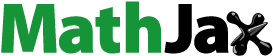
Abstract
This paper presents the strength and micro-structural characteristics of high plasticity expansive clay stabilized with ordinary Portland cement (OPC) and geopolymers. Furthermore, sustainability aspects such as cost-efficiency, energy consumption and eco-efficiency of the OPC and geopolymer-stabilized soils were compared. The experimental results revealed that the geopolymer-stabilized soils exhibit higher strength and sustainability performance than the OPC-stabilized soils. This study also explores the developed machine learning prediction models of the geopolymer soils’ unconfined compressive strength (UCS) based on experimental data from current research and previous literature. Linear regression (LR), K-nearest neighbour (KNN), random forest (RF), random forest with random search hyperparameter optimization (BRRF) and random forest with grid search hyperparameter optimization (BGRF) were used as part of ensemble algorithms to predict stabilized soils UCS. Eight parameters such as liquid limit (LL), plasticity index (PI), ground granulated blast furnace slag (S) content, fly ash (FA) content, the molarity of NaOH (M), activator to binder ratio (A/B), Na/Al and Si/Al were used as input to predict UCS of geopolymer soils. The following metrics were used to assess the models’ predictive ability for compressive strength: coefficient of determination (R2), root mean square error (RMSE), mean absolute error (MAE) and mean square error (MSE). The BRRF model has a good potential to predict the UCS of geopolymer soils, according to the findings of its testing (MAE = 0.27, MSE = 0.21, RMSE = 0.46, R2 = 0.99) and training (MAE = 0.78, MSE = 1.48, RMSE = 1.23, R2 = 0.96) phases. According to the RF model's feature importance study, slag content and liquid limit were found to influence forecasting compressive strength, while fly ash content has the least influence.
1. Introduction
Soil is used as a construction material widely across the globe for various purposes; some soils are having very poor properties in nature which should be engineered before utilization. Expansive clays are proved to be extremely problematic due to their nature of exhibiting high level of volumetric variations according to changes in the water content [Citation1,Citation2]. When they absorb water, they expand or gain volume; when water is removed, they contract or lose volume [Citation3]. Therefore, in field clayey soil layers, swelling and shrinkage alternately occur in rainy and summer seasons. Therefore, civil engineering projects erected on expansive clays suffer severe cracking that causes significant financial loss [Citation4]. The impact of expansive soils on civil engineering infrastructures has resulted in significant financial losses globally, amounting to millions of dollars in damages [Citation4]. Therefore, the economic harm brought on by expanding soils is equivalent to the combined harm brought on by many or all-natural disasters.
Various foundations capable of handling expansive soils are also developed by researchers. Physical modification techniques include sand cushions, cohesive non-swelling layer (CNS) techniques and pile-based techniques are a few of them [Citation5]. To reduce heave, the top few inches of the expansive soil profile are swapped out for a non-expanding sand mattress and CNS layers [Citation5,Citation6]. Also, constructing pile foundation over soft soil is cumbersome, solutions are studied for this problem too. The lowest portions of piles used in tension-resistant foundation systems must be secured in the unresponsive or passive zone of the soft soils stratum to prevent swelling [Citation7]. Another efficient method for improving the performance of expansive soils is chemical stabilization [Citation8]. It involves modifying the swelling qualities of expansive soils by mixing them with chemical reagents such as cement, hydrated lime (CaOH2), calcium chloride (CaCl2), fly ash, powdered granulated blast furnace slag and rice husk ash [Citation8–10]. Lime provides the most efficient pozzolanic nature which helps to reduce expansive soils’ swelling characteristics. Additionally, it has been discovered that lime makes expansive clays stronger [Citation11]. The CaCl2 is hydrophilic in nature and deliquescent [Citation12]. When clay soil blended with CaCl2, it draws moisture from the air while keeping it moist, minimizing shrinkage fractures [Citation10]. Therefore, it has been found that impregnation of CaCl2 can increase expansive clays’ strength. Concerned with sustainable approaches usage of materials that are non-sustainable should be avoided. Hence, most of the geotechnical activities call for conventional treatment, but they may accidentally affect the environment. For example, continuous raw material exploitation and cement manufacture cause short material depletion and increase the greenhouse effect by releasing too much CO2 into the environment [Citation13]. Finding unique and alternative stabilizing ingredients to lime and cement that contain sustainable elements is therefore crucial [Citation14]. Cement production is proved to be perilous in terms of environmental protection, emanating high amount of CO2 due to its production process [Citation15]. Methods to avoid cement usage by using an alternate method are also studied widely, one such method is the usage of geopolymers, which is analysed for the last few decades.
The term “geopolymer” has been used frequently in the civil engineering industry to refer to various inorganic alkali activators [Citation16]. In many civil engineering products, it served as a common cementing material. Due to their strength, chemical and thermal endurance qualities, the materials formed from those alkali-activated phases have shown to be ideal construction materials [Citation17]. Similar to how polymeric materials are made, geopolymers are substances made by a polymerization reaction [Citation18]. However, contrary to polymers, geopolymers are inorganic in nature due to the material used in its production, as a result of which it is called as inorganic polymer.
Soil strength, particularly shear strength and compressive strength is important to design engineering structures that may rest upon it. Understanding the engineering properties of chemically modified soils is typically a difficult, expensive, time-consuming and energy-intensive operation requiring much effort, equipment and time [Citation19]. For instance, at least four to six tests must be conducted to acquire valid data for estimating the compaction properties and the unconfined compressive strength (UCS) of soils. Also, soil strength should be analysed for longer curing period (7, 14, 28 days) and this adds more complexity in analysing the information in a shorter period. In order to accurately predict the compaction characteristics, UCS and other soil variables, machine learning based prediction models can be employed.
Many studies were done using fundamental machine learning models including regression and classification algorithms to understand the nature of soil. Using linear and non-linear regression techniques, conventional prediction methods for the UCS of stabilized soils are based on empirically obtained relationships from important statistical parameters [Citation20,Citation21]. These methods employ equations with various unknown coefficients that may affect how variables in the model are mapped [Citation21]. Despite being successful in some stabilized soil models, the prediction results are fundamentally flawed, primarily due to their complexity. Also, artificial intelligence and machine learning techniques proved to be the best options for creating creative solutions that can handle new issues due to large data generation and mining [Citation22]. They are employed to develop models suitable for geotechnical investigation too. Soleimani et al. [Citation23] used genetic programming to estimate the UCS of clayey soil treated with geopolymer. Multiple characteristics impacting the soil UCS were incorporated into the model, including additive percentages, plastic limit, plasticity index and others. Various investigations showed that the UCS evaluation equations produced good accuracy. Similarly, Mozumder et al. [Citation24] used the support vector regressor to forecast the shear strength of clayey soil stabilized using geopolymers. Hence, it is evident that machine learning models can be effectively utilized in the prediction complex soil properties using a simple experimental process that will avoid the cumbersome calculation process.
