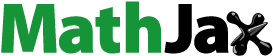
Abstract
A new area of research called intuitionistic fuzzy soft expert set is expected to overcome the drawbacks of an intuitionistic fuzzy soft set in terms of eligibility for soft expert-argument approximate function. This type of function views the power set of the universe as its co-domain and the cartesian product of attributes, experts, and their opinions as its domain. The domain of this function is larger as compared to the domain of a soft approximation function. It can manage a situation in which several expert opinions are taken into account by a single model. For the soft expert-argument approximate function with intuitionistic fuzzy setting, concepts such as set inclusion, -convexity(concave) sets, strongly
-convexity (concave) sets, strictly
-convexity (concave) sets, convex hull, and convex cone are conceived in this paper. Some set-theoretic inequalities are established with generalized properties and results on the basis of these specified notions. Additionally, by using a theoretic cum analytical approach, various elements of computational geometry, such as convex hull and convex cone, are theorized and some pertinent results are generalized.
1. Introduction
In 1999, Molodtsov [Citation1] used the idea of soft approximate function for constructing a novel model soft set in order to give fuzzy set like models [Citation2,Citation3] the parameterizations tool for dealing with uncertain data. This set makes use of the soft approximate function, which converts a single set of parameters into the starting universe's power set. There have been many discussions about the fundamentals of soft sets, but the contributions of Ali et al. [Citation4], Babitha and Sunil [Citation5,Citation6], Ge and Yang [Citation7], Li [Citation8], Maji et al. [Citation9], Pei and Miao [Citation10], and Sezgin and Atagun [Citation11] are thought to be particularly significant for characterizing the fundamental properties and set-theoretic operations of soft sets. Abbas et al. [Citation12] examined the idea of soft points and addressed its drawbacks, similarities, and difficulties. Zadeh [Citation13] introduced the concept of fuzzy set as a generalization of crisp set. In fuzzy set, each element has a membership degree. Xia [Citation14] applied fuzzy in multi-criteria decision-making problems and named as EFMCDM-method. He applied this technique to deal with the problem that uncertainty is inevitably present in the MCDM process owing to human subjectivity. Intuitionistic fuzzy sets have a stronger capacity to represent and address the ambiguity of information than previous generations of fuzzy sets. Despite the development of numerous measuring techniques, there are still certain issues with the poor axioms of distance measurement or that lack discernment and lead to counterintuitive circumstances. To solve the aforementioned problems, Xiao [Citation15] suggested a brand-new intuitionistic distance metric based on the Jensen-Shannon divergence. The Interval-Valued Intuitionistic Fuzzy Set has drawn a lot of interest because it is a powerful tool for modelling uncertainty. Using this structure, Wang et al. [Citation16] introduced the interval-valued intuitionistic fuzzy Jenson-Shannon (IVIFJS) divergence, a new distance of interval-valued intuitionistic fuzzy set that can assess the similarity or difference between inter-valued intuitionistic fuzzy sets. The Pythagorean fuzzy set is a useful tool for addressing uncertainty in real-world applications, but it is still unclear how to gauge its level of uncertainty. By considering Pythagorean fuzziness entropy in terms of membership and non-membership degrees as well as Pythagorean hesitation entropy in terms of hesitation degree, Wang et al. [Citation17] developed a novel entropy measure of Pythagorean fuzzy set. To address uncertainties with parameterizations tools, Maji et al. [Citation18] advanced bonding impression of fuzzy soft set and debated its crucial things and outcomes. Numerous researchers [Citation19,Citation20] discussed the properties of the soft set, such as subset, absolute set, not set, etc., AND, OR, etc., and applied them to real-world issues. Rahman et al. [Citation21] studies (m, n)convexity(concavity) using the structure of fuzzy soft set and discussed its certain properties. Many intriguing applications of soft set theory have been extended by certain researchers utilizing this idea of fuzzy soft sets. Fuzzy soft sets have some applications, according to Roy and Maji [Citation22]. On the basis of the theory of soft sets, Som [Citation23] defined soft relation and fuzzy soft relation. Working on intuitionistic fuzzy soft relations were Mukherjee and Chakraborty [Citation24]. Soft sets and the related ideas of fuzzy sets and rough sets were compared by Aktas and Çaǧman [Citation25]. Operations on fuzzy soft sets were established by Yang et al. [Citation26] and are based on the three fuzzy logic operators negation, triangular norm, and triangular co-norm. The soft set and fuzzy soft set were introduced into the incomplete environment by Zou and Xiao [Citation27]. For their combined forecasting strategy based on fuzzy soft sets, Xiao et al. [Citation28] employed predicting accuracy as the criterion of fuzzy membership function. The combination of an interval-valued fuzzy set and a soft set was introduced by Yang et al. [Citation29]. In fuzzy soft-sets, Kong et al. [Citation30] developed the typical parameter reduction and demonstrated that Roy and Maji's [Citation22] technique is not practical in most situations. Çaǧman and Kartaş[Citation31] introduced the concept of intuitionistic fuzzy soft set and successfully applied it in decision-making problems. Alkhazaleh et al. [Citation32] advanced the structure of soft expert set, a mingling of soft set and expert set. They categorized its fundamentals results and strongly put in decision-making problems. Alkhazaleh et al. [Citation33] instigated the model of fuzzy soft expert set by making a nice extension in soft expert set using fuzzy environment. They developed its operations and used in decision-making problems. He also introduced the concept of mappings on fuzzy soft expert set and described its properties. Broumi and Samarandache [Citation34] introduced the concept of intuitionistic fuzzy soft expert set and applied in multi-criteria decision-making problems by giving examples. They [Citation35] also introduced mappings on this structure and discussed certain theorems with examples. Smarandache [Citation36] made extension in soft set by creating a new structure called hypersoft set. He made this work by dividing the parameters into sub-parameters.
