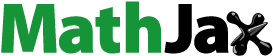
ABSTRACT
Land subsidence, a common geological phenomenon in deltaic regions, poses significant risks to infrastructures, environments, and human lives. Monitoring and understanding land subsidence are crucial for establishing resilient, adaptive, and sustainable environments. In this study, a robust multi-temporal interferometric synthetic aperture radar (MTInSAR) method was employed to monitor land subsidence in the Guangdong-Hong Kong-Macao Greater Bay Area (GBA) using 541 Sentinel-1 SAR images. Spatial auto- and cross-correlation analysis were applied to select the most reasonable explanatory variables from nine candidates in each city of the GBA. City-level geographically weighted regression (GWR) models were then constructed to explore the spatially nonstationary effects of natural and human-induced factors. The findings revealed subsidence velocities ranging from 0 to 83.7 mm/yr in the GBA from 2015 to 2021, with the dominant factors affecting subsidence varying among regions. Natural and human-related factors accounted for 51.13% and 48.87%, respectively. Further analysis of representative subsidence areas highlights the importance of continuous monitoring of ground deformation using MTInSAR, region-specific land subsidence mitigation strategies, and regular maintenance of urban infrastructure. These insights are valuable for policymakers and urban planners in comprehending the complex processes underlying land subsidence and informing decision-making processes for sustainable and resilient urban development.
1. Introduction
Land subsidence, the sinking or settling of the ground surface, is a geological phenomenon that has affected more than 200 sites in 34 countries over the past century (Bagheri-Gavkosh et al. Citation2021; Herrera-García et al. Citation2021). This process is particularly prevalent in densely populated deltaic regions (Salimi and Al-Ghamdi Citation2020; Shirzaei et al. Citation2021; Shupeng and van Genderen Citation2008). Land subsidence can be caused by various factors, including natural or human-induced causes. Natural factors, such as sedimentary deposit consolidation, occur over long periods of time and can affect large areas (An et al. Citation2023; Sarah et al. Citation2020; Teatini, Tosi, and Strozzi Citation2011). Conversely, human activities like groundwater extraction and ground or underground construction can exacerbate or accelerate the subsidence process, leading to localized geohazards (Shi et al. Citation2022). Land subsidence can also amplify sea level rise, which may increase coastal flooding (Costa, Burlando, and Priadi Citation2016; Lyu et al. Citation2019). Therefore, continuous monitoring of deltaic subsidence and an improved understanding of the driving mechanisms are necessary for policy decisions and flood-resilience plans.
Interferometric synthetic aperture radar (InSAR) is a powerful technology for the continuous monitoring of land subsidence, providing all-weather and all-day monitoring with unprecedented spatial coverage and precision levels (Bürgmann, Rosen, and Fielding Citation2000; Rosen et al. Citation2000). Conventional differential InSAR measures deformation from interferograms by removing topographic contributions using two or three images. However, these methods may suffer from serious errors due to atmospheric delay and spatial–temporal decorrelation effects (Hanssen Citation2001). Advanced multi-temporal InSAR (MTInSAR) can mitigate these errors by identifying coherent points from usually more than 25 SAR images. MTInSAR is capable of achieving high spatial resolutions and deformation accuracy ranging from centimeter to millimeter (Crosetto et al. Citation2016). The two generations of MTInSAR are persistent scatterer (PS) InSAR (PSInSAR) and distributed scatterer (DS) InSAR (DSInSAR). PSInSAR, as proposed by Ferretti, Prati, and Rocca (Citation2001), can identify high-coherence PSs in urban areas, including structures constructed by humans and bare rocks. DSInSAR, on the other hand, was designed to extract DSs like pavement and vegetation (Ferretti et al. Citation2011; Lanari et al. Citation2004). DSInSAR increases the measurement points by 5–10 times and can extract more deformation details compared to PSInSAR.
As InSAR technologies continue to advance, numerous studies have been conducted globally to gain insights into land subsidence in deltaic regions. For instance, RadarSat images revealed that New Orleans in the Mississippi River delta underwent rapid subsidence with a maximum velocity of 33 mm/yr from 2002 to 2005 (Dokka, Sella, and Dixon Citation2006). In the Mekong Delta, Vietnam, groundwater extraction caused subsidence of up to 3 cm/yr between 2007–2010, which is consistent with the results of the transient 3D aquifer simulation (Erban et al. Citation2013). Higgins et al. (Citation2014) studied land subsidence in the Ganges-Brahmaputra Delta using ALOS data and concluded that subsidence was predominantly regulated by local stratigraphy, and its rates varied greater than an order of magnitude determined by the lithology. Li et al. (Citation2020) and Ng et al. (Citation2018) analyzed land subsidence due to anthropogenic activities, such as groundwater extraction and reclamation, in the Pearl River Delta (PRD) using ENVISAT and COSMO-SkyMed images. Surface deformation along the metro network caused by tunneling works was reported in the central city of the Yangtze Delta-Shanghai using TerraSAR-X data (Yang et al. Citation2022). Other deltaic areas including the Fraser River Delta (Mazzotti et al. Citation2009), Yellow River Delta (Higgins et al. Citation2013), river deltas in Indonesia (Chaussard et al. Citation2013), and Nile Delta (Gebremichael et al. Citation2018), have also been investigated using InSAR technologies.
Previous studies have investigated the magnitude, distribution, and causes of land subsidence in deltaic regions, but have often relied on models that assume a constant relationship between explanatory factors and land subsidence across all locations (Du et al. Citation2020; Ma et al. Citation2019; Minderhoud et al. Citation2018). However, in reality, the relationships between these factors and land subsidence can vary significantly from place to place, and ignoring this spatial nonstationarity can lead to stationarity bias in spatial relationship modeling and hinder our understanding of the local mechanisms driving subsidence (Jendryke and McClure Citation2021; Jing et al. Citation2023; Kwan Citation2021). To overcome this limitation, researchers have developed spatial regression models like geographically weighted regression (GWR), which can estimate the spatially varying effects of factors by constructing a local regression for each location in the study area (Fotheringham, Brunsdon, and Charlton Citation2003). For instance, Yu et al. (Citation2020) employed GWR to analyze the nonstationary relationship between land subsidence and groundwater aquifers level changes in Beijing, while a GWR model was also used in Wuhan to explore the strengths and directions of the impacts of urbanization on land subsidence (Wang et al. Citation2022).
While these studies have harnessed GWR models to decipher nonstationary relationships, they often narrow their focus to particular factors. Our research uniquely marries robust MTInSAR with GWR. This combination facilitates accurate surface deformation velocities over extensive periods and large areas, a challenge for either method alone. Furthermore, our method goes beyond simply identifying the factors; it carefully distinguishes and explores the impacts of both natural and anthropogenic factors on subsidence. This comprehensive approach not only allows for a more detailed understanding of the deltaic subsidence mechanisms but also ensures that our model is resistant to the stationarity bias observed in previous research.
Specifically, we generated land deformation velocity in the GBA using a robust MTInSAR method, which identified both Persistent Scatterer (PS) and Distributed Scatterer (DS) points to improve accuracy. To ensure reliability, the MTInSAR results were validated with leveling data. Spatial auto- and cross-correlation analyses were then conducted using both univariate and bivariate Moran’s I indices to investigate the relationships between land subsidence and all candidate influencing factors, and to identify the most reasonable explanatory variables for subsidence in each city of the GBA. Subsequently, GWR models were constructed at the city level to explore the spatially nonstationary effects of natural and human-induced factors on subsidence. Based on the GWR results, we analyzed the dominant factors influencing land subsidence. Finally, we conducted comparisons with the traditional linear method and performed further analysis in representative subsidence areas to provide suggestions and conclusions for policymakers and urban planners.
