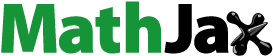
ABSTRACT
Urban population size dynamic adjustment has been widely studied for a long time; however, the dynamic adjustment at the urban hierarchy level remains unknown. This study utilises China’s urban population data to investigate the dynamic adjustment. Specifically, we utilise methods of the Gini coefficient, linear regression, and coefficients of Spearman, and Moran’s I to explore the dynamic adjustment tendency, adjustment rate and correlation of urban population size distribution of different urban hierarchies and groups of different urban hierarchies. We find that the urban population size spatial distribution is gradually even within the same urban hierarchy. The adjustment rate to be even of Megacity II is the highest, and Megacity I is the bottom. We also find the population size spatial distribution of the whole urban system is gradually uneven. We explore the dynamic process by sorting urban hierarchies into six groups. Furthermore, we find there is a complex correlation between different urban hierarchies and global spatial correlation among China cities. These results are valuable as references for Chinese policy-makers and practitioners and will enable them to adopt sustainable land use and urban population management measures. The study outcomes will also benefit future studies on spatial population size distribution in international communities.
1. Introduction
In recent years, China’s urban population is rapidly increased accompanied by individuals migrating from rural areas. Its urban population has increased from 1,307.56 million to 9207.10 million from 2005–2022, and nearly increased by 4.65 million each year. China has experienced unprecedented speed and scale of urbanisation. By the end of 2022, China’s urbanisation rate had reached 65.22% (National Bureau of Statistics of the People’s Republic of China Citation2023).
However, there are also some problems in the process of fast population urbanisation in China, such as urban construction land use expansion (Wang and Zhang Citation2022; Zhang et al. Citation2018), uneven spatial distribution of urban population (C. Jiang, Li, and Liu Citation2022; Nam Citation2017), uncoordinated development of regional economic (Y. Li and Liu Citation2018; Zhang Citation2023). Furthermore, these problems lead to a decreasing urban population density (C. Jiang, Li, and Liu Citation2022; Xu et al. Citation2019), urban disease of megacities (Yi, Qi, and Chen Citation2022), poor population agglomeration and less economic development of small cities in China (Jiang et al. Citation2020; Meng and Long Citation2022).
In recognising these problems brought about by urban population spatial distribution, the China government, at both national and city levels, has been devoting efforts to solve these problems by implementing various policies and regulations. For example, Several policies concerning the implementation of the large-scale development of the Western region were implemented in 2000 (China State Council Citation2000). They further promoted the reform of the household registration system in 2014 (China State Council Citation2014). The 13th Five-Year Plan for the rise of the Central Region in 2016 (National Development and Reform Commission of China Citation2016), The 14th Five-Year Plan for National Economic and Social Development of the People's Republic of China and the Outline of the long-range goals for 2035 in 2021 (China State Council Citation2021). These policies and regulations aim to promote the economic development of central and western regions by investing more capital to attract individuals migrating to these regions and, at the same time, regulate the population size of the megacities. The China government hopes to utilise these policies and regulations to promote an even degree of urban population spatial distribution.
However, whilst it is important to introduce coordinated economic development of cities and regulate the population size of megacities, it is more important to ensure that these efforts have achieved ideal results. Without this understanding, it is difficult to evaluate the efficiency of these policies or regulations. Therefore, it is critical to examine the dynamic adjustment of urban population spatial distribution, which is driven by urbanisation and urban system self-organization.
Since many years ago, the Chinese government has managed its cities by urban hierarchies to better promote urban economic development and regulate urban population size. The China cities are sorted into seven urban hierarchies according to urban population size (as shown in ) (China State Council Citation2014).
Table 1. Classification of urban hierarchies according to population size.
Also, many policies on regulating urban population spatial distribution were implemented according to urban hierarchies, such as the National New-Type Urbanization Plan (2014–2020) (China State Council Citation2014), The 14th Five-Year Plan for National Economic and Social Development of the People's Republic of China and the Outline of the long-range goals for 2035 (China State Council Citation2021), etc.
Therefore, it is necessary to analyse the dynamic adjustment of urban population size spatial distribution by urban hierarchies. On the one hand, the research is valuable for Chinese policy-makers and practitioners and will enable them to adopt sustainable land use and urban population management measures; on the other hand, it provides a new perspective for future studies on spatial population distribution in international communities.
2. Literature review
Mapping, as the aggregated number of methods, is one of the most widely available to study China’s urban population spatial distribution. Gaughan et al. (Citation2016) quantified the population spatial distribution patterns of China by utilising census data for 1990, 2000, and 2010 in an ensemble prediction model, and finally, they obtained fine-scale (∼100 m) patterns of population distribution for China mainland. Li and Zhou (Citation2018) argued that utilising census data to map population distribution could not satisfy the demand for urban study due to shortages of worse reflecting the spatial heterogeneity of population within spatial units, and temporally incompatible with the frequent changes of spatial unit boundary. Then, they utilised urban nighttime light index as a weight to disaggregate country-level urban population, and finally, obtained a dataset of gridded urban population with 1 km resolution in China from 2000 to 2010. However, the estimation accuracy of this method was generally not high, and the overall accuracy was about 60% (X. Li and Zhou Citation2018), which limited its wide application. Xu et al. (Citation2021) proposed a more accurate method by utilising Tencent social user and remote sensing data to map China’s urban population spatial distribution, which increased the accuracy to reach 90.4%. These studies indicate that methods of mapping urban population spatial distribution become more and more accurate with the development of geospatial technology, and this provides valuable information to analyse the urban population spatial distribution of China and other countries. However, these studies pay little attention to the dynamic adjustment of urban population spatial distribution, which provides little help to analyse the effects of management policies on adjusting urban population spatial distribution.