This research work’s primary focus is to develop a geopolymer binder for stabilizing swelling soil and to ascertain its impact. Also, authors developed machine learning based models to predict the complex engineering properties of geopolymer-stabilized soil. By examining and contrasting the prediction capabilities of linear regression, K-nearest neighbours (KNN), RF, best random forest (BRF) and best grid random forest (BGRF), this study adds to the corpus of knowledge. Furthermore, comparing machine learning techniques is proved to be crucial for developing an efficient prediction model that can be applied to real-world UCS of geopolymer soil scenarios.
2. Geopolymers and factors influencing geopolymerization
Geopolymer is a material that is inorganic, ceramic, aluminosilicate which forms long-range, covalent bond, amorphous networks. It is generally formed when alkali activators are added in powder form with silicate-based binders to create a solid aluminosilicate substance. Numerous scholars have generally referred to alkali-bound earthenware, hydro earthenware, soil cement and inorganic polymers as “Geopolymers” [Citation25,Citation26]. To increase the use of geopolymer as a polymer blend material for various industrial applications, the geopolymerization process was initially examined in 1978 [Citation25]. The typical geopolymer structure was described as a collection of interconnecting structures and chains of different minerals joined together by the influence of covalent bonds [Citation27].
Understanding the phases involved in the geopolymer creation process is crucial before understanding the setting process. The formation of a geopolymer gel matrix can happen in any media which requires changing the pace of the reaction [Citation18,Citation28]. Alkali silicate or hydroxide ions were commonly used in combination to activate silica and alumina binders having high pH concentrations, leading to the formation of the geopolymer [Citation29]. Geopolymer's chemical reaction was mostly determined using polysiliates and aluminosilicates (see Figure ). AlO4 and SiO4 are connected in the molecular network of siliates by swapping oxygen atoms, and Al3+ ions react with negative ions to form frame tetrahedra links [Citation30]. AlO4 and SiO4 are connected in the molecular structure of siliates by swapping oxygen atoms, and Al3+ ions react with negative ions to form frame tetrahedra links [Citation30,Citation31]. Polysiliates show the interaction of Al3+ and Si4+ substances with the interchange of oxygen, resulting in the polymer being formed in the structure of a ring and chain, either semi-crystalline or amorphous. However, industrial applications for polymer framers are not very common now. Other hand, ferrosialates might develop during the alkaline results in the activation of geopolymers [Citation32]. Three different kinds of iron may be discovered in the ferrosalate chain: Fe3O4 oxide, which is bound to the chain by secondary bonds, and iron which is imprisoned in the main chain or regulating load in interstitial locations. Geopolymer networks can also substitute [Si(OH)4]−. The amount of calcium oxide content typically helps to categorize the reaction mechanisms of alkali-activated materials [Citation33]. Low calcium precursors, often referred to as geopolymers, are thought to be predominantly composed of aluminium silicate. At the same time, calcium-rich substances show an alkali-activated reaction resembling that of Portland cement but instead produce calcium alumina silica hydrates (CASH) gels called tobermorite [Citation34].
For a certain soils, a specific type of additive is preferred. The decision is based on the calcium concentration of the specific class of binding substances known as precursors. Most raw materials undergo either low or high reactivity depending on the amount of calcium present. In addition, based on the curing period and gel reactivity, mineral components like alumina and silica, also present in precursor materials, react with one another to assemble various binder compounds [Citation35,Citation36]. The typical precursor aluminosilicate materials used in research works include fly ash, metakaolin, rice husk ash, bagasse ash and ground blast furnace slag [Citation37]. At room temperature, they are combined with the alkaline activator to begin the chemical production of the geopolymer [Citation38]. The main factors that influence the alkali-activated cement's ability to combine with chloride are the physical and chemical characteristics of the aluminosilicate precursor, the alkali-activator solution and the curing environment [Citation39–41]. The activator's amount and nature are crucial factors in the rheology of a geopolymer mixture that influence the structure and properties of a hardened geopolymer material [Citation42]. According to Hardjito et al. [Citation43], the source materials based on silicon and aluminium are required to create highly alkaline geopolymers because the chemical properties of geopolymers are more like those of zeolite with an amorphous morphology. Then, the alkaline solution is added to the geopolymer mixture, dissolving the silica and alumina's precursors while commencing the hydrolysis process on the surface of the precursor particles and the reaction between particles and silicate substances [Citation44]. Moreover, excess moisture acts as a lubricant between soil particles, lowering frictional forces and reducing interlocking, making the soil more susceptible to shear deformation [Citation40,Citation41].
3. Dataset preparation and methods
3.1. Materials
For testing highly plastic clayey soil was gathered from Bhimavaram, India (coordinates: 16.544893, 81.521240). The soil was oven-dried after which gradation studies were conducted as per IS 2720: Part 4. According to the soil's particle size analysis, clay, silt and sand found in the soil is found to be 86%, 9% and 5%, respectively. Table provides the soil characteristics of the clayey soil used in the study and soils from the literature.
Table 1. Soil properties.
Fly ash and ground granulated blast furnace slag are utilized as precursors in the manufacture of geopolymers. The national thermal power plant (NTPC), located in Vijayawada, India, provided FA (Class C). GGBS was procured from the local marketplace which is obtained in commercial grade. Alkali solution was made using sodium hydroxide (NaOH) for ionic dissolution from precursors. Empire Scientific Company in Vijayawada supplied sodium silicate (Na2SiO3) gel and NaOH pallets with 98% purity are used in this work. Twenty-four hours before usage, an alkali-activator solution with a molarity of 4 was prepared to ensure thorough flakes dissolving and heat of hydration dissipation. The alkali-activator to the binder was kept at 0.45 which is recommended by other researchers too. OPC of 53 grade (Indian standard) which was purchased in the local market is used as an additional binder. It has a 3.15 specific gravity and confirming to IS 12269:2013 norms.
Figure shows the scanning electron microscopy (SEM) images of the FA and S which shows the spherical shape confirming the regularly used materials.
