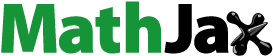
ABSTRACT
Embracing the Bayesian approach, we aimed to synthesise evidence regarding barriers and enablers to physical activity in adults with heart failure (HF) to inform behaviour change intervention. This approach helps estimate and quantify the uncertainty in the evidence and facilitates the synthesis of qualitative and quantitative studies. Qualitative evidence was annotated using the Theoretical Domains Framework and represented as a prior distribution using an expert elicitation task. The maximum a posteriori probability (MAP) for the probability distribution for the log OR was used to estimate the relationship between physical activity and each determinant according to qualitative, quantitative, and qualitative and quantitative evidence combined. The probability distribution dispersion (SD) was used to evaluate uncertainty in the evidence. Three qualitative and 16 quantitative studies were included (N = 2739). High pro-b-type natriuretic peptide (MAP = −1.16; 95%CrI: [−1.21; −1.11]) and self-reported symptoms (MAP = − 0.48; 95%CrI: [ −0.40; −0.55]) were suggested as barriers to physical activity with low uncertainty (SD = 0.18 and 0.19, respectively). Modifiable barriers were symptom distress (MAP = −0.46; 95%CrI: [−0.68; −0.24], SD = 0.36), and negative attitude (MAP = −0.40; 95%CrI: [−0.49; −0.31], SD = 0.26). Modifiable enablers were social support (MAP = 0.56; 95%CrI: [0.48; 0.63], SD = 0.26), self-efficacy (MAP = 0.43; 95%CrI: [0.32; 0.54], SD = 0.37), positive physical activity attitude (MAP = 0.92; 95%CrI: [0.77; 1.06], SD = 0.36).
Introduction
Heart Failure (HF) is a complex clinical syndrome of symptoms that suggest reduced efficiency with which the heart pumps blood around the body (National Institute for Healthcare and Excellence, Citation2018). It is a prevalent condition worldwide, affecting 2% of the general adult population (Groenewegen et al., Citation2020). The condition affects older adults, with the prevalence rising to 6.6% among individuals aged 65 and over and to 13.5% among individuals aged 80 and over (Mozaffarian et al., Citation2015). HF is a debilitating condition characterised by symptoms of peripheral water retention (i.e., oedema), breathlessness, and fatigue. Despite advances in medical treatment, HF is a major cause of morbidity and mortality (Bragazzi et al., Citation2021).
Physical activity is associated with improved quality of life (Davies et al., Citation2010; Lewinter et al., Citation2015; Sagar et al., Citation2015; Taylor et al., Citation2019), reduced hospitalisation (Sagar et al., Citation2015) and increased longevity (Belardinelli et al., Citation2012; ExTraMATCH Collaborative, Citation2004) in individuals living with HF. Therefore, regular physical activity is a key component of recommended treatment (Ponikowski et al., Citation2016). While the minimal clinically important difference in physical activity levels in HF is not known (Dibben et al., Citation2018; Shoemaker et al., Citation2013), the recommendation for older adults, in general, is to perform a minimum of 75–150 minutes per week of moderate to vigorous intensity aerobic physical activity; and engage in functional balance and muscle strength training at a moderate intensity at least three and two days a week, respectively (WHO, Citation2020).
A structured form of physical activity, exercise, is included in cardiac rehabilitation (CR) and is offered to newly diagnosed HF patients (National Institute for Healthcare and Excellence, Citation2018). However, uptake of CR programmes is less than 1% among individuals diagnosed with HF (Doherty & Harrison, Citation2017). Levels of everyday physical activity in HF are also low (Jaarsma et al., Citation2013; O’Donnell et al., Citation2020), partially due to the many challenges individuals with HF face in initiating and maintaining a physically active lifestyle, as proposed by the European Society of Cardiology (Conraads et al., Citation2012). Understanding how best to improve physical activity in individuals living with HF is warranted.
Interventions informed by a behaviour change theory are potentially promising in achieving physical activity improvements in HF (Tierney et al., Citation2011b). A recent meta-analysis found that interventions integrating an exercise programme with behaviour change theory and interventions delivered by a physiotherapist are efficacious in obtaining short-term improvements in physical activity levels among individuals living with HF (Amirova et al., Citation2021). However, the effect of these interventions varied considerably. In addition, the extent to which a theory informed interventions was limited (Amirova et al., Citation2021). Research on the barriers and enablers influencing physical activity is needed as it may inform the choice of behaviour change theory and intervention development.
Guidelines for developing behaviour change interventions recognise that the modifiable and contextual barriers and enablers need to be systematically identified and described to inform intervention design (Araújo-Soares et al., Citation2019; Hagger et al., Citation2020; Kok et al., Citation2016; Michie et al., Citation2011; O’Cathain, Croot, Duncan, et al., Citation2019). Knowledge about relevant determinants increases the intervention's chances to be effective and conserves research effort and resources (Craig et al., Citation2008; O’Cathain, Croot, Sworn, et al., Citation2019). Systematically identified evidence concerning modifiable and contextual barriers and enablers can guide theory choice and therefore inform behaviour change intervention design.
However, the factors influencing physical activity participation in individuals living with HF are not well understood. A systematic review of qualitative studies found a lack of research on individual accounts of barriers and enablers to physical activity in individuals living with HF (Tierney et al., Citation2011b). The review reported sparse summaries about physical activity extracted from the studies that elucidated beliefs and personal accounts of living with HF in general, including physical activity only as one of many themes (Tierney et al., Citation2011b). The following enablers: knowledge of risks and benefits associated with physical activity (e.g., reduced mortality and morbidity, and improved quality of life); confidence in one's ability to engage in physical activity; anticipated outcomes of physical activity; and social support, as well as a barrier such as weather, were previously identified in a systematic narrative review (Tierney et al., Citation2011b). However, these barriers and enablers have not been confirmed in quantitative studies. The review highlighted the need to explore further what influences physical activity in HF (Tierney et al., Citation2011b).
A recent paper called for the adoption of Bayesian statistics in Health Psychology research (Beard & West, Citation2017; Depaoli et al., Citation2017; Hamilton et al., Citation2017; Heino et al., Citation2018). This approach may also be useful in understanding the contextual and modifiable determinants influencing physical activity in HF. The Bayesian approach views evidence synthesis as a decision-making process (Roberts et al., Citation2002); new evidence is considered in light of existing evidence, beliefs, and practices. Beliefs are often presented in the form of qualitative research. Qualitative research is readily available from research studies on health and health management; however, its findings are not utilised in healthcare decision-making and policy development (Roberts et al., Citation2002). Qualitative research provides rich data, but the required formal systematic evaluation impedes the inclusion of qualitative evidence in decision-making and policy development (Roberts et al., Citation2002). This makes it difficult for qualitative evidence to inform policy-making (Roberts et al., Citation2002). It is also recommended to account for stakeholders’ needs – the needs of those living with HF in this instance – in research concerning intervention development (Craig et al., Citation2008). While Bayesian methods provide an opportunity to incorporate qualitative evidence in decisions about health management (Spiegelhalter et al., Citation2003). Therefore, Bayesian methods are useful when evidence from diverse sources needs to be synthesised.
However, to perform Bayesian synthesis, qualitative research should be formally and systematically catalogued before it can be integrated with quantitative findings, which is often not straightforward (Roberts et al., Citation2002). The Theoretical Domains Framework (Cane et al., Citation2012) is a tool developed through an international collaborative effort that systematically describes domains and constructs that may influence behaviour under investigation. In the current study, the identified physical activity barriers and enablers in HF were categorised in accordance with the TDF. In addition, the COM-B model, developed from a systematic synthesis of behaviour change frameworks (Michie et al., Citation2011), was used to inform future behaviour change interventions targeting physical activity in HF. In particular, following the consensus on the link between barriers and enablers, the intervention’s proposed mechanisms and strategies (Connell et al., Citation2019), and several behaviour change techniques (BCTTv1: Michie et al., Citation2013) that are likely to enhance the identified relevant enablers or reduce the barriers were proposed.
Objectives
The present review with meta-analysis aims to systematically integrate qualitative and quantitative evidence on the clinical, environmental, and psychosocial barriers and enablers influencing physical activity in those living with HF. The secondary aim, which is a response to the recent call to embrace the Bayesian approach (Beard & West, Citation2017; Depaoli et al., Citation2017; Hamilton et al., Citation2017; Heino et al., Citation2018), is to apply the Bayesian approach in synthesising evidence regarding barriers and enablers to physical activity in HF in a way that can inform behaviour change intervention development.