Zipf’s rule is another widely used method to study urban population size spatial distribution. This method was first proposed by Zipf in 1949. By using population data for Indian communities in 1911 and 1931 and for 100 metropolitan areas in the United States in 1940, he fitted each point with logarithms of population size on the vertical axis and logarithms of population size ordinals on the horizontal axis, and the results showed that the slope of the fitted line was approximately −1. This finding was named ‘Zipf’s law’ by following scholars, and it has been widely used in many studies to analyse urban population spatial distribution (Hackmann and Klarl Citation2020; Reia et al. Citation2022; Verbavatz and Barthelemy Citation2020). This method is also used to investigate China’s urban population spatial distribution. Ye and Xie (Citation2012) investigated China’s urban system population spatial distribution dynamic adjustment from 1960 to 2000 by Zipf’s method. S. Li (Citation2016) studied China’s urban population spatial distribution and its dynamic by using data from 1984, 1988, 1992, 1996, 2000 and 2004. More recently research on whether China’s urban population spatial distribution holds for Zipf’s law was proposed by Sun et al. (Citation2021), they observed China’s urban population spatial distribution by using multi-source population data from 2013 to 2018 of China’s 337 cities from Zipf’s method to establish the model. Although the method proposed by Zipf and developed by the following scholars well reflects urban population spatial distribution and its dynamic, some limitations exist including masking spatial restructuring, ignoring regional inequality and lacking in-depth research through classifying cities into different urban hierarchies according to population size (Li Citation2016; Sun et al. Citation2021; Ye and Xie Citation2012).
Although many studies have focused on China’s urban population spatial distribution by using Zipf’s rule and mapping. There is hardly any study investigating this topic from the urban hierarchy. As cites in China are always sorted into different urban hierarchies for better implementation of classified management, and this is also a common phenomenon worldwide. Therefore, we study the dynamic adjustment of urban population spatial distribution by sorting China cities into different urban hierarchies. The research findings are expected to provide the Chinese’s government with references for policy-making to further improve the even level of urban population spatial distribution and decrease the unbalance of urban economic development in the process of urbanisation. It also provides a new perspective for scholars in other countries to research urban population spatial distribution at the urban hierarchy level.
The aim of this research is to analyse the dynamic adjustment of urban population spatial distribution at the urban hierarchy level, and it contains three aspects. The first aspect is to investigate the dynamic adjustment tendency of population size spatial distribution of different urban hierarchies and groups of different urban hierarchies. The second aspect is to analyse the adjustment rates of different urban hierarchies and groups of different urban hierarchies, which further indicates the results of policy implementation. The third aspect is to explore whether there is correlation between different urban hierarchies and global spatial correlation among cities in the process of dynamic adjustment of urban population spatial distribution. All cities constitute an urban system and individuals migrate among cities, which may lead to existing correlation-ship between different urban hierarchies and among cities in the process of dynamic adjustment. The Gini coefficient is selected to analyse the first aspect. The linear regression method is utilised to study the second aspect. Spearman correlation coefficient and Moran’s I are selected to discuss the third aspect.
The remainder of this paper is organised as follows. Section 3 introduces the research methods adopted and the data presented in this paper. Section 4 research results are displayed. Section 5 discusses the results. Finally, conclusions are presented in section 6.
3. Methodology and data
3.1. Methodology
3.1.1. Gini coefficient
The Gini coefficient was first proposed by the Italian statistician and sociologist Gini, which is an internationally accepted and common indicator used to evaluate income disparity. Several scholars also use it to evaluate other indicator inequality, such as technology inequality (Qu, Li, and Guy-Roland N’Drin Citation2023), inequalities of urban green space area and ecosystem service (Chen et al. Citation2022), inequality of urban household energy consumption (M. Zhang et al. Citation2023). Its values range from 0 to 1, with 0 indicating perfect equality and 1 indicating extreme inequality. In this study, the Gini coefficient (G) is utilised to analyse the dynamic adjustment tendency of the evenness of urban population spatial distribution of different urban hierarchies and groups of urban hierarchies.
The calculation process is as follows. Consider n (i = 1, 2, … n) cities in an urban hierarchy (or an urban group), and the population size of the n cities is sorted in ascending order, denoted by ,
, …
. The Gini coefficient is then calculated as follows:
(1)
(1)
3.1.2. Linear regression
The linear regression method is utilised to analyse the dynamic adjustment rate of the Gini coefficient of each urban hierarchy and a group of urban hierarchies, which indicates the uneven dynamic adjustment rate. The linear regression is expressed as follows:
(2)
(2) where
is the Gini coefficient of the t year of an urban hierarchy,
is the time,
and
are equation parameters of formula (2). The sign of
and its absolute value are used to judge the direction and rate of the Gini coefficient dynamic adjustment.
3.1.3. Spearman’s rank correlation coefficient
The Spearman’s rank correlation coefficient was first proposed by the British statistician and sociologist Spearman, which is an internationally accepted and commonly used indicator for evaluating the correlation between two variables. Several scholars have utilised it to discuss monotonic relationships (Bashir et al. Citation2020; Xiong et al. Citation2020; Yang et al. Citation2022). Its values range from −1, indicating a perfectly negative correlation, to 1 for a perfectly positive correlation. Spearman’s rank correlation coefficient is utilised to evaluate the correlation of the Gini coefficient between different urban hierarchies. The Spearman’s rank correlation coefficient is then calculated as follows:
(3)
(3) where
is the Spearman correlation coefficient and
is the difference in the rank between urban hierarchies e and q for the jth year.
3.1.4. Moran’s I index
Moran’s I was first developed by the Australia statistician Patrick Alfred Pierce Moran, which is used to measure spatial autocorrelation (Moran Citation1950; Nowell et al. Citation2021; You, Sun, and Liu Citation2022). It reflects spatial correlation in multi-dimensional and multi-directional. Its values range from −1, indicating a perfectly negative correlation, to 1 for a perfectly positive correlation. Moran’s I is utilised to evaluate the spatial correlation of the population size spatial distribution of China cities. The Moran’s I is then calculated as follows:
(4)
(4) where n is the number of cities,
and
are the population size values of cities i and j, and
is the spatial weight matrix.
This paper analyses the correlation characteristics of population size spatial distribution of China cities by using the economic distance matrix, for urban population size distribution is closely related to individuals’ location choice, while urban economic level affects the choice. The economic distance matrix is calculated as follows:
(5)
(5) where
is the average value of the gross domestic product (GDP) per permanent inhabitant of the using years of the first city,
is the average value of the gross domestic product (GDP) per permanent inhabitant of the using years of the second city, and by analogy, then
is the average value of the gross domestic product (GDP) per permanent inhabitant of the using years of the nth city. In this formula, the order of cities is random.
