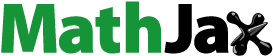
ABSTRACT
Digitisation has become an essential part of archival and library strategies to enhance access to collections. As the digital content is increasing due to large-scale digitisation projects, it is expected that providing digital access to the analogue collections will eventually reduce the number of archival records accessed in the reading room. In this paper, we investigate this issue using two approaches: system dynamics and agent-based modelling. We first analyse real data in order to identify the dynamic hypothesis of the model. Then, a sensitivity analysis is conducted on two baseline models to identify scenarios that match the real dataset. Although the two approaches suceed to simulate the number of requests in the reading room, the experimental results show that a better fit is obtained in the agent-based model when not only the number of records that have been accessed and digitised is taken into account, but also the number of times that such records have been accessed before digitisation. The proposed model can be used to explore the impact of different digitisation strategies on the decrease in access requests in the archival and library reading rooms.
1. Introduction
Archives and libraries have widely embraced digitisation as a way to provide access to physical collections (Campagnolo, Citation2020). After almost four decades of digitisation projects, digital and traditional physical access of the collections in reading rooms coexist in memory institutions. However, user expectations have changed over the years, and archives and libraries are experiencing an increasing pressure to create digital content (Bantin & Agne, Citation2010; Oliver, Citation2011), being digitisation and digital dissemination a more present topic in cultural policies (European Commission, Citation2021; Valtysson, Citation2017).
The digitisation of large collections is time-consuming and comes at a cost. According to the Collections Trust report (Poole, Citation2010, p. 3), in 2010 national archives in Europe accounted for 26.98 billion pages of archival records, of which approximately 17.27 billion are eligible/appropriate for digitisation (Poole, Citation2010). In 2017, the Europeana report (Nauta et al., Citation2017, p. 28) calculated that approximately just 10% of the collections in archives and 17% in libraries was digitally available. In order to ensure the long-term sustainability of the digitisation programmes in relation to other services within the institutions, it is important to gain an understanding of whether digitisation leads to obsolescence of the traditional access to documents in reading rooms.
According to the IFLA Guidelines (IFLA, Citation2002, pp. 13–14), digitisation projects should be set according to a selection policy that clearly specifies what material will be included and for what purpose. Criteria for selection should take into account: (1) the intellectual value of the items based on content, (2) the level of demand, and (3) physical condition of material for digitisation, as well as whether detailed cataloguing and descriptive data are available. Some institutions have chosen the strategy to digitise whole collections instead of targeting solely records that are known to be in the interest of users. The digitisation of whole collections together with new technologies facilitates new forms of access and use (IFLA, Citation2002). For example, traditional cataloguing in archives can be done with the collaboration of volunteers in crowd-sourcing projects (Andro, Citation2018), and artificial intelligence is now used for text recognition.
1.1. Modelling collections as populations
This research inserts itself in a body of work where heritage collections are viewed as populations of objects, what Strlic et al. named ”Collections Demography” (Strlič, Citation2013). This approach has many parallels with healthcare systems. Namely, a lifetime can be defined for historic collections (Strlič et al., Citation2013), and the interest is in analysing how different preventive measures and pre-existing conditions can affect the lifetime of different groups (Duran-Casablancas et al., Citation2021). As opposed to healthcare systems, however, heritage collections have so far not been studied with simulation modelling methods.
It is widely recognised that it is beneficial to model healthcare problems as complex system (Homer & Hirsch, Citation2006; Lipsitz, Citation2012; Plsek & Greenhalgh, Citation2001), evidenced by the increasing number of studies using approaches such as system dynamics (SD) and agent-based modelling (ABM) in the last two decades (Liu et al., Citation2018). The systematic literature review by Salleh et al. (Salleh et al., Citation2017, p. 940) distinguishes three main applications of system dynamics in healthcare: (1) Resource management to optimise health service flow, (2) modelling the effect of policy interventions for effective decision-making and (3) modelling of infectious disease. Point (2) is precisely aligned with the aim of this paper, which can also be expressed in the words of Cassidy et al. in another review of simulation models of healthcare systems: ”to reduce undesirable patient outcomes” (mortality and hospitalisation) and ”to reduce the total cost of care” (Cassidy et al., Citation2019). In other words, the common features between healthcare systems and heritage systems indicate that there is scope to use simulation modelling to improve decision-making in collection management.
1.2. Informing digitisation strategies
In this paper, the decision-making process of interest is the digitisation of collections. There are several strategies commonly followed by libraries and archives to prioritise digitisation. One strategy is to give priority to the most heavily demanded records. A high frequency of access indicates that the records are of interest to readers. Another strategy is Scan on Demand (SoD), which can be seen as an efficient way of selection as the records to be digitised are directly chosen by the readers (Erway & Schaffner, Citation2017; Kemp, Citation2016; Ling & McLean, Citation2004; Schaffner et al., Citation2011). In terms of sustainability, it is convenient that only those records that are of interest to the readers are digitised, since it is well known that collection items are rarely used with uniform frequency. For example, usage statistics have shown that very limited numbers of e-journals are intensively used, whereas the rest of the journals are occasionally used (Brown, Citation2003, p. 146).
Once records are digitally available, the option to order the original is usually removed (VanSnick & Ntanos, Citation2018). But, even if available, less readers may choose this option. Consequently, it can be expected that digitisation will eventually result in a decrease in the number of access requests in the reading room. For example, referring to academic library collections, Martell noted back in 2008 that ”[t]here is no end in sight to the declines in circulation and reference that many libraries are experiencing” (Martell, Citation2008, p. 406). Another example of the impact of providing digital access is shown by UK surveys that have identified a downward trend of family history research in the reading rooms, from 49% in 2014 to 41% in 2018, probably as more content is becoming available online (ARA National Surveys Group, Citation2019).