2. Study area and dataset
2.1. Study area
The GBA, encompassing nine cities in Guangdong Province and two special administrative regions (Hong Kong and Macao), represents one of the largest river deltas globally. It is located in the coastal area of South China and has a latitude and longitude range of 21°32′ to 24°26′N and 111°20′ to 115°24′E as depicted in . The topography of the GBA generally descends from north to south, comprising several characteristic landforms, such as hills, monadnocks, platforms, and plains (Wei and Wu Citation2011). The geological composition of the region is primarily composed of bedrock, hidden karst formations, as well as Quaternary loose soil and soft soil, with the latter two forming extensive alluvial sedimentary layers throughout the flat terrain and along the river within the GBA (Tang et al. Citation2010). The thickness of these sediment layers ranges from 10 to 40 m, creating a high potential for land subsidence.
Figure 1. Geographical location of the study area. The background displays digital elevation models from the Shuttle Radar Topography Mission, along with optical images sourced from Google Earth.

The GBA is among the world’s four largest bay-area urban agglomerations, with a resident population of approximately 70 million in 2018 and a population density of approximately 1250 individuals per square kilometer (Yang, Zhou, and Zhang Citation2022; Zheng et al. Citation2022). Rapid population and economic growth have led to the creation of new lands through coastal reclamation, which are susceptible to subsidence due to sedimentary consolidation (Nicholls Citation1995). Urban development, transportation construction, and industrial activities have contributed significantly to accelerated land subsidence (Ezquerro et al. Citation2014; Halder, Bandyopadhyay, and Banik Citation2021). Landslides are also common in the region, given its complex geological settings, heavy precipitation, especially during typhoons, and intense construction activities (Ouyang et al. Citation2017).
2.2. Data
2.2.1. Sentinel-1 SAR data
The velocity maps of land subsidence and corresponding deformation time-series for the GBA were generated through the utilization of multi-temporal SAR data sourced from the S1 satellite, operating at C-band frequency (as shown in ). The dataset employed consists of 541 SAR images acquired in three ascending tracks, spanning from June 2015 to December 2021. The terrain observation with progressive scans mode was utilized by the S1 satellite at an incidence angle of 39.7° and a wavelength of 5.5 cm (De Zan and Monti Guarnieri Citation2006). It achieves a coverage of 48,000 km2 and a resolution of 5 m × 20 m.
Table 1. Acquisition parameters of S1.
To eliminate terrain phase effects in differential interferometry, we used an external digital elevation model (DEM) dataset sourced from the Shuttle Radar Topography Mission (SRTM) (Farr et al. Citation2007). The spatial resolution of the globally available dataset is 30 m. Moreover, for subsidence monitoring validation, leveling data was collected from the Hong Kong International Airport (HKIA).
2.2.2. Nature-related data
Five types of nature-related data were used in this study, namely Quaternary sediment thickness (ST), digital elevation model (DEM), slope (S), topographic wetness index (TWI), and soil moisture (SM) (). Quaternary sediment thickness, which was sourced from the Guangzhou Institute of Geography (GIG), is a key indicator of the geological conditions in the GBA. Thicker sediments often result in larger subsidence due to natural consolidation and groundwater extraction. We also incorporated detailed hydrogeological data from the GIG, focusing on typical subsidence areas, to foster a deeper analysis and understanding of the subsidence phenomena observed. Additionally, topographic datasets including DEM and its secondary topographic variables, including S and TWI, were acquired from the SRTM to investigate their correlation with the landslide movements (Roy et al. Citation2022). Soil moisture data obtained from (Meng et al. Citation2021) was used to characterize terrestrial hydrological systems and evaluate how climatic factors affect land subsidence. The correlation between underground water, topographic wetness index, and soil moisture is synergistic, where changes in groundwater levels directly affect soil moisture, consequently influencing the topographic wetness index and impacting land subsidence patterns (El Ayady et al. Citation2023; Fildes et al. Citation2020).
2.2.3. Human-related data
To analyze the impact of human activities on land subsidence, demographic statistics such as population density (PD) and remote sensing products that measure urbanization levels were collected. These products include night-time light (NTL) satellite images, impervious surface area (ISA), and road density (RD) (). Population density data at the district level for eleven GBA cities were obtained from official statistical yearbooks, which is a useful indicator of the intensity of human activity and urbanization (Huang et al. Citation2021). NTL images were obtained from the global NPP-VIIRS-like NTL data time series (2000-2021), which provides a unique proxy measure for unveiling urbanization and regional development (Du et al. Citation2021; Xu et al. Citation2022). ISA was extracted from the global 30m impervious-surface dynamic dataset (GISD30) and is a common indicator of urbanization (Mathew, Khandelwal, and Kaul Citation2016). RD was generated based on Open Street Map road network data, and the road length was divided by the unit area to parallel the intensity of urbanization.
All the collected data were transformed into the UTM-WGS84 50N projection. The output resolution of the deformation map generated by InSAR was set at 500 × 500 m. In order to ensure conformity of it, all other data were resampled.
3. Method
The study employed a combination of InSAR and multi-source nature- and human-related data to investigate the spatial pattern of land subsidence in the GBA and the spatially nonstationary effects of several explanatory factors on land subsidence using MTInSAR and city-level GWR models (refer to ). The method can be divided into two main parts. In the first part, a robust MTInSAR approach was developed to generate a deformation velocity map for the period from 2015 to 2021 by jointly identifying PS and DS points. In the second part, a spatial analysis combined with multi-source data was conducted. Prior to the analysis, univariate and bivariate Moran’s I tests were applied to remove randomly distributed factors and identify reasonable explanatory factors at the city level, separately. Then, the GWR models were constructed in each city to explore the spatially nonstationary relationships between the subsidence and selected explanatory variables. Finally, the spatial pattern derived from the GWR was analyzed and verified, and the dominant factor affecting the subsidence of each location was determined.
Figure 4. Overall workflow. The philosophy of the GWR model originates from (Fotheringham, Brunsdon, and Charlton Citation2003)

3.1. Land subsidence estimation with a robust MTInSAR method
In this study, a robust MTInSAR method was employed to map surface deformation in the GBA. A total of 541 SAR images were collected, with one image selected as the main image and the remaining 540 as secondary ones. Co-registration of the auxiliary images with the main image was carried out. Consequently, differential interference processing was conducted with a multi-look of 8 × 2 and the incorporation of external SRTM DEM data. Atmospheric stratification effects were addressed using the Generic Atmospheric Correction Online Service (GOCAS) data.
A two-tier network was devised for identifying PS and DS points without the need for preliminary atmospheric removal across the study region (Ma and Lin Citation2015). The first step in identifying PS involves extracting PS candidates by applying a threshold of 0.3 to the amplitude dispersion value and connecting them in a densified Delaunay network. The signal model for the analysis can be written as:
(1)
(1) where
is the differential interferogram vector,
is the SAR image count,
is the reconstructed backscattering, and
represents the sensing matrix that includes the height and deformation velocity to be estimated. This model is used to estimate the parameters of the PS points and is a fundamental component of the PS analysis methodology. A joint application of beamforming and the robust M-estimator was used for parameter estimation. If the temporal coherence (PSt1) of the PS candidates exceeded a threshold of 0.72, the estimates of height and velocity were obtained, and the arc was retained. The most stable PS points detected in the first-tier network were then utilized as reference points to extend the remaining PS and DS points in the second-tier network. To extend the point coverage in all directions, an omnidirectional approach was adopted, and Coherence-Weighted Phase-Linking was utilized to recover the optimal phase. Thresholding values of PSt2 = 0.7 and DSt2 = 0.65 were applied to detect additional PSs and DSs, respectively. The thresholds were determined based on previous studies and empirical tests that optimized the balance between reliability and point quantity (Ma et al. Citation2019; Wu et al. Citation2023).
For each PS and DS point, both line-of-sight (LOS) subsidence velocity and temporal deformation were computed. In areas with steep terrain, the LOS deformation was transformed into the downward direction by taking into account the slope gradient, while for other points, the LOS deformation was transformed to vertical ground subsidence using the incidence angle. Finally, the obtained results were used to monitor and evaluate the ground deformation and potential geohazards in the GBA.
