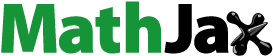
ABSTRACT
The ability to predict tourist movements has various practical applications, including recommendation, target marketing, and destination planning. Predictability determines the limit of the prediction accuracy of data and models and helps us understand the factors affecting the prediction accuracy. We first constructed a conceptual framework of factors influencing the predictability from three perspectives: tourist, destination, and space-time. In this study, we focused on factors affecting the tourist movement predictability using data collected from social media at the city level. We used two prediction models to understand the impact of the factors on predictability. We further analyzed the relationship between the factors and movement predictability. The results of this study demonstrate that the length of the tourist itinerary and the spatial scale of the study are key factors that influence model selection. In addition, the results indicate significant differences in the predictability of tourists with different tourism motivations.
1. Introduction
Understanding the predictability of tourist movement plays a fundamental role in the tourism studies. The abilities to predict tourist movement can be helpful in a wide range of applications, such as tourism recommendation, targeted advertisement, and transportation optimization. As location-based services are increasingly used in tourism applications, they have accumulated a massive amount of location data. These novel movement observations, including GPS data (G. Lau and McKercher Citation2006; Solomon et al. Citation2021; Xiao-Ting and Bi-Hu Citation2012; W. Zheng, Huang, and Li Citation2017), mobile phone data (Raun, Ahas, and Tiru. Citation2016; Xu et al. Citation2021; X. Zhao et al. Citation2018) and social media data (Majid et al. Citation2013; Su et al. Citation2020; Y. Zheng et al. Citation2021), have proved many factors shape the predictability and regularity of tourist movement.
Although several studies have focused on the movement predictability of usual human living areas, the movement predictability of tourists has not yet been widely discussed. Clearly, the movement predictability between city residents and tourists have obvious differences. Some studies have shown that certain factors affect the ability to predict tourist movements in destination cities, including distance (Xue and Zhang Citation2020), number of visits (McKercher et al. Citation2012), travel party size (X. Zhao et al. Citation2018), weather (McKercher et al. Citation2015) and socio-demographic characteristics (Cantis et al. Citation2016).
One reason for the lack of research on tourist movement predictability is that previous studies have used data that contain little information about individual tourists. Previous studies have used survey data with small sample sizes (East et al. Citation2017; Xia, Zeephongsekul, and Arrowsmith Citation2009; Xia, Zeephongsekul, and Packer Citation2011), which have more complete information about individual tourists but are limited by the large workload of manual research and generally small sample sizes, or mobile phone data (Crivellari and Beinat Citation2020; Xu et al. Citation2022), which have sufficient data volume, but the location data accuracy is affected by the distribution of the base stations. In addition, with telecommunication companies protecting user privacy, the information of individual tourists is limited.
Because the predictability of tourist movement is context-dependent (Xu et al. Citation2022), different prediction models may have different interpretations. This study applied a deep learning method and a traditional prediction method to better understand the nature of tourists' movements.
The remainder of this paper is organized as follows. Section 2 overviews related work. Here, we also mention some studies in the field of computer and information science. Section 3 describes the step-by-step performance of the prediction task. In Section 4, we analyze the results of the prediction and evaluate its accuracy. Section 5 discusses the real-life applications of our prediction model. Finally, in Section 6, we conclude the paper and suggest possible improvements to this study.
2. Literature review
2.1. Tourist movement prediction
Fennell (Citation1996) noted that the mapping and modeling of tourist movements was a worthwhile topic to explore. However, in the beginning, few researchers attempted to model the actual movement of tourists (Lew and McKercher Citation2006). This has been argued to be the case because such movements are fundamentally obvious to some extent; thus, this aspect of research has often been neglected (Haldrup Citation2004; Urry and Larsen Citation2011).
According to how tourists move, tourist movement can be divided into two types: inter-destination movement, which refers to movement from an area that generates tourists to one or more destinations, and intra-destination movement, which refers to where tourists go and where they are within a destination. G. Lau and McKercher (Citation2006) first used geographic information system (GIS) spatial analysis methods to study tourist movement within destinations. Subsequent studies have been carried out by researchers at different scales, including cities and sites.
Research on cities as destinations mostly concern tourism recommendation systems. These studies analogize the process of selecting the next attraction tourists visit to the process of consumers selecting goods from a recommendation system perspective, commonly using machine learning methods such as the Bayesian learning model (Subramaniyaswamy et al. Citation2015), support vector machine (X. Sun et al. Citation2019), and latent Dirichlet allocation (Shafqat and Byun Citation2019). With the further developments of machine learning techniques, more research has been recently conducted using deep learning prediction methods (Ameen et al. Citation2020; Crivellari and Beinat Citation2020). However, the general idea remains similar to that of machine learning and does not incorporate the specificity of tourists into movement for prediction.
Studies on tourist sites as tourist destinations began in 2009 (Xia, Zeephongsekul, and Arrowsmith Citation2009). In addition, Xia, Zeephongsekul, and Packer (Citation2011) proposed an improved method that incorporated the time-dimension-based semi-Markov process two years later. These types of Markov models have failed to build a long-term dependency on tourist movements. Thus, W. Zheng, Huang, and Li (Citation2017) developed a heuristic prediction algorithm (HPA) that considers the effects of historical locations on the prediction. However, the HPA still ignores tourist personal information and factors affecting tourist movement while mining trajectories.
2.2. Factors that influence tourist movements
Tourist movement prediction relies on the regularity of tourist movement. To obtain a deeper understanding of tourist movement for more accurate predictions, the influence of various factors must be analyzed. G. Lau and McKercher (Citation2006) assumed that tourist movements can be affected by factors from three major aspects: human 'push', physical 'pull' and time factors. In addition, because the movement of tourists involves changes that occur simultaneously in time and space, we extend the temporal influences to spatiotemporal influences by combining changes in the spatial location. Therefore, our study divides the factors affecting the prediction of tourist movement predictability into three categories: tourist, destination, and space-time, as shown in .
Figure 1. Factors influencing tourist movement predictability (G. Lau and McKercher Citation2006).

From the perspective of the tourist, the factors primarily refer to individual differences. Because novelty and unfamiliarity are crucial for tourists and as these two attributes of the tourist experience vary individually, tourists' individual differences are a main reason for the differences in individual movement. First-time and repeat visitors are a major topic. Oppermann (Citation1997) noted that first-time visitors tend to visit more locations than repeat visitors. The studies by Lehto, O'leary, and Morrison (Citation2004) and Xia et al. (Citation2008) and subsequent studies also confirmed this (Lehto, O'leary, and Morrison Citation2004; Smallwood, Beckley, and Moore Citation2012; Xia et al. Citation2008). In addition, the distance was determined to be a critical factor related to tourists' movement. Wang, Little, and DelHomme-Little (Citation2012) determined that tourist stay longer if they live far from the destination. Xue and Zhang (Citation2020) determined that tourists who live farther from a destination prefer the city's cultural heritage and famous sites. Other factors, such as gender (Xia et al. Citation2010), age (Driver Citation1974), travel party (X. Zhao et al. Citation2018), and cultural distance (Dejbakhsh, Arrowsmith, and Jackson Citation2011; Flognfeldt Jr. Citation1999) also affect the movement of tourists.
