many researchers have done their research on the fuzzy dynamical system. The concept of fuzzy derivative was first introduced by Cheng and Zadeh [Citation11], it was followed by Dubois and Parde [Citation12] who used the extension principle in their attack. Najariyan and Farahi [Citation13] studied the fuzzy optimal control of linear dynamical system with uncertain initial conditions and with uncertain parameters. They used the notation of strongly generalised Hukuhara (SGH) differentiability in a fuzzy dynamical system and for designing the fuzzy optimal control problems using the generalised Hukuhara (gH) differentiability concept [Citation14].
With important petition in clustering algorithms [Citation15] measurement ambiguity [Citation16], etc. granular computing has attracted more attention in current years. Granularity can be deliberated as a concept that reflects detailed information. Piegat with his co-workers used granular computing in the crisp and fuzzy interval arithmetic. Piegat and Landowski [Citation17] investigated crisp interval arithmetic depending on the notion of Relative Distance Measure (RDM) variables and served multidimensional RDM interval arithmetic. With effective results achieved, they displayed how necessary multidimensional RDM interval mathematics is for interval computations in comparison with standard interval mathematics. Additionally, in connection with fuzzy arithmetic, they inaugurated the notation of horizontal membership functions with the RDM variables [Citation18]. With an accession called RDM fuzzy interval mathematics commenced, they published that the result of granule gained by this accession is more reliable than the result gained by FSIA. Hereafter, depending on the Relative Distance Measure(RDM) interval mathematics and the horizontal membership functions, Mazandarani et al. [Citation19] mentioned a new definition of the differentiability of fuzzy functions called granular differentiability (gr-differentiability) [Citation20]. They confirmed that by considering FDEs under gr-differentiability and RDM interval mathematics.
In applied mathematics, optimal control theory deals with the problem to find the most appropriate control input subjecting to a given dynamic system in order to maximise or minimise the objective function. An optimal control is a set of differential equations recounting the path of the variables that maximise or minimise a function of co-state and state variables. In general, optimal control problems in both science and engineering are considered integer types of differential equations studied for a long time by many researchers. Combining the optimal control problem and fractional derivative, which is known as the fractional optimal control problem, is one of the most sensational challenges for mathematical research. Recently, some researchers consider an optimal control problem to be a fractional optimal control problem when either the dynamic constraints or objective function or both include fractional order derivative term [Citation21]. In the recent two decades, some researchers have paid attention to the fractional differential equation. There are few numbers of publications on fractional optimal control problems with uncertainty [Citation22]. The idea of combining fractional optimal control theory with fuzzy theory opened up many exciting applied problems in different areas of Mathematics, engineering and science. Garai et al. [Citation23] submitted a fuzzy multi-objective linear fractional inventory model using possibility and necessity constraints. Waliv et al. [Citation24] presented a stochastic multi-objective inventory model with a nonlinear programming approach to uncertain information. Giri et al. [Citation25] solved a price and quality-dependent multi-item inventory model under the possibilistic mean of a generalised nonlinear intuitionistic fuzzy number. Mazandrani et al. [Citation20] presented some necessary conditions for the optimality of fuzzy bang-bang control and sub-optimal control problems for the fuzzy dynamic system under granular differentiability. Najariyan and Zhao [Citation26] also developed the granular fractional derivatives and fractional differential system. Under granular fractional derivatives, these concepts were used to investigate the optimal conditions for the fuzzy fractional linear quadratic regulator problem. Son et al. [Citation27] presented an optimal control problem for a granular neutrosophic fractional differential equation with some application in engineering. Mohammed Mustafa et al. [Citation28] presented the solution to a fuzzy optimal control problem with fuzzy variation under the granular differentiability idea. De et al. [Citation29] studied a fuzzy production model of assortment items under a granular approach to control the preservation cost. Khatua et al. [Citation30] presented a fuzzy production inventory control model under gr-differentiability approach. Hati et al. [Citation31] presented a multi-objective pollution control innovation inventory model with stock-dependent demand in a finite time horizon. Also some researchers presented some ideas on the fuzzy granular approach [Citation32,Citation33].
In the real world with globalisation, the rise of new technology and the evaluation of present world marketplace over time Monopolists wants to find methods to design and develop innovation that is usually visible to the customer and should result in the maximum demand for the product. Based on our knowledge, very few researchers have constructed the fuzzy optimal control model. In the production control model, many researchers take the variables such as production cost and selling price, are crisp in nature. But in real life, we face more and more problems, which are uncertain in nature. For this reason, the fuzzy model with fuzzy variables such as production rate, selling price, demand rate, product quality, production cost and stock level is more appropriate and realistic. First time in this paper, we have introduced the fractional fuzzy optimal control inventory model with reliability-dependent imperfect production under fuzzy granular differentiability.
