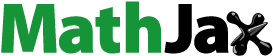
ABSTRACT
The pre-phase landslides will have a legacy effect on future landslides, changing the landslide susceptibility. This study attempts to establish a reasonable post-seismic landslide susceptibility model and analyze the spatio-temporal characteristics of landslide susceptibility in the Jiuzhaigou MS7.0 earthquake-struck region. Firstly, an integrated ‘space-ground’ monitoring technology is used to establish a multi-temporal post-seismic landslide dataset. Then, the buffer analysis method documents the spatio-temporal characteristics of post-seismic landslides. Thirdly, the distance is selected as an indicator to quantify the legacy effect. An improved time-variant model is established to evaluate the post-seismic landslide susceptibility. Finally, the spatio-temporal characteristics of landslide susceptibility are generalized, to sum up the changing law. Our results show that the post-seismic landslides are gradually closer to the pre-phase landslides with time. Distance is a critical factor in measuring the impact of pre-phase landslides on future landslides, which can improve the assessment accuracy of the post-seismic landslide susceptibility model. After a large seismic event, the correlation between landslide susceptibility and earthquakes gradually weakens. Post-seismic landslide prevention should focus on the pre-phase landslide expansion triggered by rainfall. Moreover, it should clean up the landslide deposits in time and reasonably dredge the debris flows to avoid secondary geological disasters.
1. Introduction
Strong earthquakes occurring in the mountainous region can not only trigger a large number of landslides but also cause severe vegetation destruction, surface deformation and cracks, geomorphic changes, and a large amount of loose material (Lin et al. Citation2009; Wang et al. Citation2014; Xu et al. Citation2016; Guo, Guo, and Feng Citation2021a; Carrión-Mero et al. Citation2021). This study uses the expression coseismic landslides to refer to landslides triggered within seconds to minutes of strong ground shaking and post-seismic landslides to refer to the landslide activities that occurred after a significant seismic event (Yegian, Marciano, and Ghahraman Citation1991; Wang Citation2004; Kritikos, Robinson, and Davies Citation2015; Adolfo et al. Citation2019; Fan et al. Citation2019a; Serey et al. Citation2019; Guo et al. Citation2021b). Previous studies show that the post-seismic landslides might be active over ten years, and then they will gradually become stable in strong earthquake-struck regions (e.g. Tang Citation2010; Cui et al. Citation2011; Huang and Li Citation2014; Chen et al. Citation2020). Therefore, evaluating the landslide susceptibility and summarizing the variation characteristics of post-seismic landslide susceptibility are critical for post-seismic landslide treatment and ecological restoration.
Currently, the conventional estimation models of landslide susceptibility can be divided into qualitative and quantitative ones. The qualitative model mainly relies on expert experiences to estimate susceptibility, and the quantitative model relies on data acquisition and analysis (e.g. Cao et al. Citation2019; Guzzetti et al. Citation1999; Shao et al. Citation2018; Caccavale, Matano, and Sacchi Citation2017; Zang et al. Citation2020; Adolfo Citation2021a; Arroyo-Solórzano, Quesada-Román, and Barrantes-Castillo Citation2022). The statistical models belong to quantitative models. Statistical models involve the statistical determination of combinations of variables that have led to landslide occurrence in the past. Then, they can predict the susceptibility of areas currently free of landslides but where similar conditions exist (Dai et al. Citation2001). Statistical models often have high accuracy and have been successfully used in many studies (e.g. García-Rodríguez et al. Citation2008; Budimir, Atkinson, and Lewis Citation2015; Lombardo and Mai Citation2018; Reichenbach et al. Citation2018; Adolfo Citation2021b).
Traditionally, landslide susceptibility is assumed time-invariant based on the assumption that the likelihood of landslide occurrence is time-independent for a given region and trigger event (Samia et al. Citation2020). The temporal component is usually investigated separately from the susceptibility in the above models and some early case studies (e.g. Aleotti Citation2004; Guzzetti et al. Citation1999; Wu et al. Citation2015). Because of this, few examples exist where the spatial and temporal components of the landslide hazard are combined (e.g. Ghosh et al. Citation2012; Fan et al. Citation2020). However, dynamic variations of landslide susceptibility in time are very common, and they exist in not only rainfall-induced landslides (e.g. Wang et al. Citation2022; Lin et al. Citation2022; Steger et al. Citation2022) but also earthquake-induced landslides (Roberts, Jones, and Boulton Citation2021). It is well-described that climatic, tectonic, and anthropogenic drivers may vary with time after the earthquake (Jones et al. Citation2021). Especially the pre-phase coseismic landslides could influence the landscape evolution and future landslide occurrences, known as the legacy effect. Therefore post-seismic landslide susceptibility also can be varied with time.
Recent studies have proposed the concept of time-variant landslide susceptibility based on the multi-temporal landslide dataset. It should be noted that time-variant landslide susceptibility still meets the definition of susceptibility, which does not include triggering factors. Fan et al. (Citation2020) calculated the post-seismic time-variant landslide susceptibility by updating the multi-temporal post-seismic landslide dataset inputted into the susceptibility model. However, this method ignores the legacy effect between landslides of different phases. Samia et al. (Citation2017a) accounted for landslide legacy effects across time (Lombardo and Tanyas Citation2021). Subsequently, researchers further described and quantified this phenomenon (Samia et al. Citation2017b). Based on the above research, Samia et al. (Citation2018) put the legacy effect into the landslide susceptibility model, and the results showed that compared with conventional landslide susceptibility models, models considering the landslide legacy effect could significantly improve the evaluation accuracy of landslide susceptibility (Samia et al. Citation2020). However, landslide susceptibility models based on path dependency theory generally need high-quality datasets to quantify the legacy effect of the pre-phase landslides. They need to use the accurate geometric attributes of each landslide to establish the detailed spatial association of multi-temporal post-seismic landslides. Establishing a multi-temporal landslide dataset in a large region requires many resources and time (Reichenbach et al. Citation2018), and there is insufficient data for this type of research in many areas. The existing post-seismic time-variant susceptibility models put forward high requirements for establishing landslide datasets and processing multi-temporal geometric relationships. Globally, few multi-temporal landslide datasets can be used for this method. From this point of view, this method is challenging to implement and is currently only carried out in several study cases with small areas (Samia et al. Citation2017b; Roberts, Jones, and Boulton Citation2021).
The 2017 MS7.0 Jiuzhaigou earthquake provides an excellent example to explore a reasonable post-seismic landslide susceptibility model and analyze the spatio-temporal characteristics of susceptibility. The multi-temporal landslide dataset is established using an integrated ‘space-ground’ monitoring technology. This study quantifies the legacy effect and attempts to develop an improved post-seismic time-variant landslide susceptibility model. An improved post-seismic time-variant landslide susceptibility model is simple and can be applied to more earthquake cases with a larger research area globally. This study also tries to obtain the changing laws of post-seismic landslide susceptibility by analyzing their spatio-temporal characteristics of them. It has important implications for predicting post-seismic landslides, defining hazard prevention zones, and evaluating post-seismic recovery in similar geological environments.
2. Material and methods
2.1. Study area and material
The Jiuzhaigou natural heritage site is located in the northeastern Tibetan Plateau. The terrain is high in the South and low in the North. It is the topographic transition zone from the Tibetan Plateau to Sichuan Basin. The Devonian to Triassic sedimentary rocks are prominent in the study area (e.g. Dong et al. Citation2020; Zhang et al. Citation2020). The most representative lithology is a thick sequence of deep marine deposits, including limestone, flysch complex, and sandstone. Quaternary loose deposits are distributed in river valleys (Dai et al. Citation2017). Deep-incision gullies and high mountains with slopes greater than 30° characterize the landforms. The maximum peak is over 4700 m a.s.l., and the minimum elevation is about 1200 m a.s.l. (Fan et al. Citation2018; Guo et al. Citation2021c) ().
Figure 1. Jiuzhaigou MS7.0 Earthquake. (a) The map shows the location of Jiuzhaigou, (b) Tectonic setting of the 2017 Jiuzhaigou MS7.0 Earthquake (modified by Guo et al. Citation2021b). MJF: Minjiang Fault, NHYF: North Huya Fault, TZF: Tazang Fault.