The factors from the perspective of the destination involve the attractiveness of the destination to tourists (Shen et al. Citation2023; Zhong, Sun, and Law Citation2019). The characteristics of the destination have a ‘pull’ effect on tourists, which influences their decisions on the choice of itineraries within the destination and leads to different movement patterns. And the uniqueness, variety, number, and distribution of attractions affect tourist movement (G. Lau and McKercher Citation2006). Transportation between destinations has also been proven to be closely connected to the areas visited by tourists (Le-Klähn et al. Citation2015). The emergence of shared bikes, which are a novel type of public transportation, affects the movement of tourists in tourist destinations (Y. Yang, Jiang, and Zhang Citation2021). In addition, the configuration of services and facilities in a tourist destination can also influence on the movement, such as the hotel location (W. Zheng et al. Citation2020) and guide centers.
The spatiotemporal factors are divided into space and time factors. The factors of space primarily refer to tourist movement. Tourist movement in space leads to changes in tourists' locations. By connecting the location points with timestamps in sequential order, we can obtain the spatiotemporal trajectory of the tourist. Thereafter, by analyzing the trajectories of tourists, we can extract spatiotemporal features from the trajectories. These spatiotemporal features show the characteristics of the tourist's movement in space, and each movement is inseparable from time. Xu et al. (Citation2022) examined the connection between the trajectory length and movement predictability. Tourists act as as if they maximized the expected utility of the remainder of the trip (Västberg et al. Citation2020). The time has a decisive impact. G. Lau and McKercher (Citation2006) noted that the time scheduling and length of stay are the two main factors that influence tourist movement. Typically, longer stays result in a greater travel area (Koo, Wu, and Dwyer Citation2012). Le-Klähn et al. (Citation2015) drew similar conclusions in a study on Munich. Lew and McKercher (Citation2006) suggested that when a tourist travels within an urban area, the origin point of the tourist's morning trip and destination point of the late afternoon trip may both be the tourist's residential area. On a larger time scale, holiday and seasonal factors are also important influencing factors. For seasonal factors, Jankowski et al. (Citation2010) determined a lack of clear seasonal dependency in the frequency and spatial direction of tourists' movements. By contrast, Yun, Kang, and Lee (Citation2018) determined meaningful differences in the spatiotemporal distribution of urban walking tourists by season using GPS data.
3. Methodologies
3.1. Study area and dataset
We used social media data collected from Suzhou, China. Suzhou is a city with five million residents in eastern China, west of Shanghai (). Suzhou, a city with plentiful tourism resources, received over 100 million domestic visitors a year before the COVID-19 pandemic. Suzhou is famous for its cultural and historical heritage. The most represented sites in Suzhou are the classical gardens, which were included on the World Heritage List in the last century. Ancient city sites in Suzhou cover an area of 14 square km. In addition to these historical sites, Suzhou embraces natural landscapes with lush mountains and gleaming lakes.
Figure 2. Study area and Sina Weibo dataset. (a) The location of Suzhou on the scale of Country. (b) The location of Suzhou on the scale of Province. (c) The location of sites in Suzhou and (d) The geo-tagged microblogs in Suzhou.

Social media data were collected mainly from location-based social network mobile phone applications. Sina Weibo is the most popular social media platform in China, with 340 million active monthly users (X. Liu and Hu Citation2019). The microblogs with location information posted by Sina Weibo users are typically classified into two types: check-in microblogs, which contain check-in information and geographical information, and geo-tagged microblogs, which contain only the location from which users post the microblogs.
In our study, we first selected all points of interests (POIs) related to tourism sites. Then, we used the application program interface provided by Sina Weibo to collect the selected POI check-in microblogs. Following this, we proceeded to collect their historical microblogs from recent years. Upon acquiring users' historical microblogs, we filtered those that were not within the area of Suzhou. As shown in (d), there are 470,041 users and 5,399,161 microblogs.
3.2. Tourist identification and trajectory extraction
For social media data (such as Twitter, Flickr, and Sina Weibo), only a portion of the users were actually involved with tourism activities. The purpose of tourism activity could be entertainment or relaxing visits. However, it could also be official or business visits. Both are eligible for tourism activity, although visitors traveling at their own willingness were considered only as tourists in our study. In addition, we stipulated that tourists should not be locals, and tourists must return to the residential city after the trip to the destination city. Therefore, we defined only those users who post microblogs within the sites as tourists while data preprocessing.
To collect only those users that fit our definition of a tourist, we first involves determining the location of the user's residential city. We investigated only overnight visitors instead of one-day visitors. We can then separate the user's travel segments. A residential city is an important node in a user's entire travel trajectory. We use the residential city as a split sign to divide a user's annual travel trajectory into several relatively short trips in which the start and end nodes include both residential cities. However, not all trips pay a visit to a tourist site. Thus, we must distinguish whether the user has visited tourist sites during this trip. A clustering method was used to obtain the boundaries of each site. If a user's microblog location was within the boundary of any tourist site, we assumed that the user was a tourist on this trip.
(1) Determine the user's residential city A residential city represents the people's usual environment. Only some Sina Weibo users chose the right city when registering. However, some users' city information were inaccurate. We applied an easy method for determining a users' residential city based on the information entropy introduced by Claude Shannon (Shannon Citation1948).The city with the maximum entropy value can be regard as the city of residence for each user.
For a certain user U, user-visited cities can be represented as a collection {
}. For any city
in collection C, the number of microblogs posted in city
is
. We get the number
of the microblogs that the user posts each month, and calculate the entropy according to the following formula:
(1)
(1) The entropy value
of the city
is calculated, and the city corresponding to the maximum entropy value is selected as the resident city of the user. According to the principle of information entropy, the more balanced the data distribution is, the higher the entropy value is; the longer a user stays in his/her usual city, the more evenly the number of microblogs are distributed throughout the year, which corresponds to the highest information entropy value. And if the user posts too few microblogs, there will be no valid results.
(2) Split trajectories by trips The user's movement trajectory over the course of a year can be considerably long. According to the definition of tourism from the UNWTO, a tourist must leave their usual environment to perform tourism activities. Therefore, we used the residential city as a split sign to break a long trajectory into short-term trips. In particular, we regulated a user's trip according to the following rules.
(a) Residential Rule
The residential location is the origin of a user's travel history. In our study, we assume that all users begin and end their trip at the residential city. The breakpoint of the entire trajectory is a residential city.
(b) Time Gap Rule
This rule aims to ensure that users' microblogs are sparse. When a user's microblog temporal distributions are not uniform, we cannot use the residential city as the only breakpoint. The residential city is assumed to be the city where the user returns when the trip ends. If the user's two successive microblogs are both located in the destination city and the time between the two microblogs is more than three days, we identify these two microblogs belong to different trips. The reason we set the time threshold as three days is based on the general railway time, as most railway trips between two cities cost less than three days.
To clearly show how to divide the trajectory, we used the sequence shown in . According to this sequence, the user has microblogs in the user's residential city on days 1, 9, and 12. In addition, no microblogs were posted on days 3, 7, 15, 17, 18, or 19. The remaining days observed microblogs posted from the destination cities. Applying the two rules above in this sequence, we can obtain three trips:
(1) | Start on day 2 and end on day 8. The user is in the residential city on days 1 and 9. Although the user posted no microblogs on days 3 or 7, with the time-gap rule, we assume that this is still the same trip that began on day 2. | ||||
(2) | Start on day 10 and end on day 11. The user returns to the residential city on day 12 and posts microblogs every day during the entire trip. | ||||
(3) | Start on day 13 and end on day 16. The user posts a microblog on day 20; however, the time gap between the last day with microblogs (day 16) was over three days ago. Thus, we assume that this is not the same trip that began on day 13 according to the time gap rule. |
Figure 3. Diagram of trajectory division.