3.2. Variable selection based on spatial auto- and cross-correlation analysis
To identify the reasonable explanatory variables for further regression analysis, we conducted a spatial auto-correlation test on both the InSAR deformation velocity map and each candidate explanatory variable. The univariate Moran’s I statistic was conducted at the city level to measure the spatial auto-correlation of each variable. We then removed variables that are randomly distributed because they cannot form meaningful associations with land subsidence. The Moran’s I statistic is defined as follows:
(2)
(2) where
is the total number of spatial units,
is the spatial weight between the
-th and
-th spatial units,
represents the deviation of variable observations from the mean on the
-th spatial unit. Moran’s I value varies between −1 and 1, where a positive value signifies the presence of spatial auto-correlation between geographic samples. When Moran’s I is less than or equal to zero, no spatial auto-correlation exists and we excluded the variable from the subsequent analysis.
Furthermore, we employed the bivariate Moran’s I index at the city level to measure the spatial cross-correlation between land subsidence and each explanatory variable. Explanatory variables with statistically insignificant cross-correlation were considered irrelevant to land subsidence and excluded from the subsequent regression analysis. The formula for the bivariate Moran’s I is as follows:
(3)
(3) where
represents the total number of spatial units;
denotes the spatial weight between the
-th and
-th spatial units,
represents the deviation of variable observations from the mean on the
-th spatial unit;
is analogous, but for the variable y on the j-th spatial unit. The local bivariate Moran’s I is the value calculated in each spatial unit, represented as:
(4)
(4) where z(s) is the standardized value of the variable x at the s-th spatial unit. The bivariate Moran’s I index also yields between −1 and 1, with a positive value implying a positive spatial correlation and a negative value denoting a negative spatial correlation between the two variables. When the bivariate Moran’s I index equals 0, the explanatory variable is abandoned as it signifies a random spatial pattern. As the univariate and bivariate Moran’s I indexes were conducted separately at the city level, the variables selected for further regression analysis may vary across the cities.
3.3. Spatial analysis using the GWR model for determining dominant factors
In the first step, the selected explanatory variables were assessed using the variance inflation factor (VIF) as a measure of the extent to which multicollinearity among explanatory variables increases the variance of an estimated regression coefficient (Craney and Surles Citation2002). In our study, we used a VIF threshold of 5 to identify variables with strong multicollinearity, which were excluded from subsequent analyses.
Based on the selected variables, we performed the GWR model on 11 cities respectively to further explore the spatial variability in the effects of the explanatory factors on land subsidence. The GWR model accounts for spatial non-stationarity by introducing the spatial position into the regression coefficients, which gives a local estimator of the regression function at each geographic location. Specifically, the model is defined as:
(5)
(5) where
represents the fitted land subsidence velocity for the
-th spatial unit,
is the geographic coordinates of the unit’s center point,
is the estimated constant term,
is the value of the
-th explanatory variable,
is the regression parameter estimate associated with
, which is a function of geographic location, and
is the residual error. The regression parameter
of the
-th spatial unit is calculated as follows:
(6)
(6) where
is a diagonal matrix with elements monotonically decreasing according to the distance between the
-th spatial unit and other spatial units.
In the constructed GWR models, the magnitude of the regression coefficient of each explanatory factor differs. The identification of the dominant factor at each location is determined through a comparative analysis of the absolute values of the regression coefficients corresponding to each explanatory factor. The factor with the highest absolute value is thus recognized as the dominant influence in that specific area.
4. Results
4.1. InSAR measurements and validation
Using the proposed MTInSAR method, we processed 541 S1 images collected between 2015–2021 to generate land deformation velocity for the GBA, as depicted in . The reference point, located in the presumed stable region, is marked by the black cross. Positive values with blue color correspond to ground uplift and noise, while negative values with red color represent ground subsidence and noise. In cases where ground uplift is negligible, positive values can be used to represent the noise level. We determined the standard deviation of the noise level to be 1.9 mm/yr.
Figure 5. InSAR deformation velocity map of the GBA. The orange rectangle indicates the location of . Two yellow circles indicate the location of .

A total of 25, 735, 358 measurement points were generated during the acquisition time of the S1 data. The subsidence velocity ranged from 0 to 83.7 mm/yr, with the highest rate of 83.7 mm/yr observed in Zhuhai, marked by the orange triangle. Widespread subsidence was observed in the western part of the PRD, with three identified subsidence areas, namely Jiangmen, Zhuhai-Zhongshan, and Guangzhou-Zhongshan, exhibiting an average subsidence velocity of 1.5 mm/yr.
To validate the derived subsidence velocity, we compared the InSAR measurements in the HKIA with geodetic leveling data for 100 benchmarks obtained from the Hong Kong Airport Authority. a shows the position of the leveling points. We selected InSAR points around the leveling benchmarks within a 23 m distance for comparison. The mean absolute error of deformation velocity is 2.91 mm/yr, and the standard deviation is 1.5 mm/yr. b presents the correlation between InSAR deformation velocity and ground deformation velocity calculated from leveling data, indicating an R2 value of 0.87. The findings suggested good agreement between the InSAR measurements and the leveling data, although errors may arise due to atmospheric delay, temporal decorrelation, and differences in position and time between InSAR and leveling measurements (Ding et al. Citation2004). We further illustrated the time-series deformation obtained by InSAR at two leveling points, P1 and P2 in c. The results showed good agreement between InSAR and leveling data. The deformation trend observed at P1 indicates a deceleration, which is in agreement with the compaction mechanism of reclaimed land (Kim et al. Citation2010).
4.2. Spatial auto- and cross-correlation results of candidate explanatory variables
We employed the univariate Moran’s I statistic to analyze the spatial auto-correlation of land subsidence and all candidate explanatory factors in each city of the GBA. Results from this analysis, as depicted in , indicate positive spatial auto-correlation of all candidate factors, including land subsidence (LS), the response variable, with Moran’s I value ranging from 0.36–0.88 across cities. The highest value is observed in Zhuhai (ZH) and the lowest is in Shenzhen (SZ). Despite these observed variations, all factors exhibited statistically significant spatial autocorrelation with p-values less than the threshold of 0.05, a convention frequently adopted to eliminate spurious associations resulting from random factor distributions (Fisher Citation1956). As shown in the first four rows of (a), the levels of spatial autocorrelation for the four human-related factors were relatively similar across cities, night-time light (NTL) exhibited the strongest spatial autocorrelation, road density (RD) showed the weakest, and impervious surface area (ISA) and population density (PD) displayed moderate levels. For the five natural factors considered, soil moisture (SM), DEM, and sediment thickness (ST) exhibited relatively strong spatial autocorrelation, followed by slope (S) and topographic wetness index (TWI). It is worth noting that the magnitude of spatial autocorrelation for each factor may vary significantly across cities. For example, ST showed the highest Moran’s I of 0.93 in Foshan (FS) but the smallest of 0.27 in Jiangmen (JM). Despite these variations, all factors were demonstrated to have statistically significant spatial autocorrelation with a p-value less than 0.05, which can effectively eliminate spurious associations resulting from a random distribution of factors in subsequent analysis.
Figure 7. (a) Spatial auto-correlation of land subsidence and candidate explanatory factors for each city in the GBA. (b) Spatial cross-correlation between land subsidence and candidate explanatory factors for each city in the GBA.

To identify appropriate explanatory variables for GWR analysis, we conducted an exploratory analysis using bivariate Moran’s I to examine the cross-correlation between LS and each candidate influencing factors in each city. (b) illustrates the diverse forms of cross-correlation, including statistically significant positive (red) and negative (blue) correlations as well as insignificant correlations (white). A factor with an insignificant cross-correlation with LS implies that it is not an important influence in causing subsidence in a given city and therefore was removed from the subsequent GWR analysis. Such factors include RD in Shenzhen (SZ), NTL in Guangzhou (GZ) and Dongguan (DG), and ST in Hong Kong-Macao (HM), Huizhou (HZ), and Zhaoqing (ZQ). For the other factors considered, the form and magnitude of their relationships with SL were not always consistent between cities. For natural factors, SM, TWI, and ST were found to have a negative effect on subsidence in most cities in GBA, with the most evidence for ST in Jiangmen (JM) and Zhuhai (ZH). This finding confirms that sediment consolidation is the primary cause of subsidence in the western alluvial plain (Wu et al. Citation2023). In comparison, S and DEM mostly showed a statistically significant positive correlation with SL, except in Shenzhen (SZ) where the correlation is negative. The significant differences in the forms, magnitudes, and spatial distributions of the cross-correlations between LS and candidate factors further highlight the importance of analyzing the potential causes of subsidence at the local level.