The main achievements of the paper are as follows:
For the first time, fractional fuzzy optimal control is applied to the production inventory model.
Presenting a fractional fuzzy optimal control production inventory model with reliability-dependent imperfect production.
Here the demands are selling price, stock level and quality dependent. Demand is deliberated as decreasing on the selling price and increasing on quality of product and stock level of product.
Using the granular differentiability concept and the optimal control law solve this fractional fuzzy dynamical model.
The remainder of the is organised as follows: The fuzzy dynamical system and fractional derivative are described in Section 2. The formulation of fractional fuzzy production inventory model in Section 3. The optimal condition of the model is in Section 4. Numerical result and some characteristics are discussed in Section 5. Some decisions on the result are in Section 6. Some Managerial insights discussed in Section 7. Some conclusions of this model are in Section 8.
Finally, we solved the optimal control problem by using the Pontryagin Maximum principle. Then to obtain the numerical result by using MATLAB, an algorithm is also developed. Subsequently, the numerical results are presented both in tabular form and graphically.
2. Fuzzy Dynamic System and Fractional Derivative
In this section, we recall some necessary definitions, theorems and proposition on the fuzzy dynamical system and fractional integral and derivative. Here the set of all real numbers is denoted be R, the set of all fuzzy numbers on R by .
Definition 2.1
[Citation34]
The fuzzy set is called a fuzzy number if it is normal, fuzzy convex, upper semi-continuous and compactly supported fuzzy subsets of real numbers. The fuzzy number
can be represented in a parametric form by the ordered pair of functions
, satisfying the following properties:
is a bounded non-decreasing left continuous function in (0,1], and it is right continuous at
.
is a bounded non-decreasing left continuous function in (0,1], and it is right continuous at
.
.
Based on fuzzy standard interval arithmetic; addition, subtraction, and multiplication of two fuzzy numbers and
are characterised as follows, respectively:
Addition : | [ | ||||
Subtraction : | [ | ||||
Multiplication : | [ |
Definition 2.2
[Citation35]
The fuzzy function is said to be generalised Hukuhara (gH) differentiable at
, if there exists a fuzzy number
such that the following limit exists
where ‘
’ stands for generalised Hukuhara difference and means
or
.
Definition 2.3
[Citation19]
Let be a fuzzy number. The horizontal membership function
is a representation of
as
in which ‘gr’ stands for the granule of information included in
is the membership degree of x in
is called relative distance measure (RDM) variable, and
.
Note
The horizontal membership function of is also denoted by
. Moreover, if the triangular fuzzy number
is denoted by triple (d,e,f),
, then the horizontal membership function of
can be characterised as
.
Definition 2.4
[Citation20]
Two fuzzy numbers and
are said to be equal if and only if H(
)=H(
) for all
.
Definition 2.5
[Citation20]
Let and
be two fuzzy numbers whose horizontal membership functions are
and
respectively and ‘⊙’ denote one of the four basic operations, i.e. addition, subtraction, multiplication, and division. Then
is a fuzzy number
such that H(
)=
. It should be noted that 0 does not belong to
when ‘⊙’ denotes the division operator.
Definition 2.6
[Citation20]
Let include n
distinct fuzzy numbers
,
,…..,
. The horizontal membership function of
at the point t
is denoted by H(
)=
and defined as
: [a, b]×[0, 1]×[0, 1]
[0, 1]
[a,b]
in which
=
are the RDM variables corresponding to the fuzzy numbers.
Definition 2.7
[Citation19]
The fuzzy function to be granular differentiable (gr-differentiable) at
if there exists a fuzzy number
such that the following limit exits:
.
Theorem
The fuzzy function is granular differentiable at the point
if and only if its horizontal membership function is differentiable with respect to t at that point. However, H
.
Definition 2.8
[Citation20]
Let mapping t
be a continuous fuzzy function whose horizontal membership function, i.e.
, is integrable on t
. Let
denote the integral of
on [a, b]. Then the fuzzy function
is said to be granular integrable (gr-integrable) on [a, b] if there exists a fuzzy number
such that
.
Proposition 2.1
Suppose the fuzzy function mapping t
is gr-differentiable, and
is continuous on[a, b]. Then
.
Proposition 2.2
Let the fuzzy function mapping t
and
mapping t
satisfy the conditions mentioned in Definition 2.5. Then, the relation
holds.
Proposition 2.3
Let the fuzzy function defined by t
is said to be granular partial differential w.r.t the ith variable
if and only if its horizontal membership function is differentiable w.r.t variable
. i,e
for all
Definition 2.9
[Citation36]
The Caputo fractional derivative of order
of
is defined by
Where
,
and
.