Note: The number in the circles is the date index. The circles indexed as 1,9,12 are the dates when the microblogs posted in the tourist's residence city. The circles indexed as 3,7,15,17,18,19 are the dates when microblogs were not posted. The other circles are the dates when microblogs were posted in the destination city.

These steps finally led to a total 282,532 tourists who visited one or more of Suzhou's tourist sites, and the total number of tourism-related activities (posts) was 1,611,269. These tourists contributed 568,465 trips during the time period we collected.
3.3. Location prediction models
Previous studies predicted people's next move by recognizing the regularity of historical movement. The Markov model has been frequently used to model the human movement and predict the next movement (Asahara et al. Citation2011; Ashbrook and Starner Citation2002; Gambs, Killijian, and del Prado Cortez Citation2012; L. Song et al. Citation2004; J. Yang et al. Citation2014). The Markov model uses probability to model the location transition process of users. The Markov process assumes that every subsequent location depends on the current location. Thus, based on the user's past transition probability between locations, the next location can be inferred by a probability calculation. However, the Markov model can establish only connections between adjacent locations, while ignoring the long-term dependence of locations.
Recently, deep learning has become a popular topic in almost all study areas. A recurrent neural network (RNN), which is a popular neural network, was first designed to process long sentences in text and speech tasks. The tourist trajectories are similar to text sentence because they are both inputted as a sequence. Long short-term memory (LSTM), a variant network architecture derived from the basic RNN, has been used in location prediction studies (Bao et al. Citation2021; Kong and Wu Citation2018; X. Song, Kanasugi, and Shibasaki Citation2016; K. Sun et al. Citation2020). Basic RNNs often trigger gradient disappearance or explosion owing to their simple structure, and the remembered time-step information is considerably limited. A gate structure was introduced in LSTM units, and this structure is effective in improving the gradient problem and selectively remembering or forgetting historical time-step information; thus, it is called a long short-term memory network.
As shown in , the LSTM model we used consists of an embedding, LSTM, fully connected, and SoftMax layer. The model used in this study is based on LSTM, where we could add the tourist's personalized features to the network. When dealing with a trajectory in the dataset, the first step involves uniformly aligning its length. Then, the locations of each site within the trajectory are sequentially fed into the model and transformed into low-dimensional embedding vectors. To accomplish this, we use the Word2Vec to embed the sites. The Word2Vec is one of the language models that learns semantic knowledge in an unsupervised approach from a large number of textual corpus and is widely used in natural language processing (Le and Mikolov Citation2014; Mikolov et al. Citation2013; Rong Citation2014). In our model, we treat location names as words and use Word2Vec to train a vector representation for them. Subsequently, the embedding vector is concatenated with the personalized features (which is optional) before being inputted into the LSTM layer. Following each time step's output, it is then processed sequentially through the Fully connected layer and Softmax layer. Softmax turns the output of a neural network into a probability distribution of locations. The model utilizes the location with the highest probability as its prediction output.
During the training process, the prediction results of each time step are involved in the calculation of the loss, whereas in the testing process, only the prediction results of the last time step are used. This is because, in the training phase, maximum autoregression can be performed with the data. By contrast, in the testing phase, when a user's historical trip has been determined, we focus only on the prediction results of the current location. The loss of the training and testing stage is illustrated in . It can be seen that our model is not overfitted.
To consider the effect of different models on tourist predictability, this study designs comparative experiments for the Markov and LSTM models to analyze the effect of the length of the tourist trajectory and distance traveled by the tourist within the destination based on the predictability.
3.4. Influential factors of movement prediction
Using techniques in representation learning, deep learning can learn and extract rich features from a wide variety of unstructured datasets such as of text, images, and sounds. In the study of location prediction problems, previous researchers typically considered features other than the trajectory features in the dataset as input for the model, such as geographical (Feng et al. Citation2017; S. Zhao, King, and Lyu Citation2013), temporal (Gao et al. Citation2013; Q. Liu et al. Citation2016; Yuan et al. Citation2013), and demographic (Solomon et al. Citation2021) features. By adding these features, the accuracy of the model prediction was further improved.
In this study, we used the factors influencing tourist movement predictability mentioned in Section 2.2 to analyze the impact of these factors on the predictability of models commonly used for location prediction. Using the dataset composed of social media content, we calculated and extracted different features from three perspectives: tourist, destination, and space-time. From the tourist perspective, we extracted the tourist's travel distance between the origin and destination (OD), number of visits, and gender. From the destination perspective, we tagged all 108 tourist sites using three types of labels. We introduce these labels in Section 4.2. For the spatiotemporal perspective, we first calculate the length of tourists' trajectories in Suzhou and travel distance between sites (S) as features of spatial movement, and then extract the temporal features of tourists using three scales: season, holiday, and time of arrival.
For the LSTM model, trajectory-related information can be input for the model to self-learn and mine potential movement patterns of tourists. Therefore, this study inputs tourist, destination, and spatiotemporal features into the LSTM model and analyzes the impact of the features on predictability. To make our results convincing, we ran the experiment 10 times for each factor. We split the dataset into 10 sets. For each run, we picked a different set for testing and used the remaining nine sets to train the model.
3.5. Evaluation metrics
The two metrics used for prediction accuracy include Top@k and the mean reciprocal rank (MRR):
Top@k – Top@k is a common evaluation metric in prediction and recommendation studies. In general, the output of a prediction model is a list of values ranked in the order of the predicted score. Thus, Top@k indicates that a prediction is considered correct as long as the true label is associated with one of the k highest predicted scores. Top@k is calculated as follows:
(2)
(2)
represents the number of test data.
is the predicted value for the
sample of test data corresponding to the
largest predicted score and
is the corresponding true value.
indicates the indicator function, which means that when the input is True, the output is 1, otherwise the output is 0. In our study, we used Top@1 and Top@3 as evaluation metrics.
Mean Reciprocal Rank (MRR) – This indicator considers the ranking of the true values in the prediction results, using the inverse of the ranking as a weighted weight and harmonic mean of the prediction probabilities. MRR is calculated as follows:
(3)
(3) where
represents the number of the test data, and
represents the rank of the true value in the prediction result for the
test data point; the larger the value of MRR, the higher is the ranking of the true value, and the better is the prediction model.
4. Results
4.1. Predictability based on tourist factors
In this subsection, we focus on the impact of predictability by analyzing the tourist influential factors (as mentioned in Section 3.4) using the LSTM model. Using the methods mentioned in Section 3.2, we identified a tourist's city of residence. The number of tourist visits to Suzhou can also be determined by the tourist trajectories. The tourists' gender information can be acquired by their Sina Weibo profiles. We acquired three features of tourists: travel distance (OD), number of visits, and gender.
The results imply that the model has different predictabilities for tourists with different features. As shown in , this study further analyzed the prediction accuracy of the LSTM model for the different features of the tourists. To ensure whether the results had significant differences, each tourist feature was used 10 times. Different training and test datasets were used each time.