Traditionally, statistical summaries have been used to understand how the collection is used, by collecting data on users (number of readers, age, gender, ethnicity and education level) and usage (number of requests in the reading room and requests of reproduction; Pickford, Citation2002). Basic statistical analysis is now shifting to web metrics (Kelly, Citation2014) to count the number of readers, the pages readers enter and the time spent on pages or elements of the page. This information is mostly used as a monitoring tool, and it is rarely exploited to its full potential. A more in-depth analysis of these data is needed not only to identify trends but also to identify the causes of these trends, for example, how digitisation is changing the use of the collections in the reading room and whether management strategies need to be adapted to these new developments. Determining the rate of change of reading room use and its relationship with management strategies is the main concern of this paper.
From the perspective of practitioners, it might seem self-evident that digitisation will eventually change the way we access collections as the share of the collection becoming digitally available increases. However, anecdotal evidence is not sufficient to understand how this change depends on management strategies. As Chapman et al. have pointed out, “while special collections and archives managers have at times recognised the importance of using data to drive decision making, translating this objective into reality and integrating data analysis into day-to-day operations has proven to be a significant challenge” (Chapman & Yakel, Citation2012, p. 129). As seen in other fields, the use of simulation modelling could support on this matter of integrating data analysis into decision-making.
1.3. Towards a simulation model
This paper proposes a simulation model to explore this main hypothesis: the availability of digital collections will eventually reduce the requests in the reading room, at a rate that depends on how often records have been requested in the reading room before being digitised.
The dynamics of archival collections can be reproduced with different modelling approaches, either with agent-based modelling or system dynamics. One important consideration is the level of detail needed in the model in order to reflect well the system and its stochastic elements. This is also the case of healthcare systems. Disease-related models based on system dynamics are referred as ”compartmental models”, and use an ”ageing chain” structure (Darabi & Hosseinichimeh, Citation2020). Reinforcing/balancing feedback loops are important to capture the dynamics of infectious diseases in SIR models (Ahmed et al., Citation2012). Stocks represent population with average properties that undergo different stages of the illness, and the flow between groups can be probabilistic (e.g., rate of infection and recovery; Davahli et al., Citation2020). ABM, in contrast, allows a description of each individual and to explore the dynamic interactions among agents and environment, reflecting emergent behaviours (Nianogo & Arah, Citation2015). Furthermore, the literature abounds with examples of systems that can be modelled with either SD or ABM, taking advantage of the capabilities of each approach (Ahmed et al., Citation2012; Cimler et al., Citation2018; Macal, Citation2010).
In the case of archival collections, individuals (archival records) undergo different stages (digitised and accessed in the reading room), which can be modelled with both SD and ABM. At the same time, archival collections are not uniformly requested, and the popularity of items can be difficult to represent in finite categories. This heterogeneity may be easier to model at individual level, lending itself to ABM. Other capabilities of ABM, however, are not needed in order to model this system. Namely, there is no interaction among agents, which only change states due to exogenous factors.
Therefore, this paper tests two different modelling approaches. The first and simplest model is an SD model that does not include the frequency of requests, and instead considers two categories: records can be requested and/or digitised. The second, more detailed model, is an ABM model where each item is characterised by a past frequency of requests, which changes during the simulation. The comparison between the two approaches allows us to test the main hypothesis and determines whether the accumulated number of requests is necessary in order to obtain a good fit to the experimental data. In the future, it may be possible to extend this model to include other factors that can theoretically influence the number of requests, such as the level of cataloguing or social-economic factors, or how readers have preference for accessing the records digitally or physically. In the first section of this paper, these factors will be further discussed accompanied by a causal loop diagram.
Since this is the first time that a simulation model is built in this research field, we first analyse the data from actual collections to find evidence of the impact of digitisation on the number of access requests in the reading room. The collections of the Amsterdam City Archives (SAA) are taken as a case study, as this archive has been collecting usage data in the reading room for almost 20 years and has conducted numerous digitisation projects in the last 15 years. Finally, the model is used to explore some collection-management scenarios. How digitisation of collections is affecting other services, such as the access of physical records in the reading room to anticipate whether they should adapt their services, due to the decrease in visitors in the reading room, but also at what cost.
2. The complexity of providing access to the collections
In this section, we present the causal loop diagram (CLD) that was drawn during three participatory sessions with a total of 25 participants from 14 Dutch archives and libraries. The participants were conservators, collection managers, archivists and digitisation experts. The participatory sessions were organised as part of a larger project that explores the impact of measures and activities within archival institutions on the preservation of paper collections. The CLD was arranged as two parts directly linked to each other: one side of the diagram included variables related to the preservation aspects (e.g., chemical characteristics of the collections and preservation measures), and the other side captured the dynamics of collections use. In this section, we present the part of the CLD involving how the requests to access records that are not digitally available can result in a request to access the record in the reading room or a request for digitisation (). As the focus of this paper is on modelling the access of collections, the preservation part is simply represented as the risk of mechanical degradation during handling (left part of the CLD, ).
Figure 1. Causal loop diagram on the mechanisms leading to requests for digitisation or requests to access the records in the reading room.

According to the participants, digitisation has dramatically changed how we use collections. Institutions feel an enormous pressure to make the collections digitally available. Besides the pressure from visitors and government to provide digital collection access, there are several reinforcing loops that increase this pressure even more. One is caused by the awareness of the information available to users: the more information available, the greater the awareness of it, resulting in more requests which generate more information as well. In addition, there is a reinforcing loop related to the quality of information available: the pressure to digitise results in also pressure to describe collection content, resulting again in more requests. In addition, in the long term, there is a third reinforcing loop showing that collections may be digitised again in the (near) future because the quality of current scans will not meet the quality expected by the users.
