1. Introduction
Peptide research continues to be an active scientific field because of the encouraging biomedical applications of peptides as drugs, biomarkers, and/or diagnostic tools. Nevertheless, the seemingly intrinsic tendency of peptides to cause hemotoxicity is one of the factors that has halted therapeutic peptides from reaching the market [Citation1]. In this context, computational methods that include (but are not limited to) molecular dynamics simulation and molecular docking, as well as machine learning approaches mainly focused on random forest (RF), support vector machines (SVM), and artificial neural networks (ANN) have played an essential role in the process of accelerating the discovery/design of peptides with desired biological properties [Citation1–3].
In any case, despite the importance and usefulness of the aforementioned in silico methods in assisting peptide design, at least one of the following limitations has been found. First, current methods predict unspecific biological effects, i.e. without considering the biological targets (proteins, cell lines, microorganisms, etc.) or the real values of activity or hemotoxicity. For instance, in antimicrobial research, it is a common practice to classify peptides as antimicrobial or non-antimicrobial without information about the measure of the inhibitory activity (e.g. MIC – minimum inhibitory concentration or IC50 – inhibitory concentration at 50%) and/or the different microbial species and strains. The same problem arises when predicting hemotoxicity; the methods reported above lack information regarding the type of erythrocytes (which may come from sheep, rodents, or humans) used to test the toxicity of the peptides. Consequently, if a peptide is computationally designed, such methods will not offer sufficiently accurate guidance on whether a peptide is highly active or presents considerably low hemolytic toxicity. Second, most of these popular in silico methods can predict activity or hemotoxicity, never both. This negatively impacts the rational design of peptides because the structural and physicochemical aspects required for a peptide to be active are usually different from (or opposed to) those required for that peptide to be non-hemotoxic. Third, most of the computational approaches reported to date do not provide sufficiently detailed physicochemical and/or structural interpretations, which limit the possibility of rationally designing new peptides.
In the last 10 years, several research groups have emphasized the development of in silico models based on the methodology known as Perturbation Theory & Machine Learning (PTML) [Citation4,Citation5], which have been successfully applied to diverse drug discovery areas such as infectious agents [Citation6], neurological disorders [Citation7], and oncology [Citation8]. We would like to highlight that the PTML models can overcome all the disadvantages of the computational methods mentioned. By integrating chemical and biological data coming from different sources, PTML models can simultaneously predict multiple biological endpoints [Citation6–8] against dissimilar biological targets by considering diverse assay protocols while also providing a rigorous interpretation of the physicochemical and structural aspects that can lead to the design of chemicals with the expected profile [Citation8]. Bearing in mind all these ideas, we briefly analyze the limitations mentioned above while discussing a series of guidelines for the use of the PTML methodology in peptide discovery. We also provide examples of successful applications of PTML models in the design of virtually active and non-hemolytic peptides and suggest some ideas to improve computational peptide design.
2. PTML modeling and peptide design: latest guidelines
When designing potentially active and non-hemolytic peptides through the PTML methodology, a series of steps can be followed (). First, the databases with chemical and biological data of peptides should contain the real values of the measured endpoints. Thus, it will be possible to either directly predict the activity and hemotoxicity values or choose rigorous cutoffs that will allow the future PTML model to predict peptides with high inhibitory activity and low hemotoxicity.
Figure 1. Development and application of a PTML model for peptide design. The peptide sequence and the amino acids described as building blocks are all represented for illustrative purpose only. The terms IC50, CC50 and HC50 mean the inhibitory concentration at 50%, the cytotoxic concentration at 50%, and the hemolytic concentration at 50%, respectively. The mathematical expression derived from the Box-Jenkins approach are calculated by employing Microsoft Excel. The training set usually contains the 75% of the data while the remaining 25% belongs to the test set; such a proportion can vary depending on factors that include (but are not limited to) the complexity and amount of data, as well as the type of the machine learning algorithm. The abbreviation ‘INTP’ refers to the physicochemical and structural interpretation of the peptide (sequence-based) descriptors in the PTML model.