Figure 6. Boxplots of Top@1, Top@3, and the MRR for the prediction results of the LSTM model with tourist features.

Travel distance (OD) – The prediction accuracy falls with the range of the travel distance (OD). The distance from 0 to 100 km includes several large cities near Suzhou with strong economic ties, such as Shanghai, Wuxi, and Huzhou. The distance from 100 to 500 km covers the entire Jiangsu province and some areas in neighboring provinces. Tourists with a distance of less than 500 km are highly predictable, as shown in (a). The distances from 500 to 1200 km cover most of the eastern coast of China, including megacities such as Beijing and Guangzhou. Tourists from these areas are less predictable. In addition, tourists from remote areas with distances over 1200 km were difficult to predict.
Number of visits – When a tourist arrives to Suzhou for the first time, their predictability is steadily lower. However, once a tourist becomes a familiar visitor to Suzhou, their predictability significantly increases, up to twice that of even a first-time visitor ((b)).
Gender – Gender is an important demographic characteristic. A difference in preference was observed between genders in choosing tourist sites. Based on the results using our dataset, as shown in the (c), the predictability of females is slightly higher than that of males.
4.2. Predictability based on destination factors
Similar to the tourist features in the previous subsection, we embedded the destination features into the model in the same manner. In this subsection, we consider the effects of destination factors on the predictability. Tourist sites are a core component of destinations. Therefore, based on all the sites visited by tourists in our dataset, we categorized the sites from three perspectives: site location (located in urban or rural areas), site type (natural, cultural, commercial), and site title (5A, 4A, UNESCO). It's worth stating that there are five levels of sites in China: 1A, 2A, 3A, 4A and 5A. A tourist site with a 5A score implies that it has the most beautiful scenery, the best service and perfect facilities. Then the same experiments as in the previous subsection were conducted, and shows the results.
Figure 7. Boxplots of Top@1, Top@3, and the MRR for the prediction results of the LSTM model with destination features.

Site title – Site title reflects the tourist site's reputation in the domestic and international tourism market. More well-known tourist sites have a broader tourist market and attract a wider range of tourists. From (a), we note that different site titles have similar predictability. Sites with the 4A title tend to have a higher predictability.
Site type – The site type determines the main scene of the sites. Natural sites often refer to those sites with beautiful mountain scenery. Cultural sites refer to temples, gardens, and museums that have humanistic and historical features. Commercial sites refer to the commercial complex that evolved from the historical old street, featuring shopping, leisure, and dining functions. From (b), we note that the commercial sites of Suzhou have a high predictability, for which the Top@1 is over 0.5. Natural sites were the second-most predictable. However, cultural sites, which are the main site type of Suzhou, have the lowest predictability.
Site location – The circular highway around the urban area of Suzhou is called the Zhonghuan (Central Ring) Road. We used this highway as a dividing line, dividing Suzhou into urban and rural areas. The urban area covers 5% of Suzhou and contains 40% of the tourist sites. (c) shows that the predictability of urban areas is significantly higher than that of the rural areas.
4.3. Predictability based on spatiotemporal factors
From the experiments in the previous subsection, we know that predictability varies with the tourists and destinations. Thus, in this section, we focus on more dynamic factors, including spatial and temporal factors. From the microblogs posted by tourists, we extracted three temporal features to the best of our knowledge: time of arrival, holiday, and season. We believe that the model has different predictability for tourists with respect to time factors. Therefore, the same analysis as in the previous subsections was performed, as shown in .
Figure 8. Boxplots of Top@1, Top@3, and the MRR for the prediction results of the LSTM model with time features.

From , we determined the following:
Time of arrival – Arrival time can reflect the type of tourist site to an extent; for example, tourists are mostly active in the daytime at sites with natural scenery. In (a), tourists' behavior during the day is less predictable, whereas behavior at night is relatively highly predictable. The reduced prediction range may reduce the difficulty of prediction owing to the few sites in Suzhou that are suitable for nighttime visitations.
Holiday/Workday – Generally holidays typically attract more tourists. However, from the dataset we used, this trend has an almost negligible impact on predictability. Alternatively, the predictability of weekdays and holidays does not differ for Suzhou. That is, for tourist sites in Suzhou, the movements of tourists during weekdays and holidays do not differ significantly.
Season – Several previous datasets lacked long-term datasets, making the study impossible from a seasonal perspective. As shown by the results of the experiment on the dataset ((c)), some variation is observed across seasons. The predictability is relatively low in the spring and autumn. The predictability of summer is slightly higher than these two seasons, and the most predictable season is winter.
Apart from three time factors, we extracted the spatial movement characteristics of tourists by analyzing tourists' trajectory data and the influence of tourists' movement. Moreover, the characteristics of spatial movement are a more continuous amount of change compared with the previous characteristics of tourists and destinations. Therefore, we used the Markov and LSTM models as controls, representing the traditional prediction and deep learning models, respectively. We used the number of tourist trajectory nodes and length of tourist trajectories to examine how the predictability of the two models changed with these two aspects. Here, the number of nodes in the trajectory is the trajectory length. shows the distribution of the trajectory nodes. From the figure, 90% of tourists visit no more than six sites on one Suzhou trip.
Similar to the length of the trajectory nodes, the travel distance (S) mentioned in this study is the sum of the distances between sites corresponding to the remaining trajectory nodes, excluding the last node of the entire trajectory (the last node is the predicted object). Because the distribution of tourists' travel distance (S) is closer to the long-tail distribution, we take the logarithm of the distance to better show the distribution of each distance interval, as shown in . From the figure, 90% of tourists visit no more than 80 km on one Suzhou trip.
Based on the trajectory length and tourist travel distance (S), we explored the relationship between them and the predictability. First, for the tourist trajectory length, as shown in , the prediction accuracy of the LSTM is obviously higher than that of the Markov model. Both models have lower prediction accuracy at 11 nodes, as shown in the distribution chart (), probably because of the small sample size of the data.
As the trajectory length increases, the prediction accuracy of the LSTM gradually increases (except the outlier at 11 nodes). The main improvement in the prediction accuracy is reflected by the Top@3 and MRR metrics, whereas the improvement in the Top@1 metric is insignificant. This may indicate that the input trajectory length is not a decisive factor that directly affects the prediction accuracy. The prediction accuracy of the Markov model falls sharply with three nodes and then repeatedly oscillates with the increase in the trajectory length without any significant increase. From this result, we can easily see that the model can more clearly learn the movement patterns of tourists from longer trajectories and thereby improve the prediction accuracy. The Markov model is still a good prediction model when the tourist trajectory is short, which is why many inter-site-scale tourist prediction models use Markov models and their variants (Xia, Zeephongsekul, and Arrowsmith Citation2009; Xia, Zeephongsekul, and Packer Citation2011).
For the experiment on the variation in the tourist travel distance (S), we conducted a similar experiment, and shows the results. As the tourist travel distances (S) appears to follow a long-tailed distribution, the x-axis in the figure is taken logarithmically for distances, and the prediction accuracy is calculated separately based on equal intervals, which are taken on a logarithmic scale.