At 21:19:46 on 8 August 2017, an MS7.0 earthquake occurred in Jiuzhaigou County, Aba Autonomous Prefecture, Sichuan Province, China, with an affected area of more than 4000 km2 (VII degrees seismic intensity and above). Due to the high magnitude, shallow source, high intensity of the earthquake, and fragile geological environment (high slope gradient, fragile lithology), this earthquake triggered thousands of landslides (e.g. Wu et al. Citation2018; Dong et al. Citation2020). Due to the dense vegetation in the area, the damage caused by landslides is significant and easy to identify in optical remote sensing images. Thus, the Jiuzhaigou area can provide a rare natural laboratory for studying post-seismic landslide susceptibility. Moreover, as a United Nations Educational, Scientific and Cultural Organization (UNESCO) natural heritage site, monitoring and evaluating landslides in Jiuzhaigou are essential and urgent for tourism safety and ecological environment protection and management.
This study used the pre-seismic and post-seismic Sentinel-2 images (10 m), post-seismic aerial images (1 and 0.16 m), and a series of Google Earth pre-seismic images and post-seismic images (about 0.5∼1 m) to identify landslides () and establish multi-temporal post-seismic landslide dataset. The remote sensing images with multi-spatial resolution can ensure the accuracy and completeness of the landslide dataset and avoid false extraction. This study selects Sentinel-2 images that covered the entire study area from a similar time each year (29 July 2017, 7 September 2017, 27 September 2019, and 27 August 2020) with low cloud and good data quality. The quality of remote sensing images in 2018 was poor, and the data were not used. The ortho-level aerial remote sensing image of emergency observation (10 August 2017) is provided by the Bureau of Surveying and Mapping. The post-seismic ortho-level unmanned aerial vehicle (UAV) image acquired by the UAV (CW-15) was taken on 10 June 2020. The ortho-level aerial remote sensing image of emergency observation and the UAV images cover all the key areas. The Google Earth images were taken on 13 August 2013, 21 October 2015, and 27 September 2019, covering the entire study area. These Google images with high spatial resolution were used due to without aerial images in 2013, 2017, and 2019. Moreover, all of the images are registered before using them. This processing method can also help ensure the completeness and consistency of the landslide dataset.
Table 1. Remote sensing data used in this study.
Additionally, this study selected seismic, topographic, geological, and human activity factors as the basic influencing factors of susceptibility, which had been widely used in previous studies (e.g. Huang and Li Citation2008; Keefer Citation2000). They included peak ground acceleration (PGA), slope, aspect, elevation, strata, and distances to seismogenic fault, river, and road () (Fan et al. Citation2020; Talaei Citation2014; Qi et al. Citation2011; Zou et al. Citation2022). Among them, terrain data such as slope, aspect, and elevation were obtained by ALOS DEM (12.5 m) (ASF DAAC Citationn.d.) (http://search.asf.alaska.edu/, accessed May 2, 2022). Strata and seismogenic fault data were from the 1:200000 geological map (Li et al. Citation2017) (http://dcc.ngac.org.cn/geologicalData, accessed May 2, 2022). The USGS website (USGS Earthquake Hazards Program Citation2017) (http://earthquake.usgshakemap.gov, accessed May 2, 2022) provided seismic data such as PGA. The data on human activities, such as roads and rivers, came from the national data on roads and rivers (Geospatial Data Cloud Citation2019) (http://www.gscloud.cn/sources, accessed May 2, 2022).
Figure 2. Influencing factors in Jiuzhaigou (modified by Guo et al. Citation2021c). (a) Slope, (b) Aspect, (c) Elevation, (d) PGA, (e) Seismogenic fault (Dai et al. Citation2017; Fan et al. Citation2020), (f) Strata, (g) River, (h) Road.
2.2. Methods
The steps of this study are listed as follows (): (1) The multi-temporal post-seismic landslide dataset is quickly and accurately established by the integrated ‘space-ground’ monitoring technologies, combined with automatic classification technology; (2) The spatio-temporal characteristics of multi-temporal post-seismic landslides are summarized and obtaining the typical factor quantifying the legacy impact of pre-phase landslides on future landslides; (3) The typical influencing factor is put into the susceptibility model, establishing an improved post-seismic time-variant landslide susceptibility model and quickly getting more accurate landslide susceptibility results; (4) The spatio-temporal characteristics of post-seismic landslide susceptibility are analyzed.
2.2.1. Landslide identification
The post-seismic landslides are new landslides that occur in areas free of landslides in the past (Wang Citation2004). The post-seismic landslides mainly come from two types of landslides. One is the landslide after the earthquake formed by the expansion of the pre-phase landslide (pre-seismic landslide, coseismic landslide, or post-seismic landslide in the previous phase), which is referred to as the pre-phase landslide expansion. Another one is the single landslide after the earthquake, far away from the pre-phase landslide, referred to as the new single post-seismic landslide (Li, Wang, and Liu Citation2018; Guo et al. Citation2021b).
The Jiuzhaigou area has dense vegetation, with a coverage rate of 85.5% (Deng Citation2011). In most landslide areas of Jiuzhaigou, the vegetation on the slopes was destroyed. So, it is easy to identify the bare land where landslides damaged vegetation. The vegetation in the area above 3700 m a.s.l. is sparse due to frost weathering and permanent snow, and landslides are more challenging to find there. However, previous studies showed that landslides above 3700 m are rare in the Jiuzhaigou area (Wu et al. Citation2018). Therefore, this study only extracts landslides in areas lower than 3700 m a.s.l. to ensure a more accurate dataset.
In this study, we used an integrated ‘space-ground’ monitoring technology to extract landslides within 2055 km2 of the Jiuzhaigou area. Firstly, the support vector machine (SVM) with high accuracy and efficiency (Vapnik Citation1998) was used to preliminarily identify the bare land (exposed ground without plants, snow, or water) which is larger than half a pixel (10 m/pixel) by Sentinel-2 remote sensing images (). Then, visual interpretation was used to verify whether the bare land represented the landslide results and eliminate false extraction areas by the aerial remote sensing images (aerial remote sensing image of emergency observation or UAV remote sensing images) or Google Earth images. The false extraction areas mainly include houses, roads, squares, and other impervious surfaces. The impervious surfaces such as houses, roads, and squares have significant geometric features, making them easy to identify and eliminate by visual interpretation. Finally, we conducted field investigations to verify the results for key or uncertain areas.
The multi-temporal landslide dataset is established quickly and accurately. The landslide areas of each phase in the dataset include all landslide areas in the previous phase. For example, the 2017 landslide areas include pre-seismic landslide areas and coseismic landslide areas; the 2019 landslide areas include pre-seismic landslide areas, coseismic landslide areas, and 2017–2019 post-seismic landslide areas (the landslides occurred from August 2017 to September 2019). After obtaining the accurate landslides polygons, this study used the 2017 landslide areas to erase the 2019 landslide areas to get the post-seismic landslide polygons in 2017-2019, and then this study used the 2019 landslide areas to erase the 2020 landslide areas to obtain the post-seismic landslide polygons in 2019-2020. Finally, a multi-temporal post-seismic landslide dataset was established. The sources of remote sensing data and the landslide extraction method in this study can help ensure the completeness and consistency of the landslide dataset.
2.2.2. Landslide spatio-temporal characteristics
Pre-phase landslides have legacy effects, impacting the new post-seismic landslides in the future (Roberts, Jones, and Boulton Citation2021; Samia et al. Citation2017a). However, the existing indicator quantifying the legacy effect is not simple enough. This study used buffer analysis to explore the spatio-temporal relationship between post-seismic landslides of each phase and their pre-phase landslides. Then, this study analyzed the spatio-temporal characteristics and summarized the spatio-temporal distribution law of post-seismic landslides. Finally, the distance factor is used to quantify the legacy effect in each phase. It helped to establish an improved post-seismic time-variant landslide susceptibility model.
The buffer analysis of the 2017–2019 post-seismic landslides distribution was taken as an example to explain the principle, as shown in . This method starts from the edge of the pre-phase landslides and uses 50 m (or other length) as the buffer interval to generate a multi-level buffer zone. Then, this study counted landslides falling in different buffer zones. Notably, the distance factor refers to how long the landslide of each phase is from the edge of pre-phase landslides. For 2017–2019 post-seismic landslides, buffer zones started from the edge of the landslide in 2017; for 2019–2020 post-seismic landslides, buffer zones developed from the edge of the landslide in 2019.