1.1. Research gap, motivation and novelty
Convexity is very convenient in unalike arenas like optimization, recognition and classification of certain patterns, dualism difficulties and numerous extra-linked issues in operation research. The soft expert set is the mingling of soft and expert set. It is more flexible as it manages the restrictions of soft set for the respect of adept's thoughts. In order to make the existing convexity-like literature adequate with that situation, it is a literary necessity to get hold of a basic context for resolving such sort of issues under more flexible setting, i.e. soft expert set. convexity is very useful to solve and understand the optimization problems. Classically, the main focus of linear programming was in optimization area. At the beginning, it was considered that problems were majorly classified into two categories linear and non-linear optimized problems. Later on researchers found that the right division was between convex and non-convex problems because the some non-linear problems were found difficult to the others. But the use of convexity in uncertain environment was a big challenge. Various convex fuzzy and concave fuzzy set definitions exist, however, Zadeh [Citation13] and Chaudhuri [Citation37] are credited with introducing the first convex fuzzy set definition. Concavo-convex fuzzy sets were suggested by Sarkar [Citation38] after Zadeh [Citation13], with some features. Additionally, research on convex (concave) fuzzy sets has advanced quickly in both theory and application. A few examples include [Citation39,Citation40]. First, Deli [Citation41] introduced the idea of convexity on soft set as well as fuzzy soft set. Deli proved some important results by using operations like union, intersection and compliment. He proved the following important results: (i)Intersection of convex soft sets is convex set but union is not necessarily a convex soft set. (ii) Union of concave soft sets is concave soft sets but intersection is not necessarily. (iii) The compliment of convex soft set is concave soft set and vice versa. (iv) Intersection of two strictly convex soft sets is convex but union is not necessarily. The same results have been proved for convex(concave) fuzzy soft sets. Later on, Majeed [Citation42] instigated the geometrical futures like convex hull and cone s-set environment showing the use of convexity in geometry. Salleh and Sabir [Citation43] talked about the certain features of convexity(concavity) on soft sets. Rahman et al. [Citation44,Citation45] introduced different structures of convexity(concavity) on soft and fuzzy soft sets. Ihsan et al. [Citation46,Citation47] conceptualized convexity on soft and fuzzy soft expert sets with certain properties. The research that is currently available on soft inclusions and inequalities for soft and fuzzy soft sets is only appropriate for managing soft expert argument approximate functions with intuitionistic fuzzy settings. In other words, it may be said that the literature now available on soft and fuzzy soft sets is unable to offer a mathematical model that might address all of the aforementioned real-world circumstances at once:
When evaluating alternatives with an unclear nature (entities in a universal set), fuzzy membership grades must be applied to each entity that corresponds to each parameter.
The situation where we can know the opinions of different experts in a single model without using any additional operations like union and intersection etc.
When data is of two-dimensional type, i.e. membership and non-memberships values (intuitionistic fuzzy).
Therefore, motivated by the aforementioned gap in the literature, this study aims to create a new structure intuitionistic fuzzy expert sets for convexity(concavity) that is more adaptable than existing models because it can handle their constraints and is useful for making accurate and unbiased decisions because it places a strong emphasis on parameters as well as experts and their multi-decisive opinions. Consequently, an algebraic procedure is adapted to progress a core support of -convexity(concavity),
-convexity(concavity) in
-sense and
-senses, convex hull, convex cone on intuitionistic fuzzy soft expert sets (IFSEs) as well as some essential results have been proved. Numerical cases of these structures on this model are described too. The paper's primary contributions are summarized as follows:
Under IFSEs's environment, the traditional concepts of
-convex (concave) set, strictly
-convex (concave) set, strongly
-convex (concave) set, convex hull, and convex cone are generalized with the right to a soft expert approximate function.
Set-theoretic inequalities are developed based on these set inclusions as well as other suggested notions when the concept of set inclusion for soft expert approximate function is defined.
In order to evaluate the uniqueness of the proposed study, a detailed comparison is done with relevant, already published research works.
The paper is organized as Section 3 describes the definition of -convexity and concavity and related theorems. Section 4 describes the definition of
-convexity and concavity in
and
-senses with related theorems. Section 5 formulates the concept of strictly convex(concavity). Convex hull and cone are added to Section 6. In Sections 7 and 8, respectively, the comparison and conclusion with regard to future guidelines have been reached.
