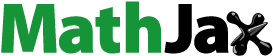
ABSTRACT
Identifying the factors influencing wetland variations is crucial for understanding the relationship of climate change with wetland conservation and management. The wetland distribution is associated with multiple variables, and the interactions among these variables are complex. In this study, we aim to explore an interpretable and quantitative analysis of factors related to wetland spatiotemporal variations on the Tibetan Plateau (TP). By combining SHapley Additive exPlanations with a spatially stratified heterogeneity model, we propose a locally explained stratified heterogeneity (LESH) model that well reveals the effects of multiple variable interactions on the spatiotemporal variations of wetlands. The results show that topographic variables are the most important variables related to the spatial distribution of wetlands on the TP, and climatic variables are the most relevant factors for the increase in the wetland area on the TP from 2015 to 2019. In addition, the interactions among multiple variables strongly influence wetlands on the TP. Among them, when other geographic variables interact with the evaporation variable, its explanatory power on wetland distribution is significantly increased. Knowledge of wetland distribution determinants can help us understand the evolution of wetlands and the impacts of climate change on wetland variations.
1. Introduction
Wetlands provide abundant ecological and climatic benefits. They are critical for hydrology, biogeochemical function, and biodiversity conservation (Chatterjee et al. Citation2015; Cohen et al. Citation2016; Gall et al. Citation2013; Russi Citation2013). The soil and biomass in wetlands can capture and store atmospheric carbon dioxide over long periods to counteract the effects of climate change (Chmura et al. Citation2003; Mitsch et al. Citation2013; Were et al. Citation2019). The Tibetan Plateau (TP) is the birthplace of many large rivers in Asia and has unique alpine wetlands (lakes, rivers, marshes, etc., under unique alpine climate conditions) (Zhao et al. Citation2015; Cao and Zhang Citation2015). As a sensitive region and magnifier of global climate change, the TP has been significantly impacted by climate conditions and environmental variability over the past three decades (Kang et al. Citation2007; Yao et al. Citation2000; Zhang et al. Citation2020). The spatiotemporal changes in wetlands and the relevant factors on the TP have attracted great attention.
Knowing the factors that influence the wetland variations on the TP could facilitate our understanding of wetland development and changes. By revealing the interactions between hydrological processes and the ecosystem, we can develop coupling models to simulate spatiotemporal hydrological patterns and processes (Xue et al. Citation2018; Zhang et al. Citation2016b). These models are essential in predicting whether wetlands will be resilient or vulnerable to climate change in the future (Zhang et al. Citation2016a). This knowledge is also helpful for making decisions regarding wetland restoration and protection (Hu et al. Citation2017). To make protection measures more effective and targeted, it is essential to set different measures for different regions according to the characteristics of wetlands (Xu et al. Citation2019). By carefully designing these partition strategies, researchers can help to protect and preserve the unique wetland ecosystems of the TP.
Numerous methods can be used to investigate the related factors of spatiotemporal variations on wetlands. These methods can be categorized into two groups: statistical models and mechanical models (Wang et al. Citation2022). Correlation analysis methods are the most widely used statistical methods. The correlation of time series data was used to investigate the relationships between variables (Wang, He, and Niu Citation2020; Wang et al. Citation2022). Regression analysis methods use curve-fitting statistics to explore spatial relationships. For example, multiple regression was used to predict the wetland extent with coastal and watershed variables and calculate the explanatory power to wetland changes (Braswell and Heffernan Citation2019). Geographically weighted regression (GWR) was used to reveal the critical influencing factors of spatiotemporal variability on wetlands (Tian et al. Citation2023). In addition, several studies have investigated the impacts of climate variables on wetland changes based on mechanical models, such as wetland hydrological models (Moshir Panahi et al. Citation2022). Xi et al. (Citation2020) explored the effects of temperature changes on the wetland areas across 1,250 inland Ramsar sites by estimating the wetland areal extent with a hydrological model.