2.2.3. Landslide susceptibility model
Statistical models are frequently used in landslide susceptibility methods. The common statistical models can be divided into single models and coupling models. The common single models include the information (I) model, certainty factor (CF) model, SVM, logistic regression (LR) model, and so on. The common coupling models are coupled by some single models, such as the information and logistic regression (I + LR) coupling model (Guo et al. Citation2021c). The coupling models are usually much better than the single models which make up them (Zhang et al. Citation2020). The I + LR coupling model is composed of the I and the LR models and is easy to implement. It has high accuracy and is suitable for landslide susceptibilities in different geological backgrounds, such as the Alpine karst region and the loess region (e.g. Fan et al., Citation2018; Tian et al. Citation2020; Guo et al. Citation2021c). Therefore, this study selected the I + LR model as the primary model to establish an improved post-seismic time-variant landslide susceptibility model. The I model, LR model, and I + LR coupling model are as follows.
In the I model, the landslide probability is represented by the entropy decrease in the landslide prediction process. The average uncertainty reduction caused by the combination of the factors equals the entropy change of the landslide system. The greater the information value is, the greater the landslide possibility is (Yin Citation2013).
In every factor (i = 1, 2, 3, … , n), the information value of each category
(j = 1, 2, 3, … , m) can be listed as follows (Ba et al. Citation2017; Pasang and Kubicek Citation2018; Citation2020):
(1)
(1) where
is the information value of the category
in the factor
;
is the landslide area in the category
;
is the total area of landslides in the whole study area;
is the area of category
;
is the total area of the whole study area;
is the number of factors considered;
is the number of categories in every factor. Therefore, the total information I, representing landslide possibility, is the sum of the information values of all influencing factors
.
(2)
(2) where
is the number of factors considered. If I < 0, the landslide possibility is lower than the average of the whole area; if I = 0, the landslide possibility is equal to the average of the whole area; if I > 0, the landslide possibility is higher than the average of the whole area. The greater the information value I is, the greater the landslide possibility.
The LR model predicts the probability of events by establishing a regression relationship between a dependent variable and multiple independent variables. In analysis, the dependent variable is a binary variable representing whether there is a landslide. The value ‘1’ and ‘0’ of
indicate the occurrence and non-occurrence of landslides, respectively. An LR model has
independent variables (
,
, … ,
). Influenced by
factors, the conditional probability of landslide is
=
(
= 1 |
,
,
, … ,
). Then the model can be expressed as (Ayalew and Yamagishi Citation2005; Mathew, Jha, and Rawat Citation2009; Conoscenti et al. Citation2014):
(3)
(3)
(4)
(4) where Z is the linear combination;
(
= 1, 2, 3, … , n) is the independent factor;
is the regression constant;
is the coefficient that measures the contribution of the independent factor
(
= 1, 2, 3, … , n);
is the prediction value of landslide probability.
In the conventional I + LR coupling model, the information value in each factor calculated by the formula (1), substitute for the
of the formula (3) as the index value of the LR model. We calculate the constant and regression coefficients of the LR model by Statistical Product Service Solutions (SPSS) 25 software (IBM Corp Citation2017) and then put them into formula (4) to obtain P, the landslide probability.
The multi-temporal post-seismic landslide dataset is updated quickly and accurately by automatic classification technology in this study. Although the post-seismic landslide susceptibility can vary with time by putting the updated multi-temporal landslide dataset into the conventional susceptibility model, the conventional susceptibility model still can not consider the legacy effect. So, the conventional susceptibility model can not accurately calculate the post-seismic time-variant susceptibility.
In this study, the distance factor came from the buffer analysis of the post-seismic landslide distribution in each phase. We put the distance factor, whose information value can represent the legacy effect of landslides, into the conventional landslide susceptibility model, establishing an improved time-variant landslide susceptibility model. The improved landslide susceptibility model not only quantifies the legacy effect but also uses the multi-temporal post-seismic landslide data. In the improved landslide susceptibility model, the information value of each factor, including the distance factor in each phase calculated by the formula (1), substitute for the
of the formula (3) as the index value of the LR model. The subsequent processing of the improved landslide susceptibility model is the same as that of the conventional I + LR coupling model. We also calculate the constant and regression coefficients of the LR model in each phase by using SPSS 25 software and then put them into formula (4) to obtain P, representing the landslide probability of each phase.
2.2.4. Accuracy verification of susceptibility results
The landslide susceptibility was divided into five classes using Jenks natural breaks optimization: very low, low, moderate, high, and very high. The Jenks natural breaks optimization divides the data into similar classes according to the natural (non-artificial) turning points or breakpoints of the data, making the classification very objective. Many studies have shown that this classification method is reasonable (e.g. Aditian, Kubota, and Shinohara Citation2018; Dai et al. Citation2017).
This study quantitatively evaluated the accuracy of the post-seismic time-variant landslide susceptibility model by the receiver operating characteristic (ROC) curves. The ROC curve is regarded as an effective method to evaluate the prediction ability of models (Swets Citation1988). The abscissa of the curve is the specificity or the false positive rate, which is the probability that the non-landslide is judged as a landslide by the model; the ordinate is the sensitivity or the true positive rate, which is the probability that the landslide is judged as a landslide. The area under the curve (AUC) is a quantitative index of the ROC curve to evaluate the model. The AUC value ranges from 0.5–1. The closer the AUC value is to 1, the better the prediction effect of the model is. If AUC = 0.5, the prediction result of the model is random; if AUC is at 0.5–0.7, the accuracy of the model is low; if AUC is at 0.7–0.9, the accuracy of the model is high; if AUC > 0.9, the accuracy of the model is very high (Fawcett Citation2006). In this study, the ROC curves and AUC values of each model were obtained by SPSS 25 software.
3. Results
3.1. Landslide inventory
The landslide areas of 2017 (after the Jiuzhaigou earthquake), 2019, and 2020 extracted in this study are 13.7, 14.9, and 16.5 km2, respectively. In the landslide identification process, visual interpretation of Google Earth images, aerial remote sensing images, and field investigations were conducted to verify the landslide results, such as landslides near Five Flower Lake, Mirror Lake, and Heye community (). It should be noted that all of the above landslide areas included the area of coseismic landslides, see .
Figure 5. Remote sensing images and field photo around Five Flower Lake. (a) 2013, (b) 2017, (c) 2020, (d) Field photo.

Figure 6. Remote sensing images and field photo around Mirror Lake. (a) 2013, (b) 2017, (c) 2020, (d) Field photo.

Figure 7. Remote sensing images and field photo around Heye community. (a) 2013, (b) 2017, (c) 2020, (d) Field photo.

Figure 8. Landslides area distribution after the 2017 Jiuzhaigou MS7.0 earthquake. (a) 2017, (b) 2019, (c) 2020.

Then, based on the above landslide areas of 2017 (after the Jiuzhaigou earthquake), 2019, and 2020, this study used the ArcGIS-Analysis-Erase tool to get the real post-seismic landslides. The results are as follows: the 2017–2019 post-seismic landslide area is 3.62 km2; the 2019–2020 post-seismic landslide area is 3.38 km2 ().
3.2. Spatio-temporal characteristics of post-seismic landslide
This study generated buffer polygons with different distances in 2017–2019 and 2019–2020. The buffer polygons of each phase were used to cut all the post-seismic landslide polygons of each phase, not one polygon. Then, this study made statistics on the area of the already cut post-seismic landslide polygons falling in different buffer polygons. The 2017–2019 and 2019–2020 results are shown in and . Over 85% of the 2017–2019 post-seismic landslides are distributed within 200 m around the 2017 coseismic landslide area. More than 95% of the 2019–2020 post-seismic landslides are distributed within 200 m around the 2017–2019 landslide area. The trends in and our field investigation show that the pre-phase landslide expansion is the primary source of landslides after the Jiuzhaigou earthquake (). Moreover, the post-seismic landslides in 2019–2020 are closer to the pre-phase landslides than those in 2017–2019 (). More than 90% of the 2019–2020 post-seismic landslides are distributed within 50 m around the 2017–2019 landslide area. It suggests that the probability of new single landslides occurring far away from the pre-phase landslides is reduced with time. The spatio-temporal distribution of landslides shows more significant spatial aggregation characteristics. Therefore, the distance to the pre-phase landslide is a reasonable indicator to quantify the legacy effect of the pre-phase landslide.
Figure 11. Examples of post-seismic landslides. Five-Flower Lake landslide edge: (a) 2017, (b) 2020; Heye landslide edge: (c) 2017, (d) 2020; Shuzheng landslide edge: (e) 2017, (f) 2020.

