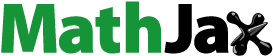
Abstract
Aim: This study addresses the challenge of predicting the response of head and neck squamous cell carcinoma (HNSCC) patients to immunotherapy. Methods: Using DNA methylation cytometry, we analyzed the immune profiles of six HNSCC patients who showed a positive response to immunotherapy over a year without disease progression. Results: There was an initial increase in CD8 T memory cells and natural killer cells during the first four cycles of immunotherapy, which then returned to baseline levels after a year. Baseline CD8 T cell levels were lower in HNSCC immunotherapy responders but became similar to those in healthy subjects after immunotherapy. Conclusion: These findings suggest that monitoring fluctuations in immune profiles could potentially identify biomarkers for immunotherapy response in HNSCC patients.
While immunotherapy has improved prognosis, the average percentage of head and neck squamous cell carcinoma (HNSCC) patients who respond to immunotherapy remains low at 15–20%.
There are still significant gaps in understanding immunotherapy response, including a limited clinical ability to identify patients who would benefit from immune checkpoint inhibitors.
This study revealed significant variations in the complete immune profile of these patients at different treatment stages, from the baseline to the 18th treatment cycle.
There was an initial increase in CD8 T memory cells and natural killer cells during the first four cycles of immune checkpoint inhibitor therapy, which then returned to baseline levels after a year.
compared with healthy individuals, HNSCC responders had significantly lower levels of CD4 T and B cells and higher levels of monocytes, both at baseline and throughout the year of immune checkpoint inhibitor treatment.
Baseline CD8 T cell levels were lower in HNSCC immunotherapy responders but became similar to those in healthy subjects after immunotherapy.
1. Background
Head and neck squamous cell carcinoma (HNSCC) accounts for about 4% of malignancies in USA [Citation1]. In 2023, it is estimated that about 66,920 individuals will be diagnosed with HNSCC and that 15,400 deaths will result [Citation1]. The overall 5-year survival rate for all oral and pharyngeal cancer is low, at approximately 68% [Citation2]. The 5-year survival rate for advanced patients is even lower, at around 40% and over 50% of these patients will suffer a recurrence within 2–3 years [Citation2-4]. Radiation, chemotherapy and debilitating surgery are current standards of care for HNSCC.
Immune checkpoint inhibitors (ICIs; nivolumab and pembrolizumab) have recently been approved for advanced and metastatic HNSCC. While immunotherapy has improved prognosis [Citation5,Citation6], the average percentage of HNSCC patients who respond to immunotherapy remains low at 15–20% [Citation7]. There are still significant gaps in understanding immunotherapy response, including a limited clinical ability to identify patients who would benefit from ICIs. While some biomarkers like PD-1 and PD-L1 expression levels are potential indicators of response, no biomarkers are currently in place clinically for HNSCC patients [Citation8].
We previously developed a cell deconvolution method from DNA methylation profiles to estimate peripheral blood cell type proportions; this method is referred to as methylation cytometry [Citation9-11]. Methylation cytometry has been widely utilized to identify associations of peripheral blood immune cell composition in health and disease, including outcomes in cancer patients [Citation12-15]. In addition, this method has been applied to determine neutrophil-lymphocyte ratio (methylation-derived NLR [mNLR]), which is a well-studied prognostic factor for poor survival in malignant diseases, including head and neck cancer [Citation16-18].
This study aimed to provide potential clinical biomarker utility of methylation cytometry in HNSCC as there is a lack of biomarkers in monitoring response to immunotherapy in HNSCC patients. This study utilizes methylation cytometry to describe immune profile changes in the peripheral blood of HNSCC patients who responded to immunotherapy for 1 year without progression. Methylation cytometry provides a convenient method to track immune profiles longitudinally as fresh blood is not required and can determine immune profiles of additional, specific immune cell types as compared with traditional complete blood count differential tests. The immune profiles of responders were also compared with those of healthy donors to address whether immunotherapy response is related to restoring a healthy immune landscape. Our ultimate goal is to predict the response of head and neck squamous cell carcinoma patients to immunotherapy using methylation cytometry.