3.2. Data
There are in 338 prefecture-level or higher cities in China. As the relevant data of some cities, only 231 cities were collected as the sample cities. As of 2019, the city of Laiwu was merged into Jinan, and we combined the data for the two cities. At last, 230 cities are the sample. Permanent residents of the municipal district are selected as the indicator to explore the dynamic adjustment tendency of urban population spatial distribution of different urban hierarchies. This indicator includes the floating population, which is different from the registered population. Indicators of permanent residents can objectively reflect urban population spatial distribution. Although some residents may be not registered population, they live in the city at most times of the year. Permanent residents are not included in the statistical data. We use the statistics of the gross domestic product of the permanent residents and the gross domestic product per permanent inhabitant of a municipal district to calculate the present study’s indicator of permanent residents, which is equal to the quotient of the two stated statistical values. The data pertaining to the two indicators from 2005 to 2020 are available from the China City Statical Yearbook released from 2006 to 2021 (CNBS, 2006–2021) and data from local city statistics bureaus.
After obtaining the data of permanent residents of the municipal district, the 230 cities are sorted into 7 urban hierarchies by using the data of 2020 according to the Notice on Adjusting the Standards for Dividing the Size of Cities issued by The China State Council in 2014 (), including 10 type Ⅰ megacities (Shanghai, Beijing, Chongqing, etc.), 15 type Ⅱ megacities (Nanjing, Qingdao, Zhengzhou, etc.), 20 type Ⅰ big cities (Changchun, NanTong, Baoding, etc.), 98 type Ⅱ big cities (Zunyi, Yangzhou, Mianyang, etc.), 68 medium cities (Ankang, Baoshan, Zhoushan, etc.), 18 type Ⅰ small cities (Jiuquan, Sanming, Wuzhong, etc.), and 1 type Ⅱ small cities (Heihe). For there is only 1 type Ⅱ small city, we combined it into type Ⅰ small cities, named the new urban hierarchy as Small city. Therefore, we obtain 6 urban hierarchies, and then, there are 19 cities in the urban hierarchy of Small city as shown in .
4. Research results
4.1. The Gini coefficients of urban population size
reports the Gini coefficients of six urban hierarchies in China, all of which are greater than zero. This indicates that the urban population distribution of each urban hierarchy is uneven. The columns of the table reflect the population size Gini coefficients of each urban hierarchy from 2005 to 2020. For example, the population size Gini coefficients of Megacity Ⅰ from 2005 to 2008 are respectively 0.2505, 0.2721 and 0.2308. The lines of the table show the population size Gini coefficients of each year of the six urban hierarchies. For example, in 2005, the population size Gini coefficients of six urban hierarchies were respectively 0.2505, 0.2408, 0.1629, 0.2259, 0.991 and 0.1680.
Table 2. The population size Gini coefficient of China’s six urban hierarchies.
The Gini coefficients reflect the even degree of population size spatial distribution of each urban hierarchy. The values of the Gini coefficients of 2020 are all smaller than in 2005, which indicates the urban population spatial distribution of each urban hierarchy is more even in 2020 than in 2005. The Gini coefficient order of the six urban hierarchies in 2005 is Big city Ⅰ < Small city < Medium city < Big city Ⅱ < Megacity Ⅱ < Megacity Ⅰ, indicating that the even degree of urban population spatial distribution of the six urban hierarchies is Megacity Ⅰ < Megacity Ⅱ< Big city Ⅱ < Medium city < Small city < Big city Ⅰ. Overall, there are fluctuations in the Gini coefficient values of all the six urban hierarchies. For example, the Gini coefficient values of Megacity Ⅰ from 2009 to 2011 are 0.2347, 0.2714 and 0.2481, which shows a fluctuation. This indicates that there are fluctuations in the process of dynamic adjustment of the population size distribution of all the six urban hierarchies. This also indicates that it cannot judge the dynamic adjustment trends of population size distribution only by the Gini coefficient values of each urban hierarchy from 2005 to 2020.
According to , the linear plot at 95% confidence interval of the population size Gini coefficients of the six urban hierarchies is drawn, as shown in .
illustrates the scatter points of the Gini coefficients of population size of each urban hierarchy. Although there are fluctuations, all the lines fitted by the scatter points are inclined to the lower right. This indicates that in the process of dynamic adjustment, the overall tendency of population size of each urban hierarchy is gradually even.
For further examining the dynamic adjustment of urban population spatial distribution among different urban hierarchies, the Gini coefficients from Megacity Ⅰ (M Ⅰ) to the Whole-Urban-System (W-U-S) are calculated by adding one urban hierarchy per step, as shown in .
Table 3. The population size Gini coefficient of China’s six urban groups.
As reported in , the lines reflect the dynamic adjustment of Gini coefficients from Megacity Ⅰ (M Ⅰ) to the Whole-Urban-System (W-U-S). The lines of indicate that the Gini coefficient values are gradually increasing with every adding urban hierarchy into the former urban group. For example, in 2005 (the second line), the population size Gini coefficients from Megacity Ⅰ to the Whole-Urban-System are respectively 0.2505, 0.3641, 0.3919, 0.4489, 0.4749 and 0.4911, indicating an uneven dynamic adjustment tendency of urban population distribution. This is a natural result due to the gap existing of the population size of different urban hierarchies, as adding one urban hierarchy per step means increasing uneven spatial distribution of population size.
The columns of reflect the population size Gini coefficients from Megacity Ⅰ to the Whole-Urban-System between 2005 and 2020. The results show that the Gini coefficient values of Megacity Ⅰ (M Ⅰ), group of Megacity Ⅰ and Megacity Ⅱ (M Ⅰ Ⅱ), and group of Megacity Ⅰ, Megacity Ⅱ, and Big city Ⅰ (M Ⅰ Ⅱ–B Ⅰ) of 2020 are all smaller than 2005, while the Gini coefficient values of rest groups of 2020 are all larger than 2005. This indicates that the urban population spatial distribution of M Ⅰ, M Ⅰ Ⅱ and M Ⅰ Ⅱ–B Ⅰ is more even in 2020 than in 2005, while the urban population spatial distribution of rest groups is more uneven in 2020 than in 2005. Overall, there are fluctuations in the Gini coefficient values of all the six urban hierarchy groups. For example, the Gini coefficient values of the group of Megacity Ⅰ (M Ⅰ), Megacity Ⅱ, Big city Ⅰ, Big city Ⅱ, and Medium city (M Ⅰ Ⅱ-B Ⅰ Ⅱ-Med) from 2011 to 2013 are 0.5101, 0.5171 and 0.5134, which shows a fluctuation. This indicates that there are fluctuations in the process of dynamic adjustment of the population size distribution of all six groups. This also indicates that it cannot judge the dynamic adjustment trends of population size distribution only by the Gini coefficient values of all the six groups from 2005 to 2020.