Table 2. Spatio-temporal distribution of post-seismic landslides in 2017–2019 and 2019–2020.
3.3. Post-seismic landslide susceptibility
This study generates five training datasets and five testing datasets in 2017–2019 and 2019–2020, respectively, to compare the accuracy and robustness of the improved and the conventional landslide susceptibility model. The method of obtaining each training and testing dataset is as follows: 3000 points are randomly selected in the landslide area by ArcGIS. Then random numbers were assigned to the landslide points by Excel. The random numbers were sorted to select the first 2250 points as training samples and the remaining 750 landslide points as testing samples. The same procedure was repeated in the non-landslide area. Finally, this study can produce a training dataset with 4500 points (2250 landslide points and 2250 non-landslide points) and a testing dataset with 1500 points (750 landslide points and 750 non-landslide points). This method ensures that the points in the training and testing datasets do not overlap.
Five training datasets are used to train the improved landslide susceptibility model and the conventional landslide susceptibility model in each phase, obtaining five improved landslide susceptibility models and five conventional landslide susceptibility models. Then the testing dataset is used to evaluate the accuracy, and the ROC curves are obtained by SPSS 25 software (). The results show that the accuracy of the five improved landslide susceptibility models is higher than that of the five conventional landslide susceptibility models in each phase. The improved landslide susceptibility model in this study is robust and highly accurate.
Figure 12. ROC curves and AUC values of landslide susceptibility models. 2017–2019: (a) Testing dataset 1, (c) Testing dataset 2, (e) Testing dataset 3, (g) Testing dataset 4, (i) Testing dataset 5; 2019–2020: (b) Testing dataset 1, (d) Testing dataset 2, (f) Testing dataset 3, (h) Testing dataset 4, (j) Testing dataset 5.
Although selecting the group of models with the best performance in this study can achieve the highest accuracy, other studies generally only have one training dataset and hope to obtain good results. Therefore, it is more realistic for us to randomly select a group instead of selecting the most effective group. Finally, this study randomly selects a group of improved and conventional landslide susceptibility models in each phase to calculate the post-seismic landslide susceptibility of the Jiuzhaigou area, as shown in and .
Figure 13. Post-seismic landslide susceptibility calculated by the improved time-variant landslide susceptibility model. (a) 2017–2019, (b) 2019–2020.

Figure 14. Post-seismic landslide susceptibility calculated by conventional landslide susceptibility model. (a) 2017–2019, (b) 2019–2020.

For spatial distribution, the post-seismic landslide susceptibilities obtained by the conventional susceptibility model and the improved landslide susceptibility model have similar spatial distribution, see and . But, the high and very high susceptibility areas of the latter are significantly reduced compared with the former. The susceptibility in Zhangzha town, which is the human habitation, is different too. The field investigation shows that the post-seismic landslide activities near Zhangzha town are weak, and no large-scale new landslides exist. Therefore, the spatial distribution of the improved landslide susceptibility model is more reasonable than the conventional susceptibility model.
This study used the coseismic landslide susceptibility in 2017 () (Guo et al. Citation2021c) as a benchmark for analyzing the changing trend of post-seismic landslide susceptibility. Then, the proportions of each susceptibility class in 2017, 2017–2019, and 2019–2020 landslide susceptibility are counted ( and ).
Figure 15. Coseismic landslide susceptibility in 2017 (modified by Guo et al. Citation2021c).

Table 3. Changes of post-seismic landslide susceptibility distribution by the improved landslide susceptibility model.
Table 4. Changes of post-seismic landslide susceptibility distribution by the conventional landslide susceptibility model.
The moderate landslide susceptibility area in 2017 accounted for the largest proportion, 31.18%. The proportions of the very high and very low landslide susceptibility areas are close, 13.56% and 10.88%, respectively. The post-seismic landslide susceptibility calculated by the improved landslide susceptibility model in 2017–2019 shows that high and very high susceptibility areas decreased rapidly compared with the coseismic landslide susceptibility in 2017. Their total proportion decreases from 32.61% to 11.00%. The very low susceptibility area quickly grows, and its proportion increases from 13.56% to 51.87%. Overall, with the landslide susceptibility from high to low, their proportions are from low to high. The post-seismic landslide susceptibility calculated by the improved landslide susceptibility model in 2019–2020 shows that the high and very high susceptibility areas continue to decrease compared with the post-seismic landslide susceptibility in 2017–2019. Their total proportion decreases from 11.00% to 8.71%. The very low susceptibility area continued to grow, and its proportion increased from 51.87% to 55.57%. Overall, with the landslide susceptibility from high to low, their proportions are from low to high. The changing trend of post-seismic landslide susceptibility calculated by the conventional landslide susceptibility model is similar to that calculated by the improved landslide susceptibility model.
Notably, this study uses the posterior method further to compare the performance of the conventional and improved models. It makes statistics of 2019–2020 post-seismic landslides distributed in every 2017–2019 post-seismic landslide susceptibility classification area (). As a result of the conventional model, 81.89% of the 2019–2020 post-seismic landslides fell in the high and very high susceptibility areas. As a result of the improved model, 80.07% of the 2019–2020 post-seismic landslides fell in high and very high susceptibility areas. The total area proportion of high and very high susceptibility areas in the improved model is 11.00%, and that in the conventional model is 19.25% ( and ). Almost the same proportion of post-seismic landslides fell in the high and very high susceptibility areas of the conventional and improved models (). But, the high and very high susceptibility areas of the conventional model are twice as large as those of the improved model. It means the improved landslide susceptibility model has better-predicting performance than the conventional one.
Table 5. 2019–2020 post-seismic landslides distributed in post-seismic landslide susceptibility classification area of 2017–2019.
3.4. Spatio-temporal characteristics of post-seismic landslide susceptibility
This study analysis spatio-temporal characteristics of post-seismic landslide susceptibility and explores the effects of the different factors on the change characteristics by calculating and ranking the Pearson correlation coefficients of influencing factors (). Pearson correlation coefficient is also called R-value, R = −1∼1. When P < 0.01, it indicates a significant correlation. The greater the is, the stronger the correlation is, and vice versa.
Table 6. Ranking of Pearson correlation coefficient in improved time-variant landslide susceptibility model.
The high landslide susceptibility area of 2017 is mainly distributed in areas with high PGA, as shown in and (d). The P-value of every factor is less than 0.01. The R-values of PGA and fault were 0.790 and 0.777, which ranked first and second, respectively. The coseismic landslide susceptibility is significantly related to earthquake-related factors, especially PGA. The high post-seismic landslide susceptibility in 2017–2019 is distributed along both sides of the seismogenic fault, as shown in and (d) (e). In Zhangzha Town, the decline of the post-seismic landslide susceptibility in 2017–2019 was apparent. The P-value of every factor is less than 0.01. The R-values of fault and PGA were 0.708 and 0.318, which ranked first and fifth, respectively. The correlation with PGA had significantly weakened. The R-value of distance was 0.598, which ranked second. The post-seismic landslides susceptibility in 2019–2020 continued to decline. The high and very high landslide susceptibility areas shrunk rapidly towards the concentration area of landslides. Except for the elevation factor, the P-value of other factors is less than 0.01. The R-values of fault and PGA were 0.603 and 0.428, which ranked second and fourth, respectively. The correlation with seismogenic fault also weakened, as shown in and (d) (e). The R-value of distance was 0.696, which ranked first. The correlation between post-seismic landslide susceptibility and distance after the earthquake is the strongest. The result indicates that the correlation between new post-seismic landslides and seismic events gradually weakened with time. The landslide susceptibility was mainly affected by earthquake-related factors such as seismogenic fault and PGA during the earthquake and gradually changed to be primarily influenced by the distance to the pre-phase landslide.
4. Discussion
In this section, we focus first on why there are legacy effects and how to quantify legacy effects. Then, we compare our post-seismic susceptibility model with similar studies in other regions and discuss the implications of our result. We also discuss the replicability of the improved model. In the end, we discuss factors affecting the spatio-temporal characteristics of post-seismic landslide susceptibility.
