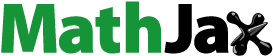
Abstract
Control charts play a vital role in process monitoring to ensure the product's desired standards. Due to rapid improvements in data collection methods, multivariate charts are preferred over univariate charts. This paper proposes a bivariate exponentially weighted moving average chart for the simultaneous monitoring of the mean vector of Gumbel's bivariate Pareto type II (also known as the Lomax distribution) time-between-events data. The performance of the proposed chart is assessed through average run length, median run length, and the standard deviation of the run length criteria. To show the implementation of the chart in the real world, illustrative examples are also presented.
1. Introduction
Quality control is a process to ensure defect-free products. Statistical process control (SPC) is a method of quality control and quality assessment that uses statistical techniques to monitor and control processes. The SPC methods not only monitor the behaviour of a process, but also help to identify the root cause of deterioration that occurred in the process. A control chart is one of the most important SPC tools that graphically monitors the variations of the process over time. The use of control charts helps to detect and eliminate the assignable causes of variation that may exist in a production process.
Traditional control charts like the Shewhart control chart are developed by assuming normality. This normality assumption is not suitable for high-quality processes and thus, the idea of time-between-events (TBE) is used to monitor them [Citation1]. The TBE charts are used to monitor the time interval between two arrivals of events. For example, in the service industry, the arrival of a customer refers to an event while the time interval between two customers is known as the TBE. Researchers introduced different TBE charts in the literature [Citation1,Citation2]. Borror et al. [Citation3] investigated the robustness of TBE using a cumulative sum (CUSUM) scheme with lognormal and Weibull distributions. Liu et al. [Citation4] compared different one-sided and two-sided exponential TBE control charts. Zhang et al. [Citation5] preferred a random-shift model over a fixed-shift model to propose a TBE control chart using the gamma distribution.
Ali et al. [Citation6] reviewed the previously proposed TBE charting schemes for monitoring high-quality processes. Ali [Citation7] assumed a class of absolutely continuous exponentiated distributions to propose TBE control charts based on the renewal process. For monitoring the scheduled time by assuming exponentially modified Gaussian (EMG) distribution, a TBE control chart was developed by Ara et al. [Citation8]. Based on the renewal process, a comparison of Shewhart-Type TBE control charts was given by Ali et al. [Citation9]. Li et al. [Citation10] compared some previously proposed memory-type control charts.
More recent contribution in this direction can be seen in [Citation11,Citation12], and references cited therein.
Most of the industrial operational procedures during production processes consist of more than one correlated quality characteristics of interest and require appropriate monitoring [Citation13]. Separate monitoring of these naturally correlated quality characteristics through univariate control charts can be misleading and inefficacious [Citation14]. Shewhart-type multivariate control charts for a mean vector were first developed by Hotelling [Citation15]. Afterward, many researchers proposed multivariate control charts, including the multivariate EWMA (MEWMA) charts by Lowry et al. [Citation16], multivariate CUSUM (MCUSUM) charts by Crosier [Citation17], and Pignatiello Jr and Runger [Citation18]. A Max-MCUSUM control chart that simultaneously detects shifts in both the mean vector and covariance matrix of a multivariate process was proposed by Cheng and Thaga [Citation19]. Golosnoy et al. [Citation20] investigated the properties for the charts given by Pignatiello Jr and Runger [Citation18] and suggested some improvements. A multivariate synthetic MSCUSUM control chart was developed by Lee et al. [Citation21]. This chart performed better for moderate and large shift detection when compared to the multivariate synthetic EWMA control chart. Yeh et al. [Citation22] reviewed multivariate control charts constructed for monitoring changes that occurred in the covariance matrix of a process. Bersimis et al. [Citation23] gave a review of the multivariate control charts.
While dealing with high-quality processes, the TBE data follow a non-normal or highly skewed distribution. Monitoring the TBE data by utilizing traditional multivariate charts with normality assumptions may lead to poor detection power and ineffective results. For monitoring univariate and multivariate skewed populations, several nonparametric control charting schemes were developed by Zou and Tsung [Citation24], Tang et al. [Citation25], Zhou et al. [Citation26], and Yue and Liu [Citation27]. These approaches may appeal but are difficult to implement because of the high computational cost. Several transformations are developed and implemented by different authors to transform non-normal multivariate data to approximately normal data using the double square-root method [Citation28]. By using the weighted standard deviation method, Chang and Bai [Citation29] developed a multivariate chart, whereas [Citation30] proposed multivariate EWMA and CUSUM control charts. The use of data transformation methods for process monitoring should be made carefully as it leads to the problem of loss of important information. The MEWMA control chart proposed by Stoumbos and Sullivan [Citation31] and Testik et al. [Citation32] showed robustness to non-normality assumption with small smoothing parameters. Based on these findings, the MEWMA chart for monitoring the mean shift vector of a Gumbel's bivariate exponential (GBE) distribution [Citation33] was considered by Xie et al. [Citation34]. This MEMWA chart performed better than the paired individual t chart and the paired individual EWMA chart for both raw and transformed data. To monitor Gumbel's bivariate exponential data, Xie et al. [Citation35] proposed a multivariate CUSUM control chart. The chart showed better results in comparison with the MEWMA chart and paired individual CUSUM chart. To monitor bivariate gamma distributed processes, Xie et al. [Citation36] proposed a synthetic MEWMA scheme.