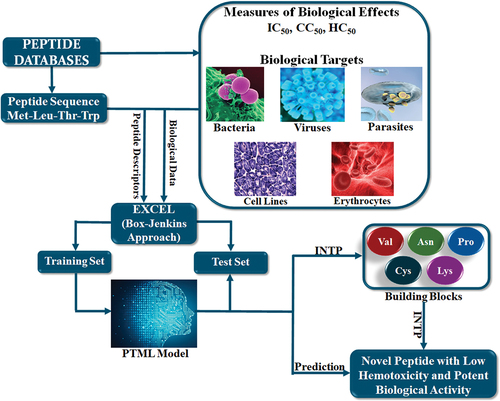
Second, although there may be many descriptors that characterize the structures and/or the amino acid sequences of the peptides, we recommend the use of graph-based topological indices. These peptide descriptors offer several advantages such as easiness and speed of calculation, and, despite having a 2D nature, they can also give 3D information [Citation9,Citation10] such as volume, conformational changes, and molecular accessibility. Also, graph-based topological indices can characterize the chemical structure of any molecule by considering different types fragments (functional groups, rings, aliphatic chains, etc.) [Citation8,Citation11]. Third, the Box-Jenkins approach focuses on the integration of different chemical and biological data [Citation6–8,Citation11], thus enabling the simultaneous prediction of multiple biological endpoints (in the case of the peptides, activity, and hemotoxicity) against diverse biological targets. This is one of the two key steps of the PTML methodology.
Fourth, after finding the best PTML model through the use of RF, SVM, ANN, or any other machine learning technique, the peptide descriptors (topological indices) must be interpreted from a physicochemical and structural point of view. When applied to a peptide, this step permits the calculation of the quantitative contribution of an amino acid to the activity and the hemotoxicity while serving as a guideline for the design of virtually active peptides with low hemolytic toxicity. The physicochemical and structural interpretation of the peptide descriptors is the second key step when performing peptide design via PTML modeling. In the final step, the designed peptides are predicted by the PTML model to confirm that the design was correctly performed.
By following these five steps, PTML models have been successfully developed to design virtual non-hemolytic peptides with antibacterial activity against different bacterial strains [Citation11], anticancer potency against multiple cancer cell lines [Citation12], and antihypertensive activity [Citation13]. Particularly, the PTML model used to design non-hemolytic peptides with antihypertensive activity was tested against two different web servers, one predicting only antihypertensive activity and the other predicting hemotoxicity. The results of the two web servers converged with that of the PTML model, confirming that the designed peptides virtually possessed antihypertensive activity and low hemotoxicity [Citation13].
3. Conclusions
Although experimental validation will always be required, computational methods are, without doubt, of great usefulness for peptide design because they establish the theoretical foundation to rationalize the discovery of bioactive peptides. Yet, they must go beyond tasks of predicting only the activity (or hemotoxicity) of peptides. The PTML methodology described here is a demonstration that the computational methods can focus on the simultaneous prediction of multiple biological endpoints while also focusing on gathering physicochemical and structural information that can be essential for peptide design and optimization. Therefore, the PTML methodology can be applied to any subfield of peptide discovery and opens encouraging horizons for the design of versatile therapeutic peptides.
4. Expert opinion
Although the PTML methodology have many advantages (always limited by the availability and quality of the data) over the other computational methods mentioned in this work, there is room for improvement. In the future, the PTML methodology can benefit from the integration of activity and hemotoxicity endpoints of peptides with their pharmacokinetic data, although the latter seem to be quite dispersed in the scientific literature.
On the other hand, we are aware that most of the computational methods for peptide design use different variants of the sequence-based or 3D descriptors. However, in addition to the development of such models, we strongly recommend the creation of a second class of PTML models based on atom- and bond-based topological indices (TIs). In this sense, when studying the interaction of peptides with non-molecular targets (cells, microorganisms, rodents, etc.), TIs can describe the structure of the peptides in more detail, where each amino acid and its different substructural moieties (functional groups, rings, aliphatic portions) are all characterized as molecular fragments. In doing so, it is possible to extract more information regarding the intrinsic influence (presence) and position of an amino acid in that peptide. In contrast, for the case of models relying on sequence-based or 3D approaches, such structural information may remain hidden or diluted within the structure of a peptide. In our opinion, the use of TIs will increase the predictive power of the models (including those derived from the PTML methodology), providing deeper insights regarding the physicochemical properties and structural aspects that a peptide needs to exhibit both potent bioactivity and low hemotoxicity. Nowadays, with the accelerated advancements of the programming algorithms, it is computationally inexpensive (e.g. any laptop with 8–12 G RAM can do it) and easy to transform many peptide sequences into SMILES strings or a 2D sdf file [Citation14], and subsequently calculate TIs by using software such as AlvaDesc [Citation15]. Models built from TIs can be used alone or in combination with those models focusing on sequence-based or 3D approaches to increase the accuracy when designing, predicting, and optimizing peptides.