4.1. Causes and quantification of legacy effects
This study shows that the new post-seismic landslides include the pre-phase landslides expansion and the new single post-seismic landslides. However, the number of new single post-seismic landslides in Jiuzhaigou is not more than 10%, and new single post-seismic landslides distribute in the whole area. Thus, the spatial distribution of new single post-seismic landslides () is scattered. These post-seismic landslides are not very active, and their activities decay rapidly. The pre-phase landslides are activated by rainfall and other factors, and their landslide areas are expanded, which is the main form of post-seismic landslide activity. This form of landslide mainly comes from two types (): (1) Since the lower rock mass slides in the pre-phase, the slope gradually forms cracks from bottom to top. The rock mass on the upper part of the slope loses the support of the lower part, and then the rainfall increases the pore water pressure of the cracks. The upper rock mass is easy to slide and forms a retrograde landslide. (2) Coseismic landslide deposits are often loose, fractured, and easy to move under heavy rain in the area. The new landslide also easily occurs at the front edge of the pre-phase landslide. The main increasing form of post-seismic landslides determines the legacy effect of pre-phase landslides on future landslides. The post-earthquake investigations and statistical results show that most of the new post-seismic landslides in each phase are distributed within 200 m away from the pre-phase landslides. Distance is the key factor in quantifying the legacy effect in the area with more pre-phase landslide expansion. This study used the multi-temporal post-seismic landslide and reasonably inputted the distance to the landslide susceptibility model, getting an improved post-seismic time-variant landslide susceptibility model.
Figure 16. Diagram showing the development of the new single post-seismic landslides. (a) Slope without landslide, (b) New single post-seismic landslides (uw: Pore water pressure).

Figure 17. Diagram showing the development of the pre-phase landslides expansion. (a) Coseismic landslide, (b) Post-seismic landslide caused by cracks propagation, (c) Post-seismic landslide caused by deposits movements, (d) Post-seismic landslides caused by cracks propagation and deposits movements (uw: Pore water pressure).

4.2. The post-seismic susceptibility model compared with similar studies
Chen et al. (Citation2019) studied the Wenchuan post-seismic landslide susceptibility in 2009 and 2011. They graded the activity intensity of landslides, calculated the susceptibility of landslides with different activity intensities, and then used Spatial Overlay to obtain the final susceptibility results. 70.2% of the post-seismic landslides fell in the high and very high susceptibility areas in 2009, whose area accounts for 32.1% of the total area of the study area. 86.1% of the post-seismic landslides fell in the high and very high susceptibility areas in 2011, whose area accounts for about 50.0% of the total area of the study area. Although over 70% of landslides fell in high and very high susceptibility areas, the high and very high susceptibility areas accounted for a large proportion.
Samia et al. (Citation2017b) made an effort to predict the follow-up landslides as a function of the geometry and location of a pre-phase landslide. A combination of geometric attributes and topographic attributes of landslides used in logistic regression increased the capability of predicting of occurrence of follow-up landslides (AUC = 0.64 ± 0.02). The accurate geometric attributes of pre-phase landslides are very vital in this method. With the deepening of the study, Samia et al. (Citation2020) tried to use a space–time clustering measure derived from Ripley’s space–time K function to quantify path dependency (legacy effect) among landslides. They compared the performance of the conventional, conventional plus path-dependent, and purely path-dependent landslide susceptibility models and found that the best-performing landslide susceptibility model was the conventional plus path-dependent model. The results demonstrated that adding landslide path dependency variables to such an improved conventional landslide susceptibility increased its performance (from an AUC value of 0.771 to an AUC value of 0.801). Although the latest method only uses point position information, the calculation process is still not simple enough.
In this study, the total area proportion of high and very high susceptibility areas in the improved model is far less than that of the conventional model, which is only half that of the conventional model. But, the proportion of post-seismic landslides that fell in the high and very high susceptibility areas of the conventional and improved models exceeds 80%. It means the improved landslide susceptibility model has better-predicting performance than the conventional one. The improved landslide susceptibility model is robust and has higher accuracy.
The improved model in this study quantifies the through simple buffer analysis, which is easier than the existing post-seismic landslide susceptibility methods. Furthermore, the improved landslide susceptibility model increases the number of landslide datasets that can be used. The multi-temporal post-seismic landslide dataset was obtained by automatic classification technology in this study. The improved landslide susceptibility model can use this post-seismic dataset without further processing. Therefore, the improved time-variant susceptibility model suits more post-seismic multi-temporal landslide datasets. It is easier to implement than the existing post-seismic time-variant landslide susceptibility methods, which can be applied to more earthquake cases with a larger research area globally.
4.3. The replicability of the improved landslide susceptibility model
The improved post-seismic landslide susceptibility model and influencing factors can be extended to landslide-prone areas with similar geological conditions, especially in areas where the expansion of pre-phase landslides is the main form of post-seismic landslide activity. To gain confidence in this method, conducting a spatio-temporal characteristics analysis of the multi-temporal landslide inventory in these areas needs to be questioned to find out the conditions for using this method. This study first analyzes the spatio-temporal characteristics of post-seismic landslides using Buffer analysis. One of its purposes is to analyze the main form of post-seismic landslide activity and determine whether the expansion of pre-phase landslides is the main form. Although the Buffer analysis method is simple, it is effective and can quickly analyze the main form of post-seismic landslide activity. Suppose the post-seismic landslides are not concentrated around the pre-phase landslide. In that case, there are more ‘new single landslides’, and the improved model of this study is not suitable for calculating post-seismic landslide susceptibility in this case.
However, the enormous energy released by an earthquake far exceeds the external forces after the earthquake. If an area did not slide during the earthquake, although it may have potential damage and may slide in the future due to external forces, this probability is not very high. In contrast, if the geological conditions of these areas are more similar to those of landslides that slid during earthquakes or nearby landslides, and they suffer greater damage (such as cracks) from the earthquake and the landslides, the probability of landslides is very high in these areas. For example, retrogressive landslides are abundant in earthquake-struck regions. Hence, the post-seismic landslides mainly come from pre-phase landslide expansions. In our other study, we used the 2008–2018 Wenchuan landslide dataset published by Fan et al. (Citation2019b) for statistical analysis, and the spatio-temporal characteristics of post-seismic landslides in Wenchuan are very similar to those in Jiuzhaigou (Guo Citation2022). The improved model also performs well in the study of Wenchuan post-seismic landslides susceptibility. Therefore, the improved model is also suitable for most post-seismic landslide studies.
4.4. Factors affecting the spatio-temporal characteristics of post-seismic landslide susceptibility
It has been found that seismogenic fault and PGA are the most important influencing factors for coseismic landslides (e.g. Keefer Citation2000; Qi et al. Citation2010; Zou et al. Citation2022). This study confirms this point again. Meanwhile, we noticed that most post-seismic landslides occurred around the pre-phase landslides. This phenomenon is because post-seismic landslides mainly come from the propagation of cracks and the movement of deposits (). The cracks and the deposits are significantly related to the pre-phase landslides and distributed around the pre-phase landslide. Therefore, the correlation between post-seismic landslide susceptibility and the distance to the pre-phase landslide is significant. The distance to the edge of the pre-phase landslide plays a vital role in the susceptibility analysis of the post-seismic landslide. Distance gradually becomes the important factor affecting the spatio-temporal characteristics of post-seismic landslide susceptibility and manifests as the high and very high landslide susceptibility areas shrink towards the concentration area of landslides.
Although post-seismic landslide is mainly triggered by precipitation, the definition of susceptibility does not include triggering factors. The fragmented geological environment is still the main influencing factor of the spatio-temporal characteristics of post-seismic landslide susceptibility. The precipitation of the Jiuzhaigou area in 2017, 2019, and 2020 was 786.2, 768.8, and 846 mm, respectively, and that of 2018 was abandoned due to not being updated in the annual hydrological report. After the earthquake, the precipitation in the Jiuzhaigou area was relatively stable. The precipitation in 2020 was the largest, but it was still within the normal precipitation range, and the fluctuation was not more than 5.5% compared with the annual average precipitation. The post-seismic landslide susceptibility of 2019–2020 remains on a decreasing trend. Therefore, although the post-seismic landslides in the Jiuzhaigou area are related to rainfall, the most crucial cause of the post-seismic landslides is still the fragmented geological environment caused by the earthquake. In this study, the distance factor is a proxy for the fragmented surface environment, so it is a crucial factor affecting the spatio-temporal characteristics of post-seismic landslide susceptibility.
In addition, We infer that the correlation between influencing factors, including distance, and the spatio-temporal characteristics of post-seismic landslide susceptibility will change over time. However, due to the limited monitoring time length available to the author, this study did not obtain the changing trend of correlation. It still needs to be further studied in different monitoring time lengths.