Method
The systematic review with meta-analysis was implemented adhering to guidance on conducting systematic reviews and meta-analyses of observational studies of aetiology, COSMOS-E (Dekkers et al., Citation2019). The review is reported following PRISMA 2020 guidelines (Page et al., Citation2021). The review's protocol was registered on PROSPERO: CRD42021232048.
Eligibility criteria
Qualitative and observational studies investigating any clinical, environmental, social, or psychological barriers and enablers to physical activity in adults diagnosed with HF were included in this review (supplement 1). Physical activity was defined as any bodily movement that requires metabolic energy expenditure (WHO, Citation2020), of any mode (e.g., walking); any intensity (e.g., moderate to vigorous); in any setting (as exercise prescription or otherwise). For practical reasons, the search results were further restricted to peer-reviewed articles in English.
Information sources
A total of 14 online databases were searched from inception to 05 January 2020 (Embase, Global Health, HMIC Health Management Information Consortium, MEDLINE; PsychINFO; CINAHL; Health policy reference centre; PsychARITCLES; PubMed; The Cochrane Library; Academic search complete, Pedro). The reference lists of the obtained articles included at full-text screening were hand searched for relevant studies meeting the inclusion criteria. In addition, ClinicalTrial.gov was searched for observational studies but yielded no results.
Search strategy
The MeSH terms and keywords describing the Population of interest (i.e., HF and nine synonyms combined using a Boolean operator ‘OR’) and Outcome of interest (i.e., physical activity and 21 synonyms combined using a Boolean operator ‘OR’) were combined using a Boolean operator ‘AND’ (supplement 2). The initial search yielded 11,678 hits.
Selection process
Two reviewers (AA and LT) independently screened titles and abstracts and selected articles meeting the criteria for full-text screening in Rayyan. Qualitative studies meeting the eligibility criteria informed the prior elicitation task (i.e., appraisal by a panel of experts). Quantitative studies were included in the frequentist meta-analysis.
Data collection process
Two reviewers (AA and LT) independently extracted relevant data items from the reports of the included studies.
Data items
The Strengthening the Reporting of Observational Studies in Epidemiology items, STROBE (von Elm et al., Citation2007) were utilised to design the data extraction form (supplement 3).
Study risk of bias assessment
Two reviewers (AA and LT) independently assessed the study-level risk of bias present in the included quantitative studies. The following sources of bias were considered: selective reporting, participant selection, missing data (including non-respondents and dropouts), confounding (measured and unmeasured confounds; time-varying confounds in cohort studies), and outcome definition and measurement (i.e., information bias) (Dekkers et al., Citation2019). Due to the lack of a comprehensive risk of bias tool designed specifically for observational studies (Page et al., Citation2018), three instruments were used jointly: the Appraisal tool for Cross-Sectional Studies (AXIS), Working Group Item Bank (WGIB), and Risk Of Bias In Non-randomised Studies – of Interventions (ROBIN-I; Page et al., Citation2018; Sanderson et al., Citation2007; Viswanathan et al., Citation2013). The ROBIN-I items concerning the randomisation procedure were omitted; an ‘intervention’ was substituted with ‘exposure’.
Synthesis methods
Bayesian updating is defined as a procedure of updating prior belief by incorporating new information. Degrees of belief about the probability of an event or an outcome, within a Bayesian prediction model, are represented in the form of a prior distribution (Spiegelhalter et al., Citation2003). Thus, prior distribution is an initial belief about a phenomenon. Likelihood distribution for a belief is the extent to which the hypothesis is likely given the newly observed evidence. Bayesian updating is the process by which the prior changes upon consideration of new evidence. The result of Bayesian updating is a new probability distribution representing the updated belief, which is called posterior probability distribution (Spiegelhalter et al., Citation2003).
Bayesian meta-analysis was conducted in RFootnote1 () following methods outlined in (Spiegelhalter et al., Citation2003). Bayesian updating was performed to obtain the log Odds Ratio (log OR) for the association between physical activity and a barrier or enabler (Roberts et al., Citation2002). In this review, qualitative evidence was used to elicit the prior distribution, and quantitative evidence was used to elicit the likelihood. Posterior distribution was obtained by updating the qualitative evidence with the quantitative evidence. Detailed statistical analysis is reported in supplement 3. Parameters for prior and likelihood were sampled from a normal distribution: N(µ, σ2).
Prior distribution
The qualitative evidence was synthesised using the Theoretical Domains Framework, TDF (Cane et al., Citation2012). Three reviewers (AA, BV, AC) independently annotated line-by-line the identified qualitative papers using the TDF. Disagreements were resolved through discussion. Then, based on the findings of the TDF analysis, a prior elicitation task was developed to capture experts’ (n = 6) beliefs about the probability distribution for physical activity conditioned on the constructs identified as relevant in qualitative evidence (i.e., informative prior). The prior elicitation task developed for this review is described in supplement 3. Six reviewers (AA; LT; BV; NA; AC; TFT) completed the expert elicitation task (). The reviewers made a judgement on whether the hypothetical HF patient met the recommended levels of physical activity or not. The log OR distribution was generated from the results of the expert elicitation task (i.e., prior) using the following parameters for the normal distribution: mean (µ) and variance (σ 2). The mean (µ) was the log OR representing the association between a construct being present in a hypothetical scenario describing a patient and the experts’ judgement that the patient was physically active was calculated. The variance (σ2) was the sampling variance of the association between a construct being present in a scenario and experts’ judgement that the hypothetical patient in the scenario is likely to be active.
where µ prior is log OR from the expert elicitation task and
is sampling variance from the expert elicitation task.
Likelihood distribution
Effect sizes reported in the individual papers were converted into log OR using compute.es library in R (Del Re, Citation2020). Meta-analysis was performed by pooling individual studies log ORs in a meta-analysis using metafor library (Viechtbauer, Citation2010). The likelihood distribution was elicited with the following parameters: the mean (µ likelihood) was the pooled log OR across the included quantitative studies; and the variance was the sampling variance across the included studies.
where µ likelihood is pooled log OR from the meta-analysis including quantitative studies, and
is sampling variance from the meta-analysis including quantitative studies.
Posterior distribution
The findings of the prior elicitation task were updated with quantitative evidence concerning each barrier/enabler (i.e., likelihood) using the formula for the Bayesian updating of a normal distribution (p63. Spiegelhalter et al., Citation2003):
where mean is: and variance is:
.
Summary measures
Standardised mean differences (SMD) were estimated to describe the impact of exposure on the levels of physical activity as follows: (a) cross-sectional assessment of the differences between the group presenting with a characteristic and the group not presenting with a characteristic (e.g., female = 1; male = 0); (b) pre- post-assessment of physical activity in a cohort study before and after an event of interest (e.g., SMD between physical activity outcome before surgery and after surgery); cross-sectional assessment of differences between exercise compliant and non-compliant participants on a range of continuous variables (e.g., SMD in self-efficacy between compliers and non-compliers). The r-z transformation was applied in the frequentist meta-analysis of coefficients to mitigate heterogeneity in measurements across studies. The Hartung-Knapp (Sidik-Jonkman) adjustment was made for the evaluation to mitigate small sample size bias (van Aert & Jackson, Citation2019). compute.es library in R was used to convert effect sizes reported in each included study into log OR.
For the Bayesian meta-analysis, the expected value for the log OR according to the expert elicitation task, quantitative evidence, and the posterior (qual + QUANT) were calculated. MAP and CrI as a summary statistic. The dispersion in the probability distribution for log OR associated with each barrier enabler was used to evaluate the level of uncertainty in the evidence in support of that barrier and enabler. The dispersion (i.e., standard deviation, SD) was interpreted relative to the dispersion in the probability distribution for physical activity in the general HF population (i.e., hyperprior) elicited from a large international study (Jaarsma et al., Citation2013) (see GitHub repositoryFootnote2). The SD> 0.70 corresponds to wide dispersion, 0.69–0.21 to medium dispersion, and SD <0.20 corresponds to narrow dispersion.
Applying findings to intervention development
The identified barriers and enablers in qualitative and quantitative evidence were mapped onto the TDF (Atkins et al., Citation2017; Cane et al., Citation2012) and COM-B (Michie et al., Citation2011). Accordingly, several corresponding behaviour change techniques (BCTs) that are likely to enhance these enablers or reduce the barriers were proposed following the consensus on the link between barriers and enablers and the strategies (Connell et al., Citation2019).
Sensitivity analysis
To assess the impact of the qualitative evidence on the findings of this meta-analysis, we performed a sensitivity analysis by excluding the qualitative evidence. In addition, the meta-analysis was stratified by the physical activity outcomes included in the identified studies.