Pareto distribution is also known as power-law probability distribution introduced by Vilfredo Pareto. It is extensively used by several researchers in different research areas such as insurance [Citation37–39], business [Citation40], reliability [Citation41–48], economics [Citation49,Citation50], finance, actuarial science and survival analysis, etc. In quality control, Guo and Wang [Citation51] proposed control charts using Pareto distribution. Nil et al. [Citation52] developed optimal design of control chart with Pareto distribution. Furthermore, Seif et al. [Citation53] and numerous other researchers have also used Pareto distribution to introduce control charts. However, Pareto distribution is rarely used for the construction of bivariate charting schemes. Considering the utility and applicability, here we have used the bivariate Pareto type II (Lomax) distribution to introduce the TBE chart.
In this article, a MEWMA control chart for Gumbel's bivariate Pareto (GBP) distribution is proposed. This chart is constructed by using the bivariate Pareto model which is an extension of the Gumbel bivariate exponential (GBE) model first developed by [Citation33]. To investigate the zero- and steady-state performance of the chart, run length profile summaries using the average run length (ARL), the standard deviation of run length (SDRL), and the median run length are used.
The rest of the article is organized as follows. Section 2 gives a brief review of Gumbel's bivariate exponential model. Section 3 describes the design structure of the MEWMA control chart for the GBP data. The charting procedure is discussed in Section 4. Section 5 explains the procedure for obtaining the average run length of the proposed chart for both zero-state and steady-state cases. Section 6 shows a comparative analysis of zero-state and steady-state run length performance. To demonstrate the implementation of the proposed chart, Section 7 presents illustrative examples using simulated and real life data sets. A multivariate extension of the GBP model is introduced in Section 8. Finally, the conclusion is given in Section 9.
2. Gumbel's bivariate exponential model
Suppose random variables () follow GBE model, then
with the joint survival function
(1)
(1) where the range of dependence parameter κ is
.
and
are considered uncorrelated if κ=1, otherwise correlated. A more general form for the GBE model developed by Lu and Bhattacharyya [Citation54] is given as
(2)
(2) where
and
are two scale parameters. The general GBE model has the following probability density function.
(3)
(3) The marginal distribution of
and
follows the exponential distribution which can be easily proven by using the joint survival function of the GBE model. Thus, the mean vector μ of
and
is equal to
(4)
(4)
while its variance-covariance matrix is
(5)
(5) The coefficient of correlation suggested by Lu and Bhattacharyya [Citation54] is given as
(6)
(6) where
denotes the gamma function.
3. Structure of a MEWMA control chart on gumbel bivariate pareto (GBP) data
Arnold [Citation55] proposed the multivariate type II Pareto distribution, which has the following joint survival function
(7)
(7) where
represents the mean,
represents the standard deviation, and α is the shape parameter that gauges how heavy the tail is. Its marginal distributions follow the univariate Pareto type II distributions. The marginal means are
(8)
(8) while variances, covariances, and correlation are
(9)
(9)
(10)
(10)
(11)
(11) Pareto Type II (Lomax) distribution occurs as an extension of Pareto Type II distribution when μ is taken equal to zero. The survival function of univariate Pareto Type II (Lomax) distribution is given by
(12)
(12) Let
and
denote the TBE data of interest and assume the joint distribution of (
) follows the Gumbel bivariate Pareto Type II (Lomax) distribution. Then,
and the joint survival function using Equation (Equation1
(1)
(1) ) is
(13)
(13)
where the dependence parameter , and
and
are independent if
. By using the joint survival function of the Gumbel bivariate Pareto (GBP) model, it can be obtained that the marginal distributions of (
) follow the univariate Pareto Type II (Lomax) distribution, where ‘α’ is the shape parameter that denotes the tail index, ‘
’ and ‘
’ are the scale parameters. Thus, the mean vector of (
) is denoted by
(14)
(14) and for
;
and
. The correlation coefficient of (
) is as follows
(15)
(15) and the covariance matrix of (
) is
(16)
(16)
4. Charting procedure of a MEWMA control chart on the GBP data
The MEWMA control chart was first developed by Lowry et al. [Citation16] to detect shifts in the mean by assuming multivariate normal distribution along with a target mean vector and variance-covariance matrix
. By taking X
as the
(i = 1, 2,…) observation vector of the process, the MEWMA statistic is defined as follows:
(17)
(17) where
represents the smoothing or learning parameter for
, and p represents the dimension of the data, the diag
is denoted by
, identity matrix by
, and
is taken equal to ‘
’, i.e. (
). This chart gives a signal when the charting statistic
where
. The variance-covariance matrix of
is denoted by
and the upper control limit (UCL) is denoted by ‘h’.
If , with a constant
, then for i
,
(18)
(18) The traditional MEWMA chart assumed that the variance-covariance matrix of the underlying multivariate normal distribution remains constant if a shift occurs in the mean. However, this assumption cannot be guaranteed for most of the multivariate skewed distributions. Stoumbos and Sullivan [Citation31] developed MEWMA control charts by considering the asymptotic covariance matrix and a small smoothing parameter to show robustness toward non-normal distributions. Later, to figure out the robustness feature, Xie et al. [Citation34] applied the MEWMA charting statistic of [Citation16] to the GBE model. Thus, here we extend the work of [Citation34] by applying Lowry's MEWMA charting statistic to the GBP model by assuming that the TBE data (
follow GB
, whereas the dependence parameter denoted by κ remains constant. The charting procedure of the MEWMA control chart for monitoring the mean vector (
) using the GBP model can be concisely summarized as follows.