5. Conclusions
This study developed a multi-temporal post-seismic landslide dataset and established an improved post-seismic time-variant landslide susceptibility model. The spatio-temporal characteristics of post-seismic landslide susceptibility are generalized, and we draw the following conclusions:
The post-seismic landslides are mainly caused by the expansion of pre-phase landslides. The new post-seismic landslides are mainly distributed around the pre-phase landslides in the Jiuzhaigou area. The spatial distribution of new post-seismic landslides significantly became closer to the pre-phase landslide with time.
Distance is the critical factor in measuring the legacy effect of the pre-phase landslides on future landslides. It can improve the assessment accuracy of the post-seismic landslide susceptibility model and reasonably calculate the post-seismic landslide susceptibility.
The coseismic landslide susceptibility is mainly affected by earthquake-related factors such as seismogenic fault and PGA. The correlation between post-seismic landslides and earthquakes gradually became weaker with time.
In general, post-seismic landslide prevention should focus on the expansion of pre-phase landslides, especially the large landslides triggered by rainfall. Moreover, it should clean up the landslide deposits in time and reasonably dredge the debris flows to avoid the secondary geological disasters.
Disclosure statement
No potential conflict of interest was reported by the author(s).
Data availability statement
The data that support the findings of this study are available from the corresponding author, upon reasonable request.
Additional information
Funding
References
- Aditian, A., T. Kubota, and Y. Shinohara. 2018. “Comparison of GIS-Based Landslide Susceptibility Models Using Frequency Ratio, Logistic Regression, and Artificial Neural Network in a Tertiary Region of Ambon, Indonesia.” Geomorphology 318 (10.1): 101–111. https://doi.org/10.1016/j.geomorph.2018.06.006.
- Adolfo, Q. R. 2021a. “Landslides and Floods Zonation Using Geomorphological Analyses in a Dynamic Basin of Costa Rica.” Revista Cartográfica 102: 125–138. https://doi.org/10.35424/rcarto.i102.901.
- Adolfo, Q. R. 2021b. “Landslide Risk Index map at the Municipal Scale for Costa Rica.” International Journal of Disaster Risk Reduction 102144, https://doi.org/10.1016/j.ijdrr.2021.102144.
- Adolfo, Q. R., F. L. Berny, H. E. Karina, S. Markus, A. Juan, and C. Ballesteros. 2019. “Relationships Between Earthquakes, Hurricanes, and Landslides in Costa Rica.” Landslides 16 (8), https://doi.org/10.1007/s10346-019-01209-4.
- Aleotti, P. 2004. “A Warning System for Rainfall-Induced Shallow Failures.” Engineering Geology 73 (3-4): 247–265. https://doi.org/10.1016/j.enggeo.2004.01.007.
- Arroyo-Solórzano, M., A. Quesada-Román, and G. Barrantes-Castillo. 2022. “Seismic and Geomorphic Assessment for Coseismic Landslides Zonation in Tropical Volcanic Contexts.” Natural Hazards 114: 2811–2837. https://doi.org/10.1007/s11069-022-05492-8.
- ASF DAAC. n.d. “ALOS DEM.” Alaska Satellite Facility. Accessed May 2, 2022. http://search.asf.alaska.edu.
- Ayalew, L., and H. Yamagishi. 2005. “The Application of GIS-Based Logistic Regression for Landslide Susceptibility Mapping in the Kakuda-Yahiko Mountains, Central Japan.” Geomorphology 65: 15–31. https://doi.org/10.1016/j.geomorph.2004.06.010.
- Ba, Q. Q., Y. M. Chen, S. S. Deng, Q. J. Wu, J. X. Yang, and J. Y. Zhang. 2017. “An Improved Information Value Model Based on Gray Clustering for Landslide Susceptibility Mapping.” ISPRS International Journal of Geo-Information 6: 18. https://doi.org/10.3390/ijgi6010018.
- Budimir, M. E. A., P. M. Atkinson, and H. G. Lewis. 2015. “A Systematic Review of Landslide Probability Mapping Using Logistic Regression.” Landslides 12 (3): 419–436. https://doi.org/10.1007/s10346-014-0550-5.
- Caccavale, M., F. Matano, and M. Sacchi. 2017. “An Integrated Approach to Earthquake-Induced Landslide Hazard Zoning Based on Probabilistic Seismic Scenario for Phlegrean Islands (Ischia, Procida and Vivara), Italy.” Geomorphology 295: 235–259. https://doi.org/10.1016/j.geomorph.2017.07.010.
- Cao, J., Z. Zhang, C. Wang, J. Liu, and L. Zhang. 2019. “Susceptibility Assessment of Landslides Triggered by Earthquakes in the Western Sichuan Plateau.” Catena 175: 63–76. https://doi.org/10.1016/j.catena.2018.12.013.
- Carrión-Mero, P., N. Montalván-Burbano, F. Morante-Carballo, A. Quesada-Román, and B. Apolo-Masache. 2021. “Worldwide Research Trends in Landslide Science.” International Journal of Environmental Research and Public Health 18: 9445. https://doi.org/10.3390/ijerph18189445.
- Chen, M., Y. H. Cai, X. D. Wang, T. Yang, and W. Gan. 2019. “Evolution and Susceptibility Evaluation of Landslide Activity Intensity in Meizoseismal Area.” Bulletin of Soil and Water Conservation 39 (01): 239–243+325. https://doi.org/10.13961/j.cnki.stbctb.2019.01.038.
- Chen, M., C. Tang, J. Xiong, Q. Y. Shi, and Y. Tie. 2020. “The Long-Term Evolution of Landslide Activity Near the Epicentral Area of the 2008 Wenchuan Earthquake in China.” Geomorphology 367: 107317. https://doi.org/10.1016/j.geomorph.2020.107317.
- Conoscenti, C., S. Angileri, C. Cappadonia, E. Rotigliano, V. Agnesi, and M. Maerker. 2014. “Gully Erosion Susceptibility Assessment by Means of GIS-Based Logistic Regression: A Case of Sicily (Italy).” Geomorphology 204: 399–411. https://doi.org/10.1016/j.geomorph.2013.08.021.
- Cui, P., S. M. He, L. K. Yao, Z. Y. Wang, and X. Q. Chen. 2011. Formation Mechanism and Risk Control of Mountain Disaster Caused by the Wenchuan Earthquake. Beijing: Science Press.
- Dai, F. C., C. F. Lee, J. Li, and Z. W. Xu. 2001. “Assessment of Landslide Susceptibility on the Natural Terrain of Lantau Island, Hong Kong.” Environmental Geology 40: 381–391. https://doi.org/10.1007/s002540000163.
- Dai, L. X., Q. Xu, X. M. Fan, M. Chang, Q. Yang, F. Yang, and J. Ren. 2017. “A Preliminary Study on Spatial Distribution Patterns of Landslides Triggered by Jiuzhaigou Earthquake in Sichuan on 8 August, 2017, and Their Susceptibility Assessment.” Journal of Engineering Geology 25 (04): 1151–1164. https://doi.org/10.13544/j.cnki.jeg.2017.04.030.
- Deng, G. P. 2011. “Study of Tourism Geosciences Landscape Formation and Protection of Jiuzhaigou World Natural Heritage Site.” PhD Diss. Chengdu University of Technology.
- Dong, X. J., Q. Xu, J. X. She, W. L. Li, F. Liu, and X. X. Zou. 2020. “Preliminary Study on Interpretation of Geological Hazards in Jiuzhaigou Based on Multi-Source Remote Sensing Data.” Geomatics and Information Science of Wuhan University 45 (3): 432–441. https://doi.org/10.13203/j.whu-gis20130532.
- Fan, X. M., G. Scaringi, G. Domènech, F. Yang, X. J. Guo, L. X. Dai, C. Y. He, Q. Xu, and R. Q. Huang. 2019b. “Two Multi-Temporal Datasets That Track the Enhanced Landsliding After the 2008 Wenchuan Earthquake.” Earth System Science Data 11 (1): 35–55. https://doi.org/10.5194/essd-11-35-2019.
- Fan, X. M., G. Scaringi, O. Korup, A. J. West, C. J. V. Westen, H. Tanyas, N. Hovius, et al. 2019a. “Earthquake-Induced Chains of Geologic Hazards: Patterns, Mechanisms, and Impacts.” Reviews Of Geophysics 57: 421–503. https://doi.org/10.1029/2018RG000626.