Spatially stratified heterogeneity (SSH) models are effective analytical frameworks for investigating the drivers of spatial variability in geographical variables. The utilization of SSH models has been on the rise in recent years for characterizing the spatial variability of geographical variables (Guo et al. Citation2022; Luo et al. Citation2022). These models enable a comparison of the spatial distribution patterns of dependent and independent variables to calculate the power of determinants (PD) (Luo et al. Citation2023). A higher PD value indicates similar spatial distributions. In the classical SSH model, spatial discretization was conducted according to equal, quantile, or geometric breaks, and no optimization occurred in this process. The detected spatial associations of this method were influenced by the rule applied to determine spatial discretization. Thus, the corresponding PD could not fully explain the spatial associations between the explanatory variables and response variables. Studies have detected a significant underestimation of PD when using the classical SSH model (Luo et al. Citation2022). To address the above problem, several models have been developed to calculate the optimal power of determinant (OPD), such as the optimal parameter based geographical detector (OPGD) and geographically optimal zones-based heterogeneity (GOZH) models (Luo, Song, and Wu Citation2021; Song et al. Citation2020; Song and Wu Citation2021; Luo et al. Citation2022). By optimizing the spatial discretization process, the corresponding OPD can significantly improve the method to fully reveal the spatial associations.
However, current SSH models still faced difficulties to explain the contributions of variables due to the complex spatial heterogeneity of wetlands in large regions. A black box exists in the current SSH models when calculating the interactions between multiple explanatory variables. There is a need to distribute the contributions in a fair way to explain the role of each variable and the interactions between multiple variables. In addition, past studies have ignored the scale effect of geographical variables on wetlands. In spatial analyses, the size of the units directly affects the level of detail captured and the results generated (Chen et al. Citation2019). If the scale of the variables changes, then the covariance between them, their correlation coefficient, and the statistical model results also change (Wu Citation2004). Therefore, analyses relying on geographical variables are relatively scale-sensitive, and it is important to choose the optimal scale when characterizing and comparing data when using these analysis methods.
In this study, we developed a locally explained stratified heterogeneity (LESH) model to calculate the contribution of each variable for explaining the spatiotemporal heterogeneity of wetlands on the TP. The multiple grid-scale wetland density from 2000 to 2019 was calculated from a wetland product (Li et al. Citation2023a; Li et al. Citation2023b). Correspondingly, the explanatory variables were collected from Google Earth Engine (GEE) and classified into three categories: geographic, climatic, and environmental variables. The Shapley value, a commonly employed concept in cooperative game theory analyses to fairly distribute the ‘payout’ among players (Shapley Citation1953; Datta, Sen, and Zick Citation2016; Lundberg and Lee Citation2017), was introduced to assign a total explanatory power to each variable. Based on this model, the contribution of each variable and the interactions among multiple variables were obtained. First, the optimal scale of analysis was determined by investigating the associations between each variable with wetland density at different scales. Second, the impacts of individual variables on the spatiotemporal variations of wetlands were analysed at the optimal scale. Third, the LESH model was used to calculate the interactions among multiple variables. Finally, the geographically optimal wetland zones over the first 15 years (slowly fluctuating period) and the following 5 years (rapid growth period) of the study period were determined. The impact of the related factor on the wetland distribution was analysed according to the geographically optimal zones, and each variable’s contribution was calculated.
2. Data
2.1. Response variable
In our study, the wetland density was used as the response variable. Compared with wetland area, wetland density was more convenient for comparison among different scales. Wetland density data at multiple scales were calculated from the yearly wetland product on the TP (Li et al. Citation2023a; Li et al. Citation2023b). The product was generated from Landsat satellite images between September and October from 2000 to 2019. Lakes, rivers, and marshes including moss marshes, herbaceous marshes and salt marshes were the main types of extracted wetlands. Validation experiments indicated that this wetland product is highly accurate (with a 96.1% user’s accuracy and 90.8% producer’s accuracy). shows the spatial (a) and temporal (b) variations of the wetlands on the TP. There are more wetlands in the north-western region and fewer in the south-eastern area. In terms of temporal variations, two distinct wetland periods were distinguished by Ruptures (Truong, Oudre, and Vayatis Citation2020), a Python library for change point detection. From 2000 to 2014, the TP wetland area fluctuated between 160,000 km2 and 185,000 km2 with no significant changes. From 2015 to 2019, the TP wetlands showed a rapidly extending trend. The wetland growth (50,725 km2) during this period was more than one-fourth of that in 2015. In subsequent analyses, we used wetland density from 2000 to 2014 as the proxy of the spatial variations of wetlands, and wetland density from 2015 to 2019 as the proxy of the spatial and temporal variations of wetlands.
