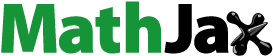
ABSTRACT
The leaf area index (LAI) inversion accuracy of the water cloud model (WCM) based on the SAR images is low. The main reason is that the WCM model assumes two independent contributions from plant and soil surfaces, without taking into account their interactions. Based on this, this study proposes an efficient LAI estimation method that combines the advanced integral equation model (AIEM) and modified water cloud model (MWCM). Images collected by GaoFen-3 synthetic aperture radar (GF-3 SAR) in the Xiangfu area in the east of Kaifeng City, Henan Province, are processed with a modified SAR-BM3D method and used as test data of the proposed method. The proposed AIEM-MWCM method that combines the AIEM and MWCM models is employed to perform the remote sensing inversion of winter wheat LAI throughout the growth cycle. The results show that the fitting accuracy of winter wheat LAI in the five growth stages achieved by the proposed AIEM-MWCM method inversion is better than that of the Dubois-MWCM model. The R2 value of the proposed method is higher than 0.8, and its root mean squared error (RMSE) is lower than 0.3.
1. Introduction
Leaf area index (LAI) is an important parameter that characterizes the energy transmission and exchange between soil, crops, and the atmosphere in farmland systems (Anderson et al. Citation2004; Jarlan et al. Citation2008). It is also a key index for measuring the biology and biochemistry of the vegetation canopy (Inge et al. Citation2004). In this paper, the LAI is defined as half of the sum of the total leaf area per unit surface area (Ren et al. Citation2018). Achieving rapid and accurate inversion of LAI is of great research significance for agricultural monitoring and biogeochemical cycles.
Traditional LAI ground survey techniques, including hemispherical photography, using optical sensors with the LAI-2000/2200, and terrestrial light detection and ranging (LIDAR) scanning, are typically time-consuming, labor intensive, and often destructive to vegetation, which limits their application and makes them unsuitable for high temporal and spatial frequency measurements (Inge et al. Citation2004; Yan et al. Citation2019). Moreover, capturing high spatial variability, which is often related to plant growth, via ground measurement is challenging. Remote sensing technology represents an effective method for regional or global crop LAI monitoring due to its advantages of a large coverage area and high efficiency. Based on optical remote-sensing images, these sensors are susceptible to meteorological conditions, such as clouds, rain, and fog, which not only reduces the inversion accuracy of crop LAI but also increases the uncertainty of the inversion result.
Since the wavelengths in the microwave region of the electromagnetic spectrum are less affected by the atmosphere, synthetic aperture radar (SAR) sensors, such as RADARSAT-1, Envisat-ASAR, and GF-3, can successfully image the Earth even when clouds are present. The main methods for LAI remote sensing inversion from the SAR remote sensing images include the empirical and water cloud models. The empirical model uses the backscattering coefficients of the horizontal transmitted and horizontally received (HH), horizontally transmitted and vertically received (HV), and vertically transmitted and vertically received (VV) polarization modes to establish a correlation with the measured LAI (Gao, Niu, and Wu Citation2010; Kurosu, Fujita, and Chibae Citation1995; Mansaray et al. Citation2017; Toan et al. Citation1989). Several studies have examined the relationship between the empirical model’s sensitivity and LAI for different frequencies and polarizations (Sonia et al. Citation2019; Pauline et al. Citation2014; Mandal et al. Citation2019). Although empirical models are easy to use, the transferability of the resulting statistical relationships usually faces certain constraints when data are collected under different conditions or using sensors with different characteristics.
The water cloud model (WCM) is a semi-empirical parameter inversion model first proposed by Attema and Ulaby in 1978 (Attema and Ulaby Citation1978). Recent research has examined the wide application of the WCM in numerous subsequent inversion studies on soil moisture and vegetation growth parameters (Emilie et al. Citation2015; Mehdi et al. Citation2015; Tao et al. Citation2016; Yadav, Prasad, and Bala Citation2021; Yang et al. Citation2016). The results have suggested that the WCM approach can be suitable for the operational monitoring of crops. However, the WCM involves many model parameters that are difficult to obtain. The WCM attempts to use straight forward vegetation parameters to represent the canopy scattering and attenuation components in the model (Shubham et al. Citation2022; Verma et al. Citation2022), which leads to an low accuracy problem of model inversion. Furthermore, the WCM ignores the mutually influencing interaction between plant and soil surfaces, which has a substantial influence on the total scattering and attenuation properties of the land surface.
In summary, The LAI inversion accuracy of the WCM based on the SAR images is low. The main reason is that the WCM model assumes two independent contributions from plant and soil surfaces, without taking into account their interactions. When the plant density is considerable, this might cause a large departure from the true scattering and attenuation properties of the ground surface. By using the GF-3 SAR images processed by the modified SAR-BM3D method, this study combines the advanced integral equation model (AIEM) and modified water cloud model (MWCM) and proposes an AIEM-MWCM inversion method for LAI in five growth stages of winter wheat. The proposed method combines the AIEM and MWCM models and makes full use of their respective advantages to invert surface parameters, which can improve inversion accuracy.