According to , the linear plot at 95% confidence interval of the population size Gini coefficients of the six urban hierarchy groups is drawn, as shown in .
illustrates the scatter points of the Gini coefficients of population size of the six urban hierarchy groups. Although there are fluctuations, lines fitted by the scatter points of Megacity Ⅰ (M Ⅰ), group of Megacity Ⅰ and Megacity Ⅱ (M Ⅰ Ⅱ), and group of Megacity Ⅰ, Megacity Ⅱ, and Big city Ⅰ (M Ⅰ Ⅱ–B Ⅰ) are inclined to the lower right, while lines fitted by the scatter points of group of Megacity Ⅰ, Megacity Ⅱ, Big city Ⅰ and Big city Ⅱ (M Ⅰ Ⅱ–B Ⅰ Ⅱ), group of Megacity Ⅰ, Megacity Ⅱ, Big city Ⅰ, Big city Ⅱ and Medium city (M Ⅰ Ⅱ–B Ⅰ Ⅱ–Med), and Whole Urban System(W–U–S) are inclined to the topper right. This indicates that in the process of dynamic adjustment, the overall tendency of population size of M Ⅰ, M Ⅰ Ⅱ, and M Ⅰ Ⅱ–B Ⅰ is gradually even, while the overall tendency of the rest urban groups is gradually uneven.
4.2. Dynamic adjustment rate of Gini coefficients
shows the regression results of Equation (2) and the regression lines of population size of China’s six urban hierarchies, and the regression line of each urban hierarchy inclines to the lower right. The determination coefficient is more than 60% except for Megacity Ⅰ, signifying that the Gini coefficients of every urban hierarchy evince a decreasing trend. The determination coefficient of Megacity Ⅰ is only 44.5%, indicating that the fitting result of the equation is not well. The regression coefficients of the fitted equations are all less than zero, indicating that the Gini coefficients of each urban hierarchy have a decreasing trend. Although the fitting result of the equation of Megacity Ⅰ is not well, it clearly reflects that its Gini coefficient has a decreasing trend.
As shown in , the absolute values of the regression coefficient represent the decreasing rates of the Gini coefficient of the six urban hierarchies. For example, the absolute values of regression coefficients of Megacity Ⅱ and Big city Ⅰ are 0.00721 and 0.00624, indicating that the decreasing rate of the Gini coefficient of Megacity Ⅱ is faster than Big city Ⅰ. Gini coefficients represent the even degree of urban population degree of each urban hierarchy, thus the decreasing rate of Gini coefficients reflects the even rate of urban population size spatial distribution of each urban hierarchy in the process of dynamic adjustment. This indicates that the dynamic adjustment rate order of the six urban hierarchies is Megacity Ⅰ < Small city < Big city Ⅱ < Medium city < Big city Ⅰ < Megacity Ⅱ.
shows the regression results of Equation (2) and the regression lines of population size of China’s six urban hierarchy groups. The regression lines of Megacity Ⅰ, group of Megacity Ⅰ and Megacity Ⅱ, and group of Megacity Ⅰ, Megacity Ⅱ, and Big city Ⅰ are inclined to the lower right, and the regression lines of group of Megacity Ⅰ, Megacity Ⅱ, Big city Ⅰ and Big city Ⅱ, group of Megacity Ⅰ, Megacity Ⅱ, Big city Ⅰ, Big city Ⅱ and Medium city, and Whole Urban System are inclined to the topper right. The determination coefficients are more than 60% except for Megacity Ⅰ and a group of Megacity Ⅰ, Megacity Ⅱ, Big city Ⅰ, Big city Ⅱ, signifying that the Gini coefficients of the first three groups evince a decreasing trend and the last three groups evince an increasing trend. The determination coefficients of Megacity Ⅰ and group of Megacity Ⅰ, Megacity Ⅱ, Big city Ⅰ, Big city Ⅱ are respectively 44.5% and 49.3%, indicating that the fitting results of the equations are not well. The regression coefficients of the fitted equations of the first three groups are smaller than zero and the last three groups are bigger than zero, indicating that the Gini coefficients of the first three groups have a decreasing trend and the last three groups have an increasing trend. Although the fitting results of the equations of Megacity Ⅰ and group of Megacity Ⅰ, Megacity Ⅱ, Big city Ⅰ, Big city Ⅱ are not well, it clearly reflects their Gini coefficients’ dynamic adjustment trends.
As shown in , the regression coefficient value of the WholeUrbanSystem is 0.00359, indicating the dynamic adjustment tendency of the population size spatial distribution of China’s urban system to be unbalanced at the rate of 0.00359 per year. For further analysis, after adding Big city Ⅱ to the urban group of Megacity Ⅰ, Megacity Ⅱ, and Big city Ⅰ, it starts to appear the dynamic adjustment of unbalanced tendency at the rate of 0.00152 per year. After adding Medium city to the urban group of M Ⅰ Ⅱ–B Ⅰ Ⅱ, the unbalanced tendency rate accelerates at the rate of 0.00331 per year. After adding a Small city to the urban group of M Ⅰ Ⅱ–B Ⅰ Ⅱ–Med, the unbalanced tendency rate accelerates again at the rate of 0.00359 per year. Before adding Big city Ⅱ to the urban group of M Ⅰ Ⅱ–B Ⅰ, the dynamic adjustment tendencies of the population spatial distribution of urban groups are gradually to be even.
4.3. Correlation of dynamic adjustment
shows the correlation process of the Gini coefficients of urban population size between different urban hierarchies following the method in 3.1.3. The correlation coefficients are shown in the lower right, and the bigger the correlation coefficient, the stronger the correlation.
illustrates that there are complex correlations between different urban hierarchies on population size adjustment. For example, at 0.01 confidence level, the dynamic adjustment of Gini coefficients except for Megacity Ⅰ, other urban hierarchies are correlated with each other. Megacity Ⅱ correlates with urban hierarchies of Big city Ⅰ, Big city Ⅱ, Medium city, and Small city with correlated coefficients of 0.9441, 0.9206, 0.9176, and 0.7941. Big city Ⅰ correlates with Megacity Ⅱ, Big city Ⅱ, Medium city, and Small city with correlated coefficients of 0.9206, 8971, 0.9294, and 0.8294, etc. The values of correlation coefficients indicate a positive correlation between all urban hierarchies. The values indicate the degree of correlation between these cities. For example, the correlation coefficient between Big city Ⅰ and Big city Ⅱ is 0.8971, and this is 0.9294 between Big city Ⅰ and Medium city, indicating that it has stronger correlation between Big city Ⅰ and Medium city than Big city Ⅰ and Big city Ⅱ.