2. Materials & methods
2.1. Study population
Adults with HNSCC slated to undergo immunotherapy were prospectively enrolled at Dana Farber Cancer Institute (DFCI). Patients provided written consent to participate in the study, following the DFCI Institutional Review Board guidelines (IRB Protocol #: 18-548). Enrolled subjects received immunotherapy, or immunotherapy with chemotherapy, every 3 weeks. Blood from each patient was collected prior to the initial treatment and at each treatment during routine clinical blood draws. Of the enrolled subjects, six who survived for a year on immunotherapy or immunotherapy/chemotherapy treatment regimen without disease progression were included in this analysis. Subjects with treatment-terminating adverse effects were excluded in this study. Some blood samples of subjects included in the study were missing due to COVID-19 pandemic restrictions. Subjects in this group were composed of five males and one female with an average age of 65 years (range: 55–83). The description of each subject is detailed in . Longitudinal blood samples from an 11-month period from one healthy 30-year-old male subject were available for DNA methylation analysis.
Table 1. Head and neck cancer immunotherapy patient characteristics.
Longitudinal sampling for B, CD4 T, CD8 T and monocyte cell counts from healthy populations (n = 15), as measured by three colored flow cytometry, were abstracted from Backteman and Ernerudh [Citation19]. To extract values from the graphs, screenshots were captured and loaded into a ‘WebPlotDigitizer’ found at https://apps.automeris.io/wpd/. The plot type selected was ‘2D (X-Y) Plot’. Axes were aligned by clicking two known points on the x-axis (X1, X2) and two known points on the y-axis (Y1, Y2). Based on this scale, the center of the data points was estimated.
2.2. Methylation cytometry
Blood DNA was extracted using Zymo Quick-DNA Miniprep Plus kit (Catalog #D4069) following the manufacturer's instructions. DNA was quantified using Qubit Broad Range assay (Thermo Scientific Catalog #Q32853), per manufacturer's instructions. DNA was sent to the Avera Institute of Human Genetics (SD, USA) for methylation measurement on the Illumina MethylationEPIC v1.0 Beadchip. The samples from the healthy donor was measured with Illumina Methylation450 Beadchip. To prepare for DNA methylation-based immune cell deconvolution, raw BeadArray data were preprocessed using the normal-exponential out-of-band (Noob) pipeline from minfi, including single-sample background and dye bias correction [Citation20]. The Noob pipeline was recommended for the 12-immune-cell-type deconvolution [Citation11]. Once processed, FlowSorted.BloodExtended.EPIC was used to estimate the proportions of 12 immune cell types in whole blood [Citation11].
As in our previous publication [Citation21], we calculated the EAA (Extrinsic epigenetic Age Acceleration) using the residuals of the linear model of Horvath's age as the outcome and the chronological age as the exposure to evaluate the increase in biological aging for the subjects. We also calculated the IAA (Intrinsic epigenetic Age Acceleration) using the residuals of the linear model of Horvath's age [Citation22] as the outcome and the chronological age as the exposure adjusted for the immune cell composition (12 cell types) to evaluate the increase in biological aging for the subjects unrelated to changes in the leukocyte composition. To avoid multicollinearity and remove the exact zero constraints, the proportions of the scaled immune cell composition (bound between 0 and 1) were transformed using the Smithson & Verkuilen [Citation23] procedure before adjustment.
2.3. Statistical analysis
PERMANOVA analysis was performed to evaluate complete, longitudinal immune profiles of HNSCC patients who responded to immunotherapy. PERMANOVA analysis using the Aitchison distance metric was conducted with vegan (v.2.6-4) R package [Citation24,Citation25]. T-tests were performed to compare immune cell type counts between HNSCC patients who responded to ICI and healthy controls. Two-sided t-tests were performed with tsum.test function in BSDA (v.1.2.2) R package [Citation26]. The statistical significance threshold was set to p-value <0.05.
To measure and compare temporal variability in the cellular landscape between patient samples (N = 6) and with the healthy control sample (N = 1), an adapted version of the Consecutive Disparity Index (CDI) [Citation27] was applied. The CDI (EquationEquation 1(1)
(1) ) is a temporal variability index that takes into account the chronological order of the values, assessing the average rate of change between consecutive values. The formulation of the CDI is given below:
(1)
(1)
Where i = 1,2,…,N is an index for subject, t = 1,2,…,Ti is an index for time, and k = 1,2,…,K is an index for cell type. Finally, yik(t) represents the predicted cell proportion for cell type k in subject i at time t. The CDI is exactly equal to zero if there is no variation in yik(t) over all t (e.g., same value over time) and greater than zero otherwise. Larger values of the CDI are indicative of a greater magnitude of fluctuation in yik(t) over time. As the above formulation of the CDI assumes that the time intervals are equally spaced, we created an adapted version of the CDI (aCDI) that accounts for the unequal spacing between time intervals, a feature of our particular data. The formulation of the aCDI (EquationEquation 2(2)
(2) ) is as follows:
(2)
(2)
Where xi(t) represents the observed time (e.g., 2 months) at time t for subject i. In contrast to the CDI in which each time interval is treated equivalently, the aCDI weights each time interval inversely proportional to the difference in time between the (t + 1)th and (t)th time. Thus, a large variation in the response, y, occurring over a short time interval will have a larger impact on the final aCDI value than the same degree of variation in y over a longer time interval. The aCDI was independently computed for each cell type and subject (N = 7, patient samples plus healthy control), and the results were visualized using a heatmap.