- Fan, X. M., G. Scaringi, Q. Xu, W. W. Zhan, L. X. Dai, Y. S. Li, X. J. Pei, Q. Yang, and R. Q. Huang. 2018. “Coseismic Landslides Triggered by the 8th August 2017 Ms 7.0 Jiuzhaigou Earthquake (Sichuan, China): Factors Controlling Their Spatial Distribution and Implications for the Seismogenic Blind Fault Identification.” Landslides 15 (5): 967–983. https://doi.org/10.1007/s10346-018-0960-x.
- Fan, X. M., A. P. Yunus, G. Scaringi, F. Catani, S. S. Subramanian, Q. Xu, and R. Q. Huang. 2020. “Rapidly Evolving Controls of Landslides After a Strong Earthquake and Implications for Hazard Assessments.” Geophysical Research Letters 2020), https://doi.org/10.1029/2020GL090509.
- Fawcett, T. 2006. “An Introduction to ROC Analysis.” Pattern Recognition Letters 27: 861–874. https://doi.org/10.1016/j.patrec.2005.10.010.
- García-Rodríguez, M. J., J. A. Malpica, B. Benito, and M. Díaz. 2008. “Susceptibility Assessment of Earthquake-Triggered Landslides in El Salvador Using Logistic Regression.” Geomorphology 95 (3): 172–191. https://doi.org/10.1016/j.geomorph.2007.06.001.
- Geospatial Data Cloud. 2019. “Vector data of rivers and roads in China.” Geospatial Data Cloud. Accessed May 2, 2022. http://www.gscloud.cn/sources.
- Ghosh, S., C. J. van Westen, E. J. M. Carranza, V. G. Jetten, M. Cardinali, M. Rossi, and F. Guzzetti. 2012. “Generating Event-Based Landslide Maps in a Data-Scarce Himalayan Environment for Estimating Temporal and Magnitude Probabilities.” Engineering Geology 128: 49–62. https://doi.org/10.1016/j.enggeo.2011.03.016.
- Guo, X. Y. 2022. “Spatio-temporal Characteristics and Susceptibility of Post-Seismic Landslides Along the High Seismic Intensity Area in the Eastern Tibetan Plateau.” PhD Diss., University of Chinese Academy of Sciences (Aerospace Information Research Institute, Chinese Academy of Sciences). https://doi.org/10.44231/d.cnki.gktxc.2022.000099.
- Guo, X. Y., B. H. Fu, J. Du, P. L. Shi, Q. Y. Chen, and W. Y. Zhang. 2021c. “Applicability of Susceptibility Model for Rock and Loess Earthquake Landslides in the Eastern Tibetan Plateau.” Remote Sensing 13: 2546. https://doi.org/10.3390/rs13132546.
- Guo, X. Y., B. H. Fu, J. Du, P. L. Shi, J. X. Li, Z. Li, J. X. Du, Q. Y. Chen, and H. Fu. 2021b. “Monitoring and Assessment for the Susceptibility of Landslide Changes After the 2017 Ms 7.0 Jiuzhaigou Earthquake Using the Remote Sensing Technology.” Frontiers in Earth Science 9: 633117. https://doi.org/10.3389/feart.2021.633117.
- Guo, X. Y., Q. Guo, and Z. K. Feng. 2021a. “Detecting the Vegetation Change Related to the Creep of 2018 Baige Landslide in Jinsha River, SE Tibet Using SPOT Data.” Frontiers in Earth Science 9: 706998. https://doi.org/10.3389/feart.2021.706998.
- Guo, J. Q., D. Peng, J. Cao, and J. Y. Yang. 2000. “Geomorphology and Quaternary Geology in Jiuzhai Valley.” Acta Geologica Sichuan 2000 (03): 183–192.
- Guzzetti, E., A. Carrara, M. Cardinali, and P. Reichenbach. 1999. “Landslide Hazard Evaluation: A Review of Current Techniques and Their Application in a Multi-Scale Study, Central Italy.” Geomorphology 31: 181–216. https://doi.org/10.1016/S0169-555X(99)00078-1.
- Huang, R. Q., and W. L. Li. 2008. “Research on Development and Distribution Rules of Geohazards Induced by Wenchuan Earthquake on 12 May 2008.” Chinese Journal of Rock Mechanics and Engineering 27 (12): 2585–2592. https://doi.org/10.3321/j.issn:1000-6915.2008.12.028.
- Huang, R. Q., and W. L. Li. 2014. “Post-earthquake Landsliding and Long-Term Impacts in the Wenchuan Earthquake Area, China.” Engineering Geology 182 (2014): 111–120. https://doi.org/10.1016/j.enggeo.2014.07.008.
- IBM Corp. 2017. IBM SPSS Statistics for Windows, Version 25.0. Armonk, NY: IBM Corp.
- Jones, J. N., S. J. Boulton, G. L. Bennett, M. Stokes, and M. R. Z. Whitworth. 2021. “Temporal Variations in Landslide Distributions Following Extreme Events: Implications for Landslide Susceptibility Modeling.” Journal of Geophysical Research: Earth Surface 126: e2021JF006067. https://doi.org/10.1029/2021JF006067.
- Keefer, D. K. 2000. “Statistical Analysis of an Earthquake-Induced Landslide Distribution - the 1989 Loma Prieta, California Event.” Engineering Geology 58 (3): 231–249. https://doi.org/10.1016/S0013-7952(00)00037-5.
- Kritikos, T., T. R. Robinson, and T. R. H. Davies. 2015. “Regional Coseismic Landslide Hazard Assessment Without Historical Landslide Inventories: A new Approach.” Journal of Geophysical Research: Earth Surface 120 (4): 711–729. https://doi.org/10.1002/2014JF003224.
- Li, C. Y., X. C. Wang, C. Z. He, X. Wu, Z. Y. Kong, and X. L. Li. 2017. “China National Digital Geological Map (Public Version at 1:200000 Scale) Spatial Database.” (dataset). Accessed May 2, 2022. http://dcc.ngac.org.cn/geologicalData.
- Li, C. R., M. Wang, and K. Liu. 2018. “A Decadal Evolution of Landslides and Debris Flows After the Wenchuan Earthquake.” Geomorphology 323: 1–12. https://doi.org/10.1016/j.geomorph.2018.09.010.
- Lin, A. M., Z. K. Ren, D. Jia, and X. J. Wu. 2009. “Co-seismic Thrusting Rupture and Slip Distribution Produced by the 2008 Mw 7.9 Wenchuan Earthquake, China.” Tectonophysics 471 (3-4): 203–215. https://doi.org/10.1016/j.tecto.2009.02.014.
- Lin, Q. G., S. Steger, M. Pittore, J. H. Zhang, L. B. Wang, T. Jiang, and Y. Wang. 2022. “Evaluation of Potential Changes in Landslide Susceptibility and Landslide Occurrence Frequency in China Under Climate Change.” Science of the Total Environment 850: 158049. https://doi.org/10.1016/j.scitotenv.2022.158049.
- Lombardo, L., and P. M. Mai. 2018. “Presenting Logistic Regression-Based Landslide Susceptibility Results.” Engineering Geology 244: 14–24. https://doi.org/10.1016/j.enggeo.2018.07.019.
- Lombardo, L., and H. Tanyas. 2021. “From Scenario-Based Seismic Hazard to Scenario-Based Landslide Hazard: Fast-Forwarding to the Future via Statistical Simulations.” Stochastic Environmental Research and Risk Assessment 36: 2229–2242. https://doi.org/10.1007/s00477-021-02020-1.
- Mathew, J., V. K. Jha, and G. S. Rawat. 2009. “Landslide Susceptibility Zonation Mapping and its Validation in Part of Garhwal Lesser Himalaya, India, Using Binary Logistic Regression Analysis and Receiver Operating Characteristic Curve Method.” Landslides 6: 17–26. https://doi.org/10.1007/s10346-008-0138-z.
- Pasang, S., and P. Kubicek. 2018. “Information Value Model Based Landslide Susceptibility Mapping at Phuentsholing, Bhutan.” In Proceedings of the 21st AGILE Conference, Lund, Sweden 12-15.
- Pasang, S., and P. Kubicek. 2020. “Landslide Susceptibility Mapping Using Statistical Methods Along the Asian Highway, Bhutan.” Geosciences (Switzerland) 10 (11): 430. https://doi.org/10.3390/geosciences10110430.