Certainty assessment
In quantifying the uncertainty in the evidence, the width of the distribution dispersion (SD) was used to estimate the level of uncertainty for each barrier or enabler separately. Wide, medium, and narrow dispersion corresponds to high, moderate, and low uncertainty, respectively.
Results
Study selection
The search results are summarised in . A total of 9026 titles and abstracts and 80 full-text articles were screened. Nineteen studies cited in supplement 4 (N = 2739) were included in the review. Studies that might appear to meet the inclusion criteria but were excluded, as well as the reasons for exclusion, are reported in supplement 5.
Study characteristics
Studies were conducted in the United States of America (n = 8), United Kingdom (n = 3), Netherlands (n = 2), Sweden (n = 2), Australia (n = 1), Germany (n = 1), Taiwan (n = 1), and South Korea (n = 1). The majority of the included studies were of a cross-sectional design (n = 7, ). The average sample size for quantitative and empirical qualitative studies were 150 and 17, respectively. Physical activity assessment methods and barriers and enablers assessment methods are reported in supplement 6. The mean age of the participants was 63.44 years old (SD = 8.39, median = 62.15, IQR: [ 59.5; 68]). The Left Ventricular Ejection Fraction (LVEF, %) was moderately low (mean = 34.52%, SD = 9%). Overall, the majority of samples in the included studies were homogeneous.
Table 1. Study characteristics.
Risk of bias in studies
The risk of bias across the included studies is reported in . The low overall risk of bias was present in 12 (75%) studies, moderate – in three (18.75%) studies (Alosco et al., Citation2012; Chien et al., Citation2014; Corvera-Tindel et al., Citation2004), and serious – in one study (Evangelista et al., Citation2001). One (6.25%) study was exposed to a serious risk of reporting bias, as only statistically significant results were reported (Evangelista et al., Citation2001). A total of nine (56.25%) studies did not have a pre-registered protocol, and therefore no information on the bias in the selection of reported results was available. The measurement bias caused by the dichotomisation of the age variable was present in two (12.50%) studies (Evangelista et al., Citation2001; Evangelista et al., Citation2003). Participant selection bias was present in one (6.25%) study (Klompstra et al., Citation2018). Out of four prospective (25%) studies, only one (6.25%) controlled for time-confounding variables by matching participants in exposed and unexposed groups (Moreno-Suarez et al., Citation2019). Only three (18.75%) studies were exposed to a low risk of bias attributed to confounding: two matched participants (i.e., gender and severity of the disease) when assessing differences in exposed and unexposed groups (Evangelista et al., Citation2003; Moreno-Soarez et al., Citation2019), and one measured appropriate confounding variables (Klompstra et al., Citation2018). The study-level risk of bias assessment is reported in supplement 7.
Figure 3. Study-level risk of bias: based on WIB, ROBIN-I, and AXIS items combined into six categories proposed by Page et al., Citation2018 with an addition of the confounding bias described in ROBIN-I.

Results of synthesis
Qualitative evidence
The qualitative evidence synthesis and results are detailed in supplement 3. Overall, the following TDF domains (presented in italics) barriers and enablers influencing physical activity performance by individuals living with HF were found uniquely in qualitative evidence: Social/Professional Role and Identity, Behavioural Regulation, Environmental Context and Resources, Social Influences, and Knowledge according to three included qualitative studies (Tierney et al., Citation2011a; Tierney et al., Citation2011b; Pihl et al., Citation2011). Coded themes included ‘Losing one's social role in daily life’, which was annotated as Social/Professional Role and Identity. It captured how the loss of participants’ social network and position in society negatively influenced their engagement in physical activity (Pihl et al., Citation2011). Another theme from the literature, ‘Need of finding practical solutions in daily life’ (Pihl et al., Citation2011), was coded as Behavioural Regulation and summarised the need for effective problem solving that enables the integration of physical activity in daily life with ease (supplement 3). One study (Tierney et al., Citation2011a) identified the relevance of the following TDF domains: Environmental Context & Resources, Social Influences, Knowledge (supplement 3).
The determinants that were reported by both qualitative and quantitative studies were: age, perceived symptoms of HF, functioning, comorbidity, negative attitude, positive physical activity attitude, social support, and self-efficacy. In qualitative studies, the influence of ageing processes was described as ‘Changing Soma’ (Beliefs about Capabilities) (Tierney et al., Citation2011b). Perceived symptoms of HF were described as ‘Fluctuating health’ (Beliefs about Consequences) which impacted physical activity participation (Tierney et al., Citation2011b). A positive attitude toward physical activity in qualitative studies was described as ‘Mental Outlook’ (Belief about Consequences) (Tierney et al., Citation2011b), negative attitude in response to physical activity was described as ‘Negative emotional responses’, (Emotion/Optimism) (Tierney et al., Citation2011b), social support was described as ‘Interpersonal Influences’ (Social Influences) (Tierney et al., Citation2011b), and self-efficacy as ‘Not believing in one's ability’ (Beliefs about Capabilities) (Pihl et al., Citation2011). These qualitative findings informed the expert elicitation task (supplement 3).
Bayesian meta-analysis results
The distributions for the log ORs for the association between physical activity and the identified barriers and enablers are described in and . The expected values according to qualitative evidence, quantitative evidence and both are summaries in .
Figure 4. The probability distribution for the expected value of the log OR of physical activity conditioned on identified determinants as suggested by the quantitative evidence (QUANT), i.e., likelihood.

Figure 5. Bayesian updating: the probability distribution for the expected value of the log OR of physical activity conditioned on identified determinants according to qualitative evidence (prior expert elicitation task), quantitative evidence alone (likelihood), and qualitative combined with quantitative evidence (qual + QUANT).

Table 2. Summary of the evidence: the expected value for the log OR according to the expert elicitation task, quantitative evidence, and the posterior (qual + QUANT) describing the association between physical activity and identified barriers and enablers.
Contextual factors:
High pro-b-type natriuretic peptide, pro-BNP (MAP value for log OR = −1.16; 95% CrI: [−1.21; −1.11]) was suggested as a barrier to physical activity with narrow distribution dispersion (SD = 0.18). Another contextual barrier with narrow dispersion (SD = 0.19) are self-reported symptoms (MAP value for log OR = 0.48; 95% CrI: [0.40; 0.55]). Contextual barriers with moderate uncertainty judged from the distribution dispersion ranging from 0.26–0.41 were age (years) (MAP value for log OR = −0.29; 95% CrI: [−0.41; −0.18]), comorbidity measured using Charlson Comorbidity Index (MAP value for log OR = −0.62; 95% CrI: [−0.76; −0.48]), depression measured using HADS-D CES-D, and PROMIS-29 (MAP value for log OR = −0.54; 95% CrI: [−0.71; −0.38]), digoxin prescription (MAP value for log OR = −1.06; 95% CrI: [−1.33; −0.79]), high doppler estimated filling pressure (MAP value for log OR = −0.71; 95% CrI: [−0.82; −0.60]), HF duration (MAP value for log OR = −0.95; 95% CrI: [−1.19; −0.71]), Left Atrial Volume index, LAV (MAP value for log OR = −1.12; 95% CrI: [−1.23; −1.01]), and living with partner (MAP value for log OR = −0.37; 95% CrI: [−0.51; −0.24]).
Contextual enablers with medium dispersion (SD ranged from 0.26–1.09) were physical functioning (measured using MOS SF-36, KCCQ; MAP value for log OR = 0.18; 95% CrI: [0.01; 0.36]), high 6-minute walking test result (6MWT; MAP value for log OR = 1.77; 95% CrI: [1.00; 2.54]), having an implantable device (left ventricular assistant device, LVAD; MAP value for log OR = 1.98; 95% CrI: [1.60; 2.36]), renal function (glomerular filtration rate (ml/min); MAP value for log OR = 1.07; 95% CrI: [0.96; 1.18]).
Modifiable factors:
Modifiable barriers were symptom distress (measured using mSAS-SF; MAP value for log OR = −0.46; 95% CrI: [−0.68; −0.24]), and negative attitude (Negative attitude scale; MAP value for log OR = −0.40; 95% CrI: [−0.49; −0.31]), the distribution dispersion of SD = 0.36 and 0.26, respectively, indicating a moderate uncertainty in the evidence.
Modifiable enablers were social support (MAP value for log OR = 0.56; 95% CrI: [0.48; 0.63]), self-efficacy (MAP value for log OR = 0.43; 95% CrI: [0.32; 0.54]), positive physical activity attitude (MAP value for log OR = 0.92; 95% CrI: [0.77; 1.06]), distribution dispersion: SD = 0.26, 0.37, and 0.36, respectively, indicating moderate uncertainty in the evidence.