3. Results
Six patients with HNSCC who received pembrolizumab (N = 5) or pembrolizumab with chemotherapy (N = 1) survived 1 year without progression (). Blood for methylation profiling was obtained from each patient during routine clinical draws, starting with pre-treatment (baseline) and continuing before each treatment at 3-week intervals (N = 67 blood samples). Some blood draws were not collected due to safety restrictions imposed during the early stages of the COVID-19 pandemic. Blood cell DNA methylation was measured using the Illumina HumanMethylation EPIC array platform. Immune cell type proportions and counts of 12 cell types were estimated with FlowSorted.BloodExtended.EPIC [Citation11]. Epigenetic age, extrinsic and intrinsic age acceleration at baseline and each treatment time point were estimated with Zhang et al.'s epigenetic clock [Citation21] (Supplementary Figure S1).
Within the initial four treatment cycles of ICI, relative and absolute memory CD8 T (CD8mem) cell levels and natural killer (NK) cells increased (, Supplementary Figure S2). The initial spike in memory CD8 T and NK cells decreased closer to baseline levels after 15–18 cycles of treatment (45–54 weeks following the initial immunotherapy treatment). Small increases in monocytes (Mono) were also observed within the first three cycles of immunotherapy. In contrast, neutrophil (Neu) proportions and counts decreased during the first four treatment cycles of immunotherapy. Although, levels of Neu also returned to near baseline levels in 15–18th cycles of ICI. A slight increase in memory and naïve B cells (Bmem and Bnv, respectively) was observed within the initial 10 cycles of immunotherapy. The levels of other cell types [basophils (Bas), eosinophils (Eos), naïve and memory CD4 T cells (CD4nv, CD4mem) and regulatory T cells (Treg)] as well as mNLR, remained relatively stable over the course of the year (Supplementary Figure S3). To further evaluate the complete peripheral immune profiles among long-term ICI responders, we conducted a PERMANOVA analysis using the Aitchison distance metric [Citation24,Citation25]. Our findings indicate statistically significant differences (999 permutations, F = 3.594, P = 0.009) in peripheral immune profiles across the treatment course, from baseline to treatment 18 (Supplementary Table S1).
Figure 1. Methylation-derived cell type proportions from peripheral blood for selected samples over approximately 1 year of immunotherapy. Each point indicates the median proportion at each treatment time point. Vertical bars around the point indicate the minimum and maximum proportion at each time point. Methylation cytometry methods reveal the proportions for 12 immune cell types for patients exhibiting response to immunotherapy treatment over the course of 18 cycles. While several cell types do not appear to show substantial changes over time, memory CD8 T and NK populations exhibit a pronounced boost over the initial 100 days (the typical time-frame for which progression-free survival is considered ‘response’) with a near return to pre-treatment baseline levels at the 1 year mark.

To quantify the changes in immune profiles of these patients from those that would be found in healthy individuals, we compared the immunotherapy responder immune profiles with publicly available immune profiles of healthy controls (A). Based on availability, samples for baseline through 3.5 and 10.5–12.5 months were selected for analyses (). Total B (memory and naive) and total CD4 T (naïve and memory) cells were significantly lower in the responders at baseline and throughout treatments. Only B cells at 11 months post-treatment showed no statistically significant difference between responders and healthy controls. Monocyte levels were significantly higher in the ICI responders at baseline and during the course of the treatment regimen.