2. Materials and methods
2.1. Materials
2.1.1. Study area
The study area used in this work was the Xiangfu area (34°30′–34°56′ N, 114°07′–114°43′ E) in the eastern part of Kaifeng City, Henan Province, which belongs to the Yellow River alluvial plain. This is a typical winter wheat growing area and an important food-producing region. It has a size of 5 km × 5 km, an average altitude of 62.5–89.3 m, an average annual temperature of 14°C, an average annual precipitation of 628 mm, and an average frost-free period of 214 days. A temperate continental monsoon climate provides sufficient sunshine, suitable temperatures, and abundant precipitation, which are conducive to crop growth. The main crops in the study area include winter wheat, rice, and corn, but according to the growing period of crops, the main crop is winter wheat. The location of the study area and distribution of sampling points are shown in .
2.1.2. Satellite data collection and processing
In this study, the GF-3 SAR data collected in the study area were downloaded from the China Centre for Resources Satellite Data and Application (CCRSDA) and used to develop the proposed model. The GF-3 SAR data were in the C band, the incidence angles ranged from 20° to 41°, and the transit days included March 2, March 21, April 7, May 28, and June 9, 2021. The operating frequency was 5.4 GHz, and the spatial resolution was 8 m. The images were processed by performing full polarization introduction, radiometric calibration, amplitude enhancement, and speckle filtering, and three scattering components were extracted using the Freeman-Durden polarimetric decomposition of the processed data. By using the TanDEM-X elevation model data (DEM), the GF-3 SAR data were calibrated to sigma nought using the calibration factors and sine of the local incidence angle obtained from the TanDEM-X DEM. To reduce speckle noise, this study performed speckle filtering using a BoxCar 5 × 5 kernel filter. The sigma-nought radiometric calibration was performed using the absolute calibration coefficients downloaded from CCRSDA (http://www.cresda.com/CN/).
The MODIS LAI product (MOD15A2) was downloaded from the United States Geological Survey (http://lpdaac.usgs.gov/). The orbital number was h26v05. The spatial resolution of the product was 500 m, and the temporal resolution was 8 days. The downloaded data were trans-projected and subjected to clipping to obtain the LAI images of the study area.
2.1.3. Field data observation
The aim of the experiment was to prevent changes in weather conditions from affecting the accuracy of the measured LAI. The LAI inversion of winter wheat in five growth stages was analyzed; the selected acquisition dates were March 1, March 24, April 20, May 27, and June 03, 2021, and they were almost synchronous with the satellite transit time. The daily acquisition interval was from 07:30 am to 09:30 am Fifty winter wheat quadrats with a size of 16 m × 16 m were used in the experiment, and three spots with the same growth status were selected. All quadrats were at least 30 m away from trees, buildings, and roads. During data sampling, sunlight was shielded, and LAI-2000 was used for measurement. In particular, a 180° covering cap was used to ensure that there were no measurement errors. In addition, the distance between the measured ground and the instrument was adjusted to 5 cm according to the standard to ensure that there was no error between the winter wheat canopy and the viewing angle range of the measurement instrument. Further, to avoid LAI measurement errors, the LAI of each sample site was measured four times, and the average value was used as the end value. The measured LAI data were divided according to the ratio of 3:2 into training and verification sets, i.e. 60 training datasets were selected for model inversion and the remaining 40 verification datasets were used for model verification. Furthermore, the longitude and latitude values of each winter wheat sampling point were recorded in real-time using a U.S. Global Positioning System (GPS) locator. The measurement process of winter wheat LAI and sampling point locations are presented in .
2.2. Methods
The proposed method for LAI estimation uses the AIEM-MWCM model that is developed using the GF-3 satellite data, as shown in . First, the modified SAR-BM3D was used to process the GF-3 satellite data by performing fully polarized guidance, radiometric calibration, amplitude enhancement, and speckle filtering to improve image quality. Then, Dubois and AIEM were used to calculate the soil backscattering coefficient and the radar vegetation index, respectively. Finally, the Dubois-MWCM and AIEM-MWCM models were constructed to retrieve the LAI values of winter wheat during the entire growth period.
2.2.1 Modified SAR-BM3D
The block-matching stage of the SAR-BM3D method aims to find the image block that is most similar to the reference block (Giovanni et al. Citation2017). The similarity measure based on the actual noise distribution is relatively single, and the filtered images can miss some details and generate unnecessary false information. Based on the SAR-BM3D method, the Pearson’s correlation coefficient is introduced to improve the similarity measure of the reference and matching blocks to improve the accuracy of image block matching and grouping. After the similarity measure is improved, the coordinated filtering and aggregation processing are performed to obtain the basic and final estimations, and the SAR image after noise reduction is obtained.
By matching and grouping a SAR image i with noise, the reference block with a size of is selected, and the similarity measure
is calculated as follows:
(1)
(1) where
is the apparent parameter.
The introduced Pearson’s correlation coefficient reflects the correlation degree between variables, and ; the Pearson’s correlation coefficient is calculated as follows:
(2)
(2) where
represents the covariance of the reference block a and matching block b;
and
represent the variances of image blocks a and b, respectively.
When searching for similar blocks using the SAR-BM3D method, the smaller the similarity measure is, the higher the similarity between the two image blocks is. Also, the larger the absolute value of
is, the higher the degree of positive (negative) correlation will be, indicating a higher similarity between the two images. Therefore, the similarity measure is redefined as follows:
(3)
(3) According to the preset similarity threshold
, M similar image blocks are searched, and a set of similar blocks is formed as follows:
(4)
(4)
The similar blocks are integrated into a three-dimensional (3D) array , and the tasks of 3D transformation, hard threshold processing, and 3D inverse transformation are performed to obtain the estimated value of the set of similar blocks as follows:
(5)
(5) where
represents the wavelet hard threshold, and
and
denote the 3D transformation and inverse transformation of a 3D array, respectively.