2. Preliminaries
This part shows the basic definitions from the literature. In this part, set of parameters will be denoted by and
as a universe of discourse and set of experts is by
and
will be a set of opinions,
.
will be used as a power set.
Definition 2.1
[Citation13]
A set ”” is named as a fuzzy set shown by
with
and
shows the membership value of
.
Definition 2.2
[Citation1]
A soft set is a pair ( where
is defined by mapping
Definition 2.3
[Citation18]
A pair is will be a fs-set on
, with
and
is being used as a collection of fuzzy subsets of
,
.
Definition 2.4
[Citation32]
A pair is known as a soft expert set with
is a mapping
and
.
Definition 2.5
[Citation34]
A pair is called an IFSEs, with
is given by
while
is being used as a collection of intuitionistic fuzzy subsets of
and
.
Definition 2.6
[Citation34]
For two IFSEs and
on
, then
with
and
⊆
, for all
.
Definition 2.7
[Citation34]
Let be an IFSEs on
, then its complement
is characterized by
=
with
is a function and
=
for each o
, here c represents a intuitionistic fuzzy complement.
Definition 2.8
[Citation34]
Let and
be two IFSESs, then these are equal if
and
.
Definition 2.9
[Citation34]
The union between IFSESs and
is again an IFSEs
;
and ∀
while s is a s-norm.
Definition 2.10
[Citation34]
The intersection of IFSEs and
on
is an IFSEs
while
; for all
where t is a t-norm.
Definition 2.11
[Citation34]
Suppose be a finite set of IFSESs. Then
The operation union of finite collection can be characterized as
.
The operation intersection of finite collection can be characterized as
Definition 2.12
[Citation41]
The fuzzy soft set on is named as a convex fuzzy soft set if
for each
and
Definition 2.13
[Citation41]
The fuzzy soft set on is named as a convex fuzzy soft set if
for each
and
3. Convex and concave intuitionistic fuzzy soft expert set
This part of the paper describes the definitions of convex(concave) intuitionistic fuzzy soft expert set and various results have been proved.
Deli [Citation41] is the first person who introduced the concept of convexity(concavity) in s-set and f-set environment. But this work is not suitable when some experts opinions is required. To deal with situation, Ihsan et al. [Citation46] made extension in the work of Deli by introducing convexity in se-set. Then Ihsan et al. [Citation47] put forward this idea in fuzzy soft expert environment by conceptualizing convexity in fse-set. Fuzz soft set is useful for handling membership values but not for non-membership environment. In order to handle these limitations, idea of convexity(concavity) is extended to intuitionistic fuzzy soft environment under multi-decisive opinion. Some dominant and valuable characteristics have been extended.
Definition 3.1
The IFSEs on is called a convex IFSEs if
for each
and
Example 3.1
Assume that an organization delivers new sorts of items and needs to take the assessment of certain specialists about these items. Suppose is used as s set of products,
represents a collection of decision parameters where
are the parameters; easy to handle, quality and moderate. Let
represents an experts set. Suppose that
The IFSEs can be described as
Example 3.2
Using Example (3.1), consider and
Let
and
be two IFSESs such that
Using union operation(max), we get
Example 3.3
Referring to Example (3.1), let and
Considering the above two IFSESs in (3.2) and using intersection operation(min), we get
Example 3.4
Considering Example (3.1) with , and
,
Take α = 0.6, and ,
then
By applying the decimal round off property, we get
and
.
It is clear that
Ihsan et al. [Citation47] have defined concavity in fuzzy soft expert environment. Now following definition is the extension of fuzzy soft expert set.
Definition 3.2
The IFSEs on is called concave IFSEs if
for each
and
.
Example 3.5
Considering Example (3.1) with , and
,
,
Take α = 0.6, and ,
then
By applying the decimal round off property, we get
and
=
.
It is clear that
Set-inclusion has already been introduced in Ihsan et al. [Citation47] for fuzzy environment. Now this work is for its extension.
Definition 3.3
Suppose be an IFSEs on
and
. Then ρ-inclusion of
is characterized as
Theorem 3.1
is a
-convex IFSESs while
and
are
-convex IFSESs.
Proof.
Assume ∃ and
and
.
∵
and
are
-convex IFSESs, so
(1)
(1)
(2)
(2) By applying the intersection operation, we have
hence the result is proved.
Remark 3.1
If is any collection of
-convex IFSESs, then the
is a
-convex IFSEs.
Theorem 3.2
Y is a -convex IFSEs on
iff for each
and
,
is
-convex IFSEs on
.
Proof.
Suppose that Y is -convex IFSEs. If
and
, then
and
.
By the convexity of Y, we have
so
is
-convex IFSEs
.
Conversely, suppose that is
-convex IFSEs for each
.
Then, for ,
is
-convex IFSEs for ρ
.
and
, we have
and
, hence
.
⇒Y is -convex IFSEs.
Theorem 3.3
is
-concave IFSEs while L is
-convex IFSEs.
Proof.
Assume that ∃.