As shown in the figure, the prediction accuracy of the two models is close when the travel distance (S) of tourists is less than 1 km. However, when the distance traveled by tourists exceeds 1 km, the prediction accuracy of the Markov model gradually decreases or even converges to 0. In contrast, LSTM is not affected by the increase in distance, and the MRR is always above 0.4 and has a relatively high prediction accuracy when less than 1 km. Such results may suggest that LSTM has the potential to better establish connections between sites with longer trajectories than the Markov model and that LSTM may uncover implicit connections between sites with longer geographical distances. This also demonstrates that black-box methods, such as LSTM are more capable of uncovering more potential interconnections of tourist movements than white-box methods based on human cognitive perspectives.
In addition to the overall movement of tourists within a destination, we focused on the local movement of tourists between sites. To this end, we calculated the distance and MRR between the predicted site and its previous sites. shows the distributions of the logarithmic distance and MRR. The vertical line at each point represents the 80% confidence interval of the MRR distribution at that point.
The distribution of the confidence intervals shows that for equal logarithmic intervals, the predictability first rises and reaches an extreme value (0.35) at a distance of approximately to
(≈1–1.25) km. Subsequently, the predictability gradually decreases and approaches 0. From these results, we infer that the predictability of tourists is not monotonic with distance. That is, the range of tourist activity is closely related to the predictability of the tourists. When the activity range of tourists is small, the predictability is not too low because the number of sites within a small spatial area is also small; however, this does not imply that closers distances between two tourist sites improve the predictability of the tourists. When the range of tourists' activities gradually increases to approximately 1–1.25 km, the predictability of tourists is at its highest, and obtaining more accurate results is easier when recommending sites to tourists. When the activity range is further increased, tourists' choices are further expanded; thus, the predictability is further reduced to 0. Our analysis leads to a possible reason for this threshold of optimal predictability, which is the point at which the most of the tourist transportation change significantly. Once this spatial range is exceeded, tourists switch from walking to transit or driving.
5. Discussion
5.1. Predictability of models
Through several experiments, we determined a difference between models in predicting a certain prediction accuracy. The advantages and disadvantages of different models are evident. For the Markov model, the model is a white-box model with straightforward assumptions. The Markov model has achieved good prediction results on a relatively small scale. However, when the model is confronted with longer and more complex trajectories, its prediction ability decreases substantially, as shown by the results in the previous section.
On the contrast, the LSTM model has a particularly good prediction accuracy, even for long trajectory inputs. In addition to learning the hidden tourist movement regularity in tourist trajectories, LSTM can consider the influence of other factors on tourist movement predictability. By adding features corresponding to the influencing factors, we determined that the features can further improve the prediction accuracy.
The length of a tourist's trajectory is not equal to the travel distance (S) of the tourist at the destination. The results indicate that the accuracy of the two models is similar for tourists with short travel distances (S). This suggests that employing complex prediction models like the deep learning model may not be advantageous when the prediction's spatial scale is limited. Furthermore, tourists constrained by limited movement often opt for short stays and are commonly referred to as short-trip tourists. As a probability-driven model, the Markov model tends to outputting tourist sites with higher probabilities, signifying greater visitation and popularity. Therefore, the Markov model's effectiveness with short-trip tourists suggests that its predictions align with the concept of experienced individuals providing recommendations to short-trip tourists, highlighting the most popular sites.
The practical significance of these findings is to provide tourism management with a basis for example-based prediction model selection. Indeed, various models are now available for tourist prediction. For decision-makers in tourism management, choosing an appropriate model and method for the situation of the destination they manage is crucial. Because the datasets, study areas, and study scales used by different researchers differ, decision making and understanding is easier from the perspective of combining the theoretical and practical models proposed by previous tourism researchers in this study to choose more suitable and smarter solutions for destination management organization.
5.2. Predictability of tourists and destination factors
The results of this experiment show that not all features improve the prediction results for a real-life dataset. Moreover, the contribution of a single feature to a deep learning prediction model is limited, and researchers cannot rely on certain features to improve the prediction accuracy of a model. For factors that improve the prediction results after inputting features into models, the main improvement is reflected by the Top@1.
The factors from the tourist perspective show the effects of individual differences on predictability. In this study, we used three factors: travel distance (OD), number of visits, and gender. The predictability decreases as the travel distance (OD) increases. According to Xue and Zhang (Citation2020), long-haul tourists prefer cultural sites, followed by natural and commercial sites (Xue and Zhang Citation2020). Even when considering cultural sites, the predictability remains low. Our study proves that long-haul tourists in Suzhou are more likely to choose cultural sites. The number of visits reflects the tourist's prior visit experience of Suzhou. As Oppermann (Citation1997) noted, the movement patterns of New Zealand repeat visitors visit fewer locations and are more concentrated in their itinerary. We obtained similar results on the Suzhou dataset, as shown in (b). For the gender factor, we determined that females had a higher predictability. Gender features showed the highest improvement in terms of prediction accuracy for the LSTM model. By comparing the differences in the spatiotemporal movements and site choices between the two genders, we determined that a higher proportion of females visited commercial attractions (36%) among the three main attraction types (natural, cultural, and commercial), slightly higher than that of males (33%). Kotzé et al. (Citation2012) noted that women obtained more gratification from shopping than men. As commercial sites are more predictable, this may contribute to female tourists being more predictable than male tourists.
To further discuss why some tourists have a high predictability, we further analyzed tourist preferences for choosing sites, as shown in . As shown in the figure, tourists' preference for the site type affects the predictability of tourists. When the travel distance is 0–100 km, the tourist prefers to visit commercial sites. Tourists prefer cultural sites when the travel distance is greater than 1200 km. Note that these two ranges of travel distances correspond to the highest and lowest predictability, respectively, according to the results in . For tourist factors such as the number of visits and gender, tourists with a higher predictability (repeat-visit and female tourists) similarly prefer commercial sites ((b,c)). Commercial sites constitute a small fraction of Suzhou's overall sites, with well-known examples including Guanqian Street, Pingjiang Road, and Jinji Lake (). These sites offer a diverse range of tourist services, including accommodation, dining, shopping, and recreational activities. Consequently, accurately predicting tourist visits to these commercial sites within Suzhou's site type distribution is not a challenging task. According to , visits to commercial sites are mainly revisited and short-haul tourists in Suzhou. These tourists' primary motivation revolves around leisure and relaxation, rather than sightseeing. These findings support those of previous studies (McKercher and Du Cros Citation2003; Xue and Zhang Citation2020).
5.3. Predictability of spatiotemporal factors
Time is important for tourists. As with most tourist budgets, this is a limited resource. Therefore, time significantly limits tourists' movement to a large extent.
The time of arrival reflects the main method of visiting sites. For example, tourists are unlikely to choose to visit magnificent natural sites at night because the visibility is too low. (a) shows that the highest predictability is in the evening. Tourists are difficult to predict during the day. The sparsity of the dataset at midnight caused the predictability to be unstable. These results imply that tourists in Suzhou are clustered more at night.
Holiday refers to various activities that tourists can participate. Some studies used holiday data to ensure that most users in the dataset were tourists (Y. Yang, Li, and Li Citation2019). However, this approach can lead to biased data that hardly demonstrates the tourists' movements during weekdays. We demonstrated that the predictability of holidays and workdays was insignificantly different.