2.2. Explanatory variables
The explanatory variables used to explain the spatial disparities of wetlands included topographical, climatic, and environmental categories derived from remotely sensed data (). All these data were collected using Google Earth Engine (GEE). Since the Landsat images used to identify wetlands were all taken from September to October, the explanatory variables were also averaged for September and October to represent the climatic and environmental conditions as much as possible.
Table 1. Explanatory variables for the wetland density.
2.2.1. Topographical variables
Topographical variables, including the elevation and derived slope data, were obtained from GEE to represent the topographical conditions of the TP. The data were collected from the digital elevation model (DEM) at a resolution of 30 m from the Space Shuttle Radar Terrain Mission (SRTM) (Farr and Kobrick Citation2000). The slope data were computed from the elevation data by the spatial analysis function in GEE.
2.2.2. Climate variables
Climate change is an essential driver of the conversion of wetland ecosystems (Mitsch et al. Citation2013; Wang, He, and Niu Citation2020). The climate variables used in this study include monthly temperature and precipitation data derived from the ERA5-Land monthly averaged data (Muñoz-Sabater et al. Citation2021). ERA5-Land is a reanalysis product that integrates observational data with the fundamental principles of physics, thereby providing a precise characterization of the climate. The data has been transformed into monthly averages with a spatial resolution of 0.125 × 0.125 degrees.
2.2.3. Environmental variables
The enhanced vegetation index (EVI), normalized difference vegetation index (NDVI), evaporation, runoff, and snowmelt were used as environmental variables to characterize the local environmental conditions. The EVI and NDVI data used in this study were obtained from Terra MODIS products (MOD13Q1) at a spatial resolution of 250 m (Didan, Munoz, and Huete Citation2015). Evaporation, runoff, and snowmelt were derived from the ERA5-Land monthly averaged data.
3. Methods
3.1. Locally explained stratified heterogeneity (LESH) model
3.1.1. Concept of the LESH
shows the difference between the three kinds of SSH models. Given the response variable and one or multiple explanatory variables, spatial discretization was conducted, and the variance in the response variable among the divided zones was calculated. In the classical SSH model (b), the PD value could not fully explain the spatial associations between the explanatory variables and response variables. Some improved models, such as the OPGD and GOZH models (c), can significantly improve the PD value to fully reveal the spatial associations. However, although the OPD can accurately estimate how multiple explanatory variables influence the response variable, we have yet to determine the contribution of each explanatory variable. For example, we may find that temperature and precipitation can together explain 50% of the wetland distribution. There is a need to determine the contribution of temperature to this interaction.
Figure 2. The development of the SSH model. (a), the response variable and the explanatory variables; (b), the concept of the power of determinant (PD); (c), the concept of the optimal power of determinant (OPD); (d), the SHAP power of determinant (SPD).

In this study, we aim to open this black box and determine the contribution of each variable to the OPD. We propose the LESH model by combining the SHAP and SSH models. The improved OPD, called the SHAP power of determinants (SPD), can fully explain the contribution of each variable regardless of how many variables are considered or how complex the interaction process is (d).
shows the flowchart of the LESH model. Given the response variable and multiple explanatory variables, the LESH model provides three outputs: the OPD value of individual variables and multiple variables, the SPD value of each variable, and the geographically optimal zones. The OPD values refer to the correlation between the dependent variable and explanatory variables. The SPD values represent the contribution of each variable. The geographically optimal zones can be applied to assess the overall impacts of multiple variables on spatial patterns of dependent variable.
Figure 3. The workflow of the LESH model. (b), calculation of the OPD by the decision tree-based SSH model; (c), the results of OPD and the spatial discretization (zones) of the response variable; (d), calculation of the SPD value based on the SHAP model; (e), the results of SPD and the optimal spatial discretization (zones) of the response variable.

To begin, the OPD values of individual variables and multiple variables were calculated based on the GOZH model (b). Subsequently, the contribution of each variable was computed according to the concept of Shapley value (d). Finally, we obtain the geographically optimal zones, which represent the strongest correlation of all possible combinations of explanatory variables to the spatial distribution of the dependent variable.