Further, we utilized the local bivariate Moran's I to analyze the cross-correlation between LS and candidate factors at a finer scale than the city level. For the nature-related data, a revealed a negative correlation between ST and LS predominantly in the western parts of the GBA, an area characterized by extensive soft soil distributions. In addition, DEM and S displayed a similar spatial pattern of positive cross-correlation with LS (b-c), especially pronounced in the central, western, and eastern areas of the GBA. e showed a positive relationship between LS and SM in the eastern GBA, while the negative correlation mostly exists in the central region. For the human-related data, there is a similar spatial distribution for PD, NTL and ISA and the positive relationship is mainly concentrated in the central and southern regions (f-h). What need to be concerned is that the phenomenon between b and c, and among f, g and h may indicate potential spatial collinearity between these variables. Further validation is essential to ascertain this and mitigate its possible influence on the explanatory power of GWR.
4.3. Spatially nonstationary relationships captured by GWR
visualizes the results of the locally weighted regression coefficients for the explanatory variables considered in the GWR for each city. The gray area represents the factor that is not included in the GWR model because it is either not correlated with subsidence or has a high degree of multicollinearity with other explanatory variables. The results showed that the relationship between each explanatory variable and land subsidence varied across different locations in the GBA. However, the spatial variability of regression coefficients for different factors may differ to some extent. For example, in a, although the strength of the relationship between ST and subsidence varied spatially, they maintained an overall negative relationship, implying that an increase in ST usually leads to a decrease in the values of subsidence (i.e. exacerbating land subsidence). Similarly, PD, NTL, and ISA show positive relationships with subsidence in most locations. In contrast, the relationships between other factors and subsidence exhibit more pronounced spatial variations. Taking slope (S) as an example, its regression coefficient varied significantly in space, reflecting its diverse and variable relationship with subsidence (c). Specifically, in the southern part of Guangzhou, the eastern part of Jiangmen, the northern part of Zhuhai, and the eastern part of Zhaoqing, slope showed a positive relationship with subsidence, while in other areas, a negative relationship is more prevalent.
Figure 9. Spatial distribution of the regression coefficients for each explanatory variable in the GWR model.

Subsequently, we determined the local dominant factors of land subsidence by identifying the factor with the maximum absolute regression coefficient at each location. As shown in , the proportion of natural and human factors identified as dominant factors are comparable, accounting for 51.12% and 48.88%, respectively. For nature factors, the most important factor responsible for subsidence is DEM, which accounts for 18.79% of dominant factors. Specifically, it contributes to 47.85% and 72.56% of impacts on subsidence in Huizhou and Shenzhen, respectively, located in the eastern and south-eastern parts of the GBA. Among the human-related factors, NTL has the largest proportion as the dominant factor contributing to subsidence, accounting for 27.86%. Its main impact area is in the western and eastern parts of GBA, including Zhaoqing and Huizhou. Their impact areas are scattered and sparsely distributed in almost every city.
5. Discussion
5.1. Comparison and validation of the GWR models
To assess the overall relationship between each variable and land subsidence, we calculated the proportion of negative and positive regression coefficients for each variable separately. The predominant proportion determined the mode of the variable in the GWR model, and we used ‘+’ and ‘-’ symbols to denote positive and negative relationships, respectively. When a variable was not included in the GWR models or had a p-value greater than 0.05 in the global bivariate Moran's I test, we indicated it with ‘0’. We cross-validated the GWR results with the global bivariate Moran’s I test, and the comparison is presented in .
Table 2. Correlation mode between land subsidence and explanatory variables in each city.
The comparison in suggests that the GWR results are generally consistent with the global bivariate Moran’s I result. However, there are a few variables that show opposite results between the two methods. For example, in Huizhou (HZ), the PD, ISA, and RD show a positive relationship in the GWR result, but a negative relationship in the global bivariate Moran's I result. These opposite results indicate that the relationship between the variables and land subsidence may vary within a city. The results also highlight the importance of using GWR analysis to capture the spatial variability of the relationships, as the global bivariate Moran's I test may not be sufficient for this purpose.
After conducting the cross-validation, we compared the R2 values of the Ordinary Least Square (OLS) and GWR models for each city. As shown in , the R2 values of the GWR models are generally higher than those of the OLS models, indicating that the GWR models were better suited to explain the spatial heterogeneity of land subsidence. Notably, the GWR models in Huizhou, Zhuhai, and Zhongshan demonstrated the highest R2 values, exceeding 0.5. These results suggest that the spatial variation of land subsidence in these cities was better explained by the GWR models. Additionally, the GWR results in Shenzhen, Hong Kong, Macao, and Foshan also showed notable improvements in R2 values compared to the OLS results (0.01). These findings highlight the importance of considering spatial heterogeneity in modeling land subsidence and suggest that GWR analysis can provide more accurate and reliable results than traditional OLS regression.
5.2. Typical natural-induced subsidence
To verify the results of the GWR analysis and gain further insight into the mechanisms behind natural-induced subsidence, we conducted further analysis on the western part of the PRD, which has been identified in previous studies as experiencing serious large-scale subsidence (Wang et al. Citation2012).
As shown in a, we identified three typical subsidence areas, including Jiangmen, Zhuhai-Zhongshan, and Guangzhou-Zhongshan. These areas exhibit a distinct ‘bowl-like’ feature in the subsidence velocity map. b and c illustrate the distribution of the Quaternary sediments and the correlation coefficients of the sediment thickness, which yields the highest absolute coefficient value among the factors evaluated in the GWR models. It can be seen in e that the coefficient values of these boreholes show a similar trend with the deformation velocity, except for P3 located in the Jiangmen subsidence bowl, which has a relatively low correlation coefficient with a high subsidence velocity. f shows the geological layers present in this area, An aquifer, as a specific geological formation, stores and transmits groundwater (Thilagavathi et al. Citation2012). In contrast, aquitards act as intervening barriers between aquifers, limiting the vertical movement of groundwater. Notably, Quaternary sediments can encompass both aquifer and aquitard layers within their composition. The spatial distribution of the three subsidence areas is in strong agreement with the distribution of Quaternary sediments, thus providing confirmation of earlier research findings (Du et al. Citation2020; Ma et al. Citation2019). The correlation coefficient values in the selected areas are generally negative, indicating that thicker sediments experience faster subsidence. This finding agrees with sediment consolidation mechanisms. The highest coefficient value, reaching −0.038, located in the Zhuhai-Zhongshan bowl, suggests that sediment consolidation contributes significantly to the settlement in this area.
Figure 12. (a) Deformation velocity and (b) sediment thickness in the eastern PRD originated from (Wu et al. Citation2023). (c) The correlation coefficient between deformation velocity and sediment thickness. (d) Distribution of dominant factors. (e) Subsidence velocity and correlation coefficient profile along the cross-section AA’. (f) Geological strata of the five boreholes indicated in (a)-(d).

To provide a more distinct visualization, we show the main component affecting subsidence in d. In the investigated area, the variable ‘sediment thickness’ dominates most of the subsidence, which further supports the primary cause of these subsidence events as sediment consolidation. We obtained the hydrogeological settings of five boreholes (P1, P2, P3, P4, and P5) located in the three-subsidence bowls from the GIG, as shown in a. The subsidence result profiles and correlation coefficients ·along the cross-section AA’ are shown in e. There is a notable correlation between the spatial distribution of subsidence velocity and the hydrogeological curves at corresponding locations, which can be attributed to the compaction of the soft soil layer. This compaction is strongly linked to the thickness of the underlying aquifer and aquitard layers. When groundwater is pumped out from an aquifer, the water pressure decreases, causing the soil particles in the aquifer and aquitard layers to compact and settle, resulting in land subsidence. This conclusion has been drawn in other deltas such as Ganges-Brahmaputra Delta, the Greater Jakarta area, and the Yangtze Delta (Higgins et al. Citation2014; Bakr Citation2015; Bucx et al. Citation2015).