Definition 2.10
[Citation26]
Let . Then, the right-sided and left-sided granular fractional integrals of order
of the function f is defined as follows
Remark 2.1
According to the above definition we can define the form of horizontal membership function of granular fractional integral as follows
Similarly, we also have
Definition 2.11
[Citation26]
Let be a granular differentiable function. Then, the right-sided and left-sided granular Caputo fractional derivatives of order
of the function f are defined as follows
Remark 2.2
By similar arguments as in Remark-2.1 we can also conclude that
.
.
2.1. Sixtuple Shortcoming of GH-differentiability
Najariyan and Farahi presented fuzzy optimal control of a linear dynamical system with fuzzy initial conditions with fuzzy parameters. They used the notation of Strongly Generalised Hukuhara (SGH) differentiability in a fuzzy dynamical system. But SGH-derivative sustains some limitations such as (i) It does not always exist. (ii) It necessitates the monotony of fuzzyness. Due to the limitations of the SGH-differentiability notation they checked a class of fuzzy optimal control problems using the gH-differentiability idea. (iii) Even though the gH-differentiability notation does not necessities the monotony of the solution of uncertain and also the existence of this kind of derivative cannot be secured. Thus the direction emerged on SGH-differentiability and gH-differentiability notation sustain from a primary shortcoming entitled unnatural behaviour in modelling phenomenon. (iv) The solutions obtained by solving the fuzzy differential equation is different from each other. (v) There are some other disadvantages connected with SGH-derivatives, gH-derivatives such as the doubling features and multiplicity of solutions which were presented in some research papers. (vi) Also due to some disadvantages such as doubling property and multiplicity of solutions connected with g-derivatives, H-derivative. These limitations motivated us to examine the fuzzy optimal control problem with fractional derivatives using a granular differentiability approach. With the significant petition in clustering algorithms, assessment ambiguity, etc. granular computing has interested more attachment in recent years. Granularity can be deliberated as an idea that reflects clear information. Mohammed Mustafa et al. [Citation28] presented the solution to a fuzzy optimal control problem with fuzzy variation under the granular differentiability idea. De et al. [Citation29] studied a fuzzy production model of assortment items under a granular approach to control the preservation cost. Khatua et al. [Citation30] presented a fuzzy production inventory control model under the gr-differentiability approach. Also, some researchers presented some ideas on the fuzzy granular approach [Citation32,Citation33]. For this purpose, we presented a reliability-dependent imperfect fuzzy production control model under a granular differentiability approach.
3. Formulation of Fractional Fuzzy Production Inventory Model
In this paper, under the following assumptions and notations, we developed a fractional fuzzy production inventory optimal control model.
3.1. Assumptions
A single-period inventory model with a finite time horizon has been considered.
Stock dependent, product price dependent, product quality dependent imprecise demand function is considered here.
The salvage value price of the finish stock is fuzzy in nature.
Depreciation rates of product quality (due to old technology) and production cost function are constant.
The effects of time value of money and inflation are negligible here.
All decision variables are fuzzy in nature.
Co-state variables are fuzzy in nature.
Shortages are not allowed.
Rate of defectiveness depends on time and reliability.
Production rate and selling price are a function of time which are taken as control variables.
3.2. Notation
X~(t) : | Stock of the product which is fuzzy in nature. | ||||
U~(t) : | Unit production rate at time t which is fuzzy in nature. | ||||
P~(t) : | At time t, fuzzy price of a product. | ||||
C~(t) : | At time t fuzzy production cost. | ||||
Q~ : | Fuzzy quality of production. | ||||
d~0 : | Constant part of fuzzy demand function. | ||||
a1 : | Rate of responsiveness of item's consumer demand to its price. | ||||
a2 : | Rate of responsiveness of item's consumer demand to its quality. | ||||
a3 : | Rate of responsiveness of item's consumer demand to its stock. | ||||
C~u0 : | Raw material cost. | ||||
C~u1 : | Development cost dependent on reliability parameter. | ||||
C~u2 : | Wear tear cost. | ||||
C(r,t) : | Unit production cost which depends on raw material cost, production rate, wear tear cost and development cost. | ||||
r~ : | fuzzy reliability parameter. | ||||
h~ : | Holding cost. | ||||
α : | Defective rate, | ||||
Ps~ : | Salvage value which is fuzzy in nature. |
3.3. Description of Mathematical Model
In this paper, we consider a fractional fuzzy optimal control inventory problem over a continuous finite time horizon whose constraints are fuzzy in nature.
In this model, we considered a single-item optimal control production inventory with dynamic selling pricing and reliability-dependent imperfect production. Here the items are produced at a changeable rate U(t) of which (where
) is a reliability-dependent defective rate. The reliability decreases the defective rate and increases the quality of product. The imperfect items are treated as disposal part. Therefore the rate of change of stock level X(t) representing the system during a finite time horizon, T is
(1)
(1)
Where (.) denotes differentiation.