3.1.2. Power of determinants (PD) and optimal power of determinants (OPD)
The PD value was a measure of the spatial association between the response variable and the explanatory variable, with a higher PD value indicating a stronger association. This value was a ratio of the variance in the wetland density within the zones, determined by explanatory variables, to the variance across the entire study area. The formula was as follows:
(1)
(1) where SSW represents the summation of squares within individual zones, SST corresponds to the summation of squares of wetland density across the entire study area,
and
denote the number and standard deviation of wetland density in each zone z (z = 1, … , h), respectively, and N and σ are the number and standard deviation of the wetland density across the study area, respectively.
In this study, the optimal PD value, which was proposed by the geographically optimal zone-based heterogeneity (GOZH) model (Luo et al. Citation2022), was used to explore the factors influencing the wetland distribution. In GOZH, the OPD is represented by the value, which is calculated as follows:
(2)
(2) where
denotes the sum of squares within zones that are recorded as D and determined by explanatory variable X. The Ω value can identify the optimal geographical zone determined by multiple explanatory variables and demonstrate the maximum PD of these explanatory variables.
3.1.3. SHAP power of determinants (SPD)
The cooperative game theory proposed by Shapley (Shapley Citation1953; Lundberg and Lee Citation2017) was used to calculate the contribution of each explanatory variable under the condition of multivariate interactions. Our method can be described as follows. Suppose that the explanatory variables include ,
,
, …
, for a total of |M| (where |M| represents the number of variables in the set of M) variables. S = {
,
,
, …
} (s< = m) is a subset within the |S| explanatory variables excluding
. Our method can distribute the total OPD value to each variable in a 'fair' way. For variable
, the SHAP power of determinants (SPD), i.e. the contribution of variable
to the
value, can be calculated by the following equation:
(3)
(3) where
denotes the SPD of variable
in the set M.
denotes that the S is a subset of M, but S does not contain the variable
, and
is the function used to calculate the OPD under the interaction of |S| (where |S| represents the number of variables in the set of S) variables.
This formula is equal to the Shapley values (Lundberg et al. Citation2020; Lundberg and Lee Citation2017) and can be understood as follows: the SPD is the weighted average of the difference between the functions of all subsets containing the variable
and those not containing the variable
(). Notably, the empty set is also a part of this set. In any combination of subsets, the contribution of the variable
can be calculated by
; then, for each variable, the mean of this contribution can be calculated over all permutations.
For a combination of variables S, the following expression can be obtained:
(4)
(4) i.e. the sum of the contributions of all variables in set S is equal to the total
value of the set. Thus, the contribution of each variable we calculated is a part of the
value.
(5)
(5) The SPD proposed herein has the following three desirable properties, consistent with the Shapley values.
Symmetry:
If and
are two explanatory variables that contribute equally to all possible combinations, i.e.
(6)
(6) for every subset S that contains neither i nor j,
, then their Shapley values are identical:
(7)
(7)
(2) | Dummy variable: |
If for an explanatory variable
and all combinations
, then:
(8)
(8) (3) Linearity:
If other methods can be used to calculate the maximum explanatory power of variables, i.e. if both and
exist, then the contribution of the variable
in the combination of these two methods is equal to the sum of the contributions under the respective methods. This ensures the extensibility of our method.
(9)
(9)
3.2. Examining wetland variations with LESH model
The framework comprises three main components (): data pre-processing, optimal scale determination, and the computational stage utilizing the LESH model. This stage involves individual variable exploration, multiple variables exploration and identifying optimal zones.
Figure 4. Schematic workflow of the process used to identify the spatiotemporal heterogeneity and influencing factors of the wetland distribution on the TP based on the LESH model.

3.2.1. Data pre-processing and the identification of the optimal analysis scale
The raw wetland data used herein were pixel-based classification images, but the LESH model requires continuous variables, such as area and density variables. Therefore, the data had to be transformed into the wetland density, i.e. the proportion of wetlands per unit area. To investigate the effects of the variable scale on the spatial distribution of wetlands, the multi-resolution data was aggregated using average function. The wetland density was aggregated from the 1-km resolution to the 10-km resolution at 1-km intervals and from the 10-km resolution to the 150-km resolution at 10-km intervals. The explanatory variables were also aggregated to different resolutions using the average function. For the elevation, slope, NDVI, and EVI variables, we first obtained 1-km-resolution data through the GEE platform and then obtained multi-resolution data by calculating the pixel means. For the five variables of temperature, precipitation, evaporation, runoff, and snowmelt, since the original resolution was 0.125°×0.125°, we first resampled these data to a 1-km resolution using the GEE platform and then aggregated them to other resolutions by calculating the average values.