Gini coefficient represents the even degree of urban population size, thus this also reflects the correlation degree of the dynamic adjustment process of population size of different urban hierarchies. The above results indicate that at 0.01 confidence level, the dynamic adjustment of population size distribution except for Megacity Ⅰ, other urban hierarchies are correlated with each other. The correlation coefficient values reflect the correlation degree of population size between different urban hierarchies.
reports the Global Moran’s I of China’s 230 cities from 2005 to 2020, all of which are significant at 0.01 level, and all of which are greater than zero and concentrate between 0.10 and 0.14. This indicates that there is a positive spatial correlation among China cities in the process of urban population size spatial distribution dynamic adjustment from 2005 to 2020. The biggest value of Moran’s I was 0.1371 in 2014. The smallest value was 0.1070 in 2008. Overall, the values of Moran’s I did not fluctuate widely from 2005 to 2020. This indicates that it exists a stable spatial positive correlation between population size and spatial distribution of China cities in the process of dynamic adjustment.
Table 4. Moran’s I of urban population size spatial distribution of China cities.
5. Discussion
5.1. The dynamic adjustment tendency of urban population size spatial distribution
Results of 4.1 indicate that in the process of dynamic adjustment, the overall tendency of population size of each urban hierarchy is gradually even. This result is consistent with the regulated policies of the China government. The China government manages its cities by sorting them into different urban hierarchies. The economic development level, administrative status, and regulated policies of cities within the same urban are similar, which determines that the average wages, infrastructure condition, social welfare, and living cost of urban individuals within the same urban hierarchy are similar (Tsai and Chiang Citation2019; H. Zhang et al. Citation2016; Y. Zhang et al. Citation2019). This further determines that urban residents within the same urban hierarchy have similar utility levels. It is well known that individuals’ location choice determines urban population size spatial distribution (Lucas and Rossi-Hansberg Citation2002; Osawa and Akamatsu Citation2020), and individuals pursue maximised utility level (Samuelson and Nordhaus Citation2010; Varian Citation2010). Therefore, the population size of cities within the same urban hierarchy is gradually to be even in the process of population size dynamic adjustment due to their similar conditions.
Further results show the population size distribution of the whole urban system of China is gradually uneven, which indicates that the tendency of even distribution adjustment of the six urban hierarchies is not in line with the dynamic adjustment tendency of the whole urban system. This further illustrates the dynamic adjustment of population size spatial distribution of cities within the same urban hierarchy to be even may not mean this also narrows the gap of population size of cities of different urban hierarchies. The further result indicates the population size spatial distribution of M Ⅰ Ⅱ–B Ⅰ is gradually even. The uneven distribution tendency of the whole urban system is mainly caused by the increasing population size gaps between Big city Ⅱ and M Ⅰ Ⅱ–B Ⅰ, Medium city and M Ⅰ Ⅱ–B Ⅰ Ⅱ, Small city and M Ⅰ Ⅱ–B Ⅰ Ⅱ–Med.
The above results illustrate that the present regulated policies of China’s government make the population size distribution of cities within the same urban hierarchies gradually even; it is also effective for even distribution of M Ⅰ Ⅱ–B Ⅰ. However, the dynamic adjustment tendency of the whole urban system is gradually uneven. Therefore, to manage the uneven dynamic tendency of the whole urban system, the further regulated policies of China’s government should be paid more attention to how to narrow the gaps of the population size between Big city Ⅱ and M Ⅰ Ⅱ–B Ⅰ, Medium city and M Ⅰ Ⅱ–B Ⅰ- Ⅱ, Small city and M Ⅰ Ⅱ–B Ⅰ Ⅱ–Med.
5.2. The dynamic adjustment rate of urban population size spatial distribution
By sorting China’s cities into six urban hierarchies, this paper investigates the even degree rate of each urban hierarchy, and we find that the dynamic adjustment rate order of population size to even is Megacity Ⅰ < Small city < Big city Ⅱ < Medium city < Big city Ⅰ < Megacity Ⅱ. To the best of our knowledge, we are first studying the dynamic adjustment of China’s urban population size spatial distribution by sorting cities into different urban hierarchies. The studying perspective is in line with the urban economic development level as well as the urban regulated policies of China’s government.
Urban economics and policies are two important factors affecting the dynamic adjustment of urban population size spatial distribution (Guo et al. Citation2023; Zhong et al. Citation2022). For better economic development, the urban population of Megacity Ⅰ is larger than other urban hierarchies, which causes many urban problems (Meng and Long Citation2022; Yi, Qi, and Chen Citation2022). To cope with these problems, the China government has implemented the strictest restrictive policies on Megacity Ⅰ including strictly controlling the increasing Hukou and urban construction land areas of Megacity especially Megacity Ⅰ (China State Council Citation2000; Citation2014; Citation2021). It is not considered the difference of population size among cities of Megacity Ⅰ of these policies, for example, the population size of Beijing is more than 20 million and Wuhan is about 10 million in 2018. As a result, not only do these policies restrict the increasing rates of population size of larger cities, but also restrict other cities of Megacity Ⅰ, which causes the even rate of population size spatial distribution to be slower. The strictest construction land regulated policies on Megacity Ⅰ raise the housing price of these cities, which increases the cost of residents living and enterprise production cost (Garriga et al. Citation2023). As relatively liberal policies of the residence registration system and land management of Megacity Ⅱ and Big city Ⅰ as well as their relatively better economic development, many individuals and enterprises migrate to these cities for maximised utilities (Hsu and Ma Citation2021). This determines Megacity Ⅱ and Big city Ⅰ with higher even rate population size spatial distribution in the process of dynamic adjustment. During the same period, the China government implemented many policies to develop the economics cities of Medium city and Small city urban hierarchies as well as more liberal policies for the residence registration system and land management (Sun et al. Citation2021). These policies increase the residents’ utilities of these cities, which is conducive to promoting population migration and the evenness of population spatial distribution of these urban hierarchies (Bashir et al. Citation2020; Xiong et al. Citation2020; Yang et al. Citation2022). However, we find that the even rate of Medium city is faster than Big city Ⅱ, which might be the result of the superposition of economic and management policy. Although the economic development level of Big city Ⅱ is better than that of Medium city, the regulated policies of the China government on Big city Ⅱ are also more strict than Medium city. In conclusion, under the superposition of economic and management policy and the interaction between different urban hierarchies, the even rates of dynamic adjustment of urban population size spatial distribution reflect Megacity Ⅰ < Small city < Big city Ⅱ < Medium city < Big city Ⅰ < Megacity Ⅱ.