A pixel i can appear in several different combinations of similar blocks. The basic estimation image is obtained from the weighted average of the overlapping image blocks, given by
(6)
(6) where
represents the estimated value of an image block
containing pixel i obtained after the 3D inverse transformation;
represents the characteristic function of a similar block;
represents the basic estimated weight, and
;
denotes the number of all non-zeros retained after hard threshold filtering.
Before performing similar block matching on the original image, it is necessary to consider data obtained in the basic estimation stage and use the position information to perform block matching on the noisy image. According to the improved similarity measure method described in Equations (1)–(6) above, the improved similarity measure is expressed as follows:
(7)
(7)
(8)
(8)
The similarity block grouping result of an image is composed of a 3D array formed by the basic estimation and a 3D array formed by the original noisy image. The two 3D arrays are transformed into the 3D domain, Wiener filtering is performed on the 3D array
, and the estimated value of the set of similar blocks is obtained by 3D inverse transformation, which can be expressed as follows:
(9)
(9) where
represents the Wiener filtering function, and
and
represent the 3D transformation and 3D inverse transformation, respectively.
Using the same method as for the basic estimation, the final estimation is obtained by the weighted average, and then, the original position is restored to obtain the denoised image as follows:
(10)
(10) where
represents the number of image blocks in the similar block set;
is the estimated value of the image block
containing pixel i obtained after the 3D inverse transformation;
denotes the characteristic function of a similar block;
represents the final estimated weight, and
; the weighted weight depends on the Wiener filter coefficient
.
Based on the methods presented above, the equivalent number of loops (ENL) and edge preservation index (EPI) were used in the experiment to evaluate the effect of SAR image noise reduction. The equivalent visual number was used to evaluate the degree of suppression of speckle noise in SAR images. Generally, the larger the value of the equivalent visual number was, the better the suppression effect of speckle noise was. The equivalent apparent number was calculated as follows:
(11)
(11) where
and
represent the mean and standard deviation, respectively;
is related to the SAR image format, and for the intensity format,
, whereas for the amplitude format,
.
In addition, the edge retention index was used to evaluate the edge retention ability of filtered images. Normally, the closer its value is to one, the better is the edge information retention ability of a method. The edge retention index was calculated as follows:
(12)
(12) where
and
represent the image pixels before and after filtering, respectively.
2.2.2. Bare soil backscatter modeling by Dubois
Dubois, Jakobl, and Engman (Citation1995) established a semi-empirical SM retrieval model for a bare soil area. The Dubois empirical model was used on the bare surface, and f∈[1.5, 11 GHz], ∈[0.34, 2.5], and
∈[30°, 60°]; the retrieval effect of the root mean square height and soil water content was the best. The Dubois model can be conceptually described as follows:
(13)
(13)
(14)
(14) where
is the VV polarization backscattering coefficient;
is the HH polarization backscattering coefficient;
is the GF-3 satellite frequency, and in this work, it has a value of 5.4 GHz;
is the scattering angle;
is the wavenumber; and
is the wavelength.
2.2.3. Bare soil backscatter modeling by AIEM
The surface backscattering coefficient values obtained by the integral equation model (IEM) were not completely consistent with the measured values. Wu et al. (Citation2001) proposed an improved IEM in 2001 and optimized the AIEM in 2004. Because of the complexity of the AIEM, it cannot be directly applied to the automatic operation of soil water content inversion. Therefore, based on the complex theoretical formula, the statistical analysis of the factors affecting the radar backscattering coefficient in the theoretical model, combined with the measured data, was used to construct a semi-empirical model of soil water content; radar backscattering was used to extract soil water content information. Finally, the AIEM was used to generate a bare soil backscatter database for the GF-3 configuration.
The (double-basis) scattering coefficient of the AIEM is expressed as follows:
(15)
(15) where p and q represent the polarization modes of transmission and reception, respectively;
is the scattering coefficient;
is the Kirchhoff field term;
is the compensating field term; and
is the cross term.
By substituting the calculation formulas of Kirchhoff's surface field term, compensation field term, and cross term into (15), the scattering coefficient of the AIEM can be obtained as follows:
(16)
(16)
(17)
(17) where
is the free space wave number of the transmission medium;
is the root-mean-square height of the soil surface;
is the nth-order Fourier transform of the surface correlation function, where
,
,
,
,
, and
;
is the incidence azimuth;
is the scattering azimuth; and
and
are functions related to Fresnel reflectance
.
(18)
(18)
(19)
(19) where
is the dielectric constant of the soil;
is a rough surface with the correlated length; and the roughness power spectrum can be expressed as follows:
(20)
(20) where
is the correlation length.
Therefore, the AIEM can be conceptually described as follows:
(21)
(21) where
is the scattering angle;
is the polarization state (HH and VV polarizations);
is the input soil moisture of the Dobson model;
is the root-mean-square height;
is the correlation length; and
is the adopted exponential autocorrelation function. The ranges (minimum and maximum) of each of the three main model parameters are summarized in .
Table 1. Value ranges of the advanced integral equation model (AIEM) parameters.