Figure 2. Immune composition of head and neck squamous cell carcinoma immunotherapy responders in comparison to healthy subjects. (A) Although limited by longitudinal data availability for healthy donors, mean counts (1000/ul) for B, total CD4 T, total CD8 T cells (both naïve and memory sub-types for each population) and monocytes, are shown for healthy and HNSCC immunotherapy responders at baseline, time period of initial immunotherapy treatment cycles (1.5, 2 and 3.5 months following initial treatment), and at around the 1-year mark (10.5 and 11 months post start of treatment). Error bars indicate one standard deviation above and below the mean. Red columns indicate mean counts for healthy controls; blue columns indicate mean counts for HNSCC immunotherapy responders. Distribution of each cell type proportion between healthy donors and HNSCC immunotherapy responders were compared with t-tests. P-value for each comparison is indicated above the bars at each timepoints. (B) Heatmap of adapted consecutive disparity index (aCDI) for each subject per cell type where ‘P’ is the healthy subject reference and ‘S1’- ‘S6’ are responders. Larger values of the aCDI are indicative of greater temporal variability of cell type proportions.

As increases in memory CD8 T cells were observed in the initial ICI treatment cycles, we hypothesized that this increase would lead to similar proportions of CD8 T cells in HNSCC immunotherapy responders as observed in healthy controls. Despite lower mean counts, the total CD8 T cells were not significantly different from healthy controls at baseline or at any time throughout the treatment regimen (A). Following our hypothesis, the mean counts for total CD8 T cells of HNSCC immunotherapy responders were more comparable to the mean counts for healthy controls at 1.5 months post-treatment and at 10.5 and 11 months post-treatment as evidenced by no statistical differences in medians, and were higher at 2 and 3.5 months post-treatment although not statistically significant (A). Our results suggest immunotherapy may raise total CD8 T cell levels to be more comparable to healthy controls' total CD8 T cell levels.
Assessments of temporal variability in the immune composition in relation to cell counts derived from a DNA methylation cytometry of a healthy subject in the 1-year period were evaluated for each cell type within each subject, independently, using the aCDI. Larger values of the aCDI are indicative of greater temporal variability of some measurements. Our results showed that the healthy control has uniformly low and stable values of the aCDI across the cell types, whereas the patient samples exhibit more volatility across the different cell types (B). Across both the patient and healthy control samples, CD8 naïve cells, monocytes and neutrophils appear to be fairly temporally stable, whereas other cell types (Eos and CD4 memory and naive) exhibit more volatility within certain patient samples (B & Supplementary Figure S4). Interestingly, CD4 and CD8 naïve cells were much lower in the HNSCC subjects longitudinally compared with the healthy subject. However, some portion of the differences between the HNSCC subjects and the healthy donor is likely due to the difference in age. Together with our comparisons to cell counts derived from healthy subjects from flow cytometry, our results suggest immunotherapy may be raising specifically CD8 T memory cell counts to be similar to the levels found in healthy subjects.
4. Discussion
Although only 15–20% of HNSCC patients respond to immunotherapy, major gaps exist in understanding who will respond. To our knowledge, few studies have investigated longitudinal immune cell profiles for cancer patients who display a long-term response to ICIs, and no studies of HNSCC patients undergoing ICI immunotherapy have presented detailed immune profiles that include naïve and memory cell types or regulatory T cells.
The immune cell profiles were determined for six HNSCC patients with a durable response to ICI treatment: progression-free for 1 year. Dynamic changes in immune cell profiles were identified during immunotherapy throughout the course of 1 year of ICI. Clinically, patients are considered to be ‘responders’ when no progression has occurred on therapy at the 100-day (3.5 months) point. At this 100-days, increased levels of CD8 T memory cells and NK cells were identified, compared with pre-treatment/baseline levels. The CD8 T memory and NK cell levels then stabilized back to baseline within 10.5–12.5 months of treatment.
While the comparison of full immune profiles was restricted due to differences in cytometry methods and a lack of publicly available longitudinal immune profiles for healthy populations, this study begins to investigate/compare the profiles of immunotherapy responders to those of a healthy reference population. The consistent volatility in immune profiles of HNSCC ICI patients compared with healthy populations suggests a limited ability of immunotherapy to restore a ‘healthy’ immune profile, even in patients who have shown promising responses. Only the CD8 T memory population from immunotherapy responders exhibits a change (increase) in cell levels comparable to ‘healthy’ profiles following treatment. Although the current study did not reveal statistically significant differences in baseline CD8 T cell levels between HNSCC immunotherapy responders and healthy subjects, baseline CD8 T cells (total) exhibited the largest difference in CD8 T cells between the two groups. A larger sample size may identify significantly lower CD8 T cells prior to immunotherapy treatments in HNSCC immunotherapy responders compared with healthy controls.