These are general trends, but on the daily basis the number of requests for digitisation depends on the willingness of the readers to access the collections digitally. During the workshop, participants identified the following factors to explain visitors’ preferences for accessing the records in the reading room or for requesting digitisation: the lower the price of scan (if not free) and the shorter the delivery time of the scan, the more willing the readers are to use the service Scan on Demand, but the more complex the research or the more the authenticity of the originals is valued, then the more the visitors are inclined to access the objects in the reading room.
In the CLD, costs of digitisation and digital storage of the scans are also included. As we will discuss in the last section of this paper, due to increasing costs and limited budgets, institutions will eventually need to review their preservation and digitisation strategies.
The participatory sessions also led to the identification of an interesting balancing loop. This loop emerges due to the fact that, after digitisation, collections are by default only available digitally. As a result, a reduction of readers in the reading room can be expected, because a growing part of the collections is becoming digitally available. Finding quantitative evidence and then modelling the dynamics that explain this balancing feedback loop is the focus of this paper.
3. Analysis of actual collection data
Information on the usage of the original archival documents (access requests) is automatically generated and stored by collection management systems. The usage data of the Amsterdam City Archives (SAA) show that since 2006 there has been a steady downward trend in the number of access requests in the reading room: a decrease of 36%, from 24.782 requests in 2006 to 15.769 requests in 2018 (). In the reported period, there are three outliers: 2007, when the archive moved to a new location and remained closed for several months, and 2011 and 2012 when there were several collaboration projects with educational programmes in archival sciences, resulting in an increased use of the collections by students.
Figure 2. Number of access requests per year in the reading room of the Amsterdam city archives between 1999 and 2018 and number of archival records digitised per year since 2004.

In 2006, after several pilot projects, the SAA started digitisation of the collections within two projects: Scan on Demand (SoD) and the digitisation of blocks of (popular) archives. The total number of archival documents digitised per year is reported in . The peaks in 2010 and 2011 are due to large digitisation projects when two archives were fully digitised. The sharp increase since 2017 is also due to the no increase in digitisation projects of archival blocks. Regarding the SoD production, ca. 4,000 records per year were digitised from 2008 to 2015, and steadily grew to 6,000 records in 2018. Until 2018, 104,169 archival records had been digitised, 42% of them (44,045 records) within the SoD programme.
From 1999 to 2018, 167,690 archival records were requested 429,108 times. The accumulation of access requests of the archival documents follows a Pareto distribution, which means that more than a half of the archival records have been accessed once, 99% have been accessed less than 15 times in 20 years (), and that the distribution has a long tail (records accessed between 15 and 113 times in 20 years). A Pareto distribution is also obtained when the frequency of requests of archival records is plotted per year.
Figure 3. Frequency distribution of records that have been requested more than 1 time in 1999, 2013 and 2018, and cumulative distribution (percentage) of the number of records that have been requested in the reading room between 1 and 113 times during a period of 20 years at the Amsterdam city archives.

However, also shows that, although the frequency distribution of access requests remained moderately stable throughout the years, two changes in the pattern of the distribution can be observed: the number of archival records that have only been accessed once increased over the years (i), whereas the access to heavily requested documents decreased since 2013 (ii). This change in pattern may be explained by the facts that every year new archival documents are requested for the first time, but the earlier it occurs the higher the chance that they are requested once more (i), and, at the same time, popular records are being digitised and, therefore, their use in the reading room decreases (ii).
The importance of taking the frequency of access requests of archival documents is further stressed in where the variable ”digitisation match” is introduced. When analysing usage data in archival collections, we distinguish between archival records and access requests of the archival records. In order to analyse the digitisation efficiency, we can then calculate not only the percentage of records digitised and accessed but also the percentage of accumulated access requests that accounsts for records that have been digitised (”digitisation match”). shows that the slopes of the decrease in requests since 2006 and of the ”digitisation match” are rather similar (,
), supporting the hypothesis that the observed decrease in access requests since 2006 is correlated to the digitisation of the collections. This dynamic hypothesis will be further tested in the simulation models.
Figure 4. Cumulative percentage of the decrease in requests at the Amsterdam city archives (2007, 2011 and 2012 not included) and “ digitisation match” since 2006. The dashed lines show the number of access requests digitised within the two digitisation programmes, SoD and the digitisation of block of archives.

Further analysis shows that, compared to the digitisation of blocks of (popular) archives, SoD is targeting more effectively the archival records that have ever been accessed in the reading room (42% of the SoD records versus 33.5% for block digitisation). In addition, if popularity of the records, understood as the number of times requested in the reading room, is taken into account in each of the two digitisation programmes (”SoD match” and ”block match”), then SoD also seems to be more effectively targeting the most heavily requested records compared to the digitisation of archival blocks, keeping in mind that the SoD production is smaller than the number of records digitised in archival block projects ().
4. Simulation model
Based on the previous findings, the hypothesis of the model is that the main driver behind the observed decrease in requests in the reading room is the digitisation of records once requested in the reading room. We start building the simplest model in SD where records are modelled as aggregates, according to whether they have been requested in the reading room and digitised. Then, a second model in ABM is developed where the archival records are modelled individually and, therefore, the popularity, understood as the instances of access in the reading room, is also modelled.
4.1. System dynamics model
The SD model includes two interconnected parts: one part represents the collection itself, whereas the second part models the use of the collections (access requests in the reading room and requests of digitisation; ).
Figure 5. Proposed system dynamics model to simulate the relationship between the level of demand in the reading room and the digitisation of the physical collections.

Regarding the use of the collections, the different types of requests are represented by three stocks:
(1) Requests in the reading room,
(2) Requests within the digitisation programme SoD,
(3) Requests for digitisation initiated by the institution, .
The purpose of the model is to simulate the decrease in , due to the digitisation programmes (
and
).