The coefficient values of these boreholes show a similar trend with the deformation velocity, except for P3 located in the Jiangmen subsidence bowl, which has a relatively low correlation coefficient with a high subsidence velocity. The phenomenon is possibly due to excessive groundwater extraction in Jiangmen, which affects the subsidence more than natural sediment consolidation. d, further validates that the dominant factor of P3 is not ‘sediment thickness’.
The investigation findings suggest that sediment thickness should be incorporated into land use planning, building design, and infrastructure development as a key consideration. This could be achieved through avoiding construction in areas with thicker sediments or implementing appropriate engineering techniques to mitigate the potential effects of subsidence. In addition, the establishment of monitoring and early warning systems to continuously assess subsidence and groundwater levels would enable proactive measures to be taken in a timely manner. By adopting a region-specific subsidence mitigation policy that incorporates these recommendations, urban planners and policymakers can proactively prevent or mitigate land subsidence, ensuring sustainable urban development and minimizing potential impacts on infrastructure, environment, and communities.
5.3. Examples of human-induced subsidence
In addition to natural factors, anthropogenic activities during urbanization can also affect subsidence significantly. presents two areas of building clusters and aggregated road networks as examples for further analysis of human-induced subsidence. Each area contains two rows. In the first row, InSAR deformation velocity, dominant human-related variables, GWR correlation coefficients of the variable, and dominant factors in this area are presented from left to right. The second row displays historical optical images that depict urban construction activities.
Figure 13. Examples of human-induced subsidence. Areas A and B are located in the construction areas of Zhongshan and Dongguan respectively.

Area A, located in Zhongshan, is a residential area that was built in 2016 and has experienced recent building expansions, with an average building height of almost 30 levels. The overall subsidence velocity in Area A ranges from 0 to 8 mm/yr, with an average of 5.32 mm/yr, as depicted in A(a). Our analysis in A(d) indicates that the dominant factor affecting ground settlement in this residential area is the intensity of NTL, a general negative correlation with subsidence as shown in A(c). NTL is often used as a proxy for urbanization and can laterally respond to human activities. Areas with higher NTL intensity generally have more development and urban infrastructure. The rapid urbanization of Zhongshan has led to the rapid expansion of residential areas, which can in turn be associated with higher levels of groundwater pumping, thus accelerating ground subsidence in the area (Wang et al. Citation2022). However, when comparing the spatial distribution of land subsidence (A(a)) and NTL intensity (A(b)), we found that the relationship between NTL intensity and land subsidence is not always straightforward and can be influenced by many factors, including local geology, hydrology, and land use practices.
Area B, located in Dongguan, is an e-commerce company with a high population density (Figure S2). The main human factor affecting this area is PD as shown in B(d). As shown in B(a), the subsidence velocity in Area B varies between 0–5.7 mm/yr, with an average of 4.26 mm/yr. The population density in this region (B(b)) is significantly higher than the neighboring regions, consistent with the distribution of deformation velocity ( B(a)). The GWR correlation coefficients of PD in this region show generatively negative values in B(c). In other words, areas with high population densities may be associated with more serious land subsidence. Dongguan is in a stage of industrial transformation, leading to the rapid development of its electronics industry (Li et al. Citation2020). An increase in employment has led to an increase in population density. In areas with high population densities, the large amount of infrastructure and buildings can exacerbate the problem of land subsidence.
Both Zhongshan and Dongguan are cities that have been developing at a high rate in the last decade. Economic growth has led to rapid population growth, construction of more buildings, acceleration of urbanization, and increased ground loads, therefore impacting ground subsidence (Chen et al. Citation2015). Zhongshan’s GDP grew from 2.75 billion in 2015–3566.2 billion in 2021, an increase of 127%. Similarly, Dongguan’s GDP expanded from 6275.06 billion in 2015–10855.35 billion in 2021, an increase of 73%. These data indicate the significant impact of urbanization and economic growth on land subsidence in these areas.
Based on the findings from the analysis of areas A and B, several recommendations can be made to urban planners and policymakers for effectively addressing the issue of subsidence. First, it is important to carefully manage the impacts of rapid urbanization, expansion of residential areas, and industrial development on land subsidence. This can be achieved through measures such as controlling population density to minimize the demand for groundwater pumping, managing groundwater pumping practices to prevent excessive groundwater extraction, and minimizing ground loads from construction activities through appropriate engineering techniques and land use planning. Secondly, collaborative efforts among government agencies, developers, and local communities should be promoted to ensure the long-term stability and resilience of urban areas. This can include joint efforts in monitoring and early warning systems, policy development, and implementation of subsidence mitigation measures.
5.4. Limitation and uncertainty
In this paper, we analyzed the explanatory variables of land subsidence qualitatively and quantitatively. Our analysis has some limitations. First, there are acknowledged limitations of InSAR techniques, including difficulties in retrieving coherent data from vegetated areas, challenges in completely removing atmospheric residues, and the inability to model building-related topographic phases not modeled in the DEM (Ding et al. Citation2008; Osmanoğlu et al. Citation2016). Additionally, the medium-resolution S1 images used in our study may not capture very small-scale local subsidence due to the resolution limitation. To address this limitation, higher spatial resolution SAR images such as TerraSAR-X and COSMO-SkyMed could be utilized, although they may have smaller spatial coverage (Costantini et al. Citation2017). Furthermore, we selected nine explanatory variables to encompass various factors that could contribute to subsidence in the GBA. For future research, it would be crucial to consider other potential variables, especially groundwater level changes and land use dynamics, which were not incorporated in this study due to data availability constraints. Moreover, due to the different resolutions of the raw data for each explanatory variable, we had to resample them to match the resolution of the subsidence velocity for subsequent analysis, which may introduce some potential bias. Future research should explore the effect of different spatial scales on the correlation between subsidence and its explanatory factors.
6. Conclusion
In the paper, we explored the spatially nonstationary effects of natural evolution and human disturbances on subsidence in the GBA using the robust MTInSAR method and city-level GWR models, with the aim of contributing to the development of sustainable and resilient environments. Based on our findings, the following conclusions can be drawn:
The robust MTInSAR method demonstrated accurate and reliable monitoring of land subsidence in the GBA, with a mean absolute error of 2.91 mm/yr and a standard deviation of 1.5 mm/yr compared to leveling data. This highlights its potential as a valuable tool for continuous monitoring of land deformation in the region. The significant magnitude of observed subsidence velocities, ranging from 0 to 83.7 mm/yr, underscores the urgency of addressing land subsidence as a sustainability concern in the GBA.
Spatial cross-validation using global and local bivariate Moran's I helped identify the most significant factors of subsidence in each city of the GBA. The city-level GWR models revealed that the spatial distribution of the selected factors influencing land subsidence varied among regions, emphasizing the need for region-specific subsidence management approaches in developing sustainable solutions.
The dominant factor analysis of the GWR results indicated that both natural and human-related factors contribute significantly to land subsidence in the GBA, with natural factors accounting for 51.13% and human-induced factors accounting for 48.87% of the observed subsidence. Further analysis highlighted the influence of sediment thickness, groundwater extraction, and construction activities during rapid urbanization on land subsidence, which should be considered to ensure resilience and sustainability in the region.
Based on these findings, we recommend that policymakers and urban planners take a proactive approach toward sustainable and resilient environments in the GBA. This includes increasing the utilization of accurate and reliable monitoring methods such as MTInSAR for continuous monitoring of land subsidence and developing region-specific mitigation strategies that consider the spatially nonstationary effects of natural and human-related factors. Additionally, regular maintenance of urban infrastructure should be prioritized to minimize the impact of human-induced factors on land subsidence. By adopting a localized and holistic approach, we can work towards mitigating land subsidence and promoting sustainable and resilient environments in the GBA and similar regions.