Step 1 | Compute the recursive statistic
| ||||
Step 2 | Calculate the MEWMA statistic at | ||||
Step 3 | The process is said to be out-of-control when |
Different estimators, like the maximum likelihood estimators for parameters of the GBE model are given by Lu and Bhattacharyya [Citation54,Citation56]. Practically, using in-control historical data, the mean vector and sample variance-covariance matrix, and
can be estimated as follows
(21)
(21)
(22)
(22) However,
and
can also be estimated by first estimating the in-control parameters of the GBP model and then computing
and
by using Equations (Equation14
(14)
(14) ) and (Equation16
(16)
(16) ).
The Monte Carlo simulation method is used with specified design parameters ω and h to achieve the desired in-control ARL (). The purpose of using the Monte Carlo simulation method for the computation of the ARL is to avoid the difficulties and complexities that occur when dealing with the previously published analytical approaches based on the multivariate normal distribution. These approaches include the probability limit method, integral equation approach, and bivariate Markov Chain method. Thus, 50,000 simulation runs are used to obtain the in-control (IC) average run length (ARL)
and out-of-control (OOC) ARL (
) with the help of simulation algorithms developed in statistical software R. Different values of smoothing parameters are used, e.g. ω= 0.02, 0.05, 0.1, 0.3, 0.5, 0.8, 1.00. A value of ‘h’ is determined for each case of ω to achieve the desired pre-fixed
.
5. Average run length of the MEWMA GBP control chart
The most commonly used approach for performance assessment of a control chart is the ARL. For the proposed MEWMA GBP control chart, we set , and the performance is studied for the zero-state and steady-state. The IC process parameters are denoted by
while the OOC process parameters are labelled by
. It is worth mentioning that the value of the dependence parameter is kept constant.
5.1. Zero-state case
Zero-state is widely reported and discussed for a control chart assessment. In the zero-state case, it is assumed that a sustained shift has occurred in the process parameter when a process starts [Citation57]. As [Citation35] (in appendix A) kept fixed the dependence parameter, we follow the same fashion to determine the control limit and performance of the proposed chart. Therefore, the ARL performance of the developed MEWMA GBP control chart depends only on the mean shift vector (,
), where
=
/
and
=
/
denote shifted means while (
,
)=(1, 1) denotes the in-control process mean. The steps to determine the optimal design parameter ‘h’ of the proposed MEWMA GBP control chart are given below.
Step 1 | Choose a desired value of | ||||
Step 2 | Select an appropriate value of the smoothing parameter, e.g. | ||||
Step 3 | Compute the |
5.2. Steady-state case
The steady-state ARL is significantly more informative than the zero-state ARL. For the steady-state case, a summary of two different shift models, the fixed-shift model, and the random-shift model, is given by Szarka III and Woodall [Citation58]. In the fixed-shift model, a shift at a particular iteration, say rth iteration, is introduced. Thus, before the occurrence of a shift, the time of running r in-control observations is called the ‘warm-up period.’ Several researchers considered this model including [Citation57,Citation59]. Contrary to the fixed-shift model, the random-shift model assumes that a shift occurs at any time rather than the occurrence of a shift after a warm-up period. Wu and Spedding [Citation60] discussed the random-shift model in detail which can be consulted for more information.
This study uses a fixed-shift model to study the performance of steady-state ARL. The steps to compute the ARL are given below.
Step 1 | Fix ‘h’ (as discussed previously), the shape parameter α, the scale parameters | ||||
Step 2 | Generating ‘r’ in-control random vectors | ||||
Step 3 | Run warm-up period by initiating the procedure with the IC ‘r’ generated random vectors before a shift in the shape parameter (α) and scale parameters ( | ||||
Step 4 | Generate OOC random vectors | ||||
Step 5 | Plot the MEWMA statistic against h and conclude the process to be OOC if | ||||
Step 6 | Repeat steps 2–5, |
6. Comparative analysis
The performance of the MEWMA GBP chart is evaluated for both zero-state and steady-state cases for different values of desired 200,370,500,
0.02, 0.05, 0.1, 0.3, 0.5, 0.8, 1.00, and with a fixed dependency parameter, i.e.
. For OOC
, the following three types of shifts are introduced for the zero- and steady-state cases.
an upward shift
a hybrid shift
a downward shift
6.1. An upward shift detection
The process has an upward shift if
has an upward shift but
has no shift, i.e.
,
or
has no shift but
has an upward shift. This presents the case
,
or both
and
has an upward shift, representing
.
The first two cases are known as the ‘single upward shifts’ while the third case is the ‘double upward shift.’ The upward shift detection is critical when the interest lies in positive events such as the success of an activity, a product's purchase order, etc.
6.2. A hybrid shift detection
The process has a hybrid shift if
has an upward shift whereas
shifts downward, that is,
,
or
has a downward shift whereas
shifts upward, i.e.
.
6.3. A downward shift detection
The process has a downward shift if
has a downward shift while
has no shift (
),
or
has no shift but
has a downward shift (
),
or both
and
have a downward shift (
).
In quality control applications when interest lies in negative events, such as defects and nonconformities, downward shift detection plays an important role. Thus, downward shift detection of the GBP model is discussed here because a shorter TBE is a signal of a downward shift.
6.4. Run length profiles of zero state case
Table tabulates the control limits assuming different smoothing parameters and . Tables and list the results for individual shifts introduced in the shape parameter α, scale parameters
, and
, also joint shifts in (
), (
) and (
). The first ten cases are classified as ‘single upward shifts’ among which the first five cases are computed assuming (
) and the last five with (
). The last four cases against each smoothing parameter denote the ‘double upward shift’ when (
).
Table 1. The in-control run length profile of MEWMA chart for κ=3, (α=6, =5,
=6) and
.
Table 2. Zero-state run length profile of MEWMA chart for upward shift domain assuming different shift levels and smoothing parameters.