In this model, the stocks represent archival documents as aggregates that can flow between four stocks, according to whether they have been digitised and/or requested. No information on the number of requests per record is included. There are four stocks referring to the collection:
(1) Records never requested or digitised,
(2) Records requested, but not digitised,
(3) Records never requested, but digitised,
(4) Records requested and digitised,
The unit of all stocks is an archival record, including the digitisation stocks. There is only one exception: the stock representing requests in the reading room. As an archival record can be accessed more than once a year in the reading room, the unit of the stock representing the accesses in the reading room is an access request.
shows that there is only a feedback loop in the model. How the records from the state ”non requested” () flows to the state ”requested” (
) depends on the portion of the requests in the reading room
requested for the first time. In the experiments section, we will show that this feedback loop is a crucial part of the model. The other inputs are for now exogenously modelled, and the input values are based on the dataset (see, in experimental results section).
Table 1. List of variables assigned to the agents.
Table 2. Input variables of the system dynamics model.
4.1.1. Mathematical model
The main output of the model is how the annual number of requests () changes during the computational experiment. We assume that the main mechanism that explains the change in
, in this case the decrease, is related to the fraction of the collection that annually becomes digitally available and it is defined as follows:
where refers to the fraction of records that have been ever requested in the reading room and also digitised, and
is the fraction of records digitally available that have not been requested in the reading room.
Let us define as the annual increase of requested records that have been digitised, expressed as a fraction:
A part of the collection that has not been requested is also digitised. We can assume that there is a chance that records that might be requested for the first time have already been digitised. Therefore, the model also includes the impact of the collection that became digitally available, even for those records that have not been requested in the reading room before. However, as for this group of records there are no data available on digital access, can only be expressed as a function of the collection becoming digitally available:
where the constant modifies the total fraction of non-requested records that have been digitised, denoting that an unknown number of records would have been accessed in the reading room for first time if they had not been digitally available.
In order to calculate EquationEquation 2(2)
(2) and EquationEquation 3
(3)
(3) , we need to define how the four stocks that form a collection change over time. We start defining the part of the collection that has been requested but not digitised (
) as follows:
where , the increase of records requested for the first time, is calculated using
, assuming that a fraction of
is requested for the first time:
where modifies
as a fraction.
The requested records, , that become digitally available,
, is the result of the two digitisation programmes:
where is the number of records digitised within SoD and
as block digitisation. As this part of the model only represents the accessed records,
and
are respectively modified by
and
.
Regarding the records that have not been accessed, then decreases due to the access of records for the first time, as well as due to the digitisation of this part of the collection, according to the following equation:
To simplify the model, it is assumed that the collection size is constant, as this is not a key parameter in the model.
Similar to the digitisation of requested records, the digitisation of non-requested records depends on the number of requests within the two digitisation programmes of SoD and block digitisation:
The system dynamics model was built in Microsoft Excel, which makes it accessible to a wide user base including decision-makers in archives.
4.2. Agent-based modelling
ABM allows to introduce more detail in the model. Rather than using stocks representing a group of records, in ABM we can describe the individual characteristics of the archival records. In this case, we can take into account not only whether records have been accessed but also whether they have been repeatedly requested in the reading room. Like in the SD model, the aim of the ABM model is to calculate the decrease in requests in the reading room since digitisation of the collection began, as part of a sensitivity analysis. Whereas in SD we made the distinction between accessed and non-accessed records, ABM might be able to reproduce the ”digitisation match”, once the number of times that individual records have been accessed is also included.
The first step of the modelling process consists of the creation of a population of agents. In this model, the population is the whole archival collection, while agents are the archival records. Different variables characterise the agents (). Agents are characterised by a Boolean variable that defines whether an agent has been requested (requested). A second Boolean variable (popular) states whether the agent is repeatedly requested. Whereas the instances of use for agents that are requested, but not popular, will be equal to one, the instances of access will accumulate for those that are popular as long as the object is not digitally available. The annual instances and the accumulation of instances of access are also variables that characterise the agents (requestsYear, requestsTotal). In addition, a statechart is created with two states: InUse and DigitalAvailable. By default, the agents are in state InUse and the transition to the next state (DigitalAvailable) occurs when the Boolean variable digitised becomes true. When the agents become digitally available, then the accumulation of instances of access stops (requestsTotal).
At initialisation, the agents representing the records requested once are set using a random Boolean function (random TRUE (), where
is the probability of true). During the run, the same random function will set new records as requested once. Only those agents with instances of access (requestsTotal) equal to 0 can be selected. The records that are repeatedly requested also increase during the run. For those records, the instances of access (requestsYear) are set using a probability distribution, and the accumulation of instances of access (requestsTotal) is updated.
At run-time, the agents follow three steps which take place annually:
(1) The number of agents that are requested for the first time is updated.
(2) If agents are not digitally available, they accumulate instances of access (requests) according to the assigned probability distribution.
(3) If agents are not digitally available, they can be selected to be digitised.
Annually, the number of agents that are digitised is chosen according to the number of requests for digitisation. That happens in three steps:
(1) The number of requests ( and
) is converted to the proportion of the collection, which is used to calculate the proportion of agents to be digitised.
(2) The agents are then randomly chosen to be digitised if they are in state InUse and depending on the accumulated instances of access (requestsTotal).
Similarly to EquationEquation 1(1)
(1) in the SD model, the last step is the calculation of the decrease in requests in the reading room (
) according to:
but in ABM is not modified by the percentage of requested records that are digitised, but by the accumulated requests of the records that become DigitalAvailable. Therefore,
(“digitisation match”), is defined as follows:
where are the agents at least once requested in the reading room (requested = true) and from this group
are those agents which have become DigitalAvailable in that year.
The model was built using the software AnyLogic 8.6.
5. Experimental results
5.1. SD experiments
In these experiments, the SAA dataset was used as input to simulate the period from 2006, when the digitisation programmes started, to 2018. In model initialisation, the collection consists of one million records and the fraction of records requested is 0.1. The collection size is assumed to be constant and the growth of the collection is neglected, since no data was available on the total number of records that forms the collection during this period. In the input values used for these experiments are summarised.