Supplemental Material
Download MS Word (2.8 MB)Disclosure statement
No potential conflict of interest was reported by the author(s).
Data availability statement
The data that support the findings of this study are available upon reasonable request.
Correction Statement
This article has been corrected with minor changes. These changes do not impact the academic content of the article.
Additional information
Funding
References
- An, Bei, Yanan Jiang, Changcheng Wang, Peng Shen, Tianyi Song, Chihao Hu, and Kui Liu. 2023. “Ground Infrastructure Monitoring in Coastal Areas Using Time-Series inSAR Technology: The Case Study of Pudong International Airport, Shanghai.” International Journal of Digital Earth 16 (1): Taylor & Francis: 2171144. https://doi.org/10.1080/17538947.2023.2171144.
- Bagheri-Gavkosh, Mehdi, Seiyed Mossa Hosseini, Behzad Ataie-Ashtiani, Yasamin Sohani, Homa Ebrahimian, Faezeh Morovat, and Shervin Ashrafi. 2021. “Land Subsidence: A Global Challenge.” Science of The Total Environment 778 (July): 146193. https://doi.org/10.1016/j.scitotenv.2021.146193.
- Bakr, Mahmoud. 2015. “Influence of Groundwater Management on Land Subsidence in Deltas.” Water Resources Management 29 (5): 1541–1555. https://doi.org/10.1007/s11269-014-0893-7.
- Bucx, T. H. M., C. J. M. van Ruiten, G. Erkens, and G. de Lange. 2015. “An Integrated Assessment Framework for Land Subsidence in Delta Cities.” Proceedings of IAHS 372 (November). Copernicus GmbH: 485–491. doi:10.5194/piahs-372-485-2015.
- Bürgmann, Roland, Paul A. Rosen, and Eric J. Fielding. 2000. “Synthetic Aperture Radar Interferometry to Measure Earth’s Surface Topography and Its Deformation.” Annual Review of Earth and Planetary Sciences 28 (1): 169–209. https://doi.org/10.1146/annurev.earth.28.1.169.
- Chaussard, Estelle, Falk Amelung, Hasanudin Abidin, and Sang-Hoon Hong. 2013. “Sinking Cities in Indonesia: ALOS PALSAR Detects Rapid Subsidence Due to Groundwater and Gas Extraction.” Remote Sensing of Environment 128 (January): 150–161. https://doi.org/10.1016/j.rse.2012.10.015.
- Chen, Beibei, Huili Gong, Xiaojuan Li, Kunchao Lei, Yinghai Ke, Guangyao Duan, and Chaofan Zhou. 2015. “Spatial Correlation Between Land Subsidence and Urbanization in Beijing, China.” Natural Hazards 75 (3): 2637–2652. https://doi.org/10.1007/s11069-014-1451-6.
- Costa, Diogo, Paolo Burlando, and Cindy Priadi. 2016. “The Importance of Integrated Solutions to Flooding and Water Quality Problems in the Tropical Megacity of Jakarta.” Sustainable Cities and Society 20: Elsevier: 199–209. https://doi.org/10.1016/j.scs.2015.09.009.
- Costantini, Mario, Fabio Malvarosa, Federico Minati, Francesco Trillo, and Francesco Vecchioli. 2017. “Complementarity of High-Resolution COSMO-SkyMed and Medium-Resolution Sentinel-1 SAR Interferometry: Quantitative Analysis of Real Target Displacement and 3D Positioning Measurement Precision, and Potential Operational Scenarios.” In 2017 IEEE International Geoscience and Remote Sensing Symposium (IGARSS), 4602–4605. https://doi.org/10.1109/IGARSS.2017.8128026.
- Craney, Trevor A., and James G. Surles. 2002. “Model-Dependent Variance Inflation Factor Cutoff Values.” Quality Engineering 14 (3): Taylor & Francis: 391–403. https://doi.org/10.1081/QEN-120001878.
- Crosetto, Michele, Oriol Monserrat, María Cuevas-González, Núria Devanthéry, and Bruno Crippa. 2016. “Persistent Scatterer Interferometry: A Review.” ISPRS Journal of Photogrammetry and Remote Sensing, Theme issue State-of-the-art in photogrammetry, remote sensing and spatial information science” 115 (May): 78–89. https://doi.org/10.1016/j.isprsjprs.2015.10.011.
- De Zan, F., and A. Monti Guarnieri. 2006. “TOPSAR: Terrain Observation by Progressive Scans.” IEEE Transactions on Geoscience and Remote Sensing 44 (9): 2352–2360. https://doi.org/10.1109/TGRS.2006.873853.
- Ding, Xiao-Li, Zhi-Wei Li, Jian-Jun Zhu, Guang-Cai Feng, and Jiang-Ping Long. 2008. “Atmospheric Effects on InSAR Measurements and Their Mitigation.” Sensors 8 (9): Molecular Diversity Preservation International (MDPI): 5426–5448.
- Ding, Xiaoli, G. X. Liu, Z. W. Li, Zhilin Li, and Y. Q. Chen. 2004. “Ground Subsidence Monitoring in Hong Kong with Satellite SAR Interferometry.” Photogrammetric Engineering & Remote Sensing 70 (10): 1151–1156. https://doi.org/10.14358/PERS.70.10.1151.
- Dokka, Roy K., Giovanni F. Sella, and Timothy H. Dixon. 2006. “Tectonic Control of Subsidence and Southward Displacement of Southeast Louisiana with Respect to Stable North America.” Geophysical Research Letters 33 (23), https://doi.org/10.1029/2006GL027250.
- Du, Yanan, Guangcai Feng, Lin Liu, Haiqiang Fu, Xing Peng, and Debao Wen. 2020. “Understanding Land Subsidence Along the Coastal Areas of Guangdong, China, by Analyzing Multi-Track MTInSAR Data.” Remote Sensing 12 (2): Multidisciplinary Digital Publishing Institute: 299. https://doi.org/10.3390/rs12020299.
- Du, Xiaoyun, Liyin Shen, Siu Wai Wong, Conghui Meng, and Zhenchuan Yang. 2021. “Night-Time Light Data Based Decoupling Relationship Analysis Between Economic Growth and Carbon Emission in 289 Chinese Cities.” Sustainable Cities and Society 73 (October): 103119. https://doi.org/10.1016/j.scs.2021.103119.
- El Ayady, Hasna, Kevin L. Mickus, Said Boutaleb, Zine El Abidine El Morjani, Mustapha Ikirri, Fatima Zahra Echogdali, Armel Zacharie Ekoa Bessa, et al. 2023. “Investigation of Groundwater Potential Using Geomatics and Geophysical Methods: Case Study of the Anzi Sub-Basin, Western Anti-Atlas, Morocco.” Advances in Space Research, August, https://doi.org/10.1016/j.asr.2023.08.044.
- Erban, Laura E., Steven M. Gorelick, Howard A. Zebker, and Scott Fendorf. 2013. “Release of Arsenic to Deep Groundwater in the Mekong Delta, Vietnam, Linked to Pumping-Induced Land Subsidence.” Proceedings of the National Academy of Sciences 110 (34): 13751–13756. https://doi.org/10.1073/pnas.1300503110.
- Ezquerro, Pablo, Gerardo Herrera, Miguel Marchamalo, Roberto Tomás, Marta Béjar-Pizarro, and Rubén Martínez. 2014. “A Quasi-Elastic Aquifer Deformational Behavior: Madrid Aquifer Case Study.” Journal of Hydrology 519: Elsevier: 1192–1204. https://doi.org/10.1016/j.jhydrol.2014.08.040.
- Farr, Tom G., Paul A. Rosen, Edward Caro, Robert Crippen, Riley Duren, Scott Hensley, Michael Kobrick, et al. 2007. “The Shuttle Radar Topography Mission.” Reviews of Geophysics 45 (2), https://doi.org/10.1029/2005RG000183.