∵L is -convex IFSEs,
or
If
, then we may write
(3)
(3) If
, then we may write
(4)
(4) From Equations (Equation1
(1)
(1) ) and (Equation2
(2)
(2) ), we have
(5)
(5) So,
is
-concave IFSEs.
Theorem 3.4
is
-convex IFSEs while P is
-concave IFSEs.
Proof.
Assume that ∃.
∵P is -concave IFSEs,
or
If
then we may write
(6)
(6) If
(7)
(7) from (Equation4
(4)
(4) ) and (Equation5
(5)
(5) ), we have
(8)
(8) which shows that
is
-convex IFSEs.
Theorem 3.5
M is -concave IFSEs on
iff for each
and
,
is
-concave IFSEs on
.
Proof.
Suppose that M is -concave IFSEs. If
and
, then
and
.
By the concavity of M, we have
hence
is a
-concave IFSEs.
Conversely suppose that is
-concave IFSEs for each
.
Subsequently, for ,
is
-concave IFSEs for ρ
.
and
,
we have and
,
hence .
it is clear that M is -concave IFSEs.
Theorem 3.6
is a
-concave IFSEs while
and
are
-concave IFSESs.
Proof.
Assume ∃ and
and
.
∵
and
are
-concave ifse-sets, so
(9)
(9)
(10)
(10) By applying union operation, we have
Here we have proved the union of two concave IFSESs, it can be proved for more than two concave ifse-sets. Hence we can generalized this result up to any countable number of concave IFSESs.
4. 
-Convex and 
-Concave intuitionistic fuzzy soft expert sets in 
and 
-Sense
This portion will present the concept of -convex and
-concave IFSESs in
and
-sense with some proved results.
Rahman et al. [Citation44] have discussed (m; n) convexity with sense for a fuzzy environment and now an extension is made by introducing this work in an intuitionistic fuzzy environment.
Definition 4.1
An IFSEs is named as a -convex IFSEs in
-sense if
for
,
and
.
Example 4.1
Referring to Example (3.1) with , and
,
Take α = 0.6, v = 1, δ = 0.5 and ,
then
. By applying the decimal round off property, we get
and also
and
.
It is clear that
Rahman et al. [Citation44] have discussed (m; n) concavity with sense for fuzzy environment and now extension is made by introducing this work in intuitionistic fuzzy environment.
Definition 4.2
An IFSEs is named as a -concave IFSEs in
-sense if
for
,
and
.
Rahman et al. [Citation44] have discussed (m; n) convexity with sense for fuzzy environment and now extension is made by introducing this work in intuitionistic fuzzy environment.
Definition 4.3
An IFSEs is named as a -convex IFSEs in
-sense if
for
,
and
.
Example 4.2
Referring to Example (3.1), Referring to Example (3.1) with , and
,
,
Take α = 0.6, v = 1, δ = 0.5, and ,
then
=
=
=
By applying the decimal round off property, we get and also
and
=
.
It is clear that
Rahman et al. [Citation44] have discussed (m; n) concavity with sense for fuzzy environment and now extension is made by introducing this work in intuitionistic fuzzy environment.
Definition 4.4
An IFSEs is named as a -concave IFSEs in
-sense if
for
,
and
.
Theorem 4.1
is a
-convex IFSEs in
-sense while
and
are
-convex IFSESs in
-sense.
Proof.
Assume ∃ and
and
.
∵
and
are
- convex IFSESs in
-sense, so
(11)
(11)
(12)
(12) By applying the intersection operation, we get
(13)
(13)
hence the result is proved.
Remark 4.1
If is any collection of
-convex IFSESs in
-sense, then the
is a
-convex IFSEs in
-sense.
Theorem 4.2
is a
-convex IFSEs in
-sense while
and
are
-convex IFSESs in
-sense.
Proof.
Assume ∃ and
and
.
∵
and
are
-convex IFSESs in
-sense, so
(14)
(14)
(15)
(15) By applying the union operation, we get
(16)
(16) hence the result is proved.
Remark 4.2
If is any collection of
-convex IFSESs in
-sense sense, then the
is a
-convex IFSEs in
-sense sense.
Theorem 4.3
L is a -convex IFSEs in
-sense on
iff for each
and
,
is
-convex IFSEs in
-sense on
.
Proof.
Suppose that L is -convex IFSEs in
-sense. If
and
, then
and
.
By the convexity of D, we have
so
is
-convex IFSEs in
-sense.
Conversely, suppose that is
-convex IFSEs in
-sense for each
.
Then, for ,
is
-convex IFSEs in
-sense for ρ
.
∵ and
, we have
and
, hence
.
which implies that L is
-convex IFSEs in
-sense.
Theorem 4.4
L is a -convex IFSEs in
-sense on
iff for each
and
,
is
-convex IFSEs in
-sense on
.
Proof.
Suppose that L is -convex IFSEs in
-sense. If
and
, then
and
.
By the convexity of L, we have
so
is
-convex IFSEs in
-sense.
Conversely, suppose that is
-convex ifse-set in
-sense for each
.