Sensitivity analysis results
The results of the sensitivity analysis comparing qualitative evidence to quantitative evidence and the results of the analysis combining both are summarised in .
Heterogeneous physical activity outcomes were combined in the main meta-analysis. This included accelerometer units (n = 2), duration, mins/day assessed using an accelerometer (n = 1), energy expenditure estimated from accelerometer data (metabolic equivalents, METs; n = 4), self-reported exercise compliance (n = 6), self-reported general physical activity, measured using International Physical Activity Questionnaire, IPAQ (n = 2), self-reported adherence to prescribed exercise self-care behaviour (n = 1), steps per day (n = 3), and one study included both energy expenditure (METs) and duration (mins/day), supplement 6.
The sensitivity analysis results stratified by physical activity outcome assessed in quantitative evidence (likelihood) are reported separately for each barrier and enabler in supplement 8. Sensitivity analysis highlighted the following changes in the evidence compared to the main results. Studies (n = 2) assessing physical activity using an accelerometer did not support depression as a considerable barrier to physical activity. Studies (n = 4) assessing the relationships between energy expenditure (METs) provided evidence with moderate uncertainty regarding perceived symptoms in comparison to the main results suggesting that perceived symptoms are a barrier with low uncertainty in the evidence. Studies (n = 3) that assessed steps per day using an accelerometer suggest considerably high uncertainty in the evidence regarding the barriers (i.e., Pro-BNP) and enablers (i.e., 6MWT, physical functioning, LVEF, Peak VO2). The findings of the meta-analysis restricted to the studies assessing self-reported physical activity duration per day, self-reported exercise recommendation compliance, self-reported physical activity (IPAQ), and physical activity as self-care behaviour did not differ from the main results (supplement 8).
Applying findings to intervention development
reports barriers and enablers identified in qualitative evidence that need to be further investigated in quantitative studies (high uncertainty) and barriers and enablers supported in quantitative evidence with low or moderate uncertainty and the behaviour change strategies that may be useful in addressing them.
Table 3. Summary of the barriers and enablers suggested by qualitative and quantitative evidence and proposed behaviour change techniques (BCTs).
Discussion
This review aimed to identify, describe, and compare contextual and modifiable barriers and enablers to physical activity in heart failure (HF) using a Bayesian approach. This work extends the limited research on the modifiable barriers and enablers for physical activity participation by individuals living with HF. Both qualitative and quantitative studies were included in this review and meta-analysis. The contextual barriers supported by the evidence with low uncertainty are high pro-BNP and perceived symptoms. Older age, Left Ventricular Ejection Fraction (LVEF, %), depression, HF duration and living with a partner. Evidence concerning the modifiable barriers: negative attitude and symptom distress; and enablers: social support, positive physical activity attitude, and self-efficacy is moderately uncertain.
This review also aimed to demonstrate the applicability of the Bayesian approach in evidence synthesis informing behaviour change interventions. MRC framework for designing complex interventions (Craig et al., Citation2008; Skivington et al., Citation2021) urges researchers to consider evidence from a diverse set of sources when developing interventions. Several methods have been proposed, including the use of mixed-methods studies where one type of evidence (e.g., qualitative) informs the research design of the complimenting study (e.g., quantitative). However, it is less clear how to compare findings from a broad range of studies (qualitative and quantitative) and how to estimate uncertainty in the evidence. The Bayesian approach presents a unique opportunity for research informing complex intervention development by providing a workflow and analysis equipped to combine different evidence and evaluate uncertainty in the evidence. This review synthesised evidence from different sources. The prior elicitation task facilitated this. The results of the expert elicitation task were updated with quantitative evidence. Such Bayesian updating of the probability of physical activity in HF conditioned on each construct summarises both qualitative and quantitative evidence. This approach was first implemented by Dixon-Woods and colleagues (Dixon-Woods et al., Citation2005; Roberts et al., Citation2002). Dixon-Woods et al. (Dixon-Woods et al., Citation2005; Roberts et al., Citation2002) advocated for integrating qualitative research in healthcare decision making because it provides valuable insights and places the patient in the heart of care by bringing their perspective into account. However, Roberts et al. (Citation2002) highlighted the following shortcoming of using Bayesian meta-analysis. Qualitative research should be formally and systematically catalogued before it can be integrated with quantitative findings, which is often not straightforward. Using the TDF (Cane et al., Citation2012) in the present review, we mitigated this limitation.
Contextual barriers and enablers
Older age is a barrier to physical activity in HF, as suggested by both qualitative and quantitative evidence. This result further reiterated the finding of a meta-analysis (Amirova et al., Citation2021) that older adults living with HF need more support to attain higher physical activity levels.
Depression is a considerable barrier, as identified by the quantitative evidence. Depression is a large burden on the HF population. It is associated with poor adherence to pharmaceutical treatment (Goldstein et al., Citation2017) and is an independent predictor of morbidity (Moudgil & Haddad, Citation2013). The physiological determinants perpetuating depression in HF include inflammation, blood cell abnormalities, Central Nervous System (CNS) changes and changes in health-protective behaviours (Huffman et al., Citation2013). The association between depression, HF, and lack of physical activity is complex. Like any cardiovascular disease, HF is a consequence of low physical activity in clinically depressed individuals (Gold et al., Citation2020). More research investigating the mechanism via which depression impacts physical activity in HF is needed.
The review findings concerning employment are in accord with a qualitative semi-structured interview study with a non-clinical sample of adults transitioning to retirement, which found that retirement is perceived as providing opportunities to become physically active (McDonald et al., Citation2015). On the other hand, authors also reported that this was not always the case, and an individualised approach may be required (McDonald et al., Citation2015). Similarly, a national survey of 1550 adults aged 60–69 in England in 2007 reported that work commitments and lack of leisure time were major barriers to physical activity (Chaudhury & Shelton, Citation2010). Context, social norms surrounding physical activity in older age may impact how physical activity is enacted in older adults who transitioned to retirement (Koeneman et al., Citation2017; McPhee et al., Citation2016).
The diagnosis of HFrEF and its duration may engender a higher risk of physical inactivity than the diagnosis of HFpEF. However, the available evidence is uncertain, and more evidence is needed before drawing any definitive conclusions. Non-cardiovascular comorbidities in HF include Diabetes Mellitus (type 2), chronic obstructive pulmonary disease (COPD), and renal dysfunction (Rushton et al., Citation2015). A frequent comorbid cardiovascular condition is atrial fibrillation (Ling et al., Citation2016). These comorbidities increase both morbidity and mortality in HF (Rushton et al., Citation2015). This review identified that a greater number of comorbidities reduce the physical activity engagement in HF. Another clinical barrier identified by the present review is longer HF duration which is likely to result in deterioration of physical functionating. Overall, it is likely that longer HF diagnosis duration, as well as multimorbidity, contribute to limited physical activity levels.
These contextual factors need to be carefully considered in both future cross-sectional studies and randomised-controlled trials evaluating the mechanism of change. Understanding the contextual determinants influencing behaviour is useful in informing the design of quantitative research studies investigating modifiable determinants influencing physical activity (Rothman et al., Citation2008). Contextual differences (i.e., age, LVEF, and depression) indicate that different approaches to behaviour change interventions for these subgroups that take into account their unique clinical characteristics and align with the European Society of Cardiology (Ponikowski et al., Citation2016) and NICE (National Institute for Healthcare and Excellence, Citation2018) guidelines are required. The review encourages the consideration of these patient characteristics in the intervention design and its tailoring. However, contextual understanding does not provide insights into what can and needs to be changed for these demographic and clinical subgroups to engage in physical activity. This urges research on modifiable barriers and enablers to physical activity in HF in these subgroups.
Modifiable barriers and enablers
Both qualitative and quantitative evidence included in this meta-analysis suggests that perceived symptoms and negative attitude (Emotion) are relevant barriers. While social support (Social Influences), positive physical activity attitude (Beliefs about Consequences) and self-efficacy (Beliefs about Capabilities) are suggested as enablers of the behaviour. Another review of qualitative and quantitative studies on barriers and enablers relevant to older adults (65–70 years old) and middle-aged adults (50–64 years old) identified that older adults might rely on social influence, social reinforcement and assistance in managing the change in lifestyle to a greater extent than the middle-aged adults (Spiteri et al., Citation2019). Older adults require social support in managing HF and daily life.