Table 3. Zero-state run length profile of MEWMA chart for upward shift domain assuming different shift levels and smoothing parameters.
Table considers different smoothing parameters and the decision intervals ‘h’ are obtained as 5.07, 7.39, 11.08 and 23.54 against the desired
of 200, respectively. Similarly the ‘h’ against the desired
of 370, are 7.04, 10.54, 16.52 and 34.56. Lastly ‘h’ against the desired
of 500 are 8.15, 12.61, 19.69, and 41.00 for the aforementioned smoothing parameters. Assuming
and
, as the value of (
) increases for both cases of ‘single upward shifts’ and ‘double upward shifts’ the ARL, MRL, and SDRL decrease. Also, increasing the smoothing parameter shows the same pattern, i.e. the ARL decreases. However, the trend changes for
and
as the value of (
) increases. The ARL, MRL, and SDRL decrease gradually but for ‘single upward shifts’ with an increase in (
), firstly the values of ARL, MRL, and SDRL decrease, but after a certain point, e.g.
or
, the desired quantities (i.e. ARL, MRL and SDRL) follow increasing-decreasing pattern. Table shows that all the results are unbiased as
except for
when
and
.
Table lists the results for where the decision intervals ‘h’ are obtained as 32.35, 40.23 and 41.83 against the desired
of 200, respectively. Similarly the ‘h’ against the desired
and 500 are listed in the table. The pattern of run length profiles with
for ‘single and double upward shifts’ is similar as obtained when
0.05. For a ‘single upward shift’ of 20%, i.e.
with
and
, the ARL is 32.87% smaller than the
. The SDRL is 133.63 and MRL is 93.0, which is smaller than the corresponding
.
Tables and tabulate the results for hybrid shifts for and
, respectively. The first five rows of each of the tables list results for
and the last five for
. As the values of ‘
’ decrease, the corresponding ARL, MRL, and SDRL increase. As ‘
’ increases, the ARL, MRL, and SDRL decrease gradually except for some cases like for
. For
, the ARL, MRL, and SDRL first increase and then decrease. For example, considering
with
,
, the
and for
, the
. After this, the ARL again follows a decreasing pattern for
which results in
.
Table 4. Zero-state run length profile of MEWMA chart for hybrid shift domain assuming different shift levels and smoothing parameters.
Table 5. Zero-state run length profile of MEWMA chart for hybrid shift domain assuming different shift levels and smoothing parameters.
Tables and list the results for ‘single downward shifts’ and ‘double downward shifts’. Considering and (
) decreases the ARL, MRL, and SDRL also decrease except for (
)=(1,0.95) and (0.95,1). By introducing decreasing shifts in the mean vector with
, the ARL, MRL, and SDRL first increase and then decrease. For
, the ARL, MRL, and SDRL increase as the shift levels in the mean vector decrease. The ARL, MRL, and SDRL show an increasing pattern when ω=0.02 and ω=0.05 are considered with increasing levels of ‘double downward shifts’. However, this pattern changes for
. For example, assuming
and ω=0.02 for
=(0.68,0.68) the
is 47.98 and for
=(0.81,0.81), the
is 91.60. While assuming
and ω=1.00 for
=(0.68,0.68), the ARL is very large and we marked this in the tables with **. For
=(0.81,0.81), the
is 582.16.
Table 6. Zero-state run length profile of MEWMA chart for downward shift domain assuming different shift levels and smoothing parameters.
Table 7. Zero-state run length profile of MEWMA chart for downward shift domain assuming different shift levels and smoothing parameters.
6.5. Run length profiles of steady state case
The previous section discussed the zero-state RL profile of the proposed chart. Contrary to the previous section, this section presents results and a discussion of the steady-state RL profile assuming r = 100. Tables – list the results for ‘single upward shifts’, ‘hybrid shifts’ and ‘single downward shifts’, respectively. By considering the resulting decision intervals ‘h’ are
,
, and
for
= 200, 370, and 500, respectively. For ‘single upward shifts’ given in Table , i.e.
=(1.40,1) and
=(1,5) the results are significant for small smoothing parameters like for
. For example, consider
,
and
=(1.40,1), the
for the steady state is 58.41, MRL = 50.00 and SDRL = 44.42 which is less than the
of zero state
which is 62.80. However, for
0.5, 0.8,1, the results become less significant for steady-state as the
of steady-state becomes larger than
of zero-state. The results reported in Table follow a similar pattern for hybrid shifts too. Table presents the steady-state ARLs which are smaller than the results listed for zero-state with few exceptions for
and
0.5, 0.8, and 1.00.
Table 8. Zero-state and steady state run length profile comparison of MEWMA chart for upward shift domain.
Table 9. Zero-state and steady state run length profile comparision of MEWMA chart for hyrid shift domain.
Table 10. Zero-state and steady state run length profile comparision of MEWMA chart for downward shift domain.
From Tables , it is evident that the proposed chart is good at detecting upward, hybrid, and downward shifts with a small smoothing parameter.
A proper selection of the smoothing parameter for shift detection and specification of control limits to achieve the desired is crucial. Considering the zero-state performance of the proposed chart, if ‘single or double upward shifts’ are introduced to the mean vector then only one case of
with
is obtained biased while all the rest results are unbiased. For hybrid shift detection, all results are unbiased except for one case in which the ARLs become too large for
.
If a small downward shift either ‘single or double downward shift’ is introduced to the mean vector with a small value of the smoothing parameter like ω = 0.02 and 0.05, then unbiased ARLs are obtained for downward shifts and biased ARLs are obtained for relatively large downward shifts and smoothing parameters.