Previous studies have suggested the role of immunotherapy in inducing dynamic changes of tumor-infiltrating CD8 T cells in the tumor microenvironment to provide therapeutic benefit in various tumor types, including HNSCC [Citation28,Citation29]. Moreover, CD8 T memory cells have been identified to increase upon other immune checkpoint blockade treatments and were associated with responding to immunotherapy in melanoma and other tumor types, but not in HNSCC [Citation30-32]. Supporting previous literature, our study was one of the first to identify that CD8 T memory cells increase in the peripheral blood of HNSCC immunotherapy responders to be of clinical utility. As the increases in CD8 T memory cells can be detected in the first few immunotherapy treatment cycles, our results suggest methylation cytometry-based immune profiling may be a useful tool to identify responders early in immunotherapy treatment for HNSCC.
Other oral diseases like periodontitis and preneoplastic oral lesions like oral lichen planus have been associated with development of oral squamous cell carcinoma [Citation33-35]. As the epigenetic modifiers like miRNA can play a role in malignant transformation into oral cancers [Citation36], examining other epigenetic factors may be important in understanding oral lesions. Furthermore, drugs like tacrolimus and other anti-inflammatory treatments have been found to reduce severity of oral lichen planus [Citation37]. Methylation cytometry may also be used to assess responders to other treatments in other oral diseases.
As only 15–20% of HNSCC patients respond to immunotherapy [Citation7], accrual of a larger sample size over a long period of time is difficult. Our conclusions are limited due to a smaller sample size for both HNSCC and non-diseased subjects. However, we incorporate numerous samples per subject across a span of a year, including 67 blood samples of HNSCC immunotherapy responders and 191 blood samples of healthy subjects. This study characterized the immune composition of 12 immune cell types from HNSCC long-term ICI responders, which, to our knowledge, has not previously been done. Moreover, while it is difficult to collect longitudinal samples from non-responders as they succumb to disease quickly, we expect to collect some longitudinal peripheral blood samples from non-responders as this study progresses. Further investigation with longitudinal data from HNSCC immunotherapy responders, non-responders and healthy subjects is needed to validate this study.
5. Conclusion
This study utilized methylation cytometry to determine levels of 12 specific immune cell types in HNSCC patients on immunotherapy, allowing tracking changes in immune cell proportions that are not measured using the standard complete blood count differential. Most notably, changes in CD8 memory T cells and NK cells were observed over the course of immunotherapy. Our results suggest the potential utility of methylation cytometry-derived immune cell profiles as biomarkers to understand better the effects of immunotherapies on the immune systems of HNSCC patients that enable long-term responses.
Author contributions
Study conception and design: BC Christensen, KT Kelsey. Data collection: R Butler, G Ramush, H Stolrow, K Sehgal, K Shirai, R Haddad. Analysis and interpretation of results: MKL, HS, ZZ, R Butler, DC Koestler, LA Salas, JK Wiencke, KT Kelsey, BC Christensen. Draft manuscript preparation: MK Lee.
Financial disclosure
This work was supported by National Institutes of Health (5R01CA253976-03) awarded to B.C.C and K.T.K and the National Cancer Institute Cancer Center Support Grant P30 CA168524. The authors have no other relevant affiliations or financial involvement with any organization or entity with a financial interest in or financial conflict with the subject matter or materials discussed in the manuscript apart from those disclosed.
Writing disclosure
No writing assistance was utilized in the production of this manuscript.
Ethical conduct of research
This study was approved by the Institutional Review Board at Dana Farber Cancer Institute (IRB Protocol #: 18-548). Patients provided written consent to participate in the study.
Data sharing statement
Data are available upon request.
Supplementary Figures S1-S4 and Supplementary Table S1
Download Zip (533.5 KB)Supplementary material
Supplemental data for this article can be accessed at https://doi.org/10.1080/17501911.2024.2343274
Competing interests disclosure
JK Wiencke and KT Kelsey are founders and scientific advisors to Cellintec, which had no role in this research. BC Christensen is an advisor to Guardant Health which had no role in this research. The authors have no other competing interests or relevant affiliations with any organization or entity with the subject matter or materials discussed in the manuscript apart from those disclosed.