The following domains were identified uniquely in qualitative research: Knowledge, Beliefs about Consequences, Environmental Context and Resources, Behavioural Regulation and Social/Professional Role and Identity. According to qualitative evidence alone, individuals living with HF are driven by the motivation to achieve the desired outcome, such as reduced symptoms and improved health (Beliefs about Consequences). According to qualitative research, the local environment that encouraged physical activity (e.g., parks; Environmental Context and Resources) was fundamental for physical activity enactment. The need to find practical solutions to overcome limitations in physical activity (i.e., problem solving; Behavioural Regulation) played a crucial role in physical activity, according to the qualitative studies included in this review. While the change in perceived social role, described as a loss of social network and position in society brought about as a result of HF, had negative implications for physical activity (Social/Professional Role and Identity). However, these were not followed up with a quantitative study to confirm their relevance in a larger sample. This meta-analysis suggests exploring and confirming the role of these barriers and enablers in quantitative research.
Study-level limitations
Currently, there is no gold standard risk of bias assessment for observational studies (Page et al., Citation2018). Therefore, this review included categories of sources of bias traditionally proposed for assessing study-level bias. These include confounding bias, selection bias, measurement bias, missing data bias, and reporting bias (Page et al., Citation2018). These collectively formed the criteria for evaluating the risk of bias across the included studies. Overall, the majority of the studies (75%) were exposed to a low risk of bias. The major source of bias in the included studies is confounding, as observational studies included in the review (81.25%) did not control for confounding effects when assessing correlates of physical activity.
Strength and limitations of this review
We have adhered to the criteria for conducting Bayesian research , supplement 9 (Depaoli et al., Citation2017). However, there are a few limitations. First, this meta-analysis offered claims about the association, not causality. Second, the prior was elicited using an expert elicitation task with a limited panel of experts. Health psychology researchers appraised qualitative evidence. They then completed a task designed to elicit a prior probability for physical activity conditioned on the constructs identified in the included qualitative studies. While this is an established technique for formalinising an informative prior, it is by definition subjective and thus depends strongly on the members of the expert panel (Albert et al., Citation2012). In this case, the panel was limited to health psychologists. It would have been beneficial to include other stakeholders, such as HF nurses or cardiologists. Third, the search was performed in January 2020 before the COVID-19 pandemic and has not been updated because the studies conducted since investigated the impact of COVID-19 and the lockdown on physical activity in HF. Such a global change posits an incomparable barrier to physical activity and is difficult to assess alongside general barriers and enablers summarised in this review. Heterogeneous physical activity outcomes were combined in the main meta-analysis. The qualitative evidence described physical activity in the general sense, while quantitative evidence included well-defined heterogeneous physical activity outcomes. Due to this limitation inherited from the qualitative data, in the main Bayesian meta-analysis, we combined heterogeneous physical activity outcomes. In a sensitivity analysis, we assessed the impact of this on the findings, where we stratified the analysis of quantitative evidence by physical activity outcome (supplement 8). Physical activity outcomes included accelerometer units (n = 2), duration, mins/day assessed using an accelerometer (n = 1), energy expenditure estimated from accelerometer data (METs; n = 4), self-reported exercise compliance (n = 6), self-reported general physical activity, IPAQ (n = 2), self-reported adherence to prescribed exercise self-care behaviour (n = 1), steps per day (n = 3), and one study included both energy expenditure (METs) and duration (mins/day). The sensitivity analysis results revealed that depression is not a considerable barrier to physical activity assessed using an accelerometer, suggesting that studies assessing physical activity using self-reports may overestimate the impact of depression on actual physical activity levels. The evidence concerning perceived symptoms is moderately uncertain when only energy expenditure (METs) is included in the analysis. While steps per day are not associated with pro-BNP and enablers such as 6MWT, physical functioning, LVEF, and Peak VO2. The latter may have resulted from the smaller number of studies being included in the stratified analysis. The findings concerning other barriers and enablers did not change once the analysis was stratified by physical activity outcome. Physical activity behaviour may be more homogeneous in HF than in the general population due to its low levels and physical limitations.
Recommendations for future research and clinical practice
Older adults (>70 years old) living with HF are at risk of low physical activity levels. It is important to explore beliefs about physical activity that are associated with older age. Research informing the development of interventions for this subgroup of the population is needed. The quantitative evidence suggests that physical activity levels are reduced in the presence of depression (Emotion). A better understanding of the mechanism through which depression impacts physical activity in HF and how it can be mitigated is needed. The quantitative evidence on physical activity conditioned on other clinical, demographic, and psychosocial barriers and enablers is uncertain. Research investigating a broad range of clinical, demographic, and psychosocial barriers and enablers to physical activity in HF is warranted. In addition, identified studies did not explore the mechanism underlying physical activity enactment, including how the barriers and enablers interact, which should be further explored in future research.
Finally, tentative suggestions are made for what a future physical activity intervention needs to include. Overall, the review findings indicate that to reduce the barriers and enhance the enablers, a behaviour change intervention containing the following BCTs are needed: identity associated with target behaviour, prompt/cues and adding objects to the environment, behavioural practice/rehearsal, and graded tasks. A previous meta-analysis of randomised controlled trials also suggested that these strategies are associated with the efficacy of interventions (Amirova et al., Citation2021). In addition, the qualitative evidence included in this review suggests that addressing the change in the social identity as a result of acquired HF diagnosis and the perceived appropriateness of physical activity in this context need to be addressed. Social Influences, Beliefs about Consequences, Behavioural Regulation, and Emotion via BCTs such as social support, information about health and emotional consequences of the behaviour, problem solving, and reducing negative attitude (Emotion), may be effective in increasing physical activity in HF, according to the present review. However, the latter suggestions need to be considered with caution, considering the high uncertainty in the evidence.
Conclusion
The identified contextual barriers and enablers to physical activity in HF need to be carefully considered when designing interventions and randomised controlled trials evaluating interventions. There is moderate evidence in support of the modifiable barriers – symptom distress (Emotion) and negative attitude (Emotion) – and modifiable enablers – social support (Social Influences), self-efficacy (Beliefs about Capabilities), and positive attitude towards physical activity (Beliefs about Consequences). Interventions targeting these barriers and enablers warrant further investigation.
The Bayesian approach in this review enabled comparative predictions about barriers and enablers, helped elicit the extent of uncertainty in the evidence and enabled the combination of qualitative and quantitative evidence in a single synthesis. Thus, the present review supports the usefulness of the Bayesian approach to evidence synthesis concerning barriers and enablers to behaviour and in the development of behaviour change interventions.
Authors contribution
AA and LT searched electronic databases, performed screening of titles and abstracts and full texts, and carried out the risk of bias evaluation. The qualitative TDF-based analysis was carried out by AA, BV, and AC. The expert elicitation task was completed by AA, LT, BV, NA, AC, and TF. The Bayesian meta-analysis was carried out by AA. The manuscript was drafted by AA. All authors contributed to the interoperation of the results and manuscript revisions.
Protocol
The review's protocol was registered on PROSPERO: CRD42021232048
Availability of data, code and other materials
Supplements:
Detailed Inclusion criteria. The scope of the review: pi(e)cos and spider
Search strategy.
Statistical analysis.
Included studies.
Studies that appear to meet the inclusion but were excluded (with the reasons for exclusion).
Physical activity assessment across studies.
Individual studies risk of bias.
The results of the sensitivity analysis stratified by physical activity outcome.
Checklist: criteria for evaluating studies employing Bayesian statistics (Depaoli et al., Citation2017).
PRISMA checklist: https://prisma.shinyapps.io/checklist/.
Supplemental Material 1
Download MS Word (26.8 KB)Supplemental Material 2
Download MS Word (20.3 KB)Supplemental Material 3
Download MS Word (165.5 KB)Supplemental Material 4
Download MS Word (20 KB)Supplemental Material 5
Download MS Word (19 KB)Supplemental Material 6
Download MS Word (102.6 KB)Supplemental Material 7
Download MS Word (300.2 KB)Supplemental Material 8
Download MS Word (992.5 KB)Supplemental Material 9
Download MS Word (24.1 KB)Supplemental Material 10
Download MS Word (30.9 KB)Disclosure statement
No potential conflict of interest was reported by the author(s).