3.2. Compaction characteristics
Compaction characteristics of the virgin and cement blended soil with varying cement proportion of 4%, 8%, 12%, 16% and 20% are studied in an air-dried soil as per ASTM 2000, D698a. Similarly, geopolymer soil blends were prepared with an alkali-activator instead of water to determine optimum activator content (OAC) and maximum dry density (MDD). Dry soil passing through 4.75 mm IS sieve was mixed homogeneously with additive (e.g. fly ash). Furthermore, one day prior prepared alkali-activator (maintaining NaOH of 4M, Na2SiO3/NaOH as 1) mixed with the fly ash blended soil (maintaining the alkali-activator to the binder as 0.45). Based on earlier research, it was found that the alkali-activator to binder ratio for efficient geopolymer synthesis was estimated to be between 0.3 and 0.6. To achieve the desired workability, homogenous compaction, eliminate air spaces and achieve the highest dry density, additional water was added proportionately because the alkali-activator was relatively restricted. To reach the maximum dry density, extra water is needed with an alkali-activator; combined water content is known as OAC [Citation44].
3.3. Unconfined compressive strength
Soil samples were prepared using PVC tubes of 40 mm diameter and 80 mm height, the soil is mixed with OMC to produce better strength. Care is taken to ensure that the water is mixed properly with the water before casting and curing. Soil samples were prepared for virgin soil and geopolymer-stabilized soil. The alkali-activator was utilized in the case of geopolymer instead of water. Following the settling phase, the homogenous mixture was transferred to PVC slip moulds and statically compacted with a hydraulic press to reach maximum dry density which is verified with mass to weight ratio to obtain the required density. The compacted specimens were demoulded and covered in plastic films to prevent moisture loss during curing which is done in ambient temperature in the moisture-controlled environment with 95% humidity. The UCS test for soil samples stabilized with geopolymer was carried out under ASTM D1633 using a uniaxial machine with a loading power of 50 kN. Figure shows the prepared samples and UCS testing in the laboratory.
3.4. Scanning electron microscopy
SEM analysis was performed to understand the micro-structural development of the geopolymer blended soils. After 28 days of geopolymerization, geopolymer-blended soil SEM micrographs were captured using the FESEM Gemini 300 instrument India.
3.5. Data curation for predictive modelling
In addition to strength studies on geopolymer-stabilized soil, this work also aims to develop a machine learning model to predict the strength. This is done with a dataset of 286 geopolymer soil mixtures with fly ash and GGBS. Some data on strength properties are also obtained from the work done by other researchers which is used for testing and validation [Citation45].
To gather the data for the machine learning model preparation, four clayey soil types were examined by collecting soil from Bhimavaram, India, and Silchar at a depth of 2 m. According to the Unified Soil Classification System (USCS), soils are categorized as CH, CH, CH and CL, with corresponding plasticity indices of 30%, 89%, 57% and 14%, respectively. An alkali-activator is added in tandem with varying proportions of fly ash and granulated blast furnace slag to the oven-dried soils. To further understand the strength development, the ratio of the alkali-activator to the binder, as well as the molarity of the alkali solution is also varied. Mozumder and Laskar [Citation45] published comprehensive details on the characteristics of blended geopolymer soils. A model was developed in that study which provided comprehensive information on predicting UCS with input parameters such as LL, PI, S%, FA%, M, A/B, Na/Al and Si/Al. Relation between the attributes of a dataset can be understood well by finding correlation between them. The correlation is calculated using Equation (1):
(1)
(1) where a and b correspond to two different features.
Figure displays the heat map and specifics of the correlation matrix as a change in the magnitude of the variables relative to one another. Eight input parameters such as LL, PI, S%, FA%, M, A/B, Na/Al and Si/Al, were employed to forecast the UCS of geopolymer blended soils. S (%) indicated a significant positive connection (+0.79) with the values of UCS, while FA (%) displayed a strong negative correlation of 0.31. Molarity, the ratio of the alkali-activator to the binder, and Na/Al showed a positive connection with UCS values of 0.16, 0.06 and 0.27, respectively.
For predicting UCS value, authors had employed five machine learning models such as linear regression, KNN, random forest, BRF and BGFR which are widely employed for prediction [Citation46,Citation47]. Concerned with geotechnical investigation linear regression, KNN and random forest are most widely employed but still there are unexplored areas including usage of BRF and BGRF.
3.6. Linear regression
It is a popular statistical technique which is employed widely and gaining much popularity in recent days concerned with civil engineering applications. Researchers had developed many tools and libraries focusing on employing the LR algorithm for various problems [Citation48].
In other words, a model in which the dependent variable (m) is established as a kernel function of the independent factors (n) can be derived using LR. Simple LR only contains one independent variable (n), whereas multiple LR contains two or more variables. The model has the following form in simple LR:
(2)
(2) The equation of a straight line is shown in Equation (2), where so and s1 stand in for the y-intercept and inclination, respectively.
3.7. K-nearest neighbours
Problems involving classification and regression can be solved using algorithm for supervised machine learning such as KNN. Most of the time, it is employed in classification issues. The input data for regression issues consist of k closest training sets provided in the identified set. The outcome depends on whether KNN is used as a regression or classification algorithm. The determining factor for the item, which is the sample mean of k's nearest neighbours, is the outcome of KNN regression. A parameter such as Euclidean or Mahalanobis can be utilized as the proximity metric to find the k of a data point [Citation49].
3.8. Random forest
One of these ensemble methods widely used is the random forest algorithm which can be effectively used to carry out classification and regression. The random forest method uses simple decision trees as the foundation learners. To develop a model for the issue, numerous decision trees are used. It uses the bootstrap combining or bagging strategy to train several trees. Bagging algorithms repeatedly choose Z random selection of testing phase from a given training phase (Y) collection and train the decision trees using those samples. Parallel generation of these decision trees prevents them from interacting with one another while they are being built. Equation (3) can be used to forecast the unknown samples (y′) once these trees have been trained:
(3)
(3) Since the major objective of this analysis was to forecast the UCS of the geopolymer blended clays, regression trees were created in the RF model. A generic RF analysis structure is shown in Figure .
The selection of the candidate hyperparameter values, or the values that must be assessed in terms of their out-of-bag performance, varies amongst search algorithms. Some techniques, such as random and grid searches, predetermine all possible hyperparameter values. Grid search, which evaluates every combination of the given discrete parameter spaces, is one of the simplest methods. However, prior to implementation, continuous parameters must be discretized. A different strategy is a random search, in which the hyperparameters’ values are chosen randomly from a predetermined hyperparameter space.