The corresponding fractional fuzzy fractional differential system is
(2)
(2)
Here the demand depends on the selling price, quality, and stock level of the product. Demand is deliberated as decreasing on the selling price and increasing on quality of product and stock level of product.
(3)
(3)
Here the unit production cost is considered as a function of raw material cost, product quantity, wear tear cost and development cost. Therefore the unit production cost is
(4)
(4)
The final stock
is sold with salvage value
where
and the corresponding marginal income is
. Then the objective function is
Subject to constraints
i.e.
(5)
(5)
Subject to constraints
(6)
(6)
4. Optimal Condition
We develop the Hamiltonian function of fractional fuzzy optimal control problem as below
(7)
(7)
Where
is Lagrange multiplier. Then the following theorem will give the necessary condition for optimality.
Theorem
Assume that is an optimal solution of fractional fuzzy optimal control problem. Then this
satisfies the following conditions.
(8)
(8)
where the first equation is known as a co-state system, the second and third are the stationary condition, the fourth equation is known as a state system and the last equation is known as the transversality condition.
Proof.
By using the formula of the Hamiltonian function (Equation10(10)
(10) ) the objective function (Equation8
(8)
(8) ) can be rewritten as follows
For
sufficiently small. Let us consider the following function
The increment of
is
(9)
(9)
Where
It is known that if is a maximiser of the objective function, then the increment
must be always non-positive i.e,
or equivalently
By using the horizontal membership function approach, we get
in which
For all
. By applying Proposition 2.3, the equation becomes
Now, we have by the definition
Thus, it implies
Using the integral by parts formula for granular Caputo fractional derivative, we get
Therefore the necessary condition for the optimality of the fractional fuzzy optimal control problem depends on the maximisation of
. This requires
or the coefficient of
,
,
,
must be zero. Hence, we obtained
Hence the system equivalent to
Therefore, the proof is completed.
By using fuzzy Granular differentiability(c.f. Section 2) the Hamiltonian function change in the following form, that is the horizontal membership function of Equation (Equation10(10)
(10) ) is
We establish the necessary conditions for maximising the granular fractional optimal control problem by applying the system (11).
The state system is
(10)
(10)
The stationary conditions are
The co-state system is
For finding the numerical values of all variables (control variables, state variables, co-state variables) and graphical values, we use the Runge–Kutta forward and backward method in MATLAB software.
This proposed model can be applied in various fields of manufacture system, like phone and television manufacturing, battery manufacturing, washing machine, etc. The illustration of this model is more realistic for using the fractional order under the granular differentiability approach. The observation can be expanded for deteriorating items with space constraints, advertisement-dependent demand, time-dependent demand, discount, etc.
5. Numerical Result
To represent the above production inventory reliability-dependent fractional fuzzy optimal control model numerically, we consider crisp and fuzzy input data given in Tables and . For these crisp and fuzzy input data, the optimum selling price, stock level, production rate, demand rate and profit are given in Tables – and also shown in Figures – for different values of β.
Figure 1. The μ-level set of selling price for . The ten curves show left and right end points of μ-level sets of fuzzy function respectively, for
. The top most line indicates the upper level of selling price
and the bottom line indicates the lower level of selling price
.
![Figure 1. The μ-level set of selling price for β=0.8. The ten curves show left and right end points of μ-level sets of fuzzy function respectively, for μ∈[0,1]. The top most line indicates the upper level of selling price Pu(t) and the bottom line indicates the lower level of selling price Pl(t).](/cms/asset/22a71c50-52e1-4e26-9ff9-8aa75ec50189/tfie_a_2152885_f0001_oc.jpg)
Table 1. Values of unchangeable parameter.
Table 2. Input data of fuzzy parameters.
Table 3. The value of selling price, stock level of product, production rate, demand rate, profit at time T for .
Table 4. The value of selling price, stock level of product, production rate, demand rate, profit at time T for .
Table 5. The value of selling price, stock level of product, production rate, demand rate, profit at time T for .
5.1. Optimum Result for 

By using the numerical value of constant parameter from Table and fuzzy parameters from Table we get the optimal value of state and control variable for . The optimum selling price
, stock level
, production rate
, demand rate
and profit
are given in Table . We get the following optimum values are
,
,
and
. The graphical representation of selling price, stock level, production rate, demand rate and profit versus time shown in Figures 1–5 respectively.
5.2. Optimum Result for 

By using the numerical value of constant parameter from Table and fuzzy parameters from Table we get the optimal value of state and control variable for . The optimum selling price
, stock level
, production rate
, demand rate
and profit
are given in Table . We get the following optimum values
,
,
and
. The graphical representation of selling price, stock level, production rate, demand rate and profit versus time shown in Figures 6–10 respectively.