2.2.4. Dubois–MWCM and AIEW-MWCM
The MWCM is an important model used to study the relationship between radar backscattering and crop parameters (Zhang et al. Citation2018), and it is defined as follows:
(22)
(22)
(23)
(23)
(24)
(24) where
is the total backscattering coefficient of the surface canopy covered by random polarimetric vegetation;
is the backscattering coefficient of the vegetation layer;
is the direct surface backscattering coefficient;
is the double-layer attenuation factor of the radar wave penetrating the crop layer;
is the incidence angle of the radar waves; M is the vegetation canopy parameter (i.e. LAI); B is the parameter that is defined by the vegetation type and incident electromagnetic wave frequency.
The model was built on the basis of the radiation transfer model, which considers only the volume scattering term from vegetation reflection and backscattering of the ground after double-layer attenuation of vegetation while ignoring other forms of scattering in vegetation and soil. The radar backscattering coefficient is sensitive to the surface dielectric constant and is closely related to soil moisture. The value of is obtained by using (13) and (14) and is then substituted into (22)–(24), and the radar vegetation index (
) is used to construct the Dubois-MWCM model, as shown in (25). Further,
is obtained by using (21) and is then substituted into (22)–(24);
is used to construct the AIEW-MWCM model, as shown in (26).
(25)
(25)
(26)
(26) where
,
, and
are the scattering components of the covariance matrix of the bulk scattering, even scattering, and odd scattering models, respectively; A and B are the Dubois-MWCM and AIEW-MWCM parameters determined by Levenberg–Marquardt nonlinear least square optimization.
The Dubois-MWCM and AIEW-MWCM denote the functions of a number of input parameters that quantify crop growth and soil surface parameters. However, SAR signals are also affected by the crop growth parameters, such as LAI, vegetation water content (VWC), and planting soil properties (). The backscattering coefficient of crops is expressed as follows:
(27)
(27) The polarization backscattering coefficient is mainly related to vegetation scattering. Therefore, the establishment of a lookup table (LUT) under the HH polarization can effectively invert the LAI obtained by the Dubois-MWCM and AIEW-MWCM models. The LUT method is, by nature, a direct comparison of the radiative transfer model backscattering value calculated from the GF-3 SAR images under the HV polarization against the backscattering value simulated by the Dubois-MWCM and AIEW-WCM models. It is a simple and widely used inversion method in the field of vegetation parameter retrieval from remote-sensing data. The LUT consists of two main steps: LUT generation and LUT searching.
In the LUT generation step, 90,000 parameter combinations were randomly generated following uniform distributions. The value ranges (i.e. the minimum and maximum values) of the five main model parameters are presented in .
Table 2. The range of all parameters in generating look up table.
In the LUT searching step, the mean square error Z was used to represent the difference between the observed and estimated backscattering values, and it was calculated as follows:
(28)
(28) where
is the backscattering value calculated from a GF-3 SAR image under the HV polarization,
is the backscattering value simulated by the AIEW-MWCM model, and N is the number of pixel points in the image.
2.2.5. Evaluation methodology
The fitting between the simulated and measured LAI values of winter wheat was evaluated through accuracy evaluation to obtain the optimal inversion model. Two common evaluation metrics, R2 (Wang et al. Citation2020) and RMSE (Amin et al. Citation2021; Jafari and Keshavarz Citation2021), were selected to evaluate the fitting accuracy, and they are respectively defined as follows:
(29)
(29)
(30)
(30) where R2 is the determination coefficient; y is the measured ecological parameter value;
is the estimated ecological parameter value;
is the average value of the measured ecological parameter; RMSE is the root mean square error;
is the measured LAI value;
is the estimated LAI value; and n is the number of samples.
3. Results and discussion
3.1. Improved filtering analysis of SAR-BM3D method
To verify the effectiveness of the SAR-BM3D method, the Frost, Kuan, SAR-BM3D, and improved SAR-BM3D filters were applied to the SAR images of the jointing stage in the measured area, as shown in and .
Figure 3. Image filtering results obtained by (a) Frost filter; (b) Kuan filter; (c) SAR-BM3D filter; and (d) improved SAR-BM3D filter.

Table 3. Objective and quantitative comparison of filtering results of different methods.
As shown in , the noise reduction effect after filtering by the Frost and Kuan filters was good, but their use made the image too smooth and blurred, resulting in many edge details being lost. The denoising effect of the SAR-BM3D filter was better than that of the Frost and Kuan filters, but the preserving effect of the image edge and texture information was incomplete. The improved SAR-BM3D filter has a good noise reduction effect; the filtered image was relatively clear, the visual effect in the homogenous area was good, and the image edge details were well-maintained.
Further, as presented in , the equivalent visual numbers of Frost and Kuan filtering were large, but the edge-preserving index was relatively small. The edge-preserving index of the wavelet threshold and SAR-BM3D approach was close to one, but the equivalent visual number was small. The improved SAR-BM3D filtering method was superior to the other filtering algorithms regarding the equivalent sight number and edge retention index. Based on the analysis results of subjective vision and objective quantitative indicators, the improved SAR-BM3D method exhibited good denoising performance and could effectively suppress the noise in SAR images and preserve the image edge details. However, there were still a few artifacts in the noisy area of SAR images with complex details.
3.2. LAI inversion by the RVIFreeman model
A regression model was constructed between the RVIFreeman and winter wheat LAI for the entire growth cycle using five types of RVIFreeman models, and the accuracy was evaluated. The evaluation results of the RVIFreeman model are presented in .
Figure 4. LAI Inversion by the RVIFreeman model. (a–e) are from the jointing, booting, heading, filling, and maturing stages, respectively.