In random forests, the number of trees (or iterations) (ntree), which represents the number of decision trees, can be changed by users to regulate the ambiguity of the models. Random forests will overfit if this number is too high, and mtree shows the number of metrics chosen randomly as candidates at each split. The ntree and mtree parameters, which have the following effects on the random forest model, will be tuned in this work. The grid search strongly suggests hyperparameters by putting all reconfigurable grids in the optimal solution (Figure ). The grid's axes each represent algorithm parameters, and each grid point corresponds to a particular set of parameters. Therefore, every point's performance needs to be optimized.
3.9. Performance metrics evaluation
The effectiveness and accuracy of all machine learning methods used to predict the UCS of geopolymer soils were assessed using four statistical performance metrics, including coefficient of determination (R2), root mean square error (RMSE), mean absolute percentage error (MAPE) and mean absolute error (MAE).
R2 is a statistical measure that represents the goodness of fit of a regression model. The ideal value for R2 is 1. The closer the value of R2 to 1, the better is the model fitted. R2 is computed by using the following equation:
(4)
(4) where SSres is the residual sum of squares and SStotal is the total sum of squares.
MAE is a simple metric and is calculated by taking the mean of the absolute difference between the actual and the predicted (Equation 5).
(5)
(5) MSE is the most widely used and simplest metric. It is the mean of the squared difference between the actual and the predicted value (Equation 6).
(6)
(6)
RMSE is the square of the MSE, and it is given by Equation (7):
(7)
(7) where
is the actual and
is the predicted value.
4. Strength and micro-structural results of geopolymer soil blends
4.1. Compaction results of cement and geopolymer-treated clays
The compaction test results for expanding clay mixed with cement, fly ash-based geopolymers and slag-based geopolymers are listed in Table . OMC and MDD of untreated soil were found to be 18.8% and 1.67 g/cc, respectively.
Table 2. Compaction results of the stabilized expansive clays.
Based on the compaction characteristics data, as the additive content was increased in the blended cement clays, MDD increased and OMC dropped. The increased flocculation that occurs in the blends with increasing additive content is responsible for the rise in MDD and decline in OMC [Citation8]. OAC and MDD decrease with increased fly ash content in the fly ash-based geopolymer clays. As fly ash, a lightweight additive replaced the expansive clay in the blends, the weight of the compacted fly ash-based geopolymers decreased in turn MDD also decreased. On the other hand, in the case of slag-based geopolymer clays, the MDD value increased and the OAC value decreased with increasing slag content. This is due to the higher unit weight of the slag than the soil (specific gravity of slag with 2.9).
4.2. UCS results of cement and geopolymer-treated clays
Table shows the UCS values of the untreated and treated soils. The UCS of untreated virgin soil is found to be 0.15 MPa. Figure depicts the stress–strain behaviour of expansive clay blended with cement content for the 28-day curing period. It should be noted that the stress–strain values for 0 days of curing are not presented here. In general, soil specimens treated with either OPC or geopolymer demonstrated an increasing trend of UCS values in the presence of additives and the time progression, reaching the maximum value at 28 days of curing [Citation50].
Table 3. UCS results of the stabilized expansive clays.
The stress–strain measurements demonstrate that, for a specific percent of strain, the stress increased as the cement content increased, this is observed for all curing periods. The cementation process occurs more frequently as the cement component of the blends rises, making the blends tougher. As a result of the cementation process, uniaxial compressive stress applied to specimens increases for a given percent of strain. As a result, cement content also raises peak stress. For instance, for cement contents of 4%, 8%, 12%, 16% and 20%, the peak stress or failure stress for the 7-day curing period was 0.21, 0.34, 0.57, 1.09 and 1.74 MPa, respectively. Greater cementation occurs in the mixes as more cementitious products grow with a longer cure time; this is exhibited in all proportions of cement addition. As a result, when the curing time increases, so does the vertical applied load that must be applied to cylindrical mix specimens with a given cement concentration. For instance, when the cement concentration in the blend was 20%, the maximum compressive stress or breakdown stress was found to be 1.74 and 2.25 MPa, respectively, for the curing durations of 7 days and 28 days.
The stress–strain properties of fly ash-based geopolymer soils with various fly ash contents (0%, 4%, 8%, 12%, 16% and 20%) over the curing periods of 7 days and 28 days. The results show that the UCS increased with increasing fly ash concentration for a given percentage strain (see Figure ). This is observed for all curing periods of soil–geopolymer mixture. A continuous geopolymer matrix is created when the amount of fly ash in the geopolymer blends is increased. This continuous geopolymer matrix bonds the clay particles and occupies the spaces with geopolymer gel to create a tightly packed microstructure. As a result, the uniaxial compressive stress that must be applied to cylindrical composite specimens increases for a given percent of strain. The stress–strain curve shows that the soils loading pattern is linear up to a level and elasto-plastic behaviour is visible in major combinations of geopolymer-stabilized soil.
Figure illustrates how UCS varied with slag content in geopolymer soils based on slag for 28 days curing. For all curing times, UCS rose as slag content increased. When the curing time was extended from 7 to 28 days, UCS increased from 2.45 to 3.57 MPa for 20% slag content, representing a 45.7% increase. The increasing trend in both curing periods is consistent with the findings by Wu et al. [Citation51]. Slag-based geopolymer soils, in contrast, display more strength than blends of fly ash-based geopolymer soils at a given additive content.
Thus, it is evident that precursor concentration and chemical components of the precursor both play a key role in the strength development in geopolymer-stabilized soil. It is explained by the fact that slag contains more reactive silica than FA. Additionally, the oxide composition significantly influences the strength growth, especially SiO2 and Al2O3 [Citation52]. Therefore, for improved strength development, it is advised that the waste additive have SiO2 content of at minimum 50% and Al2O3 content of at minimum 25%. The strength development in the stabilized soil can also be linked to the oxide composition because FA and rice husk ash are rich in SiO2 and Al2O3 and suitably satisfy the parameters above. Additionally, the chemical makeup of slag reveals a more desirable Al2O3 level than the content of fly ash.
By comparison (see Figure ), the increment of UCS for all soils blended with slag-based geopolymer samples exhibits higher strength than the fly ash-based geopolymer samples at the given precursor dosage and curing period. The primary mechanism is the formation of geopolymeric and CASH gels from the alkaline activation of fly ash and slag. With more considerable Si and Al dissolution and CASH gel precipitation, the strength development of geopolymer-stabilized soils increases. According to ASTM D4609, an increase in UCS value of more than 345 kPa is appropriate for soil stabilization [Citation53]. According to the UCS findings, the geopolymer blended soils’ strength values with fly ash and slag as precursors increased by more than 345 kPa. According to the Indian Road Congress Design Guidelines, stabilized subgrades for highways must pass an unconfined compression test with a minimum strength of 2.5 MPa [Citation54]. Since the values obtained were greater than 2.5 MPa, the UCS results suggested that the geopolymer soils blended with larger doses of slag and fly ash samples could be employed as the road subgrade.