Additional information
Funding
References
- Siegel RL, Miller KD, Fuchs HE, et al. Cancer statistics, 2022. Ca Cancer J Clin. 2022;72:7–33. doi:10.3322/caac.21708
- Howlander N, Noone A, Krapcho M, et al. SEER Cancer Statistics Review, 1975–2018 [Internet]. National Cancer Institute. 2021 [ cited 2023 Feb 11]. Available from: https://seer.cancer.gov/csr/1975_2018/
- Rettig EM, D'Souza G. Epidemiology of head and neck cancer. Surg Oncol Clin N Am. 2015;24:379–396. doi:10.1016/j.soc.2015.03.001
- Nissi L, Suilamo S, Kytö E, et al. Recurrence of head and neck squamous cell carcinoma in relation to high-risk treatment volume. Clin Transl Radiat Oncol. 2021;27:139–146. doi:10.1016/j.ctro.2021.01.013
- Cohen EEW, Soulières D, Tourneau CL, et al. Pembrolizumab versus methotrexate, docetaxel, or cetuximab for recurrent or metastatic head-and-neck squamous cell carcinoma (KEYNOTE-040): a randomised, open-label, Phase III study. Lancet. 2019;393:156–167. doi:10.1016/S0140-6736(18)31999-8
- Ferris RL, Jr GB, Jerome F, et al. Nivolumab for recurrent squamous-cell carcinoma of the head and neck. New Engl J Med. 2016;375:1856–1867. doi:10.1056/NEJMoa1602252
- Shibata H, Saito S, Uppaluri R. Immunotherapy for head and neck cancer: a paradigm shift from induction chemotherapy to neoadjuvant immunotherapy. Frontiers Oncol. 2021;11:727433. doi:10.3389/fonc.2021.727433
- Li H, van der Merwe PA, Sivakumar S. Biomarkers of response to PD-1 pathway blockade. Brit J Cancer. 2022;126:1663–1675. doi:10.1038/s41416-022-01743-4
- Houseman EA, Accomando WP, Koestler DC, et al. DNA methylation arrays as surrogate measures of cell mixture distribution. BMC Bioinform. 2012;13:86. doi:10.1186/1471-2105-13-86
- Salas LA, Koestler DC, Butler RA, et al. An optimized library for reference-based deconvolution of whole-blood biospecimens assayed using the Illumina HumanMethylationEPIC BeadArray. Genome Biol. 2018;19:64. doi:10.1186/s13059-018-1448-7
- Salas LA, Zhang Z, Koestler DC, et al. Enhanced cell deconvolution of peripheral blood using DNA methylation for high-resolution immune profiling. Nat Commun. 2022;13:761. doi:10.1038/s41467-021-27864-7
- Chen J-Q, Salas LA, Wiencke JK, et al. Immune profiles and DNA methylation alterations related with nonmuscle-invasive bladder cancer outcomes. Clin Epigenetics. 2022;14:14. doi:10.1186/s13148-022-01234-6
- Titus AJ, Gallimore RM, Salas LA, et al. Cell-type deconvolution from DNA methylation: a review of recent applications. Hum Mol Genet. 2017;26:R216–R224. doi:10.1093/hmg/ddx275
- Wiencke JK, Koestler DC, Salas LA, et al. Immunomethylomic approach to explore the blood neutrophil lymphocyte ratio (NLR) in glioma survival. Clin Epigenetics. 2017;9:10. doi:10.1186/s13148-017-0316-8
- Zhang Z, Stolrow HG, Christensen BC, et al. Down syndrome altered cell composition in blood, brain, and buccal swab samples profiled by DNA-methylation-based cell-type deconvolution. Cells. 2023;12:1168. doi:10.3390/cells12081168
- Koestler DC, Usset J, Christensen BC, et al. DNA methylation-derived neutrophil-to-lymphocyte ratio: an epigenetic tool to explore cancer inflammation and outcomes. Cancer Epidemiology Prev Biomarkers. 2017;26:328–338. doi:10.1158/1055-9965.EPI-16-0461
- Perisanidis C, Kornek G, Pöschl PW, et al. High neutrophil-to-lymphocyte ratio is an independent marker of poor disease-specific survival in patients with oral cancer. Med Oncol. 2013;30:334. doi:10.1007/s12032-012-0334-5
- Templeton AJ, McNamara MG, Šeruga B, et al. Jnci J National Cancer Inst. 2014;106(6):106:dju124. doi:10.1093/jnci/dju124