Declaration of interest
The authors have no relevant affiliations or financial involvement with any organization or entity with a financial interest in or financial conflict with the subject matter or materials discussed in the manuscript. This includes employment, consultancies, honoraria, stock ownership or options, expert testimony, grants or patents received or pending, or royalties.
Reviewer disclosures
Peer reviewers on this manuscript have no relevant financial or other relationships to disclose.
Additional information
Funding
References
- Robles-Loaiza AA, Pinos-Tamayo EA, Mendes B, et al. Traditional and computational screening of non-toxic peptides and approaches to improving selectivity. Pharmaceuticals. 2022;15:323.
- Palmer N, Maasch Jacqueline RMA, Torres Marcelo DT, et al. Molecular dynamics for antimicrobial peptide discovery. Infect Immun. 2021;89(4):e00703–20.
- Torres MDT, Cao J, Franco OL, et al. Synthetic biology and computer-based frameworks for antimicrobial peptide discovery. ACS Nano. 2021;15(2):2143–2164.
- Gonzalez-Diaz H, Arrasate S, Gomez-SanJuan A, et al. General theory for multiple input-output perturbations in complex molecular systems. 1. Linear QSPR electronegativity models in physical, organic, and medicinal chemistry. Curr Top Med Chem. 2013;13(14):1713–1741.
- Halder AK, Moura AS, Cordeiro MNDS. Moving average-based multitasking in silico classification modeling: where do we stand and what is next? Int J Mol Sci. 2022;23(9):4937.
- Vasquez-Dominguez E, Armijos-Jaramillo VD, Tejera E, et al. Multioutput perturbation-theory machine learning (PTML) model of ChEMBL data for antiretroviral compounds. Mol Pharm. 2019;16(10):4200–4212.
- Diez-Alarcia R, Yanez-Perez V, Muneta-Arrate I, et al. Big data challenges targeting proteins in GPCR signaling pathways; Combining PTML-ChEMBL models and [35 S]GTPγS binding assays. ACS Chem Neurosci. 2019;10(11):4476–4491.
- Kleandrova VV, Scotti MT, Scotti L, et al. Multi-Target drug discovery via PTML modeling: applications to the design of virtual dual inhibitors of CDK4 and HER2. Curr Top Med Chem. 2021;21(7):661–675.
- Estrada E. Physicochemical interpretation of molecular connectivity indices. J Phys Chem A. 2002;106(39):9085–9091.
- Estrada E, Molina E, Perdomo-Lopez IC. 3D structural parameters be predicted from 2D (topological) molecular descriptors? J Chem Inf Comput Sci. 2001;41(4):1015–1021.
- Kleandrova VV, Ruso JM, Speck-Planche A, et al., Enabling the discovery and virtual screening of potent and safe antimicrobial peptides. Simultaneous prediction of antibacterial activity and cytotoxicity. ACS Comb Sci. 2016;18(8): 490–498.
- Speck-Planche A, Cordeiro MNDS. Speeding up the virtual design and screening of therapeutic peptides: simultaneous prediction of anticancer activity and cytotoxicity. In: Speck-Planche A , editor. Multi-scale approaches in drug discovery. 1st ed. Amsterdam: Elsevier; 2017. p. 127–147.
- Kleandrova VV, Rojas-Vargas JA, Scotti MT , et al. PTML modeling for peptide discovery: in silico design of non-hemolytic peptides with antihypertensive activity. Mol Divers. 2021 DOI:10.1007/s11030-021-0350-z
- O’Boyle NM, Banck M, James CA, et al. Open babel: an open chemical toolbox. J Cheminformatics. 2011;3(1):33.
- Mauri A, alvaDesc: A Tool to Calculate and Analyze Molecular Descriptors and Fingerprints. In: Roy K, editor. Ecotoxicological QSARs. New York, NY: Springer US; 2020. p. 801–820.