In the steady-state case, most of the ARL results are unbiased up to for upward and hybrid shifts detection. For downward shifts, all of the steady state ARLs are smaller than the zero-state ARLs except few cases with
and
.
6.6. Performance analysis using EARL
The performance of the control chart is usually evaluated based on its run length profiles. However, an advanced specification of a shift size is required when we use ARL as a performance evaluation criterion. This constraint makes the process evaluation even more difficult if one does not have background knowledge of a shift in the process. Thus, along with ARL the performance of the proposed chart is evaluated using the expected average run length (EARL). The EARL of the MEWMA control chart is
(23)
(23) were δ is the process shift and the probability density function of the process shift is denoted by
.
(24)
(24) Assuming uniform distribution for different shifts, Equation (Equation24
(24)
(24) ) can be written as
(25)
(25)
Table presents the EARL of the MEWMA chart assuming different shift levels and smoothing parameters. The results show that for upward shifts, as the smoothing parameter increases from 0.02 to 0.1 the EARL decreases. The same is observed for the hybrid shifts. However, for it is noticed that as the smoothing parameter increases, the EARL also increases.
Table 11. Zero-state EARL of MEWMA chart assuming different shift levels and smoothing parameters.
7. Illustrative examples
This section presents one illustrative and two real data examples to show the implementation of the proposed chart in practice.
7.1. A simulated data
The IC data set consisting of 25 observations is generated by using GBP(,
,
,
) while the OOC data set is generated by GBP(
,
,
,
) model. The smoothing parameters are
(Table ).
Table 12. Data generating parameters for the simulated data control charts.
Figures – show the MEWMA charts for upward, hybrid, and downward shifts, respectively. In particular, Figure is depicted assuming shifts in the scale parameter ‘’ of the process such that
,
,
. Figure (A) with
shows the first OOC signal at the 41st observation, whereas Figure (B–C) show the first OOC signal at the 38th observation assuming
and
, respectively. Assuming
, Figure (D) shows that a shift is detected at the 41st observation, whereas Figure (E) shows that no OOC signal is detected by the
chart.
Figure 1. Comparison of the MEWMA and control charts for upward shift detection using the GBP data. (A)
(B)
(C)
(D)
(E)
chart (
).

Figure 2. Comparison of the MEWMA and control charts for hybrid shift detection using the GBP data. (A)
(B)
(C)
(D)
(E)
chart (
).

Figure 3. Comparison of the MEWMA and control charts for downward shift detection using the GBP data. (A)
(B)
(C)
(D)
(E)
chart (
).

Next, Figure depicts the charts for hybrid shifts, i.e. ,
, and
. Figure (A–B) show that the first OOC is detected for both
and
at the 29th observation. Figure (C–D) show the chart detect a shift signal at the 42nd observation for
and
, respectively. Figure (E) depicts the detection of a hybrid shift at the 43rd point for
chart assuming
.
Lastly, Figure shows the charts for a downward shift in the scale parameter ‘’ of the process such that
,
,
. Figure (A) depicts that with
, the first OOC signal is detected at the 35th observation. Figure (B–D) show that a shift is detected at the 34th point assuming
,
, and
, respectively. Figure (E) shows that the
chart with
detects no OOC signal.
Figures – compare the performance of the proposed MEWMA chart for the GBP data assuming with the
chart with
and the results show that the proposed chart outperforms the
chart to detect an OOC signal.
7.2. Real life examples
7.2.1. Patient relief time example
To explore the application of the MEWMA control chart, a data set of patient relief time is taken from [Citation61]. Ten patients received both standard and new treatments for headaches. The relief time paired data (in minutes) are (8.40, 6.90), (7.70, 6.80), (10.10, 10.30), (9.60, 9.40), (9.30, 8.00), (9.10, 8.80), (9.00, 6.10), (7.70, 7.40), (8.10, 8.00), and (5.30, 5.10). For the plausibility of Pareto Type II (Lomax) marginal distributions, a transformation is made by subtracting 5.0 from each point [p. 509][Citation61], and then a GBP model is fitted. Moreover, ten observations are very few to enable us to detect an OCC signal. Therefore, the estimated parameters are used to simulate 50 values for the monitoring purpose. In particular, 25 observations are used as phase I and the remaining 25 as phase II. The parameters are estimated by the method of moment estimation (MME), which are ,
,
and the parameter ‘κ’ is estimated by using the method of moments based on Spearman's rho which is
with standard error of 1.27. For the MEWMA chart,
with
while
and ‘h’
for the
chart. An upward shift is introduced as
,
, and
. Figure (A–C) show a signal of the upward shift is detected by the proposed MEWMA chart at the 29th observation by considering
,
, and
, respectively. However, Figure (D) shows that the
chart detects the upward shift at the 38th observation. Thus, the proposed MEWMA chart outperforms the
chart.
7.2.2. Air quality example
The air quality data set is used to show the implementation of the proposed chart. Air pollution badly affects the quality of air. The motor vehicle emissions, gases released from power plants, smoke coming from the burning of coal or wood, and fumes from chemical products like carbon dioxide (), nitrogen oxides (
), sulfur dioxide (
), carbon monoxide (
), etc., are significant air pollutants and have adverse effects on human health, animals, all living beings and environment. Thus, improvement of air quality by timely monitoring and control of increasing air pollutants is necessary.
The air quality data set contains average responses registered every hour from an array of five metal oxide chemical sensors inserted in an air quality chemical multi-sensor instrument. From the observed 13 quality variables, only two variables of interest are considered here. For detailed information about the quality variables, we refer to [Citation62] (https://archive.ics.uci.edu/ml/datasets/Air+quality).