Seasons are another important factor in tourist movements. Previous studies have used data over a short time span, which does not provide a good representation of the seasonal changes in tourists' movements. Suzhou has a subtropical monsoon climate with hot summers, abundant rainfall, and cold winters with less rainfall. Popular types of tourist sites in Suzhou are primarily gardens and ancient towns. Thus, the best seasons for visiting Suzhou are spring and autumn. As shown in (c), this increases the difficulty of predicting tourist movement. The winter has a relatively higher predictability, probably because of the fewer winter attractions in Suzhou.
The spatiotemporal factors extracted from tourist trajectories provide a new interpretation of tourist prediction. While recent deep learning methods have continued to improve the prediction accuracy on datasets, few models have attempted to improve the interpretability of the models. We attempt to correlate the features with the model predictability by analyzing the spatiotemporal features of tourists, as described in the results in Section 4.3. The findings hold practical implications for the customization of tourism products and the planning of tourist sites. Regarding overall movement, long itinerary tourists pose significant challenges in terms of prediction accuracy. This indicates that current models face difficulties in meeting the specific requirements of long itinerary tourists. Consequently, it is crucial to prioritize personalization and customization of tourism products tailored to the needs of long itinerary tourists. In terms of local movement, the extreme point of predictability shown in indicates that this is an important threshold (1–1.25 km) for tourists making their decisions to visit subsequent sites. Therefore, neighboring sites within 1 km are more suitable for bundle marketing. In addition, relatively cold sites should consider using the heat of popular sites within this range for site promotion. Furthermore managers can situate their tourism-related services and facilities within this spatial range to offer better tourism services.
6. Conclusion
This study classifies and summarizes the factors influencing tourist movement predictability in the past and proposes a conceptual framework of influencing factors based on the theory of G. Lau and McKercher (Citation2006), as shown in . This study used social media data to explore tourist movement predictability from a multi-subject and multi-factor perspective. By comparing the models, features, and tourist sites, we obtained comprehensive and detailed analysis results of the tourist predictability, as shown in . By applying prediction models and evaluating their predictability, we identified some consistencies with the findings of previous studies. We determined significant differences in movement predictability for almost every factor and discussed possible reasons. We then proposed constructive suggestions for Suzhou's tourism management and decision-makers, considering the actual tourism situation in Suzhou.
Table 1. Summary of the impact of factors on tourist movement prediction.
This study has a few limitations. The dataset used in this study was Sina Weibo, in which data bias may be difficult to avoid. This is because the user base of Sina Weibo is dominated by a younger user group. The results are not representative of the mobile-mode behavior of children and the elderly. Future studies should consider combining social media data with questionnaire data to improve the reliability of the data source.
In conclusion, we used social media data to analyze the factors that affect tourist movement prediction. We offer evidence to support the knowledge claimed by previous studies and provide a new theoretical basis for constructing tourist movement prediction models. In addition, we determined that the predictability of the LSTM model varies with different tourists, times, and sites. Therefore, future models that aim to further improve the prediction accuracy must consider the contributions of different features in the current case. New prediction models have increasingly improved in recent years; however, few of these models have been combined with existing knowledge obtained from previous studies. This study explored the impact of features generated by influential factors on tourist prediction models and attempted to explain this impact. Future prediction models can incorporate travel costs between locations, thereby achieving models characterized by enhanced accuracy and interpretability. Additionally, the order of the visited locations should also be considered.
Disclosure statement
No potential conflict of interest was reported by the author(s).
Additional information
Funding
References
- Ameen, Thaair, Ling Chen, Zhenxing Xu, Dandan Lyu, and Hongyu Shi. 2020. “A Convolutional Neural Network and Matrix Factorization-based Travel Location Recommendation Method Using Community-contributed Geotagged Photos.” ISPRS International Journal of Geo-Information 9 (8): 464. https://doi.org/10.3390/ijgi9080464.
- Asahara, Akinori, Kishiko Maruyama, Akiko Sato, and Kouichi Seto. 2011. “Pedestrian-Movement Prediction Based on Mixed Markov-Chain Model.” In Proceedings of the 19th ACM SIGSPATIAL International Conference on Advances in Geographic Information Systems, 25–33.
- Ashbrook, Daniel, and Thad Starner. 2002. “Learning Significant Locations and Predicting User Movement with GPS.” In Proceedings. Sixth International Symposium on Wearable Computers, 101–108. IEEE.
- Bao, Yi, Zhou Huang, Linna Li, Yaoli Wang, and Yu Liu. 2021. “A BiLSTM-CNN Model for Predicting Users' Next Locations Based on Geotagged Social Media.” International Journal of Geographical Information Science 35 (4): 639–660. https://doi.org/10.1080/13658816.2020.1808896.
- Cantis, Stefano De, Mauro Ferrante, Alon Kahani, and Noam Shoval. 2016. “Cruise Passengers' Behavior At the Destination: Investigation Using GPS Technology.” Tourism Management 52:133–150. https://doi.org/10.1016/j.tourman.2015.06.018.
- Crivellari, Alessandro, and Euro Beinat. 2020. “LSTM-based Deep Learning Model for Predicting Individual Mobility Traces of Short-term Foreign Tourists.” Sustainability 12 (1): 349. https://doi.org/10.3390/su12010349.
- Dejbakhsh, Sabereh, Colin Arrowsmith, and Merv Jackson. 2011. “Cultural Influence on Spatial Behaviour.” Tourism Geographies 13 (1): 91–111. https://doi.org/10.1080/14616688.2010.516396.
- Driver, B. 1974. “Toward a behavioral interpretation of recreational engagements, with implications for planning.” Elements in outdoor recreation planning.
- East, Duncan, Patrick Osborne, Simon Kemp, and Tim Woodfine. 2017. “Combining GPS & Survey Data Improves Understanding of Visitor Behaviour.” Tourism Management 61:307–320. https://doi.org/10.1016/j.tourman.2017.02.021.
- Feng, Shanshan, Gao Cong, Bo An, and Yeow Meng Chee. 2017. “Poi2vec: Geographical Latent Representation for Predicting Future Visitors.” In Thirty-First AAAI Conference on Artificial Intelligence.
- Fennell, David A. 1996. “A Tourist Space-Time Budget in the Shetland Islands.” Annals of Tourism Research 23 (4): 811–829. https://doi.org/10.1016/0160-7383(96)00008-4.
- Flognfeldt Jr., Thor. 1999. “Traveler Geographic Origin and Market Segmentation: The Multi Trips Destination Case.” Journal of Travel & Tourism Marketing 8 (1): 111–124. https://doi.org/10.1300/J073v08n01_07.
- Gambs, Sébastien, Marc-Olivier Killijian, and Miguel Núñez del Prado Cortez. 2012. “Next Place Prediction Using Mobility Markov Chains.” In Proceedings of the First Workshop on Measurement, Privacy, and Mobility, 1–6.
- Gao, Huiji, Jiliang Tang, Xia Hu, and Huan Liu. 2013. “Exploring Temporal Effects for Location Recommendation on Location-Based Social Networks.” In Proceedings of the 7th ACM Conference on Recommender Systems, 93–100.
- Haldrup, Michael. 2004. “Laid-back Mobilities: Second-home Holidays in Time and Space.” Tourism Geographies 6 (4): 434–454. https://doi.org/10.1080/1461668042000280228.
- Jankowski, Piotr, Natalia Andrienko, Gennady Andrienko, and Slava Kisilevich. 2010. “Discovering Landmark Preferences and Movement Patterns From Photo Postings.” Transactions in GIS 14 (6): 833–852. https://doi.org/10.1111/tgis.2010.14.issue-6.