5.3. Optimum Result for 

By using the numerical value of constant parameter from Table and fuzzy parameters from Table we get the optimal value of state and control variable for . The optimum selling price
, stock level
, production rate
, demand rate
and profit
are given in Table . We get the following optimum values are
,
,
and
. The graphical representation of selling price, stock level, production rate, demand rate and profit versus time shown in Figures 11–15 respectively.
6. Result Analysis
After seeing the result we made some decisions, which are given below -- from Tables – we see that for different values of β selling price decrease. Which is also graphically shown in Figures , and . In general, better quality and low selling price increase product demand. In this production inventory model, demand is dependent on a selling price that is decreasing nature of the selling price increase the demand for the product. Here from Tables – see that for different values of β demand increases. Graphical representation of demand for different value of β shown in Figures , and . So in present competitive market our model will be more helpful to meet the demands of manufacturing company. From Tables and we see that the stock level of product first increase and then decrease for and
. And also for
from Table , we see that the stock level of product increases. The stock level of product for different values of β is also graphically shown in Figures , and . Rate of change of unit production for different values β is shown graphically in Figures , and . From Tables – we see that total profit of inventory is increasing which also graphically shown in Figures , and . Here from the output data table, we get the value of selling price, production rate, demand of the product, stock of product all are intervals, not the particular values which make the decision more realistic. This model help the company/manufacturer to take the more real decision on wear house, production house, production cost and selling price. Since input and output are fuzzy in nature, so in real-world information our model is more realistic and also will be more helpful for manufacturing company to increase their profit and decrease production costs in real situation.
Figure 2. The μ-level set of stock level for . The ten curves show left and right end points of μ-level sets of fuzzy function respectively, for
. The top most line indicates the upper level of stock level
and the bottom line indicates the lower level of stock level
.
![Figure 2. The μ-level set of stock level for β=0.8. The ten curves show left and right end points of μ-level sets of fuzzy function respectively, for μ∈[0,1]. The top most line indicates the upper level of stock level Xu(t) and the bottom line indicates the lower level of stock level Xl(t).](/cms/asset/7109ca8e-b25d-47fe-ac21-b9a40a591475/tfie_a_2152885_f0002_oc.jpg)
Figure 3. The μ-level set of unit production for . The ten curves show left and right end points of μ-level sets of fuzzy function respectively, for
. The top most line indicates the upper level of unit production
and the bottom line indicates the lower level of unit production
.
![Figure 3. The μ-level set of unit production for β=0.8. The ten curves show left and right end points of μ-level sets of fuzzy function respectively, for μ∈[0,1]. The top most line indicates the upper level of unit production Uu(t) and the bottom line indicates the lower level of unit production Ul(t).](/cms/asset/8516e147-3a1b-4784-8c63-30f54ae6a6b0/tfie_a_2152885_f0003_oc.jpg)
Figure 4. The μ-level set of demand for . The ten curves show left and right end points of μ-level sets of fuzzy function respectively, for
. The top most line indicates the upper level of demand
and the bottom line indicates the lower level of demand
.
![Figure 4. The μ-level set of demand for β=0.8. The ten curves show left and right end points of μ-level sets of fuzzy function respectively, for μ∈[0,1]. The top most line indicates the upper level of demand Du(t) and the bottom line indicates the lower level of demand Dl(t).](/cms/asset/82a616ff-3897-44ea-97b2-6a1c0ed30008/tfie_a_2152885_f0004_oc.jpg)
Figure 5. The μ-level set of total profit for . The ten curves show left and right end points of μ-level sets of fuzzy function respectively, for
. The top most line indicates the upper level of total profit
and the bottom line indicates the lower level of total profit
.
![Figure 5. The μ-level set of total profit for β=0.8. The ten curves show left and right end points of μ-level sets of fuzzy function respectively, for μ∈[0,1]. The top most line indicates the upper level of total profit Ju(t) and the bottom line indicates the lower level of total profit Jl(t).](/cms/asset/aa06f28d-8d42-42e8-9058-bbed33bf2dc9/tfie_a_2152885_f0005_oc.jpg)
Figure 6. The μ-level set of selling price for . The ten curves show left and right end points of μ-level sets of fuzzy function respectively, for
. The top most line indicates the upper level of selling price
and the bottom line indicates the lower level of selling price
.