A total of four experiments were conducted. In each experiment, a new factor of the model was added as follows:
Experiment 1: All digitised records have a direct impact on the reading room requests, .
Experiment 2: Only digitised records that have been requested in the reading room, , have an impact on
.
Experiment 3: The same as experiment 2, but taken into account that every year new records are accessed for the first time, (EquationEquation 5
(5)
(5) ). At the end of the experiment, 15% of the collection has been accessed.
Experiment 4: The same as experiment 3, but the impact of the records never digitised in the reading room, , is also included and modified by
, a hypothetical weight (EquationEquation 3
(3)
(3) ).
The results show that Experiment 1 is not a realistic scenario. In this experiment, where all digitised archival records ( and
) contribute equally, the effect of digitisation on decrease in the access requests in the reading (
) is overestimated (). Interestingly, a better fit to the actual requests (Experiment 2) is obtained when only the archival records that have been requested and digitised (
) are included. However, in Experiment 2 a factor is still missing, namely that every year new archival documents are requested for the first time (
). This factor is important as it modifies the fraction of requested records that are digitally available (
), according to Equationequations 4
(4)
(4) and Equation5
(5)
(5) . Experiment 3 is therefore a more realistic scenario than the one presented in Experiment 2. However, although Experiment 3 reproduces a steady decrease in requests from 2006 to 2014, it fails to reproduce the sharper fall from 2015.
Figure 6. Comparison of the decrease in access requests according to Amsterdam city archives dataset and the output of four experiments conducted according the system dynamic approach.

In Experiment 4, we tested whether a better fit could be obtained throughout the simulated period if a hypothetical correction was applied. In this experiment, the never requested but digitised archival records were included, but instead of contributing equally to the decrease in requests (Experiment 1), this contribution was modified by a hypothetical constant value () in this experiment. Different values of
were tested, until the best fit was found.
However, no actual data is available to support this hypothetical value of . On the contrary, in the analysis of the SAA dataset, we found evidence that not only the requested archival documents but also the number of times that they have been accessed are variables that need to be taken into account when modelling the change in the number of requests in the reading room. In the next section, we test whether the sharp decrease in requests from 2015 that could not be predicted in the SD approach (Experiment 3) is obtained when the individual number of instances of access of the archival documents is also included in the model.
5.2. ABM experiments
Like in the SD experiments, the aim of the ABM approach is to predict the decrease in requests () from 2006 to 2018, using the SAA dataset as input values. However, compared to the transparency and straightforwardness of the SD model, the ABM requires more input values in several steps to define the collection, as shown by the following description of the experiment:
(1) The collection size is 1.000.000 archival records.
(2) The number of agents is 4000, except for the experiments where the importance of the number of agents is tested. In those experiments, we run the same experiment but each time with a different number of agents: 1000, 2000, 4000 and 6000.
(3) The percentage of the collection that has been requested increases every year. At the end of the run, 15% of the collection (agents) has been requested, and ca. 55% of the requested agents has been requested just once (requestsTotal = 1).
The probability of an agent being accessed once is assigned with a binomial random distribution. At initialisation, the probability parameter is of 0.009. (equivalent to an average of 378 agents (standard deviation 4.9) in ten repeated runs). During the run, the probability parameter is changed to
of 0.002. These two probabilities are chosen to obtain the number of records requested as found in the SAA dataset prior to 2006 (first year of the run) and in 2018 (last year of the run). Only agents with no instances of access (requestsTotal = 0) can be selected.
Regarding the agents that will accumulate more than one instance of access, those agents (popular = TRUE) are selected with a probability of 0.05 at initialisation. During the run, the increase of this group is 0.2% of the whole population per year.
(4) We model the initial requests of the popular agents, those accumulating access requests. The best fit to the data was obtained with an exponential distribution with a rate of at initiation. We only apply this distribution to accessed items, which means that the minimum value is 1.
The data shows that the popularity of items changes every year. However, the accumulation of instances of access tends to be gradual, rather than sudden. There are many possible ways to model this. One method that offers a good fit to the data is to consider that the number of accesses of each item (requestsYear) remains the same or increases by a randomly distributed amount per year. The best fit is obtained with a normal distribution of and standard deviation
to model the annual instances of access. Because the accumulation of instances of access (requestsTotal) cannot decrease, only positive values are added up to the accumulation of instances of access (requestsTotal). Investigating the dynamics of the change of popularity, however, was not an objective of this paper.
(5) The annual number of agents digitised within the SoD and the block digitisation programme ( and
) as well as the match percentage of SoD and block digitisation (
and
) is based on the SAA dataset.
(6) Agents are selected for digitisation if they are in state InUse and depending on the instances of use (requestsTotal 1 and requestsTotal
1). The proportion of these two groups is based on the SAA data.
Despite the effort to reproduce the use of the collections as closely to the actual data as possible, some level of information is lost in the ABM model compared to the actual data. In this experiment with 4000 agents, one agent is equivalent to 250 archival records. The conversion of input values to agents will lead to some loss of information when the input value (e.g., digitised records) is smaller than 250 archival records. In addition, as explained in point 4, the cumulative frequency distribution of requests seen in , was not obtained with a yearly Pareto distribution seen in the actual data, but an initial exponential frequency distribution modified by the annual normal distribution gave the best fit.
Therefore, to test whether we were reproducing the same data of the SAA, two output values were used during the experiments as a control output: percentage of records requested and digitised and the instances of access digitised (21% and 31%, respectively, according to the SAA data).