Then, for ,
is
-convex ifse-set in
-sense.
For ρ .
∵ and
, we have
and
, hence
.
which implies that L is
-convex IFSEs in
-sense.
Theorem 4.5
is
-concave IFSEs in
-sense while O is
-convex IFSEs in
-sense.
Proof.
Assume that ∃.
∵O is -convex IFSEs in
-sense,
or
If
, then we may write
(17)
(17) If
, then we may write
(18)
(18) From Equations (Equation17
(17)
(17) ) and (Equation18
(18)
(18) ), we have
(19)
(19) So,
is
-concave IFSEs in
-sense.
Theorem 4.6
is
-concave IFSEs in
-sense while O is
-convex IFSEs in
-sense.
Proof.
Assume that ∃.
∵O is -convex IFSEs in
-sense,
or
If
, then we may write
(20)
(20) If
, then we may write
(21)
(21) From Equations (Equation20
(20)
(20) ) and (Equation21
(21)
(21) ), we have
(22)
(22) So,
is
-concave IFSEs in
-sense.
Theorem 4.7
is
-convex IFSEs in
-sense while
is
-concave IFSEs in
-sense.
Proof.
Assume that ∃.
∵ is
-concave IFSEs in
-sense,
or
If
then we may write
(23)
(23) If
(24)
(24) From (Equation23
(23)
(23) ) and (Equation24
(24)
(24) ), we have
(25)
(25) which shows that
is
-convex IFSEs in
-sense.
Theorem 4.8
is
-convex IFSEs in
-sense while
is
-concave IFSEs in
-sense.
Proof.
Assume that ∃.
∵ is
-concave IFSEs in
-sense,
or
If
then we may write
(26)
(26) If
(27)
(27) From (Equation26
(26)
(26) ) and (Equation27
(27)
(27) ), we have
(28)
(28) which shows that
is
-convex IFSEs in
-sense.
Theorem 4.9
is
-concave IFSEs in
-sense on
iff for each
and
,
is
-concave IFSEs in
-sense on
.
Proof.
Suppose that is
-concave IFSEs in
-sense. If
and
, then
and
.
By the -concavity of
in
-sense, we have
hence
is a
-concave IFSEs in
-sense.
Conversely, suppose that is
-concave IFSEs in
-sense for each
.
Subsequently, for ,
is
-concave IFSEs in
-sense for ρ
.
and
, we have
and
, hence
it is clear that
is
-concave IFSEs in
-sense.
Theorem 4.10
is
-concave IFSEs in
-sense on
iff for each
and
,
is
-concave IFSEs in
-sense on
.
Proof.
Suppose that is
-concave IFSEs in
-sense. If
and
, then
and
.
By the -concavity of
in
-sense, we have
hence
is a
-concave IFSEs in
-sense.
Conversely suppose that is
-concave IFSEs in
-sense for each
.
Subsequently, for ,
is
-concave IFSEs in
-sense for ρ
.
and
, we have
and
, hence
,
it is clear that
is
-concave IFSEs in
- sense.
Theorem 4.11
is a
-concave IFSEs in
-sense while
and
are
-concave IFSEss in
-sense.
Proof.
Assume ∃ and
and
.
∵
and
are
-concave IFSEss in
-sense, so
(29)
(29)
(30)
(30) By applying the union operation, we get
(31)
(31)
Here we have proved the union of two concave IFSEss in -sense, it can be proved for more than two concave IFSEss in
-sense. Hence we can generalized this result up to any countable number of concave IFSEss in
-sense.
5. Strongly and strictly convex (concave)
This section contains definitions of strongly convex IFSEs, strictly convex IFSEs, strongly concave IFSEs, strictly concave IFSEs and describes their characteristics.
Salleh and Sabir [Citation43] conceptualized some characteristics of convexity(concavity) like strictly and strongly in s-set environment. Now these properties (Definitions 5.1, 5.2, 5.3, 5.4) have been generalized for intuitionistic fuzzy environment.
Definition 5.1
The IFSEs over are called strongly convex IFSEs if
for each
,
and
=(0, 1).
Definition 5.2
The IFSEs over are called strongly concave IFSEs if
for each
,
and
.
Definition 5.3
The IFSEs over are called strictly convex IFSEs if
for each
,
and
.
Definition 5.4
The IFSEs on are said to be strictly concave IFSEs if
for each
,
and
.
Theorem 5.1
Suppose be a strictly convex IFSEs. If ∃α ∈
∀
,
such that
then
is a convex IFSEs.
Proof.
Assume that and ∃
,
(32)
(32) if
, then (30) contradicts that
is a strictly convex IFSEs.
Now if we take and
, suppose
and
So
⇒
(33)
(33) now
(34)
(34) From (Equation32
(32)
(32) ), (Equation33
(33)
(33) ) and
, we have
(35)
(35) also from (Equation31
(31)
(31) ), (Equation32
(32)
(32) )
and strictly convex IFSEs condition, we have
(36)
(36) or
(37)
(37) thus (Equation34
(34)
(34) ) and (Equation36
(36)
(36) ) contradict the fact.