- Ferretti, Alessandro, Alfio Fumagalli, Fabrizio Novali, Claudio Prati, Fabio Rocca, and Alessio Rucci. 2011. “A New Algorithm for Processing Interferometric Data-Stacks: SqueeSAR.” IEEE Transactions on Geoscience and Remote Sensing 49 (9): 3460–3470. https://doi.org/10.1109/TGRS.2011.2124465.
- Ferretti, A., C. Prati, and F. Rocca. 2001. “Permanent Scatterers in SAR Interferometry.” IEEE Transactions on Geoscience and Remote Sensing 39 (1): 8–20. https://doi.org/10.1109/36.898661.
- Fildes, Stephen G., F. Ian, Nara M. Clark, and Glyn Ashman. Somaratne. 2020. “Mapping Groundwater Potential Zones Using Remote Sensing and Geographical Information Systems in a Fractured Rock Setting, Southern Flinders Ranges, South Australia.” Journal of Earth System Science 129 (1): 160. https://doi.org/10.1007/s12040-020-01420-1.
- Fisher, Ronald A. 1956. Statistical Methods and Scientific Inference. Oxford, England: Hafner Publishing Co.
- Fotheringham, A. Stewart, Chris Brunsdon, and Martin Charlton. 2003. Geographically Weighted Regression: The Analysis of Spatially Varying Relationships. Oxford, England: John Wiley & Sons.
- Gebremichael, Esayas, Mohamed Sultan, Richard Becker, Mohamed El Bastawesy, Omar Cherif, and Mustafa Emil. 2018. “Assessing Land Deformation and Sea Encroachment in the Nile Delta: A Radar Interferometric and Inundation Modeling Approach.” Journal of Geophysical Research: Solid Earth 123 (4): 3208–3224. https://doi.org/10.1002/2017JB015084.
- Halder, Bijay, Jatisankar Bandyopadhyay, and Papiya Banik. 2021. “Monitoring the Effect of Urban Development on Urban Heat Island Based on Remote Sensing and Geo-Spatial Approach in Kolkata and Adjacent Areas, India.” Sustainable Cities and Society 74 (November): 103186. https://doi.org/10.1016/j.scs.2021.103186.
- Hanssen, Ramon F. 2001. Radar Interferometry: Data Interpretation and Error Analysis. Dordrecht: Springer Science & Business Media.
- Herrera-García, Gerardo, Pablo Ezquerro, Roberto Tomás, Marta Béjar-Pizarro, Juan López-Vinielles, Mauro Rossi, Rosa M. Mateos, et al. 2021. “Mapping the Global Threat of Land Subsidence.” Science 371 (6524): American Association for the Advancement of Science: 34–36. https://doi.org/10.1126/science.abb8549.
- Higgins, Stephanie A., Irina Overeem, Michael S. Steckler, James P. M. Syvitski, Leonardo Seeber, and S. Humayun Akhter. 2014. “InSAR Measurements of Compaction and Subsidence in the Ganges-Brahmaputra Delta, Bangladesh.” Journal of Geophysical Research: Earth Surface 119 (8): 1768–1781. https://doi.org/10.1002/2014JF003117.
- Higgins, Stephanie, Irina Overeem, Akiko Tanaka, and James P. M. Syvitski. 2013. “Land Subsidence at Aquaculture Facilities in the Yellow River Delta, China.” Geophysical Research Letters 40 (15): 3898–3902. https://doi.org/10.1002/grl.50758.
- Huang, Xiao, Zhenlong Li, Yuqin Jiang, Xinyue Ye, Chengbin Deng, Jiajia Zhang, and Xiaoming Li. 2021. “The Characteristics of Multi-Source Mobility Datasets and How They Reveal the Luxury Nature of Social Distancing in the U.S. During the COVID-19 Pandemic.” International Journal of Digital Earth 14 (4): Taylor & Francis: 424–442. https://doi.org/10.1080/17538947.2021.1886358.
- Jendryke, Michael, and Stephen C. McClure. 2021. “Spatial Prediction of Sparse Events Using a Discrete Global Grid System; a Case Study of Hate Crimes in the USA.” International Journal of Digital Earth 14 (6): Taylor & Francis: 789–805. https://doi.org/10.1080/17538947.2021.1886356.
- Jing, Fengrui, Zhenlong Li, Shan Qiao, Jiajia Zhang, Banky Olatosi, and Xiaoming Li. 2023. “Using Geospatial Social Media Data for Infectious Disease Studies: A Systematic Review.” International Journal of Digital Earth 16 (1): Taylor & Francis: 130–157. https://doi.org/10.1080/17538947.2022.2161652.
- Kim, Sang-Wan, Shimon Wdowinski, Timothy H. Dixon, Falk Amelung, Jeong Woo Kim, and Joong-Sun Won. 2010. “Measurements and Predictions of Subsidence Induced by Soil Consolidation Using Persistent Scatterer InSAR and a Hyperbolic Model.” Geophysical Research Letters 37 (5), https://doi.org/10.1029/2009GL041644.
- Kwan, Mei-Po. 2021. “The Stationarity Bias in Research on the Environmental Determinants of Health.” Health & Place 70 (July): 102609. https://doi.org/10.1016/j.healthplace.2021.102609.
- Lanari, R., O. Mora, M. Manunta, J. J. Mallorqui, P. Berardino, and E. Sansosti. 2004. “A Small-Baseline Approach for Investigating Deformations on Full-Resolution Differential SAR Interferograms.” IEEE Transactions on Geoscience and Remote Sensing 42 (7): 1377–1386. https://doi.org/10.1109/TGRS.2004.828196.
- Li, Xun, Eddie Chi-Man Hui, Wei Lang, Shali Zheng, and Xiaozhen Qin. 2020. “Transition from Factor-Driven to Innovation-Driven Urbanization in China: A Study of Manufacturing Industry Automation in Dongguan City.” China Economic Review 59 (February): 101382. https://doi.org/10.1016/j.chieco.2019.101382.
- Lyu, Hai-Min, Shui-Long Shen, An-Nan Zhou, and Wan-Huan Zhou. 2019. “Flood Risk Assessment of Metro Systems in a Subsiding Environment Using the Interval FAHP-FCA Approach.” Sustainable Cities and Society 50 (October): 101682. https://doi.org/10.1016/j.scs.2019.101682.
- Ma, Peifeng, and Hui Lin. 2015. “Robust Detection of Single and Double Persistent Scatterers in Urban Built Environments.” IEEE Transactions on Geoscience and Remote Sensing 54 (4): IEEE: 2124–2139.
- Ma, Peifeng, Weixi Wang, Bowen Zhang, Jili Wang, Guoqiang Shi, Guangqing Huang, Fulong Chen, Liming Jiang, and Hui Lin. 2019. “Remotely Sensing Large-and Small-Scale Ground Subsidence: A Case Study of the Guangdong–Hong Kong–Macao Greater Bay Area of China.” Remote Sensing of Environment 232: Elsevier: 111282.
- Mathew, Aneesh, Sumit Khandelwal, and Nivedita Kaul. 2016. “Spatial and Temporal Variations of Urban Heat Island Effect and the Effect of Percentage Impervious Surface Area and Elevation on Land Surface Temperature: Study of Chandigarh City, India.” Sustainable Cities and Society 26: Elsevier: 264–277. https://doi.org/10.1016/j.scs.2016.06.018.
- Mazzotti, S., A. Lambert, M. Van der Kooij, and A. Mainville. 2009. “Impact of Anthropogenic Subsidence on Relative Sea-Level Rise in the Fraser River Delta.” Geology 37 (9): 771–774. https://doi.org/10.1130/G25640A.1.
- Meng, Xiangjin, Kebiao Mao, Fei Meng, Jiancheng Shi, Jiangyuan Zeng, Xinyi Shen, Yaokui Cui, Lingmei Jiang, and Zhonghua Guo. 2021. “A Fine-Resolution Soil Moisture Dataset for China in 2002–2018.” Earth System Science Data 13 (7): Copernicus GmbH: 3239–3261. https://doi.org/10.5194/essd-13-3239-2021.