In the first experiment, we explored the effect of the number of agents on the variability of the output in repeated runs. To explore this aspect, each experiment was repeated 10 times. shows that a higher variability can be expected from a lower number of agents, but from a certain number of agents in this case, 4,000, the expected variability remains similar. It is also worth to notice that only the models containing more than 4,000 agents reproduced correctly the expected outcome of the number of access in the reading room.
Figure 7. Comparison of the ABM output (number of access requests in the reading room at the end of the run, ) depending on the number of agents. Error bars indicate the standard deviation.

The ABM experiments confirmed that the accumulated instances of access of the heavily requested records are an important element in order to reproduce the results of the reference dataset (). It is important to notice that the model distinguishes between those agents requested once and those requested more than once. Choosing the right proportion of agents within each of these two groups is key to obtain accurate results. The proportion of the agents requested once is based on the data analysis of the SAA dataset. However, in this model, the selection of the agents requested more than once occurs randomly, and no further criteria of the instances of access (requestsTotal) is applied.
Figure 8. Comparison of the decrease in access requests according to Amsterdam city archives dataset and the output of the system dynamic model and three ABM experiments.

Hence, in the next experiments, we investigated whether similar results could be obtained if, rather than modelling the number of access of each agent according to a distribution, a fixed value of the instances of access is assigned to the repeatedly accessed agents (popular = TRUE). Two possibilities were tested: in one experiment, the agents accumulated every year 2 instances of access as long as they were not digitally available, and in the second experiment, 20 instances of access. Interestingly, shows that when two instances of access are assigned, similar results are obtained to those seen in the SD model, where no distinction was made between accessed once and repeatedly accessed. However, when agents are assigned with 20 instances of access, then the output is close to the one where the instances of access were modelled using a frequency distribution. It seems that assigning 20 instances of access works as the average of the values resulting from the Pareto distribution.
6. Digitisation strategies
In this section, we present how the model can inform collection management decisions by simulating the impact of digitisation strategies on the traditional access of the collections in the reading room. In the presented example, we explore at what point the SoD requests () are expected to surpass the number of requests in the reading room (
), as more and more records become digitally available. Knowing when this will happen is important to managers because it indicates that the reading room can probably be replaced by the Scan on Demand service.
In order to answer this question, we performed several runs to model the number of requests in the reading room for a period of 10 years, according to four different scenarios. The output of the ABM model using the SAA dataset seen in the previous section was taken as starting point of the run. summarises the four runs, with different inputs for the number of records digitised per year ( and
) and the SoD and block match (
and
).
Table 3. Description of four runs to model the effect of digitisation on the number of requests in the reading room.
As seen in the model description, the output of the ABM model is the annual number of requests (), which is not necessarily equivalent to records, as archival records can be accessed more than once in a year, resulting in several access requests per record. On the contrary, the unit of digitisation requests always refers to records. Therefore, shows the predicted decrease in the number of records, which are the access requests (
) converted to records by applying a correction factor of 1.5 (based on the annual average of requests per record in the SAA dataset).
Figure 9. Decrease in the number of requested records in the reading according to four digitisation strategies as described in Table 3. The dashed line represent a digitisation production of 5,000 and 10,0000 records per year.

The calculations show that only if the present digitisation production of 5,000 records per year is increased to 10,000, then within 10 years the turning point will be reached when the annual number of requests in the reading room will be similar to the annual scanning on demand production, which means that by then replacing the reading room service by the scanning on demand will become an option. The results also show that different strategies (e.g., exclusively SoD and/or block digitisation programme) produce similar outputs, as long as a production of 10,000 records is maintained.
Such strategy of doubling the scan production comes at a cost. Looking at the costs involved in the two processes of providing access of the collections, physically and digitally, the costs of maintaining the reading room open will remain similar over the years, whereas the costs for digital storage will annually rise due to the increasing amount of data generated by the scanning of the collections. Often the creation of digital content (the action of scanning the archival records) is seen as the most expensive step of digitisation (Beagrie, Citation2010; Poole, Citation2010). However, in the mid-long term, digital storage to provide fast online access to the collections might become even more expensive than the process of scanning, due to the increasing number of scans being stored. This is already the case at the SAA. Therefore, digitisation is a relatively expensive investment that must be based on a clear vision with regard to service provision and further developments on how collections will be used.
The costs of digitisation vary depending on the location. However, once the costs are known for a specific archival institution, the capabilities of this model could be easily be extended to economic forecasts. In the case of the Amsterdam City Archives, ca. half of the investment needed to maintain a production of 10,000 records digitised per year could be covered by the cost savings of suspending the reading room service. However, when an institution contemplates the option of shifting to a fully digital access service, two aspects need to be considered. First, not only the quality of the scan, but even more importantly, the delivery time of the scan will effect the readers’ satisfaction. And secondly, the need of providing physical access to a certain part of the collection will remain, either because standard digitisation of those records is not possible, or because readers will still require to access the physical records in cases where other values, such as the materiality of the objects, matters.
7. Conclusions
In this study, we proposed two modelling approaches, system dynamics and agent-based modelling, to investigate the effect of digitisation on the level of demand of archival documents in the reading rooms of institutions. Based on the data analysis conducted on the actual usage data of the Amsterdam City Archives (SAA), we identified the main hypothesis that might explain the observed decrease in the number of access requests, namely the impact of digitisation projects. This analysis formed the basis for the development of the proposed simulation models.
The data analysis indicated that there is evidence to support the hypothesis that the digitisation of the original collections will eventually result in the decrease of access requests in the reading room. How fast digital access will overtake the traditional physical access depends on how digitisation strategies are targeting those archival records that have been (heavily) requested by the readers. In this regard, we found that Scan on Demand is performing better than the projects initiated by the institution where (part of) whole collections are selected for digitisation.