![Figure 6. The μ-level set of selling price for β=0.9. The ten curves show left and right end points of μ-level sets of fuzzy function respectively, for μ∈[0,1]. The top most line indicates the upper level of selling price Pu(t) and the bottom line indicates the lower level of selling price Pl(t).](/cms/asset/fc44fd4f-bf66-4837-9da6-42fa47c7c73b/tfie_a_2152885_f0006_oc.jpg)
Figure 7. The μ-level set of stock level for . The ten curves show left and right end points of μ-level sets of fuzzy function respectively, for
. The top most line indicates the upper level of stock level
and the bottom line indicates the lower level of stock level
.
![Figure 7. The μ-level set of stock level for β=0.9. The ten curves show left and right end points of μ-level sets of fuzzy function respectively, for μ∈[0,1]. The top most line indicates the upper level of stock level Xu(t) and the bottom line indicates the lower level of stock level Xl(t).](/cms/asset/f7537550-ee75-4112-b398-23a316fecc93/tfie_a_2152885_f0007_oc.jpg)
Figure 8. The μ-level set of unit production for . The ten curves show left and right end points of μ-level sets of fuzzy function respectively, for
. The top most line indicates the upper level of unit production
and the bottom line indicates the lower level of unit production
.
![Figure 8. The μ-level set of unit production for β=0.9. The ten curves show left and right end points of μ-level sets of fuzzy function respectively, for μ∈[0,1]. The top most line indicates the upper level of unit production Uu(t) and the bottom line indicates the lower level of unit production Ul(t).](/cms/asset/102faa49-4642-4b45-899f-ccb6032c7594/tfie_a_2152885_f0008_oc.jpg)
Figure 9. The μ-level set of demand for . The ten curves show left and right end points of μ-level sets of fuzzy function respectively, for
. The top most line indicates the upper level of demand
and the bottom line indicates the lower level of demand
.
![Figure 9. The μ-level set of demand for β=0.9. The ten curves show left and right end points of μ-level sets of fuzzy function respectively, for μ∈[0,1]. The top most line indicates the upper level of demand Du(t) and the bottom line indicates the lower level of demand Dl(t).](/cms/asset/1a8db3b2-c30a-46ac-9749-e95cb0da4c15/tfie_a_2152885_f0009_oc.jpg)
Figure 10. The μ-level set of total profit for . The ten curves show left and right end points of μ-level sets of fuzzy function respectively, for
. The top most line indicates the upper level of total profit
and the bottom line indicates the lower level of total profit
.
![Figure 10. The μ-level set of total profit for β=0.9. The ten curves show left and right end points of μ-level sets of fuzzy function respectively, for μ∈[0,1]. The top most line indicates the upper level of total profit Ju(t) and the bottom line indicates the lower level of total profit Jl(t).](/cms/asset/4b9b0a91-72ae-4d0b-9c23-37e2079dcbe9/tfie_a_2152885_f0010_oc.jpg)
Figure 11. The μ-level set of selling price for . The ten curves show left and right end points of μ-level sets of fuzzy function respectively, for
. The top most line indicates the upper level of selling price
and the bottom line indicates the lower level of selling price
.
![Figure 11. The μ-level set of selling price for β=1. The ten curves show left and right end points of μ-level sets of fuzzy function respectively, for μ∈[0,1]. The top most line indicates the upper level of selling price Pu(t) and the bottom line indicates the lower level of selling price Pl(t).](/cms/asset/1ebae8ff-6f60-4420-8283-f3203ee5886f/tfie_a_2152885_f0011_oc.jpg)
Figure 12. The μ-level set of stock level for . The ten curves show left and right end points of μ-level sets of fuzzy function respectively, for
. The top most line indicates the upper level of stock level
and the bottom line indicates the lower level of stock level
.
![Figure 12. The μ-level set of stock level for β=1. The ten curves show left and right end points of μ-level sets of fuzzy function respectively, for μ∈[0,1]. The top most line indicates the upper level of stock level Xu(t) and the bottom line indicates the lower level of stock level Xl(t).](/cms/asset/d0e7ef84-6128-4d78-a0af-d867785534da/tfie_a_2152885_f0012_oc.jpg)
Figure 13. The μ-level set of unit production for . The ten curves show left and right end points of μ-level sets of fuzzy function respectively, for
. The top most line indicates the upper level of unit production
and the bottom line indicates the lower level of unit production
.
![Figure 13. The μ-level set of unit production for β=1. The ten curves show left and right end points of μ-level sets of fuzzy function respectively, for μ∈[0,1]. The top most line indicates the upper level of unit production Uu(t) and the bottom line indicates the lower level of unit production Ul(t).](/cms/asset/00ebbdb2-22bb-4143-b43e-c47a20b44f91/tfie_a_2152885_f0013_oc.jpg)
7. Managerial Insights
In this article, we have studied a reliability-dependent imperfect production inventory optimal control fractional order model for an uncertain environment under granular differentiability. We have evaluated several decision variables like price, stock and production rate. A manager can solve/tackle any kind of complex situation in an entire industry with proper insights. This study has the performance based on a granular differentiability approach with fractional order to find the maximum profit of the manufacture company. Managerial insights in the following way. (i) The reliability decrease the defective rate and increase the quality of the product. (ii) The imperfect item is treated as a disposal part. (iii) Demand function is dependent on selling price, quality of the product and stock level of product which presents the demand as more realistic. (iv) Rate of change of stock level is of fractional order in an uncertain environment.