In this study, we investigate the optimal scale to analyse the distribution of wetlands on the TP using multiscale data in the LESH model. First, for each year and each scale, the OPD values of all nine variables were calculated. Second, for each scale, a box plot of all OPD values of the nine variables over two decades was obtained. Third, the mean OPD values of the nine variables over two decades were calculated. Finally, the optimal scale was selected according to the change rate between the adjacent scales. The locally estimated scatterplot smoothing (LOESS) model (Jacoby Citation2000) was used to fit the mean OPD values into a curve, and then the change rate of OPD values at different scales was calculated. The scale with a change rate of less than 5% was selected as the optimal scale (Song et al. Citation2020; Luo, Song, and Wu Citation2021).
3.2.2. Calculating of OPD values of individual variables
Based on the optimal scale, we analysed the effects of individual explanatory variables on the wetland density from 2000 to 2019 using the LESH model. First, the annual data were leveraged to calculate the OPD values. The order of importance of the variables was determined by comparing the OPD values calculated from the individual variables. Second, the annual wetland density and explanatory variables were classified into two periods considering that the wetland area showed two distinct phases, i.e. 2000-2014 and 2015-2019. The change rates of each explanatory variable in both periods were calculated. By comparing the changes in OPD values of each explanatory variable, we obtained the key variables dominating the wetland distributions in different periods.
3.2.3. Calculating of SPD values
This study detected and analysed the interactions of two variables as a case study. We calculated the interactions between variable pairs and their respective contributions (SPD) using the LESH model. Specifically, the annual data were classified into two periods. Then, we iterated through all possible combinations of variables to compute the OPD under different combinations of variables. Finally, the SPD values were calculated for each variable. The SPD value is the weighted average of all OPD values of the combinations containing the targeted variable with that of the combinations without the targeted variable.
3.2.4. Identification of geographically optimal zones
Stratified variables from the spatial discretization were used to identify the geographically optimal zones. According to the stratified variables, wetland density was grouped into geographically optimal zones, indicating the highest homogeneity within zones and the highest heterogeneity between zones.
Since all possible combinations of variables were iterated in the above calculations, we can obtain the geographically optimal combination of variables that is most relevant to wetland density. Subsequently, the contribution of each variable to these optimal zones was analysed, thereby providing insights into how multiple variables impact the spatial patterns of wetlands. Finally, a comprehensive analysis of the geographical, climate, and environmental variables was conducted based on the geographically optimal zones, aiming to reveal the regional spatial associations with the wetland density.
4. Results
4.1. The optimal scale for analysing wetland distribution
The optimal scale was identified for the heterogeneity analysis of the wetland distribution. a shows the variations in Ω values at different scales. The explanatory power of the wetland distribution gradually increased with the scale of analysis. From the 1-km to the 60-km scale, the growth rate consistently increased. The highest value (0.140) was reached at the 60-km scale (b). As the scale increased, the growth rate of Ω value started to decelerate. By the scale reached 120-km, the growth rate of Ω value was lower than 0.05. The choice of scale involved a trade-off between the strength of interpretation and the granularity of the study, since a high scale leads to a decrease in the number of image elements and a decrease in the granularity of the study. In this study, we chose 120-km, where the growth rate of Ω value was below 0.05, as the optimal scale for analysis.
4.2. Impacts of individual variables
shows the Ω values of different variables at the 120-km scale over 20 years. From the perspective of the three categories of variables, topographic variables had the highest spatial associations with wetland density, followed by climatic and environmental variables. In terms of individual variables, the slope variable has the highest average Ω value among the nine variables, explaining 49.2% of the spatial variability in the wetland distribution on average (20 years). Among the nontopographic variables, vegetation accounted for the most important role in the wetland distribution. Among them, the Ω values of EVI and NDVI were 0.320 and 0.318, respectively. Runoff had a Ω value of 0.279 for the wetland distribution.