- Kong, Dejiang, and Fei Wu. 2018. “HST-LSTM: A Hierarchical Spatial-Temporal Long-Short Term Memory Network for Location Prediction.” In IJCAI, Vol. 18. 2341–2347.
- Koo, Tay T. R., Cheng-Lung Wu, and Larry Dwyer. 2012. “Dispersal of Visitors Within Destinations: Descriptive Measures and Underlying Drivers.” Tourism Management 33 (5): 1209–1219. https://doi.org/10.1016/j.tourman.2011.11.010.
- Kotzé, Theuns, Ernest North, Marilize Stols, and Lezanne Venter. 2012. “Gender Differences in Sources of Shopping Enjoyment.” International Journal of Consumer Studies 36 (4): 416–424. https://doi.org/10.1111/ijcs.2012.36.issue-4.
- Lau, W. G. 2007. Mapping tourist movement patterns: A GIS approach. Kowloon, Hong Kong: The Hong Kong Polytechnic University.
- Lau, Gigi, and Bob McKercher. 2006. “Understanding Tourist Movement Patterns in a Destination: A GIS Approach.” Tourism and Hospitality Research 7 (1): 39–49. https://doi.org/10.1057/palgrave.thr.6050027.
- Le-Klähn, Diem-Trinh, Jutta Roosen, Regine Gerike, and C. Michael Hall. 2015. “Factors Affecting Tourists' Public Transport Use and Areas Visited At Destinations.” Tourism Geographies 17 (5): 738–757. https://doi.org/10.1080/14616688.2015.1084527.
- Le, Quoc, and Tomas Mikolov. 2014. “Distributed Representations of Sentences and Documents.” In International Conference on Machine Learning, 1188–1196. PMLR.
- Lehto, Xinran Y., Joseph T. O'leary, and Alastair M. Morrison. 2004. “The Effect of Prior Experience on Vacation Behavior.” Annals of Tourism Research 31 (4): 801–818. https://doi.org/10.1016/j.annals.2004.02.006.
- Lew, Alan, and Bob McKercher. 2006. “Modeling Tourist Movements: A Local Destination Analysis.” Annals of Tourism Research 33 (2): 403–423. https://doi.org/10.1016/j.annals.2005.12.002.
- Li, Xiang Robert, Chia-Kuen Cheng, Hyounggon Kim, and James F. Petrick. 2008. “A Systematic Comparison of First-time and Repeat Visitors Via a Two-phase Online Survey.” Tourism Management29 (2): 278–293. https://doi.org/10.1016/j.tourman.2007.03.010.
- Liu, Xiaojun, and Wei Hu. 2019. “Attention and Sentiment of Chinese Public Toward Green Buildings Based on Sina Weibo.” Sustainable Cities and Society 44:550–558. https://doi.org/10.1016/j.scs.2018.10.047.
- Liu, Qiang, Shu Wu, Liang Wang, and Tieniu Tan. 2016. “Predicting the Next Location: A Recurrent Model with Spatial and Temporal Contexts.” In Thirtieth AAAI Conference on Artificial Intelligence.
- Majid, Abdul, Ling Chen, Gencai Chen, Hamid Turab Mirza, Ibrar Hussain, and John Woodward. 2013. “A Context-aware Personalized Travel Recommendation System Based on Geotagged Social Media Data Mining.” International Journal of Geographical Information Science 27 (4): 662–684. https://doi.org/10.1080/13658816.2012.696649.
- McKercher, Bob, and Hilary Du Cros. 2003. “Testing a Cultural Tourism Typology.” International Journal of Tourism Research 5 (1): 45–58. https://doi.org/10.1002/(ISSN)1522-1970.
- McKercher, Bob, Noam Shoval, Erica Ng, and Amit Birenboim. 2012. “First and Repeat Visitor Behaviour: GPS Tracking and GIS Analysis in Hong Kong.” Tourism Geographies 14 (1): 147–161. https://doi.org/10.1080/14616688.2011.598542.
- McKercher, Bob, Noam Shoval, Eerang Park, and Alon Kahani. 2015. “The [limited] Impact of Weather on Tourist Behavior in An Urban Destination.” Journal of Travel Research 54 (4): 442–455. https://doi.org/10.1177/0047287514522880.
- Mikolov, Tomas, Kai Chen, Greg Corrado, and Jeffrey Dean. 2013. “Efficient Estimation of Word Representations in Vector Space.” arXiv preprint arXiv:1301.3781.
- Oppermann, Martin. 1997. “First-time and Repeat Visitors to New Zealand.” Tourism Management 18 (3): 177–181. https://doi.org/10.1016/S0261-5177(96)00119-7.
- Raun, Janika, Rein Ahas, and Margus Tiru. 2016. “Measuring Tourism Destinations Using Mobile Tracking Data.” Tourism Management 57: 202–212. https://doi.org/10.1016/j.tourman.2016.06.006.
- Rong, Xin. 2014. “Word2vec Parameter Learning Explained.” arXiv preprint arXiv:1411.2738.
- Shafqat, Wafa, and Yung-Cheol Byun. 2019. “A Recommendation Mechanism for Under-emphasized Tourist Spots Using Topic Modeling and Sentiment Analysis.” Sustainability 12 (1): 320. https://doi.org/10.3390/su12010320.
- Shannon, Claude Elwood. 1948. “A Mathematical Theory of Communication.” Bell System Technical Journal 27 (3): 379–423. https://doi.org/10.1002/bltj.1948.27.issue-3.
- Shen, K., J. D. Schmöcker, W. Sun, and A. G. Qureshi. 2023. “Calibration of sightseeing tour choices considering multiple decision criteria with diminishing reward.” Transportation 50 (5): 1897–1921.
- Smallwood, Claire B., Lynnath E. Beckley, and Susan A. Moore. 2012. “An Analysis of Visitor Movement Patterns Using Travel Networks in a Large Marine Park, North-western Australia.” Tourism Management 33 (3): 517–528.
- Solomon, Adir, Amit Livne, Gilad Katz, Bracha Shapira, and Lior Rokach. 2021. “Analyzing Movement Predictability Using Human Attributes and Behavioral Patterns.” Computers, Environment and Urban Systems 87: 101596. https://doi.org/10.1016/j.compenvurbsys.2021.101596.
- Song, Xuan, Hiroshi Kanasugi, and Ryosuke Shibasaki. 2016. “Deeptransport: Prediction and Simulation of Human Mobility and Transportation Mode at a Citywide Level.” In Proceedings of the Twenty-Fifth International Joint Conference on Artificial Intelligence, 2618–2624.
- Song, Libo, David Kotz, Ravi Jain, and Xiaoning He. 2004. “Evaluating Location Predictors with Extensive Wi-Fi Mobility Data.” In IEEE Infocom 2004, Vol. 2, 1414–1424. IEEE.
- Su, Xing, Bas Spierings, Martin Dijst, and Ziqi Tong. 2020. “Analysing Trends in the Spatio-temporal Behaviour Patterns of Mainland Chinese Tourists and Residents in Hong Kong Based on Weibo Data.” Current Issues in Tourism 23 (12): 1542–1558. https://doi.org/10.1080/13683500.2019.1645096.