Non-methane hydrocarbons and nitrogen dioxide concentration in the air can cause headaches, breathing difficulties, loss of consciousness, vomiting, severe eye skin irritation, heart irregularity, brain damage, and even death. The true hourly averaged overall Non-methane hydrocarbons (NMHCs) concentration (in microg/) and true hourly averaged nitrogen dioxide (
) concentration (in microg/
) are denoted as
and
variables, respectively. By excluding the missing values, 30 values are taken from the data set to implement the chart.
Using the data set reported in Table , the parameters are estimated by using the MME. The estimated parameters are ,
,
. However, the estimator proposed by Lu and Bhattacharyya [Citation54] is used to estimate the dependence parameter
(26)
(26) Thus,
. Using these estimated parameters, 50 observations are generated in which the first 25 observations are phase I and the last 25 observations are phase II. In particular, the phase II observations are generated using the shape parameter
and scale parameters
,
. For the MEWMA chart, Figure (A), we used
and the decision interval ‘h’
. The first OOC signal is observed at the 28th point. Figure (B) assumed
with decision interval ‘h’
and the OOC signal is detected at the 29th observation. From Figure (C) with
and ‘h’
, the OOC signal is detected at the 28th observation. Figure (D) presents
chart assuming
and decision interval ‘h’
. This chart detects a single downward shift at the 43rd observation. Thus the proposed chart is better than the
chart.
Figure 5. Comparison of the MEWMA chart and chart using for the air quality data set. (A)
(B)
(C)
(D)
chart (
).

Table 13. Air quality data set.
8. Multivariate extension of the GBP model
The GBE model was extended to the Gumbel multivariate exponential (GME) model by Xie et al. [Citation34]. Similarly, one can extend the Gumbel bivariate Pareto (GBP) model to the Gumbel multivariate Pareto (GMP) model. If the random variables , an extension to the survival function from bivariate to the multivariate case can be given as follows.
(27)
(27) where
are the shape parameters
are the scale parameters and the dependence parameter κ lies between
. The marginal distribution of (
) follows univariate Pareto Type II (Lomax) distribution with ‘α’ as the shape parameter and
as the scale parameters. The mean vector of (
) is denoted by
as given below
(28)
(28) and the variance-covariance matrix of (
) is
(29)
(29)
The correlation coefficient (i, k ∈ 1, 2,…, p and i≠k) of any combination of (
) is independent of i and k [Citation34].
(30)
(30) Thus, the present framework can be easily extended to multivariate case.
9. Conclusion
The study proposed an efficient MEWMA control chart for monitoring Gumbel's bivariate Pareto type II (Lomax) TBE data. The performance of the control chart is assessed for both zero-state and steady-state with the help of run length profiles including ARL, SDRL, and MRL based on Monte Carlo simulations. The implementation of the control chart in real-life scenarios is also discussed. Different mean shifts are used to study the performance of the chart. The study results indicated that our proposed chart performed well for the steady-state case as most of the of the steady-state case are less than the
s of the zero-state case. The real data applications show that the proposed chart is more efficient than the
chart. Hence, the proposed chart outperforms the Shewhart
chart in both simulations as well as real data cases.
It is worth mentioning that a signal obtained by a multivariate chart should be properly identified, although one can argue that the purpose of a joint chart is not to detect the changes in the individual quality characteristics. For the identification of shifts, one proposal is to use separate charts for each marginal by compromising the correlation. The second proposal is based on the idea of [Citation63] by using the likelihood ratio test.
This research work can be extended to other multivariate continuous as well as discrete distributions like bivariate Levy and bivariate negative binomial distributions. Also, biased behaviour of the ARL is noticed in a few cases and an unbiased design can be proposed in the future. Furthermore, new and advanced multivariate control charts for monitoring the mean and dispersion of the process simultaneously can be constructed.
Acknowledgments
The authors would like to thanks the comments and suggestions of the anonymous reviewers and editor to improve the presentation of the work.
Disclosure statement
No potential conflict of interest was reported by the author(s).
Data availability statement
Data sharing is not applicable to this article as no new data were created or analysed in this study.
References
- Xie M, Goh TN, Kuralmani V. Statistical models and control charts for high-quality processes. New York, NY: Springer Science & Business Media; 2002.
- Chan L, Xie M, Goh TN. Cumulative quantity control charts for monitoring production processes. Int J Prod Res. 2000;38(2):397–408. doi: 10.1080/002075400189482
- Borror CM, Keats JB, Montgomery DC. Robustness of the time between events CUSUM. Int J Prod Res. 2003;41(15):3435–3444. doi: 10.1080/0020754031000138321
- Liu J, Xie M, Goh T, et al. A comparative study of exponential time between events charts. Qual Technol Quant Manag. 2006;3(3):347–359. doi: 10.1080/16843703.2006.11673120
- Zhang C, Xie M, Liu J, et al. A control chart for the gamma distribution as a model of time between events. Int J Prod Res. 2007;45(23):5649–5666. doi: 10.1080/00207540701325082
- Ali S, Pievatolo A, Göb R. An overview of control charts for high-quality processes. Qual Reliab Eng Int. 2016;32(7):2171–2189. doi: 10.1002/qre.v32.7
- Ali S. Time-between-events control charts for an exponentiated class of distributions of the renewal process. Qual Reliab Eng Int. 2017;33(8):2625–2651. doi: 10.1002/qre.v33.8
- Ara J, Ali S, Shah I. Monitoring schedule time using exponentially modified Gaussian distribution. Qual Technol Quant Manag. 2020;17(4):448–469. doi: 10.1080/16843703.2019.1668164
- Ali S, Shah I, Wang L, et al. A comparison of Shewhart-type time-between-events control charts based on the renewal process. IEEE Access. 2020;8:113683–113701. doi: 10.1109/Access.6287639
- Li J, Yu D, Song Z, et al. Comparisons of some memory-type control chart for monitoring Weibull-distributed time between events and some new results. Qual Reliab Eng Int. 2022;38(7):3598–3615. doi: 10.1002/qre.v38.7
- Hu X, Qiao Y, Zhou P, et al. Modified one-sided EWMA charts for monitoring time between events. Commun Statist–Simul Comput. 2023;52(3):1041–1056. doi: 10.1080/03610918.2021.1872632
- Rizzo C, Di Bucchianico A. Generalized likelihood ratio control charts for high-purity (high-quality) processes. Qual Reliab Eng Int. 2023;39(2):523–531. doi: 10.1002/qre.v39.2
- Mason RL, Young JC. Multivariate statistical process control with industrial applications. Philadelphia, PA: SIAM; 2002.