Figure 6. The OPD values of the explanatory variables of the wetland distribution. SLO refers to slope, ELE refers to elevation, EVI refers to enhanced vegetation index, NDVI refers to Normalized Difference Vegetation Index, TEM refers to temperature, PRE refers to precipitation, EVA refers to Evaporation, SM refers to snowmelt, RO refers to runoff.

We further examined the spatial associations between the individual variables and the wetland density during two periods. shows the Ω values of each variable during 2000-2014 and 2015-2019. The results also reveal the dominant impact of the topographical variables on the wetland distribution. However, the importance of variables changed during the two periods. During 2015-2019, the Ω values of topographical variables such as the slope and elevation decreased by 17.6% and 26.0%, respectively, while the Ω values of NDVI, EVI and temperature increased by 26.0%, 43.1%, and 73.5% (b), respectively, compared with 2000-2014. The decreased Ω values of the topographical variables indicated a decrease in the explanatory power on the wetland distribution. This suggested that the newly formed wetlands had fewer spatial associations with the topographical variables but were more significantly influenced by meteorological variables such as temperature and environmental variables.
Figure 7. The change of OPD values in the two periods. (a), the OPD values in the 2000-2014 and 2015-2019; (b), the percentage change of OPD values between the two periods. SLO refers to slope, ELE refers to elevation, EVI refers to enhanced vegetation index, NDVI refers to Normalized Difference Vegetation Index, TEM refers to temperature, PRE refers to precipitation, EVA refers to Evaporation, SM refers to snowmelt, RO refers to runoff.

4.3. Impacts of the interactions of variable pairs
The proposed LESH model can reveal the contribution of each variable in the interactions of multiple variables. The results show that the interactions between topographic variables and other variables had the strongest explanatory power for the wetland distribution (). Environmental and climatic variables play a secondary role in explaining the distribution of wetlands. Among the interactions involving nontopographic variables, the interactive effect of NDVI and TEM had the greatest impact on the wetland distribution. The Ω values were 0.40 and 0.43 in the 2000-2014 and 2015-2019 periods, respectively.
Figure 8. SHAP power of determinants (SPD) values between two variables. (a), in the 2000-2014; (b), in the 2015-2019. The pie chart proportions correspond to variables on the x- and y-axes and are distinguished by colors. SLO refers to slope, ELE refers to elevation, EVI refers to enhanced vegetation index, NDVI refers to Normalized Difference Vegetation Index, TEM refers to temperature, PRE refers to precipitation, EVA refers to Evaporation, SM refers to snowmelt, RO refers to runoff.

It is noteworthy that some nonlinearly enhanced interactions between the variables were detected, as evidenced by the interactive Ω value being greater than the sum of the individual Ω values of the two variables. For example, the interactions between evaporation and other variables exhibited nonlinearly enhanced effects. In the 2000-2014 period, the Ω value of evaporation and temperature was 0.325, of which evaporation accounted for 0.141 and temperature accounted for 0.184. When the individual variables were used, the Ω value of temperature was 0.09 and that of evaporation was 0.05. In the 2015-2019 period, the Ω value of evaporation and slope was 0.71 (0.62 for slope and 0.09 for evaporation). When the individual variables were used, the Ω value of the slope was 0.59 and that of evaporation was 0.05. In addition, we found that the higher the correlation between variables was, the weaker the interaction-derived enhancement was. For example, NDVI and EVI exhibited high correlations, with correlation coefficients of 0.98, while the interaction between these two variables was very weak.
For the temporal pattern, the topographic variables (e.g. elevation, slope) accounted for significantly lower proportions of the pairwise interactions between variables in the 2015-2019 period than in the 2000-2014 period (). This finding was consistent with the results of the individual variables analysis, i.e. increased wetlands were not significantly correlated with the topographic variables.