8. Conclusion
We have presented a production inventory optimal control model with fractional order dynamical system in the fuzzy environment under granular derivatives. In this considered model the imprecise and fuzziness data are operated by using the horizontal membership function approach. The important result concentrate on optimal control problem and solvability for an abstract class of fractional differential system with uncertainty relating to some production inventory. All production company wants to increase the production demands and profit more. To increase profit, the machinery system has to go through a long-run process. In the long-run process due to machine breakdown, the imperfect item is produced. The perfect items are ready for sale and the imperfect items are reworked at a cost to make the original quality of product. So the demands are dependent on quality, selling price, and stock level of product. This single-item deals with reliability-dependent defective rate, reliability and production rate dependent on unit production cost, raw material cost and wear tear cost. Here we introduced the marginal selling price of left stock for which profit increased. The fractional optimal control problem corresponding to this fractional dynamical system is investigated with the aim to maximise the profit. A blueprint to numerically solve the deliberated problem is also presented. For inventory optimal control model SGH-differentiability, gH-differentiability suffers from some drawbacks. The main advantage of this proposed model is the unnatural behaviour of the nonlinear fuzzy differential equation is not found by using the granular differentiability approach. Another advantage of this proposed model is the doubling property of solution for a fuzzy differential equation which arises for solving the fuzzy differential equation under SGH-differentiability or gH-differentiability is vanished by using a granular differentiability approach. This model can be applied in various fields of manufacture system, like phone and television manufacturing, battery manufacturing, washing machine, etc. The observation can be expanded for deteriorating items with space constraint, advertisement-dependent demand, time-dependent demand, discount, etc. In future, this model can be expanded in different environments such as stochastic, fuzzy-stochastic and rough-fuzzy with fractional derivatives under a granular-differentiability approach.
Ethical approval
The article does not contain any studies with human participants or animals performed by any of the authors.
Informed consent
Informed consent was obtained from all individual participants included in the study.
Disclosure statement
No potential conflict of interest was reported by the author(s).
Additional information
Funding
Notes on contributors
S. Hati
S. Hati is a Teacher in Department of Mathematics in Mugberia Gangadhar Mahavidyalaya, West Bengal, India. He qualified GATE in the year 2019. His research interests are in Inventory and optimal control of production systems in fuzzy, uncertain environments, fuzzy differential equations and fuzzy dynamical system.
K. Maity
K. Maity is an Associate Professor in Department of Mathematics in Mugberia Gangadhar Mahavidyalaya, West Bengal, India. He has received Prof. M.N.Gopalan Award for best doctoral thesis in Operational Research organized by ORSI, India, 2006. Dr. Maity has published at least 60 papers in International journals like IJOR, EJOR, AMC, AMM, MCM, Inf. Science, FODM, Opt. and Eng., Int. J. of System Science and other journal. His research led is inventory management, operation research, uncertain theory, fuzzy-rough optimization.
References
- Panda D, Kar S, Maity K, et al. A single period inventory model with imperfect production and stochastic demand under chance and imprecise constraints. Eur J Oper Res. 2008;188(1):121–139.
- Panda D, Maiti M. Multi-item inventory model with price dependent demand under flexibility and reliability consideration and imprecise space constraints, a geometric programming approach. Math Comput Model. 2009;49(9-10):1733–1749.
- Sarkar B. An inventory model with reliability in an imperfect production process. Appl Math Comput. 2012;218:4881–4891.
- Hazari S, Maity K, Dey JK, et al. Advertisement policy and reliabitlity dependent imperfect production inventory control problem in bi-fuzzy environment. Int J Oper Res. 2015;22(3):342–365.
- Roul JN, Maity K, Kar S, et al. Multi-item reliability dependent imperfect production inventory optimal control models with dynamic demand under uncertain resource constraint. Int J Prod Res. 2015;53(16):4993–5016.
- Khara B, Dey JK, Mondal SK. An inventory model under development cost dependent imperfect production and reliability dependent demand. J Manag Anal. 2017;4:258–275.
- Khatua D, De A, Maity K, et al. Use of e and g operators to a fuzzy production inventory control model for substitute items. RAIRO. 2017;53:473–486.
- Khatua D, Maity K. Stability of fuzzy dynamical system based on quasi-level-wise system. J Intell Fuzzy Syst. 2017;33(6):3515–3528.