As shown in , the inversion accuracy of the LAI of winter wheat during the entire growth stage obtained by the RVIFreeman model was low and could not meet the inversion requirements. The inversion accuracy of the mature stage was the highest among all the stages, with R2 = 0.5629 and RMSE = 0.6980. The second-best stage regarding the inversion accuracy was the booting stage, with R2 = 0.5484 and RMSE = 0.7521. The jointing stage had the least inversion accuracy, with R2 = 0.5295 and RMSE = 0.6988. Therefore, in the high-LAI area, the main component of the total scattering power was the volume scattering component, while secondary scattering and surface scattering were weak. However, in the low-LAI area, the radar scattering effect was relatively complex, and it was easy to form strong secondary scattering components, which reduced the sensitivity of the volume scattering component ratio value to the LAI value. In summary, for the radar vegetation index, the spectral saturation problem of winter wheat could be overcome by the penetration of radar to a certain extent.
3.3. LAI inversion by the backscattering coefficient of Dubois-MWCM and AEIM-MWCM
The Dubois-MWCM and AEIM-MWCM models were employed to extract the backscattering values of the HH and VV polarization modes from the GF-3 SAR images, respectively. The LAI observations and predicted values were then compared, as shown in and .
Table 4. Accuracy evaluation of the backscattering coefficient model of Dubois-MWCM.
Table 5. Accuracy evaluation of the backscattering coefficient model of AEIM-MWCM.
Because the interaction mechanism between radar and vegetation was complex and affected by surface conditions, there was no strong correlation between the two backscattering coefficients and LAI, as shown in and . The correlation between the HH polarization backscattering coefficient and the LAI was higher than that of the VV polarization backscattering coefficient because the HH polarization could better reflect the secondary and surface scattering of the surface.
3.4. LAI inversion by Dubois-MWCM
Because the polarization backscattering coefficient was mainly related to vegetation scattering, the parameters of the Dubois-MWCM were determined by the nonlinear least squares method under the HH cross-polarization, as shown in . The relationship between the measured and predicted LAI values obtained by the Dubois-MWCM under the HH polarization at different stages is presented in .
Figure 5. Correlation analysis of Dubois-MWCM and LAI. (a–e) are from the jointing, booting, heading, filling, and maturing stages, respectively.

Table 6. Model parameters at HH polarizations.
As shown in , under the HH polarization, the LAI inversion value obtained by the Dubois-MWCM had a strong correlation with the measured LAI. Among the five growth stages, the fitting accuracy between the jointing stage and measured LAI was the highest, having R2 = 0.7865 and RMSE = 0.4589, and it was followed by the maturation (R2 = 0.7850, RMSE = 0.4534), filling (R2 = 0.7754, RMSE = 0.4589), heading (R2 = 0.7731, RMSE = 0.4486), and booting stages (R2 = 0.7584, RMSE = 0.4651). In the Dubois-MWCM model, the SAR backscattering coefficient was directly used in the winter wheat LAI inversion, and the impact of ground surface reflection was neglected, which led to low LAI inversion accuracies.
Among the five growth stages of winter wheat, the jointing stage had the highest regional LAI inversion accuracy. When the winter wheat jointed and completely covered the ground surface, the accuracy of winter wheat LAI inversion was the highest because the microwave scattering process was simple, and the Dubois-MWCM contained fewer parameters at this growth stage.
3.5. LAI inversion by AEIM-MWCM
The parameters of the AEIM-MWCM model were determined by the nonlinear least squares method under the HH cross-polarization, as shown in . The AEIM-MWCM model was used to invert LAI by employing the least-squares algorithm and LUT method. The relationship between the LAI observations and the values predicted by the AEIM-MWCM model under the HH polarization is presented in .
Figure 6. Correlation analysis of AEIM-MWCM and LAI. (a–e) are from the jointing, booting, heading, filling, and maturing stages, respectively.

Table 7. Model parameters at HH polarizations.
As shown in , the AEIM-MWCM model achieved the best inversion of winter wheat LAI at the maturing stage, having an R2 of 0.8867 and an RMSE of 0.2989. The inversion accuracy of the stages decreased in the following order: jointing (R2 = 0.8720 and RMSE = 0.3524), filling (R2 = 0.8695 and RMSE = 0.3726), booting (R2 = 0.8535 and RMSE = 0.4213), and heading stage (R2 = 0.8519 and RMSE = 0.3395). In summary, the differences between the measured LAI and LAI value estimated from the GF-3 data were small, and the LAI retrieval results were satisfactory. These results indicate that the proposed LAI method for GF-3 data was reliable and that GF-3 data could provide acceptable performance for LAI estimation.
The C-band microwave signal penetrated the wheat layer to the ground surface, and it was reflected by the ground surface and received by the sensor. The microwave energy reflected by the ground surface accounted for a large proportion of the total microwave energy. Unlike the AEIM-MWCM model, the Dubois-MWCM model could effectively use the energy reflected from the crop canopy and ground surface. Therefore, the inversion accuracy of the AEIM-MWCM model was higher than that of the Dubois-MWCM model. Among the five growth stages of winter wheat, the jointing stage had the highest regional LAI inversion accuracy, and the heading stage had the lowest LAI inversion accuracy. When the winter wheat jointed and completely covered the ground surface, the accuracy of winter wheat LAI inversion was the highest because the microwave scattering process was simple, and the submodel contained fewer parameters at this growth stage. In contrast, at the heading stage, winter wheat ears and stem leaves showed obvious stratification, the microwave scattering process was complex, and the submodel contained a large number of parameters; thus, the LAI inversion accuracy of winter wheat was the lowest in the heading stage.