To investigate the population size spatial distribution of the whole urban system, this paper sorts China cities into six groups from Megacity Ⅰ to Whole Urban System by adding one urban hierarchy per step. The results indicate that the dynamic adjustment rates of population size spatial distribution to even have decelerated after every adding one urban hierarchy from Megacity Ⅰ to M Ⅰ Ⅱ–B Ⅰ. Then, after adding Big city Ⅱ into M Ⅰ Ⅱ–B Ⅰ, the equation regression coefficient of the M Ⅰ Ⅱ–B Ⅰ Ⅱ becomes ‘+0.00152’, which indicates the dynamic adjustment of population size spatial distribution becomes uneven. After adding Medium city to M Ⅰ Ⅱ–B Ⅰ Ⅱ, the equation regression coefficient increases to ‘+0.0031’; After adding Small city to M Ⅰ Ⅱ–B Ⅰ Ⅱ–Med, the equation regression coefficient increases to ‘+0.00359’. The above results indicate the population size gaps of Megacity Ⅰ, Megacity Ⅱ and Big city Ⅰ had decreased from 2005 to 2020, which makes the dynamic adjustment tendency of population size distribution even; However, the population size gaps of the Whole Urban System had increased from 2005 to 2020, which make the dynamic adjustment tendency of population size distribution uneven.
The above results indicate that the China government can ease the regulation policy of population size increasing of Big city Ⅱ, which may increase its even rate of dynamic adjustment tendency of population size spatial distribution and narrow the population size gap between Big city Ⅱ and M Ⅰ Ⅱ–B Ⅰ. It is also suggested to increase policies to support Medium city and Small city, such as improving urban transport infrastructure, medical and education conditions, as well as increasing investment to promote economic development, which may be beneficial to absorb more individuals to migrate to these cities to decrease the population size gap.
5.3. The correlation in the process of dynamic adjustment of population size spatial distribution
The analysis of 5.2 and the results of 4.3 indicated that the dynamic adjustment of population spatial distribution is a complex process. It contains different urban economic development levels of each city, policies affecting and the interaction between different urban hierarchies and cities, which may determine the complicated correlations between different urban hierarchies and cities. All cities of a nation constitute a complex system. Individuals migrate into a city, which changes the population size and evenness of the urban hierarchy of the city. It also altered the evenness distribution of other urban hierarchies as well as the whole urban system due to the correlation between different urban hierarchies and spatial correlation of cities. This is in line with the research of Henderson and Venables (Citation2009) and Helsley and Strange (Citation2014). They proposed population migration among cities changes the population distribution of the whole urban system because all cities constitute a complex system. The finding supplements the literature of the urban system by the population spatial distribution change of one urban hierarchy may affect other urban hierarchies due to the existence of correlation, as well as the population spatial distribution change of one city affect other cities due to the lobal spatial correlation.
6. Conclusion
This study aims to explore the dynamic adjustment of China’s population size spatial distribution from the perspective of urban hierarchy. We utilise methods of the Gini coefficient, linear regression, and coefficients of Spearman and Moran’s I to explore the dynamic adjustment tendency, adjustment rate and correlation of urban population size distribution from the perspective of urban hierarchy. We find that overall, the population size spatial distribution is gradually even at the same urban hierarchy. In this process, the dynamic adjustment rate of each urban hierarchy is different, and the order is Megacity Ⅰ < Small city < Big city Ⅱ < Medium city < Big city Ⅰ < Megacity Ⅱ. We also find that overall, the population size spatial distribution of the whole urban system is gradually uneven, which may be caused by the population size gap between Big city Ⅱ and M Ⅰ Ⅱ–B Ⅰ, Medium city and M Ⅰ Ⅱ–B Ⅰ- Ⅱ, Small city and M Ⅰ Ⅱ–B Ⅰ Ⅱ–Med. Furthermore, we discover that there are positive correlations between different urban hierarchies and positive globale spatial correlations among all the cities in the process of the dynamic adjustment of urban population size spatial distribution.
The contributions of this paper are as follows: (a) This paper sorts Chinese cities into six urban hierarchies according to the urban population size, which is in line with the urban regulating policies according to urban hierarchies of the Chinese government as well as their urban economic development level. It also provides an opportunity to observe the dynamic adjustment of urban population size and spatial distribution of different urban hierarchies in more detail. (b) This paper sorts China's cities into six groups to explore the dynamic process causing the population size distribution to be uneven in the whole urban system, which is useful for making regulated policies more targeted. (c) This paper explores the even rates of six urban hierarchies and six urban groups, as well as the correlation between urban hierarchies and global correlation among cities in the process of dynamic adjustment of urban population size distribution, which conducts a useful try for investigating the dynamic adjustment process and mechanism of urban population size spatial distribution.
The limitations of this study are suitable for further research. First, the mechanism leading to the results should be analysed in depth. Second, the implementation of policies on population distribution should be further studied.
Disclosure statement
The authors declare no conflict of interest.
References
- Bashir, Muhammad Farhan, Bilal Benjiang Ma, Bushra Komal, Muhammad Adnan Bashir, Duojiao Tan, and Madiha Bashir. 2020. “Correlation Between Climate Indicators and COVID-19 Pandemic in New York, USA.” Science of The Total Environment 728 (August): 138835. https://doi.org/10.1016/j.scitotenv.2020.138835.
- Chen, Yi, Ying Ge, Guofu Yang, Zhaoping Wu, Yuanyuan Du, Feng Mao, and Shun Liu. 2022. “Inequalities of Urban Green Space Area and Ecosystem Services Along Urban Center-Edge Gradients.” Landscape and Urban Planning 217 (January): 104266. https://doi.org/10.1016/j.landurbplan.2021.104266.