The relationship between digitisation of the collections and the use of the original collections was further investigated using simulation. The system dynamic approach showed that making those archival documents digitally available that had not been requested before has an almost negligible effect on the observed decrease in the access requests in the simulated period of almost 15 years. The observed decrease in access requests can be mostly explained by the increase in at least once requested records becoming digitally available. However, the results of the sensitivity analysis show that the system dynamics model slightly underestimated the expected decrease in access requests in the reading room. The system dynamics model does not take into account the popularity of the archival records, understood as the number of access requests per record. Therefore, it can be expected that the SD approach will produce more satisfactory predictions for those collections where the group of repeatedly requested records accounts for a relatively small percentage of the total of requests.
More accurate fit in the decrease in access requests were obtained when the agent-based simulation approach was applied. In this model, agents represented archival documents with own characteristics, for instance, the number of access requests per year. The results of the ABM confirmed that the digitisation of heavily requested records will have a more noticeable impact on the reduction of access requests compared to records that have only been requested once in the past. A frequency distribution was used in order to model the instances of access. However, further investigation showed that it is not essential to reproduce the Pareto distribution of the number of access requests observed in the actual collection in order to obtain satisfactory results. The ABM experiments pointed out that making the differentiation between these two groups, records requested once and those with a high level of demand, might be sufficient to model the use of the collections in the reading room. In view of these results, we expect that if the differentiation between these two is included in the system dynamics model the accuracy of the results could be improved, since the level of detail of ABM seems not to be essential.
The presented models should be seen as baseline models where a decrease in the number of requests in the reading room is a response to the collections becoming digitally available. In such case, we are assuming that other factors identified in the causal loop diagram remain unchanged, such as the preference of the readers to use Scan on Demand instead of visiting the reading room. For now the results of the presented model, which only include a few key variables, indicate that this simple model is valid to explore how Scan on Demand is succeeding in digitising the most frequently requested records compared to other digitisation strategies, and, eventually, at what point it is expected that the corpus of the collection digitally available is significant enough to start seeing changes on the traditional use of the collections in the reading room.
The behaviour of the readers is a crucial part of the system that has been omitted for now, but it could be included in future models. Having a more elaborated model, then archives could use simulation modelling to explore whether certain measures (e.g., reducing the delivery time of scans, improving cataloguing, etc.) will effect how collections are used, but also at what cost. This information will support the development of (digitisation) strategies that aim to enhance the access of the collections, and to anticipate whether work processes need to be adjusted in response to the shifting use of physical collections to digital scans.
This study has provided valuable information on the frequency of use of the physical collections. In the future work, we will further expand the model by linking the use and preservation of the collections to one single model, to explore how digitisation strategies can contribute to lessening the risk of wear and tear of the most vulnerable collections.
Acknowledgments
The support of the Amsterdam City Archives throughout the project is gratefully acknowledged. Thanks are due to Nelleke van Zeeland for insightful discussion. The authors would like to acknowledge the financial support of Metamorfoze, the Dutch National Program for the Preservation of Paper Heritage, and the EPSRC Centre for Doctoral Training in Science and Engineering in Arts, Heritage and Archaeology (SEAHA).
Disclosure statement
No potential conflict of interest was reported by the author(s).
Additional information
Funding
References
- Ahmed, A., Greensmith, J., & Aickelin, U. (2012). Variance in system dynamics and agent based modelling using the SIR model of infectious disease. Proceedings - 26th european conference on modelling and simulation, ECMS 2012.
- Andro, M. (2018). Digital libraries and crowdsourcing. John Wiley & Sons.
- ARA National Surveys Group. (2019). Survey of Visitors to UK Archives 2018. https://static1.squarespace.com/static/60773266d31a1f2f300e02ef/t/6082ce882cf05f454db26b6c/1619185289634/Survey_of_Visitors_to_UK_Archives_2018_-_National_Headline_Report_.pdf
- Bantin, J., & Agne, L. (2010). Digitizing for value: A user-based strategy for university archives. Journal of Archival Organization, 8(3–4), 244–250. https://doi.org/10.1080/15332748.2010.550791
- Beagrie, N. (2010). Keeping research data safe factsheet. Cost issues in digital preservation of research data. https://www.beagrie.com/static/resource/KRDS_Factsheet_0711.pdf
- Brown, L. A. (2003). Useful or useless use statistics? A summary of conference presentations on usage data from the 22nd annual Charleston conference, issues in book and serial acquisition. Serials Review, 29(2), 145–150. https://doi.org/10.1080/00987913.2003.10764814
- Campagnolo, A. (Ed.). (2020). Book conservation and digitization: The challenges of dialogue and collaboration. ARC Humanities Press.
- Cassidy, R., Singh, N. S., Schiratti, P. R., Semwanga, A., Binyaruka, P., Sachingongu, N., Chama-Chiliba, C. M., Chalabi, Z., Borghi, J., & Blanchet, K. (2019). Mathematical modelling for health systems research: A systematic review of system dynamics and agent-based models. BMC Health Services Research, 19(11), 5–17. https://doi.org/10.1186/s12913-019-4627-7
- Chapman, J., & Yakel, E. (2012). Data-driven management and interoperable metrics for special collections and archives user services. RBM: A Journal of Rare Books, Manuscripts, and Cultural Heritage, 13(2), 129–151. https://doi.org/10.5860/rbm.13.2.379
- Cimler, R., Tomaskova, H. K., Dolezal, J., Pscheidl, P, O., Kuca, K., & Kuca, K. (2018). Numeric, agent-based or system dynamics model? Which modeling approach is the best for vast population simulation? Current Alzheimer Research, 15(8), 789–797. https://doi.org/10.2174/1567205015666180202094551
- Darabi, N., & Hosseinichimeh, N. (2020). System dynamics modeling in health and medicine: A systematic literature review. System Dynamics Review, 36(1), 29–73. https://doi.org/10.1002/sdr.1646
- Davahli, M. R., Karwowski, W., & Taiar, R. (2020). A system dynamics simulation applied to healthcare: A systematic review. International Journal of Environmental Research and Public Health, 17(16), 1–27. https://doi.org/10.3390/ijerph17165741
- Dillon, C., Lindsay, W., Taylor, J., Fouseki, K., Bell, N., & Strlič, M. (2012). Collections demography: Stakeholders’ views on the lifetime of collections. Climate for collections conference. Munich, doerner institut 79,4558.