- Qi, S. W., Q. Xu, H. X. Lan, B. Zhang, and J. Y. Liu. 2010. “Spatial Distribution Analysis of Landslides Triggered by 2008.5.12 Wenchuan Earthquake, China.” Engineering Geology 116 (1-2): 95–108. https://doi.org/10.1016/j.enggeo.2010.07.011.
- Qi, S. W., Q. Xu, B. Zhang, Y. D. Zhou, H. X. Lan, and L. H. Li. 2011. “Source Characteristics of Long Runout Rock Avalanches Triggered by the 2008 Wenchuan Earthquake, China.” Journal of Asian Earth Sciences 40: 896–906. https://doi.org/10.1016/j.jseaes.2010.05.010.
- Reichenbach, P., M. Rossi, B. D. Malamud, M. Mihir, and F. Guzzetti. 2018. “A Review of Statistically-Based Landslide Susceptibility Models.” Earth-science Reviews 180: 60–91. https://doi.org/10.1016/j.earscirev.2018.03.001.
- Roberts, S., J. Jones, and S. Boulton. 2021. “Characteristics of Landslide Path Dependency Revealed Through Multiple Resolution Landslide Inventories in the Nepal Himalaya.” Geomorphology, https://doi.org/10.1016/j.geomorph.2021.107868.
- Samia, J., A. Temme, A. Bregt, J. Wallinga, F. Guzzetti, and F. Ardizzone. 2020. “Dynamic Path-Dependent Landslide Susceptibility Modelling.” Natural Hazards and Earth System Sciences 20: 271–285. https://doi.org/10.5194/nhess-20-271-2020.
- Samia, J., A. Temme, A. Bregt, J. Wallinga, F. Guzzetti, F. Ardizzone, and M. Rossi. 2017a. “Do Landslides Follow Landslides? Insights in Path Dependency from a Multi-Temporal Landslide Inventory.” Landslides 14 (2): 547–558. https://doi.org/10.1007/s10346-016-0739-x.
- Samia, J., A. Temme, A. Bregt, J. Wallinga, F. Guzzetti, F. Ardizzone, and M. Rossi. 2017b. “Characterization and Quantification of Path Dependency in Landslide Susceptibility.” Geomorphology 292: 16–24. https://doi.org/10.1016/j.geomorph.2017.04.039.
- Samia, J., A. Temme, A. K. Bregt, J. Wallinga, J. Stuiver, F. Guzzetti, F. Ardizzone, and M. Rossi. 2018. “Implementing Landslide Path Dependency in Landslide Susceptibility Modelling.” Landslides 15 (11): 2129–2144. https://doi.org/10.1007/s10346-018-1024-y.
- Serey, A., L. Piñero-Feliciangeli, S. A. Sepúlveda, P. Fernando, N. P. David, and M. William. 2019. “Landslides Induced by the 2010 Chile Megathrust Earthquake: A Comprehensive Inventory and Correlations with Geological and Seismic Factors.” Landslides 16: 1153–1165. https://doi.org/10.1007/s10346-019-01150-6.
- Shao, X. Y., S. Y. Ma, C. Xu, P. F. Zhang, B. Y. Wen, Y. Y. Tian, Q. Zhou, and Y. Cui. 2018. “Planet Image-Based Inventorying and Machine Learning-Based Susceptibility Mapping for the Landslides Triggered by the 2018 MW6.6 Tomakomai, Japan Earthquake.” Remote Sensing 11: 978. https://doi.org/10.3390/rs11080978.
- Steger, S., M. Moreno, A. Crespi, P. J. Zellner, S. L. Gariano, M. T. Brunetti, M. Melillo, et al. 2022. “Deciphering Seasonal Effects of Triggering and Preparatory Precipitation for Improved Shallow Landslide Prediction Using Generalized Additive Mixed Models.” Natural Hazards and Earth System Sciences, 1483–1506. https://doi.org/10.5194/nhess-23-1483-2023.
- Swets, J. A. 1988. “Measuring the Accuracy of Diagnostic Systems.” Science 240: 1285–1293. https://doi.org/10.1126/science.3287615.
- Talaei, R. 2014. “Landslide Susceptibility Zonation Mapping Using Logistic Regression and its Validation in Hashtchin Region, Northwest of Iran.” Journal of the Geological Society of India 84: 68–86. https://doi.org/10.1007/s12594-014-0111-5.
- Tang, C. 2010. “Activity Tendency Prediction of Rainfall Induced Landslides and Debris Flows in the Wenchuan Earthquake Areas.” Journal of Mountain Science 2010 (03): 87–95. https://doi.org/10.16089/j.cnki.1008-2786.2010.03.009.
- Tian, Q., B. Zhang, J. F. Guo, H. Z. Liu, Z. L. Chang, Y. J. Li, and F. M. Huang. 2020. “Landslide Susceptibility Assessment Based on the Coupling Model of Information Value and Logistic Regression.” Science Technology and Engineering 20: 8460–8468.
- USGS Earthquake Hazards Program. 2017. “Shakemap.” United States Geological Survey. Accessed May 2, 2022. http://earthquake.usgshakemap.gov
- Vapnik, V. N. 1998. “Statistical Learning Theory.” In Encyclopedia of the Sciences of Learning 41 (4): 3185.
- Wang, G. X. 2004. Landslide Science and Landslide Control Technology. Beijing: China Railway Press.
- Wang, N., W. Cheng, M. Marconcini, F. Bachofer, C. Liu, J. Xiong, and L. Lombardo. 2022. “Space-time Susceptibility Modeling of Hydro-Morphological Processes at the Chinese National Scale.” Engineering Geology 301, https://doi.org/10.1016/j.enggeo.2022.106586.
- Wang, M., W. T. Yang, P. J. Shi, C. Xu, and L. Y. Liu. 2014. “Diagnosis of Vegetation Recovery in Mountainous Regions After the Wenchuan Earthquake.” IEEE Journal of Selected Topics in Applied Earth Observations and Remote Sensing 7 (7): 3029–3037. https://doi.org/10.1109/JSTARS.2014.2327794.
- Wu, C. H., P. Cui, Y. S. Li, A. A. Irasema, C. Huang, and S. J. Yi. 2018. “Seismogenic Fault and Topography Control on the Spatial Patterns of Landslides Triggered by the 2017 Jiuzhaigou Earthquake.” Journal of Mountain Science 15 (4): 793–807. https://doi.org/10.1007/s11629-017-4761-9.
- Wu, Y. M., H. X. Lan, X. Gao, L. P. Li, and Z. H. Yang. 2015. “A Simplified Physically Based Coupled Rainfall Threshold Model for Triggering Landslides.” Engineering Geology 195 (195): 63–69. https://doi.org/10.1016/j.enggeo.2015.05.022.
- Xu, C., X. W. Xu, L. L. Shen, Q. Yao, X. B. Tan, W. J. Kang, S. Y. Ma, et al. 2016. “Optimized Volume Models of Earthquake-Triggered Landslides.” Scientific Reports 6: 29797. https://doi.org/10.1038/srep29797.
- Yegian, M. K., E. A. Marciano, and V. G. Ghahraman. 1991. “Earthquake-induced Permanent Deformations: Probabilistic Approach.” Journal of Geotechnical Engineering 117 (1): 35–50. https://doi.org/10.1061/(ASCE)0733-9410(1991).
- Yin, K. L. 2013. Risk Analysis of Landslide Disaster. Beijing: Science Press.
- Zang, M. D., S. W. Qi, Y. Zou, Z. P. Sheng, and B. S. Zamora. 2020. “An Improved Method of Newmark Analysis for Mapping Hazards of Coseismic Landslides.” Natural Hazards and Earth System Sciences 20 (3): 713–726. https://doi.org/10.5194/nhess-20-713-2020.
- Zhang, Q. K., S. X. Ling, X. N. Li, C. W. Sun, J. X. Xu, and T. Huang. 2020. “Comparison of Landslide Susceptibility Mapping Rapid Assessment Models in Jiuzhaigou County, Sichuan Province, China.” Chinese Journal of Rock Mechanics and Engineering 08: 1595–1610. https://doi.org/10.13722/j.cnki.jrme.2020.0029.
- Zou, Y., S. W. Qi, S. F. Guo, B. W. Zheng, Z. F. Zhan, N. W. He, X. L. Huang, X. K. Hou, and H. Y. Liu. 2022. “Factors Controlling the Spatial Distribution of Coseismic Landslides Triggered by the Mw 6.1 Ludian Earthquake in China.” Engineering Geology 2022, https://doi.org/10.1016/j.enggeo.2021.106477.