3.6. AEIM-MWCM verification
To verify the reliability of the LAI inversion model, the LAI value obtained by the AEIM-MWCM inversion was studied, and the measured LAI was taken as a true value. The regression analysis was conducted between the remaining 40 true values, other than the modeling samples and the LAI data obtained by the AEIM-MWCM model to obtain the correlation between the LAI inversion value and the true value in each growth stage, as shown in .
Figure 7. The AEIM-MWCM model and LAI verification analysis. (a–e) are from the jointing, booting, heading, filling, and maturing stages, respectively.

As shown in , the R2 values between the LAI of the five growth stages of winter wheat (the jointing, booting, heading, filling, and maturing stages) inverted by the AEIM-MWCM model and the measured LAI were 0.8518, 0.8551, 0.8643, 0.8531, and 0.8609, and the RMSE values were 0.3924, 0.3762, 0.3869, 0.3989, and 0.3864, respectively. The comprehensive analysis results in showed that although the correlation of each growth stage was different, the R2 was over 0.85, and the maximum RMSE was 0.3989, indicating that the LAI inversion of winter wheat in the five growth stages performed by the AEIM-MWCM reflected the growth and changes in winter wheat with preferable inversion results. The proposed model that combined the AEIM and MWCM models performed well and was feasible and applicable for regional winter wheat LAI inversion.
3.7. AEIM-MWCM cross validation
To further verify the accuracy of the inversion model, LAI images of the five typical growth stages of winter wheat obtained by inversion were resampled to 500 km, and 100 points were randomly selected. The LAI (GF-3 LAI) of the five growth stages of winter wheat obtained from the GF-3 remote sensing images and the MODIS LAI products (MOD15A2) of the corresponding phases were regressed and analyzed to obtain the correlation of the five growth stages of winter wheat, as shown in .
Figure 8. Correlation analysis between GF-3 LAI and MODIS LAI. (a–e) are from the jointing, booting, heading, filling, and maturing stages, respectively.

As shown in , the MODIS LAI and GF-3 LAI were strongly correlated in the five typical growth stages of winter wheat. Their correlation was the strongest at the jointing stage, with R2 = 0.8658 and RMSE = 0.3910, and it was followed by those of the heading (R2 = 0.8595 and RMSE = 0.3998), booting (R2 = 0.8479 and RMSE = 0.4318), filling (R2 = 0.8393 and RMSE = 0.4211), and maturing stages (R2 = 0.8374 and RMSE = 0.4236). According to the statistics of the mean values of 100 sampling points, the mean values of GF-3 LAI and MODIS LAI at the jointing stage were 1.45 and 1.25, respectively. At the booting stage, the mean value of the GF-3 LAI was 2.68, and the mean value of the MODIS LAI was 2.54. The mean LAI values of the GF-3 and MODIS at the heading stage were 4.43 and 4.21, respectively. The mean value of the GF-3 LAI was 2.65, and the mean value of the MODIS LAI was 2.59 at the filling stage. The mean LAI values of the GF-3 and MODIS at the maturing stage were 1.31 and 1.14, respectively. The LAI of winter wheat at the five growth stages obtained by the MODIS was lower than the GF-3 LAI, which was related to the low spatial resolution of the MODIS and serious pixel mixing. The phenomenon of over- or under-estimation found in this study has been confirmed in other studies (Li et al. Citation2021).
3.8. Mapping of LAI inversion by AEIM-MWCM
Based on the verified reliability of each inversion model, the AEIM-MWCM was employed to generate winter wheat LAI spatial distribution maps for the five growth stages, as shown in .
Figure 9. LAI images inverted by AEIM-MWCM. (a–e) are from the jointing, booting, heading, filling, and maturing stages, respectively.

The investigation results revealed that winter wheat in the test region was mainly distributed in the central areas. As shown in (a)–9(e), the non-vegetated area was red, and the vegetated area was colored. The LAI of winter wheat at the jointing stage was between 1 and 2, with an average value of 1.67. The larger LAI values of winter wheat were mainly concentrated in the central area, which was caused by the earlier sowing of winter wheat in this region. The LAI values of winter wheat at the booting, heading, and filling stages were 2.3–2.5, 2.9–4.3, and 2.5–3, with average values of 2.39, 3.94, and 2.52, respectively. Meanwhile, the larger LAI was mainly concentrated in the central area, whereas the LAI of winter wheat in the southwest area was generally smaller. The LAI value of winter wheat in the maturation stage was between 1 and 1.5, with an average value of 1.12. The LAI of winter wheat was mainly concentrated in the south. In summary, the LAI results of winter wheat in the five growth stages obtained by the AEIM-MWCM model were consistent with the local winter wheat growth status and situation.
However, there are several limitations in the application of the proposed model. For instance, in this study, the parameter calibration process is performed manually, so the obtained parameters might not be optimal. In the future, the automatic parameter optimization effect could be strengthened through a combination of different global optimization algorithms.