- Subramaniyaswamy, V., V. Vijayakumar, R. Logesh, and V. Indragandhi. 2015. “Intelligent Travel Recommendation System by Mining Attributes From Community Contributed Photos.” Procedia Computer Science 50: 447–455. https://doi.org/10.1016/j.procs.2015.04.014.
- Sun, Xiaoyu, Zhou Huang, Xia Peng, Yiran Chen, and Yu Liu. 2019. “Building a Model-based Personalised Recommendation Approach for Tourist Attractions From Geotagged Social Media Data.” International Journal of Digital Earth 12 (6): 661–678. https://doi.org/10.1080/17538947.2018.1471104.
- Sun, Ke, Tieyun Qian, Tong Chen, Yile Liang, Quoc Viet Hung Nguyen, and Hongzhi Yin. 2020. “Where to Go Next: Modeling Long-and Short-Term User Preferences for Point-of-Interest Recommendation.” In Proceedings of the AAAI Conference on Artificial Intelligence, Vol. 34, 214–221.
- Urry, John, and Jonas Larsen. 2011. The Tourist Gaze 3.0 (3rd ed.). London: Sage.
- Västberg, Oskar Blom, Anders Karlström, Daniel Jonsson, and Marcus Sundberg. 2020. “A Dynamic Discrete Choice Activity-based Travel Demand Model.” Transportation Science 54 (1): 21–41. https://doi.org/10.1287/trsc.2019.0898.
- Wang, Erda, Bertis B Little, and Beverly Ann DelHomme-Little. 2012. “Factors Contributing to Tourists' Length of Stay in Dalian Northeastern China—A Survival Model Analysis.” Tourism Management Perspectives 4:67–72. https://doi.org/10.1016/j.tmp.2012.03.005.
- Xia, Jianhong Cecilia, Colin Arrowsmith, Mervyn Jackson, and William Cartwright. 2008. “The Wayfinding Process Relationships Between Decision-making and Landmark Utility.” Tourism Management 29 (3): 445–457. https://doi.org/10.1016/j.tourman.2007.05.010.
- Xia, Jianhong Cecilia, Fiona H. Evans, Katrina Spilsbury, Vic Ciesielski, Colin Arrowsmith, and Graeme Wright. 2010. “Market Segments Based on the Dominant Movement Patterns of Tourists.” Tourism Management 31 (4): 464–469. https://doi.org/10.1016/j.tourman.2009.04.013.
- Xia, Jianhong Cecilia, Panlop Zeephongsekul, and Colin Arrowsmith. 2009. “Modelling Spatio-temporal Movement of Tourists Using Finite Markov Chains.” Mathematics and Computers in Simulation 79 (5): 1544–1553. https://doi.org/10.1016/j.matcom.2008.06.007.
- Xia, Jianhong Cecilia, Panlop Zeephongsekul, and David Packer. 2011. “Spatial and Temporal Modelling of Tourist Movements Using Semi-Markov Processes.” Tourism Management 32 (4): 844–851. https://doi.org/10.1016/j.tourman.2010.07.009.
- Xiao-Ting, Huang, and Wu Bi-Hu. 2012. “Intra-attraction Tourist Spatial-temporal Behaviour Patterns.” Tourism Geographies 14 (4): 625–645. https://doi.org/10.1080/14616688.2012.647322.
- Xu, Yang, Jiaying Xue, Sangwon Park, and Yang Yue. 2021. “Towards a Multidimensional View of Tourist Mobility Patterns in Cities: A Mobile Phone Data Perspective.” Computers, Environment and Urban Systems 86:101593. https://doi.org/10.1016/j.compenvurbsys.2020.101593.
- Xu, Yang, Dan Zou, Sangwon Park, Qiuping Li, Suhong Zhou, and Xinyu Li. 2022. “Understanding the Movement Predictability of International Travelers Using a Nationwide Mobile Phone Dataset Collected in South Korea.” Computers, Environment and Urban Systems 92:101753. https://doi.org/10.1016/j.compenvurbsys.2021.101753.
- Xue, Lan, and Yi Zhang. 2020. “The Effect of Distance on Tourist Behavior: A Study Based on Social Media Data.” Annals of Tourism Research 82:102916. https://doi.org/10.1016/j.annals.2020.102916.
- Yang, Yang, Lan Jiang, and Zili Zhang. 2021. “Tourists on Shared Bikes: Can Bike-sharing Boost Attraction Demand?.” Tourism Management 86:104328. https://doi.org/10.1016/j.tourman.2021.104328.
- Yang, Yang, Dong Li, and Xiang Li. 2019. “Public Transport Connectivity and Intercity Tourist Flows.” Journal of Travel Research 58 (1): 25–41. https://doi.org/10.1177/0047287517741997.
- Yang, Jie, Jian Xu, Ming Xu, Ning Zheng, and Yu Chen. 2014. “Predicting Next Location Using a Variable Order Markov Model.” In Proceedings of the 5th ACM SIGSPATIAL International Workshop on GeoStreaming, 37–42.
- Yuan, Quan, Gao Cong, Zongyang Ma, Aixin Sun, and Nadia Magnenat Thalmann. 2013. “Time-Aware Point-of-Interest Recommendation.” In Proceedings of the 36th International ACM SIGIR Conference on Research and Development in Information Retrieval, 363–372.
- Yun, Hee Jeong, Dong Jin Kang, and Myong Jae Lee. 2018. “Spatiotemporal Distribution of Urban Walking Tourists by Season Using GPS-based Smartphone Application.” Asia Pacific Journal of Tourism Research 23 (11): 1047–1061. https://doi.org/10.1080/10941665.2018.1513949.
- Zhao, Shenglin, Irwin King, and Michael R. Lyu. 2013. “Capturing Geographical Influence in POI Recommendations.” In International Conference on Neural Information Processing, 530–537. Springer.
- Zhao, Xi, Xiaoni Lu, Yuanyuan Liu, Jun Lin, and Jun An. 2018. “Tourist Movement Patterns Understanding From the Perspective of Travel Party Size Using Mobile Tracking Data: A Case Study of Xi'an, China.” Tourism Management 69:368–383. https://doi.org/10.1016/j.tourman.2018.06.026.
- Zheng, Weimin, Xiaoting Huang, and Yuan Li. 2017. “Understanding the Tourist Mobility Using GPS: Where is the Next Place?.” Tourism Management 59:267–280. https://doi.org/10.1016/j.tourman.2016.08.009.
- Zheng, Weimin, Haipeng Ji, Congren Lin, Wenhui Wang, and Bilian Yu. 2020. “Using a Heuristic Approach to Design Personalized Urban Tourism Itineraries with Hotel Selection.” Tourism Management 76:103956. https://doi.org/10.1016/j.tourman.2019.103956.
- Zheng, Yunhao, Naixia Mou, Lingxian Zhang, Teemu Makkonen, and Tengfei Yang. 2021. “Chinese Tourists in Nordic Countries: An Analysis of Spatio-Temporal Behavior Using Geo-Located Travel Blog Data.” Computers, Environment and Urban Systems 85:101561. https://doi.org/10.1016/j.compenvurbsys.2020.101561.
- Zhong, Lina, Sunny Sun, and Rob Law. 2019. “Movement Patterns of Tourists.” Tourism Management75:318–322. https://doi.org/10.1016/j.tourman.2019.05.015.