4.4. Optimal variable combinations and heterogeneous spatial partitioning
The geographically optimal wetland distribution zones in the two periods were detected by the LESH model. As shown in , in the 2000-2014 period, four variables, the slope, elevation, runoff, and precipitation, were used to identify the 12 wetland zones on the TP. All zones could be divided into two groups based on slope. The first group included zones A and B with slopes greater than 5.5°. These zones were located mainly in the south-eastern region and along the margins of the TP, where a large number of high mountains are distributed, resulting in steep gradients at large scales (120 km). The wetlands here are predominantly low-density riverine wetlands. The second group was composed of the remaining zones, which had slopes less than 5.5°. These zones were located mainly in the central TP, that is, the source region of the three rivers and the Qiangtang Plateau. Plateaus dominate this region with small changes in slope, and the factors that control the distribution of wetlands include multiple variables.
Figure 9. Geographically optimal results of the LESH model. (a), Geographically optimal wetland zones on the TP in 2000-2014; (b), the decision tree of identifying optimal zones; (c), and statistical summaries of explanatory variables in each optimal zone. SLO refers to slope, ELE refers to elevation, PRE refers to precipitation, RO refers to runoff.

During the period from 2015 to 2019, wetlands on the TP were grouped into 11 regions by five variables: the slope, elevation, EVI, temperature, and NDVI (). The rules for dividing the zones in 2015-2019 differed from those applied in 2000-2014. For example, the region that was divided into regions E and I in 2015-2019 was divided into five regions (C, E, F, G, J) in 2000-2004, with a more fragmented distribution. Climate change on the TP has also led to changes in division rules even though the same regions, for example, the division rules between region K and the other regions were dominated by the slope until 2014 but became temperature-dependent from 2015-2019.
Figure 10. Geographically optimal results of the LESH model. (a), Geographically optimal wetland zones on the TP in 2000-2014; (b), the decision tree of identifying optimal zones; (c), the statistical summaries of explanatory variables in each optimal zone. SLO refers to slope, ELE refers to elevation, EVI refers to enhanced vegetation index, NDVI refers to Normalized Difference Vegetation Index, TEM refers to temperature.

shows the SPD values of the optimal variable combinations that determine the geographically optimal zones in the two periods. In 2000-2014, the interactions of four variables explained 77.3% of the wetland distribution on the TP, a significantly greater proportion than that explained by single variables or when using all nine variables. The slope was the most dominant variable, contributing 41% of the explanatory power, and the elevation variable contributed 25%. Runoff and precipitation contributed the remaining 10% of the explanatory power. From 2000 to 2014, the wetland area did not change extensively, so static variables such as the slope and elevation contributed largely to the wetland distribution.
Figure 11. The SPD values of key variables. (a) shows the SPD values in 2000-2014; (b) shows the SPD values in 2015-2019.

During the 2015-2019 period, the five variables of the slope, elevation, EVI, temperature, and NDVI divided the TP into 11 regions. The interactions among these five variables explained 75.1% of the wetland distribution on the TP. Unlike the previous 15 years, the explanatory powers of the slope and elevation on wetland distribution decreased to 33% and 19%, respectively. The EVI and temperature became the main explanatory factors during the period of rapid wetland growth, contributing 9% and 7%, respectively.
5. Discussion
5.1. Methodological contributions
This study proposed a LESH model to investigate the linkages between the wetland distribution and geographical variables on the TP. Compared with other methods (), the LESH model has the following advantages.
Table 2. Comparison of several commonly used methods.
First, the LESH model can accurately calculate the spatial associations between geographical variables and the wetland distribution, including linear and nonlinear relationships. However, linear regression models cannot capture the nonlinear relationships between variables.
Second, the LESH model explores spatial associations by considering the interaction among explanatory variables. Geographical variables often exhibit complex interrelationships and are seldom independent of each other (Song and Wu Citation2021). Therefore, for linear models, non-independent variables can result in unstable outcomes, reduced explanatory power, and even erroneous conclusions (Brauer and Curtin Citation2018).
Third, the LESH model can fairly allocate the contributions of each variable to the spatiotemporal distribution of wetlands. SPD value provides a consistent and objective approach to discerning the variable with the most substantial influence. The SPD value based on Shapley value is the only method of contribution assignment that can satisfy several desirable theoretical properties.
Finally, the LESH model is interpretable throughout the process, including decision tree-based OPD calculation and optimal zones identification, and variable contribution assignment based on Shapley value. It should be mentioned that GWR is the only algorithm among those compared that can map out the spatial correlation within local regions (Local spatial variations).