4. Conclusions
In this study, the AIEM-MWCM model is used to invert the LAI of winter wheat in the Xiangfu area east of Kaifeng City, Henan Province, during the entire growth cycle. The results indicate that the improved SAR-BM3D filtering method reduces the loss of detail information caused by the excessive smoothing of SAR images. The results also indicate that in the RVIFreeman model, the problem of vegetation index saturation can be overcome to a certain extent by the penetration of radar. The results also show that the MWCM has the potential to provide improvements in the LAI estimation and discrimination of the scattering response from vegetation and bare soil. In addition, it is shown that the modeled backscattering coefficient calculated by the MWCM is better than that obtained by the WCM. Therefore, the MWCM can be successfully used to estimate the LAI of wheat crops. The AIEM-MWCM model has higher fitting accuracy than the Dubois-MWCM model in inverting the LAI of winter wheat during the entire growth cycle, with R2 higher than 0.8 and RMSE lower than 0.22, which corresponds to the actual growth status of local winter wheat. The experiments in this paper are based on limited field measurement data in a single study area, resulting in some limitations in the model and conclusions. It is necessary to apply the model to different regions, expand the sample data, and conduct repeated verification experiments in subsequent studies.
Acknowledgement
We appreciate the detailed suggestions and comments of anonymous reviewers. The authors thank Guosheng Cai, Yushi Zhou, and Qinggang Zhang for assistance with flight tests and the other members of the Institute of Remote Sensing and Surveying and Mapping for assistance with LAI measurements.
Disclosure statement
No potential conflict of interest was reported by the author(s).
Data availability statement
The code used in this study are available by contacting the corresponding author.
Additional information
Funding
References
- Amin, E., V. Jochem, J. P. Rivera-Caicedo, L. Pipia, A. Ruiz-Verdú, and J. Moreno. 2021. “Prototyping Sentinel-2 Green LAI and Brown LAI Products for Cropland Monitoring.” Remote Sensing of Environment 255: 112168. https://doi.org/10.1016/j.rse.2020.112168.
- Anderson, M. C., C. M. U. Neale, F. Li, J. M. Norman, W. P. Kustas, H. Jayanthi, and J. Chavez. 2004. “Upscaling Ground Observations of Vegetation Water Content, Canopy Height, and Leaf Area Index During SMEX02 Using Aircraft and Landsat Imagery.” Remote Sensing of Environment 92 (4): 447–464. https://doi.org/10.1016/j.rse.2004.03.019.
- Attema, E. P. W., and F. T. Ulaby. 1978. “Vegetation Modeled as a Water Cloud.” Radio Science 13: 357–364. https://doi.org/10.1029/rs013i002p00357.
- Dubois, P. C., V. Z. Jakobl, and T. Engman. 1995. “Measuring Soil Moisture with Imaging Radars.” IEEE Transactions on Geoscience and Remote Sensing 33 (4): 915–926. https://doi.org/10.1109/36.406677.
- Emilie, B., W. François, C. François, B. Patrick, and D. Pierre. 2015. “Maize Leaf Area Index Retrieval from Synthetic Quad pol SAR Time Series Using the Water Cloud Model.” Remote Sensing 7: 16204–16225. https://doi.org/10.3390/rs71215818.
- Gao, S., Z. Niu, and C. Y. Wu. 2010. “Multi-polarization EnvisatASAR Images as a Function of Leaf Area Index (LAI) of White Poplar and Desert Date Plantations.” International Journal of Remote Sensing 31 (4): 1095–1102. https://doi.org/10.1080/01431160903283827.
- Giovanni , C., E. G. Mireille, S. Giuseppe, and V. Luisa. 2017. “Multitemporal SAR Image Despeckling Based on Block-Matching and Collaborative Filtering.” IEEE Transactions on Geoscience and Remote Sensing 55 (10): 5467–5480. https://doi.org/10.1109/TGRS.2017.2707806.
- Inge, J., F. Stefan, N. Kris, M. Bart, C. Pol, W. Marie, and B. Frédéric. 2004. “Review of Methods for in Situ Leaf Area Index Determination: Part I. Theories, Sensors and Hemispherical Photography.” Agricultural & Forest Meteorology 121 (1–2): 19–35. https://doi.org/10.1016/j.agrformet.2003.08.027.
- Jafari, M., and A. Keshavarz. 2021. “Improving CERES-Wheat Yield Forecasts by Assimilating Dynamic Landsat-Based Leaf Area Index: A Case Study in Iran.” Journal of the Indian Society of Remote Sensing, 1–14. https://doi.org/10.1007/s12524-021-01359-w.
- Jarlan, L., G. Balsamo, S. Lafont, A. Beljaars, J. C. Calvet, and E. Mougin. 2008. “Analysis of Leaf Area Index in the ECMWF Land Surface Model and Impact on Latent Heat and Carbon Fluxes: Application to West Africa.” Journal of Geophysical Research: Atmospheres 113 (24): 1–22. https://doi.org/10.1029/2007JD009370.
- Kurosu, T., M. Fujita, and K. Chibae. 1995. “Monitoring of Rice Crop Growth from Space Using the ERS-1 C-Band SAR.” IEEE Transactions on Geoscience and Remote Sensing 33 (4): 1092–1096. https://doi.org/10.1109/36.406698.
- Li, X. J., H.Q Du, G. M. Zhou, F. J. Mao, M. Zhang, N. Han, W. L. Fan, et al. 2021. “Phenology Estimation of Subtropical Bamboo Forests Based on Assimilated MODIS LAI Time Series Data.” ISPRS Journal of Photogrammetry and Remote Sensing 173: 262–277. https://doi.org/10.1016/j.isprsjprs.2021.01.018.