Additional information
Funding
Notes
1 GitHub repository: https://github.com/AliyaAM/bayesian_meta_analysis.
References
- Albert, I., Donnet, S., Guihenneuc-Jouyaux, C., Low-Choy, S., Mengersen, K., & Rousseau, J. (2012). Combining expert opinions in prior elicitation. Bayesian Analysis, 7(3), 503–532. https://doi.org/10.1214/12-BA717
- Alosco, M. L., Spitznagel, M. B., Miller, L., Raz, N., Cohen, R., Sweet, L. H., Colbert, L. H., Josephson, R., Waechter, D., Hughes, J., Rosneck, J., & Gunstad, J. (2012). Depression is associated with reduced physical activity in persons with heart failure. Health Psychology: Official Journal of the Division of Health Psychology, American Psychological Association, 31(6), 754–762. https://doi.org/10.1037/a0028711
- Amirova, A., Fteropoulli, T., Williams, P., & Haddad, M. (2021). Efficacy of interventions to increase physical activity for people with heart failure: A meta-analysis. Open Heart, 8(1), e001687. https://doi.org/10.1136/openhrt-2021-001687
- Araújo-Soares, V., Hankonen, N., Presseau, J., Rodrigues, A., & Sniehotta, F. F. (2019). Developing behavior change interventions for self-management in Chronic Illness: An integrative overview. European Psychologist, 24(1), 7–25. https://doi.org/10.1027/1016-9040/a000330
- Atkins, L., Francis, J., Islam, R., O’Connor, D., Patey, A., Ivers, N., Foy, R., Duncan, E. M., Colquhoun, H., Grimshaw, J. M., Lawton, R., & Michie, S. (2017). A guide to using the Theoretical Domains Framework of behaviour change to investigate implementation problems. Implementation Science, 12(1), 77. https://doi.org/10.1186/s13012-017-0605-9
- Beard, E., & West, R. (2017). Using Bayesian statistics in health psychology: a comment on Depaoli et al. (2017). Health Psychology Review, 11(3), 298–301. https://doi.org/10.1080/17437199.2017.1349544
- Belardinelli, R., Georgiou, D., Cianci, G., & Purcaro, A. (2012). 10-year exercise training in chronic heart failure:randomized controlled trial. Journal of the American College of Cardiology, 60(16), 1521–1528. https://doi.org/10.1016/j.jacc.2012.06.036
- Bragazzi, N. L., Zhong, W., Shu, J., Abu Much, A., Lotan, D., Grupper, A., Younis, A., & Dai, H. (2021). Burden of heart failure and underlying causes in 195 countries and territories from 1990 to 2017. European Journal of Preventive Cardiology, 28(15), 1682–1690. https://doi.org/10.1093/eurjpc/zwaa147
- Cane, J., O’Connor, D., & Michie, S. (2012). Validation of the theoretical domains framework for use in behaviour change and implementation research. Implementation Science, 7(1), 37. https://doi.org/10.1186/1748-5908-7-37
- Chaudhury, M., & Shelton, N. (2010). Physical activity among 60–69-year-olds in England: Knowledge, perception, behaviour and risk factors. Ageing and Society, 30(8), 1343–1355. https://doi.org/10.1017/S0144686X10000486
- Chien, H.-C., Chen, H.-M., Garet, M., & Wang, R.-H. (2014). Predictors of physical activity in patients with heart failure: a questionnaire study: A questionnaire study. The Journal of Cardiovascular Nursing, 29(4), 324–331. https://doi.org/10.1097/JCN.0b013e31828568d6
- Connell, L. E., Carey, R. N., de Bruin, M., Rothman, A. J., Johnston, M., Kelly, M. P., & Michie, S. (2019). Links between behavior change techniques and mechanisms of action: An expert consensus study. Annals of Behavioral Medicine, 53(8), 708–720. https://doi.org/10.1093/abm/kay082
- Conraads, V. M., Deaton, C., Piotrowicz, E., Santaularia, N., Tierney, S., Piepoli, M. F., & Ponikowski, P. P. (2012). Adherence of HF patients to exercise: Barriers and possible solutions. European Journal of HF, 14(5), 451–458. https://doi.org/10.1093/eurjhf/hfs048.
- Corvera-Tindel, T., Doering, L. V., Gomez, T., & Dracup, K. (2004). Predictors of noncompliance to exercise training in heart failure. The Journal of Cardiovascular Nursing, 19(4), 269–277; quiz 278–279. https://doi.org/10.1097/00005082-200407000-00006
- Craig, P., Dieppe, P., Macintyre, S., Michie, S., Nazareth, I., Petticrew, M., & Medical Research Council Guidance. (2008). Developing and evaluating complex interventions: The new medical research council guidance. BMJ, 337, a1655. https://doi.org/10.1136/bmj.a1655
- Davies, E. J., Moxham, T., Rees, K., Singh, S., Coats, A. J. S., Ebrahim, S., Lough, F., & Taylor, R. S. (2010). Exercise training for systolic heart failure: Cochrane systematic review and meta-analysis. European Journal of Heart Failure, 12(7), 706–715. https://doi.org/10.1093/eurjhf/hfq056
- Dekkers, O. M., Vandenbroucke, J. P., Cevallos, M., Renehan, A. G., Altman, D. G., & Egger, M. (2019). COSMOS-E: Guidance on conducting systematic reviews and meta-analyses of observational studies of etiology. PLoS Medicine, 16(2), e1002742. https://doi.org/10.1371/journal.pmed.1002742
- Del Re, A. C. (2020). CRAN - Package compute.es. Retrieved February 5, 2022, from https://cran.r-project.org/web/packages/compute.es/index.html.
- Depaoli, S., Rus, H. M., Clifton, J. P., van de Schoot, R., & Tiemensma, J. (2017). An introduction to Bayesian statistics in health psychology. Health Psychology Review, 11(3), 248–264. https://doi.org/10.1080/17437199.2017.1343676
- Dibben, G. O., Dalal, H. M., Taylor, R. S., Doherty, P., Tang, L. H., & Hillsdon, M. (2018). Cardiac rehabilitation and physical activity: Systematic review and meta-analysis. Heart, 104(17), 1394–1402. https://doi.org/10.1136/heartjnl-2017-312832
- Dixon-Woods, M., Agarwal, S., Jones, D., Young, B., & Sutton, A. (2005). Synthesisinging qualitative and quantitative evidence: a review of possible methods. Journal of Health Services Research & Policy, 10(1), 45–53. https://doi.org/10.1177/135581960501000110
- Doherty, P. J., & Harrison, A. S. (2017). The National Audit of Cardiac Rehabilitation: Annual Statistical Report 2017.
- Dontje, M. L., van der Wal, M. H. L., Stolk, R. P., Brügemann, J., Jaarsma, T., Wijtvliet, P. E. P. J., van der Schans, C. P., & de Greef, M. H. G. (2014). Daily physical activity in stable heart failure patients. The Journal of Cardiovascular Nursing, 29(3), 218–226. https://doi.org/10.1097/JCN.0b013e318283ba14
- Evangelista, L. S., Berg, J., & Dracup, K. (2001). Relationship between psychosocial variables and compliance in patients with heart failure. Heart & Lung: The Journal of Critical Care, 30(4), 294–301. https://doi.org/10.1067/mhl.2001.116011
- Evangelista, L. S., Doering, L. V., Dracup, K., Westlake, C., Hamilton, M., & Fonarow, G. C. (2003). Compliance behaviors of elderly patients with advanced heart failure. The Journal of Cardiovascular Nursing, 18(3), 197–206; quiz 207–208. https://doi.org/10.1097/00005082-200307000-00005
- ExTraMATCH Collaborative. (2004). Exercise training meta-analysis of trials in patients with chronic heart failure (ExTraMATCH). Bmj, 328(7433), 189. https://doi.org/10.1136/bmj.37938.645220.EE
- Gallagher, R., Luttik, M.-L., & Jaarsma, T. (2011). Social support and self-care in heart failure. The Journal of Cardiovascular Nursing, 26(6), 439–445. https://doi.org/10.1097/JCN.0b013e31820984e1
- Gold, S. M., Köhler-Forsberg, O., Moss-Morris, R., Mehnert, A., Miranda, J. J., Bullinger, M., Steptoe, A., Whooley, M. A., & Otte, C. (2020). Comorbid depression in medical diseases. Nature Reviews Disease Primers, 6(1), 69. https://doi.org/10.1038/s41572-020-0200-2
- Goldstein, C. M., Gathright, E. C., & Garcia, S. (2017). Relationship between depression and medication adherence in cardiovascular disease: The perfect challenge for the integrated care team. Patient Preference and Adherence, 11, 547–559. https://doi.org/10.2147/PPA.S127277
- Groenewegen, A., Rutten, F. H., Mosterd, A., & Hoes, A. W. (2020). Epidemiology of heart failure. European Journal of Heart Failure, 22(8), 1342–1356. https://doi.org/10.1002/ejhf.1858
- Hagger, C., Hamilton, H., & Lintunen, T. (2020). The Handbook of Behavior Change (Cambridge Handbooks in Psychology). (M. S. Hagger, L. D. Cameron, K. Hamilton, N. Hankonen, & T. Lintunen, Eds.).