If and
, suppose
then
(38)
(38) From (Equation32
(32)
(32) ), (Equation37
(37)
(37) ) and
, we have
(39)
(39) Also,
becomes
(40)
(40) now from (Equation32
(32)
(32) ), (Equation39
(39)
(39) )
and strictly convex IFSEs condition, we have
(41)
(41) Hence (Equation39
(39)
(39) ) and (Equation40
(40)
(40) ) contradict the fact.
Theorem 5.2
Suppose be a strictly concave IFSEs. If ∃α ∈
∀
,
such that
then
is a concave IFSEs.
Proof.
Assume that and ∃
,
(42)
(42) if
, then (Equation42
(42)
(42) ) contradicts that
is a strictly concave IFSEs.
now if we take and
, suppose
and
. so
⇒
(43)
(43) now
(44)
(44) From (Equation41
(41)
(41) ), (Equation43
(43)
(43) ) and
, we have
(45)
(45) now from (Equation42
(42)
(42) ), (Equation44
(44)
(44) )
and strictly concave se-set condition, we have
(46)
(46) or
(47)
(47) thus (Equation46
(46)
(46) ) and (Equation47
(47)
(47) ) contradicts the fact.
If and
, suppose
then
(48)
(48) From (Equation42
(42)
(42) ), (Equation48
(48)
(48) ) and
, we have
(49)
(49) Also,
becomes
(50)
(50) Now from (Equation42
(42)
(42) ), (Equation49
(49)
(49) ),
and strictly concave IFSEs condition, we have
(51)
(51) Hence (Equation49
(49)
(49) ) and (Equation5
(5)
(5) ) contradict the fact.
Theorem 5.3
Suppose be a convex IFSEs. If ∃α ∈
∀
,
with
then
is a strictly convex IFSEs.
Proof.
Assume such that
(52)
(52) If
, then above equation becomes
(53)
(53) But by the convexity condition, we have
(54)
(54) By Equations (Equation52
(52)
(52) ) and (Equation53
(53)
(53) ), we get
(55)
(55) Continuing with
, we get
(56)
(56) or
(57)
(57) By supposition and (Equation56
(56)
(56) ), we have
(58)
(58) We generalize it for taking
(59)
(59) Suppose
with
for any value of n.
Then we see from above equation
(60)
(60) Also, suppose
with
for any n. we have
(61)
(61) Also when,
, subsequently by using the convexity of IFSEs and Equation (Equation61
(61)
(61) ) we have
which contradicts Equation (Equation59
(59)
(59) ).
Now when , subsequently by using the supposition of theorem and Equation (Equation53
(53)
(53) ), we have
which contradicts Equation (Equation61
(61)
(61) ).
Corollary 5.1
Suppose be a concave IFSEs. If ∃α ∈
∀
,
with
then
is a strictly concave IFSEs.
Proof.
This is simple by taking the complement of the above equations.
Theorem 5.4
Suppose be a strongly convex soft fuzzy expert set. If ∃α ∈
∀
,
with
(62)
(62) then
is a convex IFSEs.
Proof.
Assume such that
(63)
(63) If
then Equation (Equation62
(62)
(62) ) contradicts the fact that
is a convex IFSEs.
If , select
with
.
Suppose ,
Equation (Equation62(62)
(62) ) becomes
(64)
(64)
(65)
(65) By Equations (Equation62
(62)
(62) ), (Equation63
(63)
(63) ) and (Equation64
(64)
(64) ), we have
And this contradicts that
is a strongly convex IFSEs.
Corollary 5.2
Suppose be a strongly concave IFSEs. If ∃α ∈
∀
,
with
(66)
(66) then
is a concave IFSEs.
Proof.
This can be done by using the proof of the above Theorem 5.4. In Theorem 5.4, definition of strongly convex IFSEs has been used and to prove this corollary just make use of strongly concave IFSEs.
Theorem 5.5
Suppose be a convex IFSEs. If ∃α ∈
∀
,
such that
then
then
is a strongly convex IFSEs.
Proof.
Assume ∃ ()
such that
(67)
(67) By the condition of convex IFSEs and from above equation, we have
(68)
(68) Moreover, it can be written as
(69)
(69) While
,
with selecting
Also by the definition of convexity of IFSEs and above defining , we get
(70)
(70)
(71)
(71) Using Equations (Equation68
(68)
(68) ), (Equation69
(69)
(69) ), (Equation70
(70)
(70) ) and given statement, we have
which contradicts to Equation (Equation67
(67)
(67) ).
Corollary 5.3
Suppose be a concave IFSEs. If ∃α ∈
∀
,
such that
then
then
is a strongly concave IFSEs.
Proof.
This can be done by using the proof of the above Theorem 5.5. In Theorem 5.5, definition of strongly convex IFSEs with certain conditions has been used and to prove this corollary just make use of strongly concave IFSEs with the same certain conditions.
6. Convex hull and convex cone
In this section, a new concept of convex hull and cone for IFSEs and
has been developed with the help of existing concept of convex hull and cone on s-set by Majeed [Citation42]. The notation X(Z) will be used for collection of IFSEss.