- China State Council. 2000. “Circular of The State Council on Implementing Several Policies and Measures for the Large-scale Development of the Western Region.” http://www.gov.cn/gongbao/content/2001/content_60854.htm.
- China State Council. 2014. “Opinions of The State Council on Further Promoting the Reform of the Household Registration System.” http://www.gov.cn/gongbao/content/2014/content_2729568.htm.
- China State Council. 2021. “The 14th Five-Year Plan for National Economic and Social Development of the People's Republic of China and the Outline of the Long-range Goals for 2035.” https://www.gov.cn/xinwen/2021-03/13/content_5592681.htm.
- Garriga, Carlos, Aaron Hedlund, Yang Tang, and Ping Wang. 2023. “Rural-Urban Migration, Structural Transformation, and Housing Markets in China.” American Economic Journal: Macroeconomics 15 (2): 413–440. https://doi.org/10.1257/mac.20160142.
- Gaughan, Andrea E., Forrest R. Stevens, Zhuojie Huang, Jeremiah J. Nieves, Alessandro Sorichetta, Shengjie Lai, Xinyue Ye. 2016. “Spatiotemporal Patterns of Population in Mainland China, 1990 to 2010.” Scientific Data 3 (1): 160005. https://doi.org/10.1038/sdata.2016.5.
- Guo, Wei, Jinke Liu, Xuesheng Zhao, Wei Hou, Yunxuan Zhao, Yongxing Li, Wenbin Sun, and Deqin Fan. 2023. “Spatiotemporal Dynamics of Population Density in China Using Nighttime Light and Geographic Weighted.” International Journal of Digital Earth 16 (1): 2704–2723. https://doi.org/10.1080/17538947.2023.2233493.
- Hackmann, Angelina, and Torben Klarl. 2020. “The Evolution of Zipf’s Law for U.S. Cities.” Papers in Regional Science 99 (3): 841–852. https://doi.org/10.1111/pirs.12498.
- Helsley, Robert W., and William C. Strange. 2014. “Coagglomeration, Clusters, and the Scale and Composition of Cities.” Journal of Political Economy 122 (5): 1064–1093. https://doi.org/10.1086/676557.
- Henderson, J. Vernon, and Anthony J. Venables. 2009. “The Dynamics of City Formation.” Review of Economic Dynamics 12 (2): 233–254. https://doi.org/10.1016/j.red.2008.06.003.
- Hsu, Wen-Tai, and Lin Ma. 2021. “Urbanization Policy and Economic Development: A Quantitative Analysis of China’s Differential Hukou Reforms.” Regional Science and Urban Economics 91 (November): 103639. https://doi.org/10.1016/j.regsciurbeco.2020.103639.
- Jiang, Changjun, Jintao Li, and Jilai Liu. 2022. “Does Urbanization Affect the Gap Between Urban and Rural Areas? Evidence from China.” Socio-Economic Planning Sciences 82: 101271. https://doi.org/10.1016/j.seps.2022.101271.
- Jiang, Zhidian, Weixin Zhai, Xiangfeng Meng, and Ying Long. 2020. “Identifying Shrinking Cities with NPP-VIIRS Nightlight Data in China.” Journal of Urban Planning and Development 146 (4): 04020034. https://doi.org/10.1061/(ASCE)UP.1943-5444.0000598.
- Li, Shujuan. 2016. “Rank-Size Distributions of Chinese Cities: Macro and Micro Patterns.” Chinese Geographical Science 26 (5): 577–588. https://doi.org/10.1007/s11769-015-0792-8.
- Li, Yingcheng, and Xingjian Liu. 2018. “How Did Urban Polycentricity and Dispersion Affect Economic Productivity? A Case Study of 306 Chinese Cities.” Landscape and Urban Planning 173 (May): 51–59. https://doi.org/10.1016/j.landurbplan.2018.01.007.
- Li, Xiaoma, and Weiqi Zhou. 2018. “Dasymetric Mapping of Urban Population in China Based on Radiance Corrected DMSP-OLS Nighttime Light and Land Cover Data.” Science of The Total Environment 643 (December): 1248–1256. https://doi.org/10.1016/j.scitotenv.2018.06.244.
- Lucas, Robert E., and Esteban Rossi-Hansberg. 2002. “On the Internal Structure of Cities.” Econometrica 70 (4): 1445–1476. https://doi.org/10.1111/1468-0262.00338.
- Meng, Xiangfeng, and Ying Long. 2022. “Shrinking Cities in China: Evidence from the Latest Two Population Censuses 2010–2020.” Environment and Planning A: Economy and Space 54 (3): 449–453. https://doi.org/10.1177/0308518X221076499.
- Moran, P. A. P. 1950. “Notes on Continuous Stochastic Phenomena.” Biometrika 37 (1/2): 17. https://doi.org/10.1093/biomet/37.1-2.17.
- Nam, Kyung-Min. 2017. “Is Spatial Distribution of China’s Population Excessively Unequal? A Cross-Country Comparison.” The Annals of Regional Science 59 (2): 453–474. https://doi.org/10.1007/s00168-017-0839-0.
- National Bureau of Statistics of China. 2023. “Statistical Bulletin of the People's Republic of China on National Economic and Social Development for 2022.” https://mp.weixin.qq.com/s?__biz=MjM5Njg5MjAwMg==&mid=2651527489&idx=1&sn=ab6af7cfde0cd4ca89e2072938179e63&scene=0.
- National Development and Reform Commission of China. 2016. “The National Development and Reform Commission Issued a Circular on the 13th Five-Year Plan to Promote the Rise of the Central Region.” https://www.ndrc.gov.cn/xxgk/zcfb/tz/201612/t20161226_962830.html.
- Nowell, Lisa H., Patrick W. Moran, Laura M. Bexfield, Barbara J. Mahler, Peter C. Van Metre, Paul M. Bradley, Travis S. Schmidt, Daniel T. Button, and Sharon L. Qi. 2021. “Is There an Urban Pesticide Signature? Urban Streams in Five U.S. Regions Share Common Dissolved-Phase Pesticides but Differ in Predicted Aquatic Toxicity.” Science of The Total Environment 793 (November): 148453. https://doi.org/10.1016/j.scitotenv.2021.148453.