- Hamilton, K., Marques, M. M., & Johnson, B. T. (2017). Advanced analytic and statistical methods in health psychology. Health Psychology Review, 11(3), 217–221. https://doi.org/10.1080/17437199.2017.1348905
- Heino, M. T. J., Vuorre, M., & Hankonen, N. (2018). Bayesian evaluation of behavior change interventions: A brief introduction and a practical example. Health Psychology and Behavioral Medicine, 6(1), 49–78. https://doi.org/10.1080/21642850.2018.1428102
- Huffman, J. C., Celano, C. M., Beach, S. R., Motiwala, S. R., & Januzzi, J. L. (2013). Depression and cardiac disease: Epidemiology, mechanisms, and diagnosis. Cardiovascular Psychiatry and Neurology, 2013, 1–14. https://doi.org/10.1155/2013/695925
- Jaarsma, T., Strömberg, A., Ben Gal, T., Cameron, J., Driscoll, A., Duengen, H.-D., Inkrot, S., Huang, T.-Y., Huyen, N. N., Kato, N., Köberich, S., Lupón, J., Moser, D. K., Pulignano, G., Rabelo, E. R., Suwanno, J., Thompson, D. R., Vellone, E., Alvaro, R., … Riegel, B. (2013). Comparison of self-care behaviors of heart failure patients in 15 countries worldwide. Patient Education and Counseling, 92(1), 114–120. https://doi.org/10.1016/j.pec.2013.02.017
- Klompstra, L., Jaarsma, T., & Strömberg, A. (2018). Self-efficacy mediates the relationship between motivation and physical activity in patients with heart failure. The Journal of Cardiovascular Nursing, 33(3), 211–216. https://doi.org/10.1097/JCN.0000000000000456
- Koeneman, M. A., Chorus, A., Hopman-Rock, M., & Chinapaw, M. J. M. (2017). A novel method to promote physical activity among older adults in residential care: An exploratory field study on implicit social norms. BMC Geriatrics, 17(1), 8. https://doi.org/10.1186/s12877-016-0394-z
- Kok, G., Gottlieb, N. H., Peters, G.-J. Y., Mullen, P. D., Parcel, G. S., Ruiter, R. A. C., Fernández, M. E., Markham, C., & Bartholomew, L. K. (2016). A taxonomy of behaviour change methods: An intervention mapping approach. Health Psychology Review, 10(3), 297–312. https://doi.org/10.1080/17437199.2015.1077155
- Lee, H., Boo, S., Yu, J., Suh, S.-R., Chun, K. J., & Kim, J. H. (2017). Physical functioning, physical activity, exercise self-efficacy, and quality of life among individuals with chronic heart failure in Korea: A cross-sectional descriptive study. The Journal of Nursing Research: JNR, 25(2), 131–139. https://doi.org/10.1097/JNR.0000000000000150
- Lewinter, C., Doherty, P., Gale, C. P., Crouch, S., Stirk, L., Lewin, R. J., LeWinter, M. M., Ades, P. A., Køber, L., & Bland, J. M. (2015). Exercise-based cardiac rehabilitation in patients with heart failure: A meta-analysis of randomised controlled trials between 1999 and 2013. European Journal of Preventive Cardiology, 22(12), 1504–1512. https://doi.org/10.1177/2047487314559853
- Ling, L.-H., Kistler, P. M., Kalman, J. M., Schilling, R. J., & Hunter, R. J. (2016). Comorbidity of atrial fibrillation and heart failure. Nature Reviews Cardiology, 13(3), 131–147. https://doi.org/10.1038/nrcardio.2015.191
- McDonald, S., O’Brien, N., White, M., & Sniehotta, F. F. (2015). Changes in physical activity during the retirement transition: A theory-based, qualitative interview study. International Journal of Behavioral Nutrition and Physical Activity, 12(1), 25. https://doi.org/10.1186/s12966-015-0186-4
- McPhee, J. S., French, D. P., Jackson, D., Nazroo, J., Pendleton, N., & Degens, H. (2016). Physical activity in older age: Perspectives for healthy ageing and frailty. Biogerontology, 17(3), 567–580. https://doi.org/10.1007/s10522-016-9641-0
- Michie, S., Richardson, M., Johnston, M., Abraham, C., Francis, J., Hardeman, W., Eccles, M. P., Cane, J., & Wood, C. E. (2013). The behavior change technique taxonomy (v1) of 93 hierarchically clustered techniques: Building an international consensus for the reporting of behavior change interventions. Annals of Behavioral Medicine, 46(1), 81–95. https://doi.org/10.1007/s12160-013-9486-6
- Michie, S., van Stralen, M. M. & West, R. (2011). The behaviour change wheel: A new method for characterising and designing behaviour change interventions. Implementation Science, 6(1), 42. https://doi.org/10.1186/1748-5908-6-42
- Moreno-Suarez, I., Liew, S., Dembo, L. G., Larbalestier, R., & Maiorana, A. (2019). Physical activity is higher in patients with LVADs compared to chronic heart failure. Medicine and Science in Sports and Exercise. https://doi.org/10.1249/MSS.0000000000002104
- Moudgil, R., & Haddad, H. (2013). Depression in heart failure. Current Opinion in Cardiology, 28(2), 249–258. https://doi.org/10.1097/HCO.0b013e32835ced80
- Mozaffarian, D., Benjamin, E. J., Go, A. S., Arnett, D. K., Blaha, M. J., Cushman, M., de Ferranti, S., Després, J.-P., Fullerton, H. J., Howard, V. J., Huffman, M. D., Judd, S. E., Kissela, B. M., Lackland, D. T., Lichtman, J. H., Lisabeth, L. D., Liu, S., Mackey, R. H., Matchar, D. B., … American Heart Association Statistics Committee and Stroke Statistics Subcommittee, X. X. (2015). Heart disease and stroke statistics—2015 update. Circulation, 131(4), e29–322. https://doi.org/10.1161/CIR.0000000000000152
- National Institute for Healthcare and Excellence. (2018). NICE: Overview. Chronic heart failure in adults: diagnosis and management. Guidance. Retrieved March 23, 2020, from https://www.nice.org.uk/guidance/ng106.
- O’Cathain, A., Croot, L., Duncan, E., Rousseau, N., Sworn, K., Turner, K. M., O'Cathain, A., Yardley, L., & Hoddinott, P. (2019). Guidance on how to develop complex interventions to improve health and healthcare. BMJ Open, 9(8), e029954. https://doi.org/10.1136/bmjopen-2019-029954
- O’Cathain, A., Croot, L., Sworn, K., Duncan, E., Rousseau, N., Turner, K., Yardley, L., & Hoddinott, P. (2019). Taxonomy of approaches to developing interventions to improve health: A systematic methods overview. Pilot and Feasibility Studies, 5(1), 41. https://doi.org/10.1186/s40814-019-0425-6
- O’Donnell, J., Smith-Byrne, K., Velardo, C., Conrad, N., Salimi-Khorshidi, G., Doherty, A., Dwyer, T., Tarassenko, L., & Rahimi, K. (2020). Self-reported and objectively measured physical activity in people with and without chronic heart failure: UK biobank analysis. Open Heart, 7(1), e001099. https://doi.org/10.1136/openhrt-2019-001099
- Oka, R. K., Gortner, S. R., Stotts, N. A., & Haskell, W. L. (1996). Predictors of physical activity in patients with chronic heart failure secondary to either ischemic or idiopathic dilated cardiomyopathy. The American Journal of Cardiology, 77(2), 159–163. https://doi.org/10.1016/s0002-9149(96)90588-3
- Page, M. J., McKenzie, J. E., Bossuyt, P. M., Boutron, I., Hoffmann, T. C., Mulrow, C. D., Shamseer, L., Tetzlaff, J. M., Akl, E. A., Brennan, S. E., Chou, R., Glanville, J., Grimshaw, J. M., Hróbjartsson, A., Lalu, M. M., Li, T., Loder, E. W., Mayo-Wilson, E., McDonald, S., … Moher, D. (2021). The PRISMA 2020 statement: An updated guideline for reporting systematic reviews. BMJ, 372, n71. https://doi.org/10.1136/bmj.n71
- Page, M. J., McKenzie, J. E., & Higgins, J. P. T. (2018). Tools for assessing risk of reporting biases in studies and syntheses of studies: A systematic review. BMJ Open, 8(3), e019703. https://doi.org/10.1136/bmjopen-2017-019703
- Pihl, E., Fridlund, B., & Mårtensson, J. (2011). Patients' experiences of physical limitations in daily life activities when suffering from chronic heart failure; a phenomenographic analysis. Scandinavian Journal of Caring Sciences, 25(1), 3–11.