Figure (a–e) displays the microstructure of geopolymer blended soil, fly ash-based geopolymer soil and blast furnace slag-based geopolymer soil obtained by SEM analysis. Due to unreacted soil particles, samples of blended soil and geopolymer without any precursors revealed a non-homogeneous geopolymer matrix. Additionally, the geopolymer's less dense matrix contributes to its poor compressive strength because the curing process is carried out at room temperature, the presence of a non-homogeneous geopolymer system results in a weak response between the soil and geopolymers and an inefficient polymerization process [Citation55].
Figure 11. SEM images of the geopolymer soils blended with FA and S: (a) 8% FA, (b) 8% S, (c) 16% FA, (d) 16% S and (e) 20% S.

Fly ash-based geopolymer soil demonstrates the mechanism of geopolymer reaction with fly ash. The soil and fly ash fragments are still discernible in any case. The geopolymer matrix nearly completely encased the soil and fly ash particles. Small spaces, earth and fly ash fragments can still be detected. The spherical particles were also discovered to be covered in spongy particles. The accumulation of geopolymer gel in the sample revealed the geopolymerization of soil and fly ash particles [Citation56]. Small spaces and non-homogeneous geopolymers are two factors in the weakening of geopolymers [Citation57]. However, compared to soil-based geopolymers without fly ash, those that contain fly ash can yield materials with greater strength.
The most potent geopolymerization effect was demonstrated in slag-based geopolymer soils, where fewer unreacted particles were visible. A more uniform, dense and comparatively smooth intervening geopolymer matrix resulted from the entire reaction of the soil and slag particles. The geopolymer reaction over time after silica and alumina were dissolved (see in Figure ) confirmed the substantial increase in strength.
The soil and slag particles were fully geopolymerized, resulting in dense, comparatively smooth and encircled particles, which produced increased strength. Compared to other geopolymer blends, slag addition to soil-based geopolymer demonstrated the highest strength. These results are in accordance with Abdila et al. [Citation55].
4.3. Sustainability aspects of geopolymer soils
To assess the performance based on sustainability, the cost-effectiveness of clays treated with geopolymer and OPC is compared. Based on the UCS-to-cost ratio, the treated soils’ cost-efficiency is assessed. As previously indicated, the materials utilized in this study were purchased from local vendors. According to the most recent delivery record, the specific cost of each material is computed and displayed in Indian rupees (INR). The estimated cost-efficiency for soils treated with 20% cement, 20% fly ash-based geopolymers and 20% slag-based geopolymers are 0.0008, 0.0006 and 0.001 MPa/INR/m3, respectively.
Alsalman et al. [Citation58] calculated the energy needed and CO2 emissions of the manufacturing of specific materials. Energy consumption of 1 m3 stabilized soils manufacture results in geopolymer soils performing better in sustainability than OPC blended clays. Industrial waste products like fly ash and slag were used as precursors in the geopolymer soils, which lessens the need for natural resources like limestone, which is used to produce energy-intensive cement. Additionally, compared to cement-stabilized soils, the manufacture of 1 m3 geopolymer soils emits less CO2 (see Table ), which can aid in lowering the carbon footprint of the building sector.
Table 4. Sustainability indices comparison of stabilized clays with OPC, fly ash-based geopolymers and slag-based geopolymers.
The manufacturing of geopolymer soils uses less energy than cement production from an energy efficiency aspect. In addition, less energy is needed to create geopolymer soils than to prepare soils blended with cement. By storing carbon dioxide in the material, geopolymer soils used in carbon sequestration can also aid in removing carbon from the atmosphere. This can assist in balancing the emissions produced during production (see Figure ). Geopolymer soils are more desirable and environmentally friendly than OPC-stabilized soils. In which, geopolymer itself are environmentally friendly cementing agents with low energy consumption, low toxicity and are stable at high temperature as well as have high durability [Citation59,Citation60]. Overall, using geopolymer soils can offer the construction sector energy and eco-efficient solutions by lowering waste and resource usage, reducing energy use during manufacturing and lengthening the lifespan of the building.
4.4. UCS prediction results using machine learning techniques
The second aim of this research is to develop the machine learning model for the prediction of UCS value with the available database. For the same R2, RMSE, MSE and MAE were used to evaluate the prediction performance of the LR, KNN, RF, BRRF and BGRF methods to predict the compression strength of geopolymer soils for training and test data sets. However, it has been found that the R2 score, commonly known as the coefficient of determination, is the most appropriate number for model evaluation [Citation61]. While R2 values below 0.60 suggest unsatisfactory findings, values between 0.60 and 0.75 show reasonable results, values between 0.75 and 0.95 indicate strong model prediction and values over 0.95 imply outstanding prediction [Citation62]. Lowest RMSE and MAE indicate higher prediction ability with minimal prediction error [Citation63]. To determine the model's efficacy, the best model from the current study was compared with models from earlier investigations.
Figure compares the projected UCS values produced by a linear regression approach to the pertinent measurements. To handle any potential overfitting issues in the linear regression, ridge regression, a regularized variant of the linear regression approach, was used. The ridge regression decreases the size of the coefficients by imposing a penalty on the size of the coefficients. By minimizing a penalized residual sum of squares, this penalty is achieved. Additionally, ridge regression can improve penalized coefficients, which typically minimize variance and lessen overfitting. According to Table , the R2 values from the ridge regression model are the same 0.83 for the training and testing sets.
Table 5. Performance metrics comparison of various models.
The measured and predicted UCS values for the training and testing sets are shown in Figure . As observed, most data points are situated around the line of equality (red line). In the training group, the RF model has R2 = 0.99, MSE = 0.41 and RMSE = 0.65. However, with R2 = 0.95, MSE = 2.02 and RMSE = 1.42, the test set performance is marginally worse than the training sets. In general, a lower R2 denotes overfitting in the testing set. However, given the strong R2 and low RMSE values obtained, this is not a serious concern for the RF model investigation. The RF approach uses numerous regression trees and sections of the input variables at random to find intrinsic correlations between features in genuine scenarios. The randomization considerably improves the model's durability. Each regression tree in the RF model could be considered an ensemble method because it splits at nodes. To reduce the amount of variance in a single tree, the RF model combines these functions. The ridge regression fared much worse than the RF. This is likely because the linear regression model could not account for the non-linear relationship between the UCS and the covariates.