- Montgomery DC. Introduction to statistical quality control. 8th ed. New York: John Wiley & Sons; 2019.
- Hotelling H. Multivariate quality control illustrated by air testing of sample bombsights. In: Eisenhart C, Hastay MW, and Wallis WA, editors. Techniques of statistical analysis. New York: McGraw Hill; 1947. p. 111–184.
- Lowry CA, Woodall WH, Champ CW, et al. A multivariate exponentially weighted moving average control chart. Technometrics. 1992;34(1):46–53. doi: 10.2307/1269551
- Crosier RB. Multivariate generalizations of cumulative sum quality-control schemes. Technometrics. 1988;30(3):291–303. doi: 10.1080/00401706.1988.10488402
- Pignatiello Jr JJ, Runger GC. Comparisons of multivariate CUSUM charts. J Qual Technol. 1990;22(3):173–186. doi: 10.1080/00224065.1990.11979237
- Cheng SW, Thaga K. Multivariate Max-CUSUM chart. Qual Technol Quant Manag. 2005;2(2):221–235. doi: 10.1080/16843703.2005.11673095
- Golosnoy V, Ragulin S, Schmid W. Multivariate CUSUM chart: properties and enhancements. AStA Adv Statist Anal. 2009;93(3):263–279. doi: 10.1007/s10182-009-0107-4
- Lee MH, Khoo MBC, Xie M. An optimal control procedure based on multivariate synthetic cumulative sum. Qual Reliab Eng Int. 2014;30(7):1049–1058. doi: 10.1002/qre.v30.7
- Yeh AB, Lin DK, McGrath RN. Multivariate control charts for monitoring covariance matrix: a review. Qual Technol Quant Manag. 2006;3(4):415–436. doi: 10.1080/16843703.2006.11673124
- Bersimis S, Psarakis S, Panaretos J. Multivariate statistical process control charts: an overview. Qual Reliab Eng Int. 2007;23(5):517–543. doi: 10.1002/qre.v23:5
- Zou C, Tsung F. A multivariate sign ewma control chart. Technometrics. 2011;53(1):84–97. doi: 10.1198/TECH.2010.09095
- Tang A, Sun J, Hu X, et al. A new nonparametric adaptive EWMA control chart with exact run length properties. Comput Ind Eng. 2019;130:404–419. doi: 10.1016/j.cie.2019.02.045
- Zhou M, Zhou Q, Geng W. A new nonparametric control chart for monitoring variability. Qual Reliab Eng Int. 2016;32(7):2471–2479. doi: 10.1002/qre.v32.7
- Yue J, Liu L. Multivariate nonparametric control chart with variable sampling interval. Appl Math Model. 2017;52:603–612. doi: 10.1016/j.apm.2017.08.005
- Kittlitz Jr RG. Transforming the exponential for SPC applications. J Qual Technol. 1999;31(3):301–308. doi: 10.1080/00224065.1999.11979928
- Chang YS, Bai DS. A multivariate t2 control chart for skewed populations using weighted standard deviations. Qual Reliab Eng Int. 2004;20(1):31–46. doi: 10.1002/qre.v20:1
- Chang YS. Multivariate CUSUM and EWMA control charts for skewed populations using weighted standard deviations. Commun Statist–Simul Comput. 2007;36(4):921–936. doi: 10.1080/03610910701419596
- Stoumbos ZG, Sullivan JH. Robustness to non-normality of the multivariate EWMA control chart. J Qual Technol. 2002;34(3):260–276. doi: 10.1080/00224065.2002.11980157
- Testik MC, Runger GC, Borror CM. Robustness properties of multivariate EWMA control charts. Qual Reliab Eng Int. 2003;19(1):31–38. doi: 10.1002/qre.v19:1
- Gumbel EJ. Bivariate exponential distributions. J Am Stat Assoc. 1960;55(292):698–707. doi: 10.1080/01621459.1960.10483368
- Xie Y, Xie M, Goh TN. Two MEWMA charts for Gumbel's bivariate exponential distribution. J Qual Technol. 2011;43(1):50–65. doi: 10.1080/00224065.2011.11917845
- Xie F, Sun J, Castagliola P, et al. A multivariate CUSUM control chart for monitoring Gumbel's bivariate exponential data. Qual Reliab Eng Int. 2021;37(1):10–33. doi: 10.1002/qre.v37.1
- Xie F, Mukherjee A, Sun J, et al. A synthetic multivariate exponentially weighted moving average scheme for monitoring of bivariate gamma distributed processes. Qual Reliab Eng Int. 2022;38(2):848–876. doi: 10.1002/qre.v38.2
- Asrabadi BR. Estimation in the Pareto distribution. Metrika. 1990;37:199–205. doi: 10.1007/BF02613522
- Kleiber C, Kotz S. Statistical size distributions in economics and actuarial sciences. Hoboken, New Jersey: John Wiley & Sons; 2003.