- Mandal, D., M. Hosseini, H. McNairn, V. Kumar, A. Bhattacharya, Y. S. Rao, S. Mitchell, L. D. Robertson, A. Davidson, and K. Dabrowska-Zielinska. 2019. “An Investigation of Inversion Methodologies to Retrieve the Leaf Area Index of Corn from C-Band SAR Data.” International Journal of Applied Earth Observation and Geoinformation 82: 101893. https://doi.org/10.1016/j.jag.2019.06.003.
- Mansaray, L. R., D. Zhang, Z. Zhou, and J. Huang. 2017. “Evaluating the Potential of Temporal Sentinel-1A Data for Paddy Rice Discrimination at Local Scales.” Remote Sensing Letters 8: 967–976. https://doi.org/10.1080/2150704X.2017.1331472.
- Mehdi, H., M. N. Heather, M. Amine, and P. Anna. 2015. “Estimation of Leaf Area Index (LAI) in Corn and Soybeans Using Multi-Polarization C-and L-Band Radar Data.” Remote Sensing of Environment 170: 77–89. https://doi.org/10.1016/j.rse.2015.09.002.
- Pauline , D., C. Thomas, H. M. Laurence, and C. Samuel. 2014. “Combined use of Multi-Temporal Optical and Radar Satellite Images for Grassland Monitoring.” Remote Sensing 6 (7): 6163–6182. https://doi.org/10.3390/rs6076163.
- Ren, Z. B., Y. X. Du, X. Y. He, R. L. Pu, H. F. Zheng, and H. D. Hu. 2018. “Spatiotemporal Pattern of Urban Forest Leaf Area Index in Response to Rapid Urbanization and Urban Greening.” Journal of Forestry Research 29 (03): 785–796. https://doi.org/10.1007/s11676-017-0480-x.
- Shubham, K. S., P. Rajendra, K. S. Prashant, A. Y. Suraj, P. Y. Vijay, and S. Jyoti. 2022. “Incorporation of First-Order Backscattered Power in Water Cloud Model for Improving the Leaf Area Index and Soil Moisture Retrieval Using Dual-Polarized Sentinel-1 SAR Data.” Remote Sensing of Environment, https://doi.org/10.1016/J.RSE.2023.113756.
- Sonia, A., N. Andrew, de B. Kees, S. Andrew, L. Alice, M. Aileen, and J. P. Q. Eduardo. 2019. “Relating X-Band SAR Backscattering to Leaf Area Index of Rice in Different Phenological Phases.” Remote Sensing 11 (12): 1462. https://doi.org/10.3390/rs11121462.
- Tao, L., J. Li, J. Jiang, and X. Chen. 2016. “Leaf Area Index Inversion of Winter Wheat Using Modified Water-Cloud Model.” IEEE Geoscience & Remote Sensing Letters 13 (6): 816–820. https://doi.org/10.1109/LGRS.2016.2546945.
- Toan, T. L., H. Laur, E. Mougin, and A. Lopes. 1989. “Multitemporal and Dual-Polarization Observations of Agricultural Vegetation Covers by X-Band SAR Images.” IEEE Transactions on Geoscience and Remote Sensing 27: 709–718. https://doi.org/10.1109/TGRS.1989.1398243.
- Verma, B., R. Prasad, P. K. Srivastava, S. A. Yadav, P. Singh, and R. K. Singh. 2022. “Investigation of Optimal Vegetation Indices for Retrieval of Leaf Chlorophyll and Leaf Area Index Using Enhanced Learning Algorithms.” Computers and Electronics in Agriculture 192: 106581. https://doi.org/10.1016/j.compag.2021.106581.
- Wang, Y. B., Y. H. Wang, Z. H. Li, P. T. Yu, and X. S. Han. 2020. “Interannual Variation of Transpiration and its Modeling of a Larch Plantation in Semiarid Northwest China.” Forests 11 (12): 1303. https://doi.org/10.3390/f11121303.
- Wu, T. D., K. S. Chen, J. C. Shi, and A. K. Fung. 2001. “A Transitio Model for the Reflection Coefficient in Surface Scattering.” IEEE Transactions on Geoscience and Remote Sensing 39 (9): 2040–2050. https://doi.org/10.1109/36.951094.
- Yadav, V. P., R. Prasad, and R. Bala. 2021. “Leaf Area Index Estimation of Wheat Crop Using Modified Water Cloud Model from the Time-Series SAR and Optical Satellite Data.” Geocarto International 36: 791–802. https://doi.org/10.1080/10106049.2019.1624984.
- Yan, G. J., R. H. Hu, J. H. Luo, M. Weiss, H. L. Jing, X. H. Mu, D. H. Xie, and W. M. Zhang. 2019. “Review of Indirect Optical Measurements of Leaf Area Index: Recent Advances, Challenges, and Perspectives.” Agricultural and Forest Meteorology 265 (15): 390–411. https://doi.org/10.1016/j.agrformet.2018.11.033.
- Yang, Z., K. Li, Y. Shao, B. Brisco, and L. Liu. 2016. “Estimation of Paddy Rice Variables with a Modified Water Cloud Model and Improved Polarimetric Decomposition Using Multi-Temporal RADARSAT-2 Images.” Remote Sensing 8 (10): 878. https://doi.org/10.3390/rs8100878.
- Zhang, L. L., Q. Y. Meng, S. Yao, Q. Wang, J. Y. Zeng, S. H. Zhao, and J. W. Ma. 2018. “Soil Moisture Retrieval from the Chinese GF-3 Satellite and Optical Data Over Agricultural Fields.” Sensors 18 (8): 2675. https://doi.org/10.3390/s18082675.