The BRRF model outperforms the other models with lowest RMSE of 0.46 for the training sets, as shown in Figure . Concerned with performance, geopolymer soils that fit well in the UCS have RMSE, MSE and MAE values less than 0.50 [Citation63]. Furthermore, the approaches RF, BRRF and BGRF anticipate the actual readings effectively, as shown in Figures . The prediction graph, where the values are gathered along the prediction line shows reduced dispersion compared to the LR and KNN (see ). It also illustrates the predicted values for the model prepared using RF and improved RF was relatively similar to the measured data. These findings show that BRRF has a good ability to pick up new information from training data.
The values for the training and test data sets for all the models for the UCS of geopolymer soils are listed in Table , together with the findings of R2, RMSE, MAE and MAPE. The errors in the training data set show how adequate they produced model is, while the errors in the test data show how generalizable the developed model is. Furthermore, since the coefficients of determination R2 for all models, except the LR model, are higher than 0.9, it can be shown that they all exhibit good concordance with the training data, proving that they are capable of accurately predicting the UCS of geopolymer soils. When comparing the metrics of all the models for the test data, it can be seen in Table that BRRF outperforms them in terms of prediction accuracy and generalizability. This is because it exhibits a satisfactory value of higher R2 and low values of MAE, RMSE and MSE. Therefore, these promising results should be used to choose BRRF as a good model even though all the models’ statistical metrics on the testing data are similar.
Results from the RF model are compared with those from the most recent machine learning models to highlight the advanced RF model's predictive capability in this study. Artificial neural networks (ANN), multi-gen genetic programming (MGP) and the multivariable regression model (MVR) were three machine learning approaches that were taken into consideration. The models mentioned above, accuracy was evaluated using MAE, which is a crucial measure for assessing a model's performance. To show that the study's BRRF model is effective, the MAE values of previously developed models MVR [Citation45], MGP [Citation23], ANN [Citation45] and the present study BRRF were shown in Figure . As shown, the study's suggested model has the lowest MAE (Figure ).
The RF is a more accurate regression technique than a single regression tree. This was accomplished at the expense of the interpretability of the tree-based approaches, in any case. It is practical to assess the variable relevance in the RF, much like a single regression tree. The relative relevance of the variables in the RF model is depicted in Figure . The method that aids in comprehending the effects of each input variable on the output variable is known as feature importance. High-sensitivity input factors have a more significant effect on the output variable. As a result, the input factors significantly influence the prediction of the output variable. Using normalized importance, it was possible to assess how each of the input variables such as LL%, PI%, S%, FA%, M, A/B, Na/Al and Si/Al affected the uncertainty of the UCS (MPa) output variable.
All input variables, including LL, PI, S%, FA%, M, A/B, Na/Al and Si/Al, are crucial for estimating the UCS of geopolymer soils. The findings of this feature importance analysis are shown in Figure , where the percentage of ground granulated blast furnace slag, the liquid limit and Na/Al are the most important input variables in predicting the UCS of the geopolymer soils. On the other hand, fly ash content and Si/Al ratio were shown to be the least useful variables for predicting the UCS of geopolymer soils. These results are consistent with those of earlier studies. The S% has been proven to be crucial in determining the UCS of geopolymer soils. Additionally, the heat map in Figure demonstrates that the UCS is inversely related to the S%. In other words, the development of UCS in geopolymer soils is significantly influenced by geopolymers with high Si and Al concentration.
5. Conclusions
One crucial task for civil engineers involved in geotechnical construction activities is the selection of good soil stabilizers which will help to attain higher UCS and provide sustainable performance. Focusing on this, the authors evaluated the efficacy of geopolymer-stabilized soils and developed models for forecasting strength properties using machine learning techniques. The study produced the findings which are summarized as follows:
Obtained UCS results show that geopolymer blended clay containing 20% of slag offers better strength than that of fly ash-based geopolymer and OPC-treated clay blends.
An increase in the UCS of the geopolymer mix proved to be depending on the precursor type and its dosage.
Higher degree of geopolymerization was observed in the slag-based stabilized soils, with a more uniform, dense, effective dilution of Si and Al and a comparatively smooth intervening geopolymer matrix.
Slag-based geopolymer clays are more efficient in terms of cost/strength ratio than that of OPC-treated clays for a given additive content. Furthermore, fly ash-based geopolymer exhibits lower carbon emissions and energy consumption than slag-based geopolymer clays and OPC-treated clays.
The BRRF model showed a high coefficient of determination of 0.96 in the test data set, indicating that it is more reliable for forecasting. Additionally, no overfitting was generated, as concluded by the extremely low RMSE (1.23) values on the training and test sets.
According to the variable significance determined by the RF model, ground granulated blast furnace slag (%S), liquid limit (LL) and the ratios of Na to Al were the factors that had the most significant influence on the development of UCS. In contrast, fly ash (%F) and ratios of Si to Al had the least.
Hence, we suggest utilizing slag-based soil stabilizer incorporating geopolymer for enhanced soil strength and also suggest that the BRRF model can be used for predicting the strength of the soil.
Disclosure statement
No potential conflict of interest was reported by the author(s).
References
- Ikeagwuani CC, Nwonu DC. Emerging trends in expansive soil stabilisation: a review. J Rock Mech Geotech Eng. 2019;11(2):423–440. doi:10.1016/j.jrmge.2018.08.013
- Tiwari N, Satyam N, Shukla SK. An experimental study on micro-structural and geotechnical characteristics of expansive clay mixed with EPS granules. Soils Found. 2020;60(3):705–713. doi:10.1016/j.sandf.2020.03.012
- Zimar Z, Robert D, Sidiq A, et al. Waste-to-energy ash for treating highly expansive clays in road pavements. J Clean Prod. 2022;374:133854. doi:10.1016/j.jclepro.2022.133854
- Phanikumar BR. Effect of lime and fly ash on swell, consolidation and shear strength characteristics of expansive clays: a comparative study. Geomech Geoeng Int J. 2009;4(2):175–181. doi:10.1080/17486020902856983
- Rao MR, Rao AS, Babu DR. Efficacy of lime-stabilised fly ash in expansive soils. Proc Inst Civil Eng Ground Improv. 2008;161(1):23–29. doi:10.1680/grim.2008.161.1.23
- Watanabe K, Nakajima S, Fujiwara T, et al. Construction and field measurement of high-speed railway test embankment built on Indian expansive soil “Black Cotton Soil”. Soils Found. 2021;61(1):218–238. doi:10.1016/j.sandf.2020.08.008
- Rao AS, Phanikumar BR, Babu RD, et al. Pullout behavior of granular pile-anchors in expansive clay beds in situ. J Geotech Geoenviron Eng. 2007;133(5):531–538. doi:10.1061/(ASCE)1090-0241(2007)133:5(531)
- Phanikumar BR, Nagaraju TV. Engineering behaviour of expansive clays blended with cement and GGBS. Proc Inst Civil Eng Ground Improv. 2018;171(3):167–173.