- Nadeau TP, Teorey TJ. A Pareto model for OLAP view size estimation. Inf Syst Frontiers. 2003;5:137–147. doi: 10.1023/A:1022693305401
- Cheng C, Chen J, Bai J. Exact inferences of the two-parameter exponential distribution and Pareto distribution with censored data. J Appl Stat. 2013;40(7):1464–1479. doi: 10.1080/02664763.2013.788613
- Hong C-W, Wu J-W, Cheng C-H. Implementing lifetime performance index for the pareto lifetime businesses of the service industries. Qual Quant. 2009;43:291–304. doi: 10.1007/s11135-007-9110-6
- Kuş C, Kaya MF. Estimation for the parameters of the Pareto distribution under progressive censoring. Commun Statist–Theory Methods. 2007;36(7):1359–1365. doi: 10.1080/03610920601077089
- Ouyang L-Y, Wu S-J. Prediction intervals for an ordered observation from a Pareto distribution. IEEE Trans Reliab. 1994;43(2):264–269. doi: 10.1109/24.295005
- Parsi S, Ganjali M, Farsipour NS. Simultaneous confidence intervals for the parameters of Pareto distribution under progressive censoring. Commun Statist–Theory Methods. 2009;39(1):94–106. doi: 10.1080/03610920802687785
- Soliman AA. Bayes prediction in a pareto lifetime model with random sample size. J R Statist Soc Ser D: The Statistician. 2000;49(1):51–62. doi: 10.1111/1467-9876.00178
- Wong A. Approximate studentization for pareto distribution with application to censored data. Statist Papers. 1998;39:189–201. doi: 10.1007/BF02925406
- Wu S-F. Interval estimation for a Pareto distribution based on a doubly type II censored sample. Comput Stat Data Anal. 2008;52(7):3779–3788. doi: 10.1016/j.csda.2007.12.015
- Wu S-J, Chang C-T. Inference in the Pareto distribution based on progressive type II censoring with random removals. J Appl Stat. 2003;30(2):163–172. doi: 10.1080/0266476022000023721
- Ismaïl S. A simple estimator for the shape parameter of the Pareto distribution with economics and medical applications. J Appl Stat. 2004;31(1):3–13. doi: 10.1080/0266476032000148911
- Krishnaji N. Characterization of the Pareto distribution through a model of underreported incomes. Econometrica: Journal of the Econometric Society. 1970;38:251–255. doi: 10.2307/1913007
- Guo B-C, Wang B-X. Control charts for the Pareto distribution. Appl Math–A J Chinese Universities. 2015;30(4):379–396. doi: 10.1007/s11766-015-3355-y
- Nil N, Kraleti SR, Kambagowni VS. Optimal design of-control chart with Pareto in-control times. Int J Adv Manuf Technol. 2010;48(9-12):829–837. doi: 10.1007/s00170-009-2348-5
- Seif A, Faraz A, Sadeghifar M. Evaluation of the economic statistical design of the multivariate t2 control chart with multiple variable sampling intervals scheme: NSGA-II approach. J Stat Comput Simul. 2015;85(12):2442–2455. doi: 10.1080/00949655.2014.931404
- Lu J-C, Bhattacharyya GK. Inference procedures for bivariate exponential model of Gumbel. Stat Probab Lett. 1991;12(1):37–50. doi: 10.1016/0167-7152(91)90162-K
- Arnold B.C. Pareto distributions. Boca Raton, FL: CRC Press Taylor & Francis Group; 2015.
- Lu J-C, Bhattacharyya GK. Inference procedures for a bivariate exponential model of gumbel based on life test of component and system. J Stat Plan Inference. 1991;27(3):383–396. doi: 10.1016/0378-3758(91)90051-F
- Dickinson RM, Roberts DAO, Driscoll AR, et al. CUSUM charts for monitoring the characteristic life of censored Weibull lifetimes. J Qual Technol. 2014;46(4):340–358. doi: 10.1080/00224065.2014.11917976
- Szarka III JL, Woodall WH. On the equivalence of the bernoulli and geometric cusum charts. J Qual Technol. 2012;44(1):54–62. doi: 10.1080/00224065.2012.11917881
- Xu S, Jeske DR. Weighted EWMA charts for monitoring type I censored Weibull lifetimes. J Qual Technol. 2018;50(2):220–230. doi: 10.1080/00224065.2018.1436830
- Wu Z, Spedding TA. Evaluation of ATS for CRL control chart. Process Control Qual. 1999;11(3):183–191. doi: 10.1163/156856699750248540
- Gross AJ, Lam CF. Paired observations from a survival distribution. Biometrics. 1981;37(3):505–511. doi: 10.2307/2530563
- De Vito S, Massera E, Piga M, et al. On field calibration of an electronic nose for benzene estimation in an Urban pollution monitoring scenario. Sensors Actuators B: Chemical. 2008;129(2):750–757. doi: 10.1016/j.snb.2007.09.060
- McCracken AK, Chakraborti S, Mukherjee A. Control charts for simultaneous monitoring of unknown mean and variance of normally distributed processes. J Qual Technol. 2013;45(4):360–376. doi: 10.1080/00224065.2013.11917944