Definition 6.1
The convex hull of an IFSEs can be defined as
=
∈ X(Z) are IFSEs
.
Theorem 6.1
Let then its convex hull is given by as
where
Proof.
Using definition of convex hull of , we have
=
such that
. Now define the IFSEs as
now we have to show that
. To prove this, we will show
As we know that every IFSEs is a convex IFSEs. Then by using the definition of convex IFSEs, we have
Using the intersection to the left side of the above relation, we get
Now we will prove that
. It is enough to show that
is a convex IFSEs. Actually, since
(C, S) is the smallest convex IFSEs containing (C, S),
. Suppose
and
such that
and
, with
=1 and
=1. Therefore,
such that
and
As
, so
. Using the definition of IFSEs described above to
and to x =
, we have
i.e.
hence the required result is proved.
Definition 6.2
Let be IFSEs, then it is called a cone if
for all
and
.
If the IFSEs is convex, then it is named as a convex cone.
Theorem 6.2
A IFSEs is said to be convex cone iff for every
S and
.
Proof.
Suppose be an IFSEs, then by definition statement (1) is proved.
Now to prove 2nd statement take and for any
, then by definition of convex cone
and
From the above equations, we have
hence the 2nd result is proved. Conversely, we have to show that
is convex IFSEs.
By first condition is cone. By making use of 1st and 2nd conditions with
, it is clear that
is convex IFSEs.
i.e.
Which shows that
is convex IFSEs.
Corollary 6.1
If is IFSEs then it is a convex cone iff
for all
and
.
7. Comparison
A comparison analysis of the model has been shown in Table . In this table, some prominent characteristics of existing models have been compared with proposed structure. These characteristics include membership function , non-membership (
), single argument approximate function
and multi-decisive opinion
. The Yes and No will be denoted by
and
in the following Table . From Table , it is clear that our proposed model is more generalized than the above described models.
Table 1. Comparison with different models.
8. Conclusions
In this article, -convexity cum concavity,
-convexity cum concavity in
-sense and
-sense, convex hull and convex cone are developed for IFSEs. Several classical axiomatic properties and operational results have been generalized under IFSEs. Although the proposed framework is an intelligent approach for convex optimization and parameterization with entitlement of fuzzy membership grades and intuitionistic fuzzy non-membership grades yet it has limitations regarding the consideration of neutral grades; therefore, the future work includes the extension of this study for such environments to address its limitations. Moreover, the generalization of various other variants of classical convexity may also a part of its future work.
Disclosure statement
No potential conflict of interest was reported by the author(s).
References
- Molodtsov D. Soft set theory-first results. Comput Math Appl. 1999;37(4–5):19–31. DOI:10.1016/s0898-1221(99)00056-5.
- Atanassov KT. Intuitionistic fuzzy sets. Fuzzy Sets Syst. 1986;20(1):87–96.
- Smarandache F. Neutrosophy: neutrosophic probability, set, and logic: analytic synthesis and synthetic analysis. Rehoboth (DE): American Research Press; 1998.
- Ali MI, Feng F, Liu X, et al. On some new operations in soft set theory. Comput Math Appl. 2009;57(9):1547–1553.
- Babitha KV, Sunil J. Soft set relations and functions. Comput Math Appl. 2010;60(7):1840–1849.
- Babitha KV, Sunil J. Transitive closures and orderings on soft sets. Comput Math Appl. 2011;62(5):2235–2239.
- Ge X, Yang S. Investigations on some operations of soft sets. World Acad Eng Technol. 2011;75:1113–1116.
- Li F. Notes on soft set operations. ARPN J Syst Softw. 2011;1(6):205–208.
- Maji PK, Biswas R, Roy AR. Soft set theory. Comput Math Appl. 2003;45(4–5):555–562.
- Pei D, Miao D. From soft set to information system. In: Proceedings of the International Conference of Granular Computing; Beijing: IEEE; July 2005. p. 617–621.
- Sezgi A, Atagün AO. On operations of soft sets. Comput Math Appl. 2011;61(5):1457–1467.
- Abbas M, Guo Y, Murtaza G. A survey on different definitions of soft points: limitations, comparisons and challenges. J Fuzzy Ext Appl. 2021;2(4):334–343.
- Zadeh LA. Fuzzy sets. Inf Control. 1965;8(3):338–353.
- Xiao F. EFMCDM: evidential fuzzy multicriteria decision making based on belief entropy. IEEE Trans Fuzzy Syst. 2019;28(7):1477–1491.
- Xiao F. A distance measure for intuitionistic fuzzy sets and its application to pattern classification problems. IEEE Trans Syst Man Cybern Syst. 2019;51(6):3980–3992.
- Wang Z, Xiao F, Ding W. Interval-valued intuitionistic fuzzy Jenson-Shannon divergence and its application in multi-attribute decision making. Appl Intell. 2022;52:1–17.
- Wang Z, Xiao F, Cao Z. Uncertainty measurements for Pythagorean fuzzy set and their applications in multiple-criteria decision making. Soft Comput. 2022;26(19):9937–9952.