- Duran-Casablancas, C., Strlič, M., Beentjes, G., de Bruin, G., van der Burg, J., & Grau-Bové, J. (2021). A comparison of preservation management strategies for paper collections. Studies in Conservation, 66(1), 23–31. https://doi.org/10.1080/00393630.2020.1790264
- Erway, R., & Schaffner, J. (2017). Shifting gears.Gearing up to get into the flow (2nd ed.). OCLC Research.
- European Commission (2021). Shaping Europe’s digital future.Digital cultural heritage Available online https://digital-strategy.ec.europa.eu/en/policies/cultural-heritage. Accessed on 31 January 2022
- Homer, J. B., & Hirsch, G. B. (2006). System dynamics modeling for public health: Background and opportunities. American Journal of Public Health, 96(3), 452–4582. https://doi.org/10.2105/AJPH.2005.062059
- IFLA. (2002). Guidelines for digitization projects for collections and holdings in the public domain, particularly those held by libraries and archives. https://www.ifla.org/files/assets/preservation-and-conservation/publications/digitization-projects-guidelines.pdf
- Kelly, E. J. (2014). Assessment of digitized library and archives materials: A literature review. Journal of Web Librarianship, 8(4), 384–403. https://doi.org/10.1080/19322909.2014.954740
- Kemp, J. (2016). How digitisation integrates in the world of archives preservation. Journal of the Institute of Conservation, 39(1), 57–63. https://doi.org/10.1080/19455224.2015.1105836
- Ling, T., & McLean, A. (2004). Taking it to the people. Why the national archives of Australia embraced digitisation on demand. Australian Academic and Research Libraries, 35(1), 2–15. https://doi.org/10.1080/00048623.2004.10755253
- Lipsitz, L. A. (2012). Understanding health care as a complex system: The foundation for unintended consequences. JAMA - Journal of the American Medical Associatio, 308(3), 243–244. https://doi.org/10.1001/jama.2012.7551
- Liu, S., Xue, H., Li, Y., Xu, J., & Wang, Y. (2018). Investigating the diffusion of agent-based modelling and system dynamics modelling in population health and healthcare research. Systems Research and Behavioral Science, 35(2), 203–215. https://doi.org/10.1002/sres.2460
- Macal, C. M. (2010). To agent-based simulation from system dynamics. Proceedings of the 2010 winter simulation conference, Eds.B. Johansson, S. Jain, J. Montoya-Torres, J. Hugan, & E. Yucessan,371–382.
- Martell, C. (2008). The absent user. physical use of academic library collections and services continues to decline 1995-2006. Journal of Academic Librarianship, 34(5), 400–407. https://doi.org/10.1016/j.acalib.2008.06.003
- Nauta, G. J., van den Heuvel, W., & Teunisse, S. (2017). Europeana DSI 2-access to digital resources of European heritage deliverable D4.4. Report on ENUMERATE core survey 4. https://pro.europeana.eu/files/Europeana_Professional/Projects/Project_list/ENUMERATE/deliverables/DSI-2_Deliverable%20D4.4_Europeana_Report%20on%20ENUMERATE%20Core%20Survey%204.pdf
- Nianogo, R. A., & Arah, O. A. (2015). Agent-based modeling of noncommunicable diseases: A systematic review. American Journal of Public Health, 105(3), e20–e31. https://doi.org/10.2105/AJPH.2014.302426
- Oliver, J. (2011). The digital archive. In L. Hugues (Eds.). Facet, Evaluating and measuring the value, use and impact of digital collections. London:Facet Publishing. (pp. 49–60 / Chapter 4).
- Pickford, C. (2002). Archives: A statistical overview. Cultural Trends, 12(48), 1–36. https://doi.org/10.1080/09548960209390339
- Plsek, P. E., & Greenhalgh, T. (2001). The challenge of complexity in health care. British Medical Journal, 323(7313), 625–628. https://doi.org/10.1136/bmj.323.7313.625
- Poole, N. (2010). The cost of digitising Europe’s cultural heritage. Collection Trust.
- Salleh, S., Thokala, P., Brennan, A., Hughes, R., & Booth, A. (2017). Simulation modelling in healthcare. An umbrella review of systematic literature reviews. PharmacoEconomics, 35(9), 937–949. https://doi.org/10.1007/s40273-017-0523-3
- Schaffner, J., Snyder, F., & Supple, S. (2011). Scan and deliver. Managing user-initiated digitization in special collections and archives. OCLC Research.
- Strlič, M. (2013). Collections demography part 1. On dynamic evolution of populations of objects. Collections Demography colloquium, 23 July 2013, UCL Centre for Sustainable Heritage. https://www.youtube.com/watch?v=BaObm6D0Sn8&list=PLN1qgCLlJmUab1-CbTfX6gtXxTxH2QM-F&index=1
- Strlič, M., Thickett, D., Taylor, J., & Cassar, M. (2013). Damage functions in heritage science. Studies in Conservation, 58(2), 80–88. https://doi.org/10.1179/2047058412Y.0000000073
- Valtysson, B. (2017). From policy to platform: The digitization of Danish cultural heritage. International Journal of Cultural Policy, 23(5), 545–561. https://doi.org/10.1080/10286632.2015.1084300
- VanSnick, S., & Ntanos, K. (2018). On digitisation as a preservation measure. Studies in Conservation, 63(Suppl.1), 282–287. https://doi.org/10.1080/00393630.2018.1504451