- Khatua D, Maity K, Kar S. Product-process innovation and fuzzy learning effect in finite time horizon. Int J Fuzzy Syst. 2019;21(5):1560–1570.
- Zadeh LA. Fuzzy sets. Inf Control. 1965;8(3):338–353.
- Chang SL, Zadeh LA. On fuzzy mapping and control. IEEE Trans Syst Man Cybern. 1972;2:30–34.
- Dubois D, Prade H. Towards fuzzy differential calculus: part 3 differentiation. Fuzzy Sets Syst. 1982;8(3):225–233.
- Najariyan M, Farah MH. Optimal control of fuzzy linear controlled system with fuzzy initial conditions. Iran Fuzzy Syst. 2013;10:21–35.
- Najariyan M, Farahi MH. A new approach for solving a class of fuzzy optimal control system under generalised hukuhara differentiability. J Franklin Inst. 2015;352(5):1836–1849.
- Grcek A, Pedrycz W. Clustering granuler data and their characterization with information granules of higher type. IEEE Trans Fuzzy Syst. 2015;23(4):850–860.
- Qian Y, Li Y, Linga J, et al. Fuzzy granular structure distance. IEEE Trans Fuzzy Syst. 2015;23(6):2245–2259.
- Piegat A, Landowski M. Horizontal membership function and examples of its applications. Int J Fuzzy Syst. 2015;17(1):22–30.
- Piegat A., Landowski M. Is fuzzy number the right result of arithmetic operations on fuzzy number?. Adv Fuzzy Logic Techno. 2017;3:181–194.
- Mazandarani M, Pariz N, Kamyad AV. Granular differentiability of fuzzy-number-valued function. IEEE Trans Fuzzy Syst. 2018;26(1):310–323.
- Mazandarani M, Pariz N. Sub-optimal control of fuzzy linear dynamical system under granular differentiability concept. ISA Trans. 2018;76:1–17.
- Agrawal OP. A quadraic numerical scheme for fractional optimal control problems. J Dyn Syst Meas Control. 2008;130(1):011010–011016.
- Vu H, Hoa NV. Uncertain fractional differential equation on a time scale under granular differentiability concept. Comp Appl Math. 2019;38(3):38–110.
- Garai T, Garg H. Multi-objective linear fractional inventory model with possibility and necessity constraints under generalised intuitionistic fuzzy set environment. CAAI Trans Intell Technol. 2019;4(3):175–181.
- Waliv RH, Mishra U, Garg H, et al. A nonlinear programming approach to solve the stochastic multi-objective inventory model using the uncertain information. Arab J Sci Eng. 2020;45(8):6963–6973.
- Giri SK, Garai T, Garg H, et al. Possibilistic mean of generalized non-linear intuitionistic fuzzy number to solve a price and quality dependent demand multi-item inventory model. Comput Appl Math. 2020;40:110–134.
- Najariyan M, Zhao Y. Fuzzy fractional quadratic regulator problem under granular fuzzy fractional derivatives. IEEE Trans Fuzzy Syst. 2018;26(4):2273–2288.
- Son NTK, Dong NP, Long HV, et al. A linear quadratic regulator problem governed by granular neutrosophic fractional differential equations. ISA Trans. 2020;97:296–316.
- Mustafa M, Gong Z, Osman M. The solution of fuzzy variational problem and fuzzy optimal control problem under granular differentiability concept. Int J Comput Math. 2020;98(4):1495–1520.
- De A, Khatua D, Kar S. Control the preservation cost of a fuzzy production inventory model of assortment items by using the granular differentiability approach. Comput Appl Math. 2020;25(4):2687–2701.
- Khatua D, Maity K, Kar S. A fuzzy production inventory control model using granular differentiability approach. Soft Comput. 2021;25(4):2687–2701.
- Hati S, Maity K. Pollution control multi-objective inventory model of product-process innovation with stock dependent demand and gathering knowledge accumulation in finite time horizon. Contemp Issues Bus Econ. 2022;1:83–100.
- Najariyan M, Zhao Y. Granular fuzzy PID controller. Expert Syst Appl. 2021;167:114182.
- Najariyan M, Qiu L. Interval type-2 fuzzy differential equation and stability. IEEE Trans Fuzzy Syst. 2021;30(8):2915–2929.
- Zimmermann HJ. Fuzzy set theory and its application. New York: Spinger Business Economics; 2001.
- Bede B, Stefanini L. Generalized differentiability of fuzzy valued function. Fuzzy Set Syst. 2013;230:119–141.
- Kilbas AA, Srivastava HM, Trujillo JJ. Theory and applications of fractional differential equation. Amsterdam: Elsevier; 2006. (North-Holland mathematics studies, 204).