- Jain A, Choudhary AK, Jha JN. Influence of rice husk ash on the swelling and strength characteristics of expansive soil. Geotech Geol Eng. 2020;38(2):2293–2302. doi:10.1007/s10706-019-01087-6
- Lakkimsetti B, Nayak S. Experimental investigation and performance evaluation of lithomargic clay stabilized with granulated blast furnace slag and calcium chloride. Int J Geosyn Ground Eng. 2022;8(1):1–16. doi:10.1007/s40891-021-00345-z
- Sivapullaiah PV, Sridharan A, Bhaskar Raju KV. Role of amount and type of clay in the lime stabilization of soils. Proc Inst Civil Eng Ground Improv. 2000;4(1):37–45. doi:10.1680/grim.2000.4.1.37
- Bhuvaneshwari S, Robinson R, Gandhi S. Micro-fabric and mineralogical studies on the stabilization of an expansive soil using inorganic additives. Int J Geotech Eng. 2010;4(3):395–405. doi:10.3328/IJGE.2010.04.03.395-405
- Mahasenan N, Smith S, Humphreys K. The cement industry and global climate change: current and potential future cement industry CO2 emissions. Greenhouse gas control technologies, 6th international conference; 2003, p. 995–1000. doi:10.1016/B978-008044276-1/50157-4
- Sinha P, Iyer KK. Effect of stabilization on characteristics of subgrade soil: a review. Adv Comput Methods Geomech. 2020;55:667–682. doi:10.1007/978-981-15-0886-8_54
- Miller SA, Monteiro PJ, Ostertag CP, et al. Comparison indices for design and proportioning of concrete mixtures taking environmental impacts into account. Cem Concr Compos. 2016;68:131–143. doi:10.1016/j.cemconcomp.2016.02.002
- Nawaz M, Heitor A, Sivakumar M. Geopolymers in construction-recent developments. Constr Build Mater. 2020;260:120472. doi:10.1016/j.conbuildmat.2020.120472
- Komnitsas KA. Potential of geopolymer technology towards green buildings and sustainable cities. Procedia Eng. 2011;21:1023–1032. doi:10.1016/j.proeng.2011.11.2108
- Duxson P, Fernández-Jiménez A, Provis JL, et al. Geopolymer technology: the current state of the art. J Mater Sci. 2007;42(9):2917–2933. doi:10.1007/s10853-006-0637-z
- Li L, Zhang X. A new triaxial testing system for unsaturated soil characterization. Geotech Test J. 2015;38(6):823–839.
- Sharma LK, Singh TN. Regression-based models for the prediction of unconfined compressive strength of artificially structured soil. Eng Comp. 2018;34(1):175–186. doi:10.1007/s00366-017-0528-8
- Alzabeebee S, Alshkane YM, Rashed KA. Evolutionary computing of the compression index of fine-grained soils. Arab J Geosci. 2021;14(19):1–17.
- Yin ZY, Jin YF, Liu ZQ. Practice of artificial intelligence in geotechnical engineering. J Zhejiang Univer Sci A. 2020;21(6):407–411. doi:10.1631/jzus.A20AIGE1
- Soleimani S, Rajaei S, Jiao P, et al. New prediction models for unconfined compressive strength of geopolymer stabilized soil using multi-gen genetic programming. Measurement. 2018;113:99–107.
- Mozumder RA, Laskar AI, Hussain M. Empirical approach for strength prediction of geopolymer stabilized clayey soil using support vector machines. Constr Build Mater. 2017;132:412–424. doi:10.1016/j.conbuildmat.2016.12.012
- Davidovits J. Geopolymers and geopolymeric materials. J Ther Anal. 1989;35(2):429–441. doi:10.1007/BF01904446
- Ricciotti L, Occhicone A, Ferone C, et al. Development of geopolymer-based materials with ceramic waste for artistic and restoration applications. Materials. 2022;15(23):8600. doi:10.3390/ma15238600
- Provis JL, Van Deventer JSJ. Geopolymers: structures, processing, properties and industrial applications. New York: CRC Press; 2009.
- Kumar S, Kumar R. Mechanical activation of fly ash: effect on reaction, structure and properties of resulting geopolymer. Ceram Int. 2011;37(2):533–541. doi:10.1016/j.ceramint.2010.09.038
- Khale D, Chaudhary R. Mechanism of geopolymerization and factors influencing its development: a review. J Mater Sci. 2007;42(3):729–746. doi:10.1007/s10853-006-0401-4
- Morsy MS, Alsayed SH, Al-Salloum Y, et al. Effect of sodium silicate to sodium hydroxide ratios on strength and microstructure of fly ash geopolymer binder. Arab J Sci Eng. 2014;39(6):4333–4339. doi:10.1007/s13369-014-1093-8
- Matsuoka M, Yokoyama K, Okura K, et al. Synthesis of geopolymers from mechanically activated coal fly ash and improvement of their mechanical properties. Minerals. 2019;9(12):791. doi:10.3390/min9120791
- Kamseu E, Kaze CR, Fekoua JNN, et al. Ferrisilicates formation during the geopolymerization of natural Fe-rich aluminosilicate precursors. Mat Chem Phys. 2020;240:122062. doi:10.1016/j.matchemphys.2019.122062
- Lei J, Law WW, Yang EH. Effect of calcium hydroxide on the alkali-silica reaction of alkali-activated slag mortars activated by sodium hydroxide. Constr Build Mater. 2021;272:121868. doi:10.1016/j.conbuildmat.2020.121868
- Provis JL, Bernal SA. Binder chemistry–blended systems and intermediate Ca content. In: Provis J, van Deventer J, editors. Provis, J., van Deventer, J. (eds) Alkali activated materials. RILEM State-of-the-Art Reports, Dordrecht: Springer; 2014. p. 125–144.