- Backteman K, Ernerudh J. Biological and methodological variation of lymphocyte subsets in blood of human adults. J Immunol Methods. 2007;322:20–27. doi:10.1016/j.jim.2007.01.021
- Aryee MJ, Jaffe AE, Corrada-Bravo H, et al. Minfi: a flexible and comprehensive Bioconductor package for the analysis of Infinium DNA methylation microarrays. Bioinformatics. 2014;30:1363–1369. doi:10.1093/bioinformatics/btu049
- Zhang Z, Reynolds SR, Stolrow HG, et al. Deciphering the role of immune cell composition in epigenetic age acceleration: Insights from cell-type deconvolution applied to human blood epigenetic clocks. Aging Cell. 2024;23:e14071. doi:10.1111/acel.14071
- Horvath S. DNA methylation age of human tissues and cell types. Genome Biol. 2013;14:3156. doi:10.1186/gb-2013-14-10-r115
- Smithson M, Verkuilen J. A better lemon squeezer? maximum-likelihood regression with beta-distributed dependent variables. Psychol Methods. 2006;11:54–71. doi:10.1037/1082-989X.11.1.54
- Dixon P. VEGAN, a package of R functions for community ecology. J Veg Sci. 2003;14:927–930. doi:10.1111/j.1654-1103.2003.tb02228.x
- Aitchison J, Greenacre M. Biplots of compositional data. J R Stat Soc: Ser C (Appl Stat). 2002;51:375–392. doi:10.1111/1467-9876.00275
- Kitchens LJ. BSDA: Basic Statistics and Data Analysis [Internet]. 2023. Available from: https://github.com/alanarnholt/BSDA
- Fernández-Martínez M, Vicca S, Janssens IA, et al. The consecutive disparity index, D: a measure of temporal variability in ecological studies. Ecosphere. 2018;9(12):9. doi:10.1002/ecs2.2527
- Zhou L, Zeng Z, Egloff AM, et al. Checkpoint blockade-induced CD8+ T cell differentiation in head and neck cancer responders. J Immunother Cancer. 2022;10:e004034. doi:10.1136/jitc-2021-004034
- Kurtulus S, Madi A, Escobar G, et al. Checkpoint blockade immunotherapy induces dynamic changes in PD-1-CD8+ tumor-infiltrating T cells. Immunity. 2019;50:181–194.e6. doi:10.1016/j.immuni.2018.11.014
- Tietze JK, Angelova D, Heppt MV, et al. The proportion of circulating CD45RO+CD8+ memory T cells is correlated with clinical response in melanoma patients treated with ipilimumab. Eur J Cancer. 2017;75:268–279. doi:10.1016/j.ejca.2016.12.031
- de Coaña YP, Wolodarski M, Poschke I, et al. Ipilimumab treatment decreases monocytic MDSCs and increases CD8 effector memory T cells in long-term survivors with advanced melanoma. Oncotarget. 2017;8:21539–21553. doi:10.18632/oncotarget.15368
- Jin Y, Tan A, Feng J, et al. Prognostic impact of memory CD8(+) T cells on immunotherapy in human cancers: a systematic review and meta-analysis. Front Oncol. 2021;11:698076. doi:10.3389/fonc.2021.698076
- Komlós G, Csurgay K, Horváth F, et al. Periodontitis as a risk for oral cancer: a case–control study. BMC Oral Heal. 2021;21:640. doi:10.1186/s12903-021-01998-y
- Tsushima F, Sakurai J, Uesugi A, et al. Malignant transformation of oral lichen planus: a retrospective study of 565 Japanese patients. BMC Oral Heal. 2021;21:298. doi:10.1186/s12903-021-01652-7
- Arduino PG, Magliano A, Gambino A, et al. Risk of malignant transformation in 3173 subjects with histopathologically confirmed oral lichen planus: a 33-year cohort study in Northern Italy. Cancers. 2021;13:5740. doi:10.3390/cancers13225740
- Polizzi A, Santonocito S, Distefano A, et al. Analysis of oral lichen planus severity on micro-RNA linked with malignant transformation risks. Oral Dis. 2023. doi:10.1111/odi.14758
- Polizzi A, Santonocito S, Giudice AL, et al. Analysis of the response to two pharmacological protocols in patients with oral lichen planus: a randomized clinical trial. Oral Dis. 2023;29:755–763. doi:10.1111/odi.13960