- Ponikowski, P., Voors, A. A., Anker, S. D., Bueno, H., Cleland, J. G. F., Coats, A. J. S., Falk, V., González-Juanatey, J. R., Harjola, V.-P., Jankowska, E. A., Jessup, M., Linde, C., Nihoyannopoulos, P., Parissis, J. T., Pieske, B., Riley, J. P., Rosano, G. M. C., Ruilope, L. M., Ruschitzka, F., … van der Meer, P. (2016). 2016 ESC guidelines for the diagnosis and treatment of acute and chronic heart failure: The Task Force for the diagnosis and treatment of acute and chronic heart failure of the European Society of Cardiology (ESC)Developed with the special contribution of the Heart Failure Association (HFA) of the ESC. European Heart Journal, 37(27), 2129–2200. https://doi.org/10.1093/eurheartj/ehw128
- Pozehl, B. J., Mcguire, R., Duncan, K., Hertzog, M., Deka, P., Norman, J., Artinian, N. T., Saval, M. A., & Keteyian, S. J. (2018). Accelerometer-measured daily activity levels and related factors in patients with heart failure. The Journal of Cardiovascular Nursing, 33(4), 329–335. https://doi.org/10.1097/JCN.0000000000000464
- Roberts, K. A., Dixon-Woods, M., Fitzpatrick, R., Abrams, K. R., & Jones, D. R. (2002). Factors affecting uptake of childhood immunisation: A Bayesian synthesis of qualitative and quantitative evidence. The Lancet, 360(9345), 1596–1599. https://doi.org/10.1016/S0140-6736(02)11560-1
- Rothman, K. J., Lash, T. L., & Greenland, S. (2008). Modern Epidemiology.
- Rushton, C. A., Satchithananda, D. K., Jones, P. W., & Kadam, U. T. (2015). Non-cardiovascular comorbidity, severity and prognosis in non-selected heart failure populations: A systematic review and meta-analysis. International Journal of Cardiology, 196, 98–106. https://doi.org/10.1016/j.ijcard.2015.05.180
- Sagar, V. A., Davies, E. J., Briscoe, S., Coats, A. J. S., Dalal, H. M., Lough, F., Rees, K., Singh, S., & Taylor, R. S. (2015). Exercise-based rehabilitation for heart failure: Systematic review and meta-analysis. Open Heart, 2(1), e000163. https://doi.org/10.1136/openhrt-2014-000163
- Sanderson, S., Tatt, I. D., & Higgins, J. P. T. (2007). Tools for assessing quality and susceptibility to bias in observational studies in epidemiology: A systematic review and annotated bibliography. International Journal of Epidemiology, 36(3), 666–676. https://doi.org/10.1093/ije/dym018
- Shoemaker, M. J., Curtis, A. B., Vangsnes, E., & Dickinson, M. G. (2013). Clinically meaningful change estimates for the six-minute walk test and daily activity in individuals with chronic heart failure. Cardiopulmonary Physical Therapy Journal, 24(3), 21–29. https://doi.org/10.1097/01823246-201324030-00004
- Skivington, K., Matthews, L., Simpson, S. A., Craig, P., Baird, J., Blazeby, J. M, Boyd K. A., Craig N., French D. P., McIntosh E., Petticrew M., Rycroft-Malone J., White M., & Moore, L. (2021). A new framework for developing and evaluating complex interventions: Update of Medical Research Council guidance. BMJ, 374, n2061. https://doi.org/10.1136/bmj.n2061
- Snipelisky, D., Kelly, J., Levine, J. A., Koepp, G. A., Anstrom, K. J., McNulty, S. E., Zakeri, R., Felker, G. M., Hernandez, A. F., Braunwald, E., & Redfield, M. M. (2017). Accelerometer-measured daily activity in heart failure With Preserved Ejection Fraction: Clinical correlates and association with standard heart failure severity indices: Clinical correlates and association with standard heart failure severity indices. Circulation. Heart Failure, 10(6), e003878. https://doi.org/10.1161/CIRCHEARTFAILURE.117.003878
- Spiegelhalter, D. J., Abrams, K. R., & Myles, J. P. (2003). Bayesian approaches to clinical trials and health-care evaluation. John Wiley & Sons, Ltd. https://doi.org/10.1002/0470092602.
- Spiteri, K., Broom, D., Bekhet, A. H., de Caro, J. X., Laventure, B., & Grafton, K. (2019). Barriers and motivators of physical activity participation in middle-aged and older adults—A systematic review. Journal of Aging and Physical Activity, 27(4), 929–944. https://doi.org/10.1123/japa.2018-0343
- Taylor, R. S., Long, L., Mordi, I. R., Madsen, M. T., Davies, E. J., Dalal, H., Rees, K., Singh, S. J., Gluud, C., & Zwisler, A.-D. (2019). Exercise-Based Rehabilitation for Heart Failure: Cochrane Systematic Review, Meta-Analysis, and Trial Sequential Analysis. JACC: Heart Failure, 7(8), 691–705. https://doi.org/10.1016/j.jchf.2019.04.023
- Tierney, S., Elwers, H., Sange, C., Mamas, M., Rutter, M. K., Gibson, M., Neyses, L., & Deaton, C. (2011a). What influences physical activity in people with heart failure? A qualitative study. International Journal of Nursing Studies, 48(10), 1234–1243. http://doi.org/10.1016/j.ijnurstu.2011.03.003
- Tierney, S., Mamas, M., Skelton, D., Woods, S., Rutter, M. K., Gibson, M., Neyses, L., & Deaton, C. (2011b). What can we learn from patients with heart failure about exercise adherence? A systematic review of qualitative papers. Health Psychology, 30(4), 401–410. https://doi.org/10.1037/a0022848
- van Aert, R. C. M., & Jackson, D. (2019). A new justification of the hartung-knapp method for random-effects meta-analysis based on weighted least squares regression. Research Synthesis Methods, 10(4), 515–527. https://doi.org/10.1002/jrsm.1356
- van der Wal, M. H. L., Jaarsma, T., Moser, D. K., Veeger, N. J. G. M., van Gilst, W. H., & van Veldhuisen, D. J. (2006). Compliance in heart failure patients: the importance of knowledge and beliefs. European Heart Journal, 27(4), 434–440. https://doi.org/10.1093/eurheartj/ehi603
- van der Wal, M. H. L., van Veldhuisen, D. J., Veeger, N. J. G. M., Rutten, F. H., & Jaarsma, T. (2010). Compliance with non-pharmacological recommendations and outcome in heart failure patients. European Heart Journal, 31(12), 1486–1493. https://doi.org/10.1093/eurheartj/ehq091
- Viechtbauer, W. (2010). Conducting meta-analyses in R with the metafor package. Journal of Statistical Software, 36(3), 1–48. https://doi.org/10.18637/jss.v036.i03.
- Viswanathan, M., Berkman, N. D., Dryden, D. M., & Hartling, L. (2013). Assessing risk of bias and confounding in observational studies of interventions or exposures: Further development of the RTI item bank. Agency for Healthcare Research and Quality (US).
- von Elm, E., Altman, D. G., Egger, M., Pocock, S. J., Gøtzsche, P. C., Vandenbroucke, J. P., & STROBE Initiative. (2007). Strengthening the reporting of observational studies in epidemiology (STROBE) statement: Guidelines for reporting observational studies. BMJ, 335(7624), 806–808. https://doi.org/10.1136/bmj.39335.541782.AD
- Werhahn, S. M., Dathe, H., Rottmann, T., Franke, T., Vahdat, D., Hasenfuß, G., & Seidler, T. (2019). Designing meaningful outcome parameters using mobile technology: a new mobile application for telemonitoring of patients with heart failure: Designing meaningful outcome parameters using mobile technology. ESC Heart Failure, 6(3), 516–525. https://doi.org/10.1002/ehf2.12425
- Witham, M. D., Argo, I. S., Johnston, D. W., Struthers, A. D., & McMurdo, M. E. T. (2006). Predictors of exercise capacity and everyday activity in older heart failure patients. European Journal of Heart Failure, 8(2), 203–207. https://doi.org/10.1016/j.ejheart.2005.03.008
- WHO. (2020). Physical activity. Retrieved January 5, 2022, from https://www.who.int/news-room/fact-sheets/detail/physical-activity.