- Vafaei M, Allahverdi A. High strength geopolymer binder based on waste-glass powder. Adv Powd Technol. 2017;28(1):215–222. doi:10.1016/j.apt.2016.09.034
- Ibrahim M, Johari MAM, Maslehuddin M, et al. Influence of nano-SiO2 on the strength and microstructure of natural pozzolan based alkali activated concrete. Constr Build Mater. 2018;173:573–585. doi:10.1016/j.conbuildmat.2018.04.051
- Shehata N, Sayed ET, Abdelkareem MA. Recent progress in environmentally friendly geopolymers: a review. Sci Total Environ. 2021;762:143166. doi:10.1016/j.scitotenv.2020.143166
- Ghadir P, Razeghi HR. Effects of sodium chloride on the mechanical strength of alkali activated volcanic ash and slag pastes under room and elevated temperatures. Const Build Mater. 2022;344:128113. doi:10.1016/j.conbuildmat.2022.128113
- Shariatmadari N, Hasanzadehshooiili H, Ghadir P, et al. Compressive strength of sandy soils stabilized with alkali-activated volcanic ash and slag. J Mater Civ Eng. 2021;33(11):04021295. doi:10.1061/(ASCE)MT.1943-5533.0003845
- Razeghi HR, Ghadir P, Javadi AA. Mechanical strength of saline sandy soils stabilized with alkali-activated cements. Sustainability. 2022;14(20):13669. doi:10.3390/su142013669
- Samadi P, Ghodrati A, Ghadir P, et al. Effect of seawater on the mechanical strength of geopolymer/cement stabilized sandy soils. Proceedings of the TMIC 2022 Slope Stability Conference (TMIC 2022); 2023; vol. 13, p. 121–129. doi:10.2991/978-94-6463-104-3_12
- Zhao J, Tong L, Li B, et al. Eco-friendly geopolymer materials: a review of performance improvement, potential application and sustainability assessment. J Clean Prod. 2021;307:127085. doi:10.1016/j.jclepro.2021.127085
- Hardjito D, Wallah SE, Sumajouw DM, et al. Fly ash-based geopolymer concrete. Aust J Struct Eng. 2005;6(1):77–86. doi:10.1080/13287982.2005.11464946
- Parthiban D, Vijayan DS, Koda E, et al. Role of industrial based precursors in the stabilization of weak soils with geopolymer – a review. Case Stud Constr Mater. 2022;16:e00886.
- Mozumder RA, Laskar AI. Prediction of unconfined compressive strength of geopolymer stabilized clayey soil using artificial neural network. Comput Geotech. 2015;69:291–300. doi:10.1016/j.compgeo.2015.05.021
- Mahmoodzadeh A, Mohammadi M, Ibrahim HH, et al. Artificial intelligence forecasting models of uniaxial compressive strength. Transp Geotech. 2021;27:100499. doi:10.1016/j.trgeo.2020.100499
- Zhang G, Ding Z, Wang Y, et al. Performance prediction of cement stabilized soil incorporating solid waste and propylene fiber. Materials. 2022;15(12):4250.
- Nagaraju TV, Mantena S, Azab M, et al. Prediction of high strength ternary blended concrete containing different silica proportions using machine learning approaches. Results Eng. 2023;17:100973.
- Hamed A, Sobhy A, Nassar H. Accurate classification of COVID-19 based on incomplete heterogeneous data using a KNN variant algorithm. Arab J Sci Eng. 2021;46(9):8261–8272. doi:10.1007/s13369-020-05212-z
- Ghadir P, Ranjbar N. Clayey soil stabilization using geopolymer and portland cement. Constr Build Mater. 2018;188:361–371. doi:10.1016/j.conbuildmat.2018.07.207
- Wu D, Zhang Z, Chen K, et al. Experimental investigation and mechanism of fly ash/slag-based geopolymer-stabilized soft soil. Appl Sci. 2022;12(15):7438. doi:10.3390/app12157438
- Chindaprasirt P, De Silva P, Sagoe-Crentsil K, et al. Effect of SiO2 and Al2O3 on the setting and hardening of high calcium fly ash-based geopolymer systems. J Mater Sci. 2012;47(12):4876–4883. doi:10.1007/s10853-012-6353-y
- Onyejekwe S, Ghataora GS. Soil stabilization using proprietary liquid chemical stabilizers: sulphonated oil and a polymer. Bull Eng Geol Environ. 2015;74:651–665. doi:10.1007/s10064-014-0667-8
- Gupta A, Arora VK, Biswas S. Contaminated dredged soil stabilization using cement and bottom ash for use as highway subgrade fill. Int J Geo-Eng. 2017;8:1–13.
- Abdila SR, Abdullah MMAB, Ahmad R, et al. Evaluation on the mechanical properties of ground granulated blast slag (GGBS) and fly ash stabilized soil via geopolymer process. Materials. 2021;14(11):2833.
- Abdullah HH, Shahin MA, Walske ML. Geo-mechanical behavior of clay soils stabilized at ambient temperature with fly-ash geopolymer-incorporated granulated slag. Soils Found. 2019;59(6):1906–1920. doi:10.1016/j.sandf.2019.08.005
- Abbas IS, Abed MH, Canakci H. Development and characterization of eco-and user-friendly grout production via mechanochemical activation of slag/rice husk ash geopolymer. J Build Eng. 2023;63:105336. doi:10.1016/j.jobe.2022.105336
- Alsalman A, Assi LN, Kareem RS, et al. Energy and CO2 emission assessments of alkali-activated concrete and ordinary Portland cement concrete: a comparative analysis of different grades of concrete. Clean Environ Syst. 2021;3:100047. doi:10.1016/j.cesys.2021.100047
- Barbosa VF, MacKenzie KJ. Thermal behaviour of inorganic geopolymers and composites derived from sodium polysialate. Mater Res Bull. 2003;38(2):319–331. doi:10.1016/S0025-5408(02)01022-X
- Pacheco-Torgal F, Abdollahnejad Z, Miraldo S, et al. An overview on the potential of geopolymers for concrete infrastructure rehabilitation. Constr Build Mater. 2012;36:1053–1058. doi:10.1016/j.conbuildmat.2012.07.003
- Soni U, Roy A, Verma A, et al. Forecasting municipal solid waste generation using artificial intelligence models—a case study in India. SN Appl Sci. 2019;1(2):1–10. doi:10.1007/s42452-018-0157-x
- Hair JF, Risher JJ, Sarstedt M, et al. When to use and how to report the results of PLS-SEM. Eur Bus Rev. 2019;31(1):2–24. doi:10.1108/EBR-11-2018-0203
- Feby B, Achu AL, Jimnisha K, et al. Landslide susceptibility modelling using integrated evidential belief function based logistic regression method: A study from Southern Western Ghats, India. Remote Sens Appl Soc Environ. 2020;20:100411.