- Osawa, Minoru, and Takashi Akamatsu. 2020. “Equilibrium Refinement for a Model of Non-Monocentric Internal Structures of Cities: A Potential Game Approach.” Journal of Economic Theory 187 (May): 105025. https://doi.org/10.1016/j.jet.2020.105025.
- Qu, Jingjing, Aijun Li, and Morié Guy-Roland N’Drin. 2023. “Measuring Technology Inequality Across African Countries Using the Concept of Efficiency Gini Coefficient.” Environment, Development and Sustainability. https://doi.org/10.1007/s10668-022-02236-3.
- Reia, Sandro M., P. Suresh, C. Rao, Marc Barthelemy, and Satish V. Ukkusuri. 2022. “Spatial Structure of City Population Growth.” Nature Communications 13 (1): 5931. https://doi.org/10.1038/s41467-022-33527-y.
- Samuelson, Paul A., and William D. Nordhaus. 2010. Economics The McGraw-Hill Series Economics. 19th ed. Boston: McGraw-Hill Irwin.
- Sun, Xiangdong, Ouyang Yuan, Zhao Xu, Yanhui Yin, Qian Liu, and Ling Wu. 2021. “Did Zipf’s Law Hold for Chinese Cities and Why? Evidence from Multi-Source Data.” Land Use Policy 106 (July): 105460. https://doi.org/10.1016/j.landusepol.2021.105460.
- Tsai, I-Chun, and Shu-Hen Chiang. 2019. “Exuberance and Spillovers in Housing Markets: Evidence from First- and Second-Tier Cities in China.” Regional Science and Urban Economics 77 (July): 75–86. https://doi.org/10.1016/j.regsciurbeco.2019.02.005.
- Varian, Hal R. 2010. Intermediate Microeconomics: A Modern Approach. 8th ed. New York: W.W. Norton & Co.
- Verbavatz, Vincent, and Marc Barthelemy. 2020. “The Growth Equation of Cities.” Nature 587 (7834): 397–401. https://doi.org/10.1038/s41586-020-2900-x.
- Wang, Jie, and Xiaoling Zhang. 2022. “Land-Based Urbanization in China: Mismatched Land Development in the Post-Financial Crisis Era.” Habitat International 125 (July): 102598. https://doi.org/10.1016/j.habitatint.2022.102598.
- Xiong, Xin, Qing Xu, Guowang Jin, Hongmin Zhang, and Xin Gao. 2020. “Rank-Based Local Self-Similarity Descriptor for Optical-to-SAR Image Matching.” IEEE Geoscience and Remote Sensing Letters 17 (10): 1742–1746. https://doi.org/10.1109/LGRS.2019.2955153.
- Xu, Gang, Limin Jiao, Man Yuan, Ting Dong, Boen Zhang, and Chunmeng Du. 2019. “How Does Urban Population Density Decline Over Time? An Exponential Model for Chinese Cities with International Comparisons.” Landscape and Urban Planning 183 (March): 59–67. https://doi.org/10.1016/j.landurbplan.2018.11.005.
- Xu, Yong, Yimeng Song, Jixuan Cai, and Hong Zhu. 2021. “Population Mapping in China with Tencent Social User and Remote Sensing Data.” Applied Geography 130 (May): 102450. https://doi.org/10.1016/j.apgeog.2021.102450.
- Yang, Kai, Rui Li, Cassie Castorena, and B. Shane Underwood. 2022. “Correlation of Asphalt Binder Linear Viscoelasticity (LVE) Parameters and the Ranking Consistency Related to Fatigue Cracking Resistance.” Construction and Building Materials 322 (March): 126450. https://doi.org/10.1016/j.conbuildmat.2022.126450.
- Ye, Xinyue, and Yichun Xie. 2012. “Re-Examination of Zipf’s Law and Urban Dynamic in China: A Regional Approach.” The Annals of Regional Science 49 (1): 135–156. https://doi.org/10.1007/s00168-011-0442-8.
- Yi, Yanchun, Ji Qi, and Dong Chen. 2022. “Impact of Population Agglomeration in Big Cities on Carbon Emissions.” Environmental Science and Pollution Research 29 (57): 86692–86706. https://doi.org/10.1007/s11356-022-21722-9.
- You, Xiaotong, Yanan Sun, and Jiawei Liu. 2022. “Evolution and Analysis of Urban Resilience and Its Influencing Factors: A Case Study of Jiangsu Province, China.” Natural Hazards 113 (3): 1751–1782. https://doi.org/10.1007/s11069-022-05368-x.
- Zhang, Zhuoyuan. 2023. Handbook of Chinese Economics. Singapore: Springer Nature Singapore. https://doi.org/10.1007/978-981-99-0420-4.
- Zhang, Hong, Linjun Li, Eddie Chi-Man Hui, and Vera Li. 2016. “Comparisons of the Relations Between Housing Prices and the Macroeconomy in China’s First-, Second- and Third-Tier Cities.” Habitat International 57 (October): 24–42. https://doi.org/10.1016/j.habitatint.2016.06.008.
- Zhang, Mingming, Jinghui Liu, Liyun Liu, and Dequn Zhou. 2023. “Inequality in Urban Household Energy Consumption for 30 Chinese Provinces.” Energy Policy 172 (January): 113326. https://doi.org/10.1016/j.enpol.2022.113326.
- Zhang, Zengxiang, Fang Liu, Xiaoli Zhao, Xiao Wang, Lifeng Shi, Jinyong Xu, and Sisi Yu. 2018. “Urban Expansion in China Based on Remote Sensing Technology: A Review.” Chinese Geographical Science 28 (5): 727–743. https://doi.org/10.1007/s11769-018-0988-9.
- Zhang, Yu, Liyin Shen, Chenyang Shuai, Jing Bian, Mengcheng Zhu, Yongtao Tan, and Gui Ye. 2019. “How Is the Environmental Efficiency in the Process of Dramatic Economic Development in the Chinese Cities?” Ecological Indicators 98 (March): 349–362. https://doi.org/10.1016/j.ecolind.2018.11.006.
- Zhong, Sujuan, Mingshu Wang, Yi Zhu, Zhigang Chen, and Xianjin Huang. 2022. “Urban Expansion and the Urban–Rural Income Gap: Empirical Evidence from China.” Cities 129 (October): 103831. https://doi.org/10.1016/j.cities.2022.103831.