- Maji PK. Fuzzy soft sets. J Fuzzy Math. 2001;9(3):589–602.
- Maji PK, Biswas R, Roy AR. Soft set theory. Comput Math Appl. 2003;45(4–5):555–562.
- Pei D, Miao D, From soft set to information system. In: International conference of granular computing; IEEE. 2005. Vol. 2, p. 617–621.
- Rahman AU, Saeed M, Ahmad MR. (m; n)-Convexity-cum-Concavity on fuzzy soft set with applications in first and second sense. Punjab Univ J Math. 2021;53(1):19–33.
- Roy AR, Maji PK. A fuzzy soft set theoretic approach to decision making problems. J Comput Appl Math. 2007;203:412–418.
- Zhang Yh, Yuan Xh. Soft relation and Fuzzy soft relation. In: Cao BY, Nasseri H, editors. Information & Engineering and Operations Research & Management. Advances in Intelligent Systems and Computing, Vol. 211. Berlin: Springer. doi:10.1007/978-3-642-38667-1_21.
- Mukherjee A, Chakraborty SB. On intuitionistic fuzzy soft relations. Bull Kerala Math Assoc. 2008;5(1):35–42.
- Aktas H, Çaǧman N. Soft sets and soft groups. Inf Sci. 2007;177:2726–2735.
- Yang X, Yu D, Yang J, et al. Generalization of soft set theory: from crisp to fuzzy case, In: Bing-Yuan Cao, editor, Fuzzy Information and Engineering: Proceedings of ICFIE2007; Berlin: Springer; 2007. p. 345–355. (Advances in Soft Computing; 40). doi:10.1007/978-3-540-71441-5_39.
- Zou Y, Xiao Z. Data analysis approaches of soft sets under incomplete information. Knowl Base Syst. 2008;21:941–945.
- Xiao Z, Gong K, Zou Y. A combined forecasting approach based on fuzzy soft sets. J Comput Appl Math. 2009;228:326–333.
- Yang X, Lin TY, Yang J, et al. Combination of interval-valued fuzzy set and soft set. Comput Math Appl. 2009;58:521–527.
- Kong Z, Gao L, Wang L, et al. The normal parameter reduction of soft sets and its algorithm. Comput Math Appl. 2008;56:3029–3037.
- Çaǧman N, Karataş S. Intuitionistic fuzzy soft set theory and its decision making. J Intell Fuzzy Syst. 2013;24(4):829–836.
- Alkhazaleh S, Salleh AR. Soft expert sets. Adv Decis Sci. 2011;1–12:757868. DOI:10.1155/2011/757868 .
- Alkhazaleh S, Salleh AR. Fuzzy soft expert set and its application. Appl Math (Irvine). 2014;5(9):1349–1368. DOI:10.4236/am.2014.59127 .
- Broumi S, Smarandache F. Intuitionistic fuzzy soft expert sets and its application in decision making. J New Theor. 2015;1:89–105.
- Broumi S, Smarandache F. Mapping on intuitionistic fuzzy soft expert sets. J New Results Sci. 2015;4(9):1–10.
- Smarandache F. Extension of soft set to hypersoft set, and then to plithogenic hypersoft set. Neutrosophic Sets Syst. 2018;22(1):168–170.
- Chaudhuri BB. Concave fuzzy set: a concept complementary to the convex fuzzy set. Pattern Recognit Lett. 1992;13(2):103–106.
- Sarkar D. Concavoconvex fuzzy set. Fuzzy Sets Syst. 1996;79(2):267–269.
- Ammar EE. Some properties of convex fuzzy sets and convex fuzzy cones. Fuzzy Sets Syst. 1999;106(3):381–386.
- Yang X. Some properties of convex fuzzy sets. Fuzzy Sets Syst. 1995;72(1):129–132.
- Irfan D. Convex and concave sets based on soft sets and fuzzy soft sets. J New Theor. 2019;29:101–110.
- Majeed SN. Some notions on convex soft sets. Ann Fuzzy Math Inform. 2016;12(4):517–526.
- Salih HM, Sabir OP. Some new properties of convex and concave soft sets. Diyala J Pure Sci. 2019;15(1):87–98. DOI:10.24237/djps.1501.471b .
- Rahman AU, Saeed M, Arshad M, et al. (m; n)-Convexity-cum-Concavity on fuzzy soft set with applications in first and second sense. Pac Univ J Math. 2021;53(1):19–33.
- Rahman AU, Saeed M, Arshad M, et al. A conceptual framework of m-Convex and m-Concave sets under soft set environment with properties. Trans Math Comput Sci. 2021;1(1):40–60.
- Ihsan M, Saeed M, Rahman AU. A rudimentary approach to develop context for convexity cum concavity on soft expert set with some generalized results. Punjab Univ J Math. 2021;53(9):621–629.
- Ihsan M, Rahman AU, Saeed M, et al. Convexity-cum-concavity on fuzzy soft expert set with certain properties. J Intell Fuzzy Log Intell Syst. 2021;21(3):233–242.