- Minderhoud, P. S. J., L. Coumou, L. E. Erban, H. Middelkoop, E. Stouthamer, and E. A. Addink. 2018. “The Relation Between Land Use and Subsidence in the Vietnamese Mekong Delta.” Science of The Total Environment 634 (September): 715–726. https://doi.org/10.1016/j.scitotenv.2018.03.372.
- Ng, Charmaine, Hongjie Chen, Shin Giek Goh, Laurence Haller, Zhixin Wu, Francis Rathinam Charles, Aurore Trottet, and Karina Gin. 2018. “Microbial Water Quality and the Detection of Multidrug Resistant E. Coli and Antibiotic Resistance Genes in Aquaculture Sites of Singapore.” Marine Pollution Bulletin 135 (October): 475–480. https://doi.org/10.1016/j.marpolbul.2018.07.055.
- Nicholls, Robert J. 1995. “Coastal Megacities and Climate Change.” GeoJournal 37: Springer: 369–379.
- Osmanoğlu, Batuhan, Filiz Sunar, Shimon Wdowinski, and Enrique Cabral-Cano. 2016. “Time Series Analysis of InSAR Data: Methods and Trends.” ISPRS Journal of Photogrammetry and Remote Sensing 115: Elsevier: 90–102.
- Ouyang, Chaojun, Kaiqi Zhou, Qiang Xu, Jianhua Yin, Dalei Peng, Dongpo Wang, and Weile Li. 2017. “Dynamic Analysis and Numerical Modeling of the 2015 Catastrophic Landslide of the Construction Waste Landfill at Guangming. Shenzhen, People’s Republic of China.” Landslides 14: Springer: 705–718.
- Rosen, P. A., S. Hensley, I. R. Joughin, F. K. Li, S. N. Madsen, E. Rodriguez, and R. M. Goldstein. 2000. “Synthetic Aperture Radar Interferometry.” Proceedings of the IEEE 88 (3): 333–382. https://doi.org/10.1109/5.838084.
- Roy, Priyom, Tapas R. Martha, Kirti Khanna, Nirmala Jain, and K. Vinod Kumar. 2022. “Time and Path Prediction of Landslides Using InSAR and Flow Model.” Remote Sensing of Environment 271: Elsevier: 112899.
- Salimi, Mohsen, and Sami G. Al-Ghamdi. 2020. “Climate Change Impacts on Critical Urban Infrastructure and Urban Resiliency Strategies for the Middle East.” Sustainable Cities and Society 54 (March): 101948. https://doi.org/10.1016/j.scs.2019.101948.
- Sarah, Dwi, Lambok M. Hutasoit, Robert M. Delinom, and Imam A. Sadisun. 2020. “Natural Compaction of Semarang-Demak Alluvial Plain and Its Relationship to the Present Land Subsidence.” Indonesian Journal on Geoscience 7 (3): 273–289.
- Shi, Xuguo, Tongtong Zhu, Wei Tang, Mi Jiang, Houjun Jiang, Chen Yang, Wei Zhan, Zutao Ming, and Shaocheng Zhang. 2022. “Inferring Decelerated Land Subsidence and Groundwater Storage Dynamics in Tianjin–Langfang Using Sentinel-1 InSAR.” International Journal of Digital Earth 15 (1): Taylor & Francis: 1526–1546. https://doi.org/10.1080/17538947.2022.2122610.
- Shirzaei, Manoochehr, Jeffrey Freymueller, Torbjörn E. Törnqvist, Devin L. Galloway, Tina Dura, and Philip S. J. Minderhoud. 2021. “Measuring, Modelling and Projecting Coastal Land Subsidence.” Nature Reviews Earth & Environment 2 (1): Nature Publishing Group: 40–58. https://doi.org/10.1038/s43017-020-00115-x.
- Shupeng, C., and J. van Genderen. 2008. “Digital Earth in Support of Global Change Research.” International Journal of Digital Earth 1 (1): Taylor & Francis: 43–65. https://doi.org/10.1080/17538940701782510.
- Tang, Cheng, Di Zhou, Rudolf Endler, Jinqing Lin, and Jan Harff. 2010. “Sedimentary Development of the Pearl River Estuary Based on Seismic Stratigraphy.” Journal of Marine Systems 82: Elsevier: S3–S16.
- Teatini, P., L. Tosi, and T. Strozzi. 2011. “Quantitative Evidence That Compaction of Holocene Sediments Drives the Present Land Subsidence of the Po Delta, Italy.” Journal of Geophysical Research: Solid Earth 116 (B8), https://doi.org/10.1029/2010JB008122.
- Thilagavathi, R., S. Chidambaram, M. V. Prasanna, C. Thivya, and C. Singaraja. 2012. “A Study on Groundwater Geochemistry and Water Quality in Layered Aquifers System of Pondicherry Region, Southeast India.” Applied Water Science 2: Springer: 253–269. https://doi.org/10.1007/s13201-012-0045-2.
- Wang, Zhengyu, Yaolin Liu, Yang Zhang, Yanfang Liu, Baoshun Wang, and Guangxia Zhang. 2022. “Spatially Varying Relationships Between Land Subsidence and Urbanization: A Case Study in Wuhan, China.” Remote Sensing 14 (2): Multidisciplinary Digital Publishing Institute: 291. https://doi.org/10.3390/rs14020291.
- Wang, Hua, Tim J. Wright, Yongping Yu, Hong Lin, Lilong Jiang, Changhui Li, and Guangxin Qiu. 2012. “InSAR Reveals Coastal Subsidence in the Pearl River Delta, China: Coastal Subsidence in the PRD.” Geophysical Journal International, 22. https://doi.org/10.1111/j.1365-246X.2012.05687.x.
- Wei, Xing, and ChaoYu Wu. 2011. “Holocene Delta Evolution and Sequence Stratigraphy of the Pearl River Delta in South China.” Science China Earth Sciences 54: Springer: 1523–1541.
- Wu, Zherong, Peifeng Ma, Yi Zheng, Feng Gu, Lin Liu, and Hui Lin. 2023. “Automatic Detection and Classification of Land Subsidence in Deltaic Metropolitan Areas Using Distributed Scatterer InSAR and Oriented R-CNN.” Remote Sensing of Environment 290 (May): 113545. https://doi.org/10.1016/j.rse.2023.113545.
- Xu, Gang, Jingling Su, Chang Xia, Xi Li, and Rui Xiao. 2022. “Spatial Mismatches Between Nighttime Light Intensity and Building Morphology in Shanghai, China.” Sustainable Cities and Society 81: Elsevier: 103851.
- Yang, Mengshi, Ru Wang, Menghua Li, and Mingsheng Liao. 2022a. “A PSI Targets Characterization Approach to Interpreting Surface Displacement Signals: A Case Study of the Shanghai Metro Tunnels.” Remote Sensing of Environment 280 (October): 113150. https://doi.org/10.1016/j.rse.2022.113150.
- Yang, Ting, Kaile Zhou, and Chi Zhang. 2022b. “Spatiotemporal Patterns and Influencing Factors of Green Development Efficiency in China’s Urban Agglomerations.” Sustainable Cities and Society 85: Elsevier: 104069.
- Yu, Hairuo, Huili Gong, Beibei Chen, Kaisi Liu, and Mingliang Gao. 2020. “Analysis of the Influence of Groundwater on Land Subsidence in Beijing Based on the Geographical Weighted Regression (GWR) Model.” Science of The Total Environment 738 (October): 139405. https://doi.org/10.1016/j.scitotenv.2020.139405.
- Zheng, Yuanmao, Yuanrong He, Qiang Zhou, and Haowei Wang. 2022. “Quantitative Evaluation of Urban Expansion Using NPP-VIIRS Nighttime Light and Landsat Spectral Data.” Sustainable Cities and Society 76: Elsevier: 103338. https://doi.org/10.1016/j.scs.2021.103338.