The LESH model can also be applied to study similar problems, especially those involving complex interactions among multiple variables. The LESH model is desirable for calculating the contribution of each variable and the interactions between variables and can be used in factor analyses, driving force explorations, and other applications in different fields, such as the natural sciences, social sciences, and environmental pollution (Wang and Xu Citation2017; Guo et al. Citation2022).
5.2. Limitations and interpretations of the findings
The proposed LESH model investigated the associations between the response variable and explanatory variables based on statistical data analysis rather than by inferring causality from the mechanism. Based on the LESH model, the related factors of the wetland spatiotemporal variation were detected. We obtained four main findings:
First, 120-km was found to be a suitable scale for exploring the relationships between the wetland distribution and environmental variables on the TP. Under this condition, the spatial associations between wetlands and environmental variables are highly significant.
Second, we found that topographic variables were the most important variables determining the spatial distribution of wetlands on the TP, while climate variables were important in controlling the increased wetland areas. Temperature had an essential effect on the wetland area changes. Over the past few decades, the TP has generally become increasingly warm and wet (Kuang and Jiao Citation2016). As a region sensitive to global climate change, the TP is warming more rapidly than the worldwide average (Duan and Xiao Citation2015). The period from 2015 to 2019 was the hottest five-year period on record (Global Climate Status Statement 2019). The increased glacier meltwater and earlier permafrost thawing due to global warming have provided more abundant water recharge, resulting in the formation of new wetlands. The wetland data and explanatory variables we used were both derived from the mean values of September-October, which might introduce a bias in the result. The TP receives most of its precipitation in summer (Zhu and Sang Citation2018), so a greater influence of temperature and precipitation on wetlands would be found using summer data. Nonetheless, considering that we used multi-year data and focused on the interannual variation of wetlands, this mitigates the uncertainty caused by the data time of September-October.
Third, we investigated the interactions between two variables and the contribution of each variable. The spatiotemporal variabilities in wetlands are influenced by complex geographical factors and their interactions. The results show that the interactions of topographic variables with other variables have the strongest explanatory powers for the wetland distribution. Among the interactions involving nontopographic variables, the synergistic effect of the NDVI and TEM variables had the greatest influence on wetland distribution. In addition, nonlinear enhancement effects were observed between evaporation and several other variables, such as between evaporation and temperature, evaporation and precipitation, and evaporation and NDVI. Although there is a complex feedback mechanism between wetlands and vegetation, vegetation is more likely to respond to wetland changes rather than being the driver of wetland spatiotemporal variabilities.
Finally, we identified the geographically optimal wetland distribution zones in two periods using the LESH model. We identified the major factors influencing wetlands in different regions within different periods, thereby enhancing our knowledge and understanding of the drivers of the spatiotemporal pattern of TP wetlands.
Our study also has the following limitation: the impact of human activities is not considered due to a lack of quantifiable data on the entire study area. Previous research shows that agricultural activities are an important driver in the decline of wetlands (Nie and Li Citation2011). However, compared to the climate change on the TP wetlands, the impact of human activities may be very limited (Chen et al. Citation2013).
6. Conclusion
In this study, a locally explained heterogeneity model was proposed to explore the heterogeneity of the spatiotemporal distribution of wetlands on the TP from 2000 to 2019. The LESH model can reveal the maximum spatial associations between the wetland density and multiple related variables and can fairly distribute the contributions of each variable and the interactions among multiple variables. Based on this model, we sought to improve our general understanding of how (and which) spatial factors are related to the wetland distribution and the extent to which the variation in the wetland distribution across the TP can be explained by geographical factors.
The results of the spatial patterns of wetland density variabilities in different phases obtained with the LESH model show that topographic variables (slope and elevation) were the most important variables determining the spatial distribution of wetlands on the TP, and temperature was an important reason for the increased wetland area observed from 2015 to 2019 on the TP. Multivariate interactions increased the explanatory power of the model to wetland distribution on the TP, and the interactions of the evaporation variable with other variables had enhancement effects.
This study enriches the theory of spatial stratification heterogeneity and analyses the wetland distribution heterogeneity in the TP over the past 20 years. Knowledge of wetland distribution determinants can help us understand the development and evolution of wetlands and the impacts of climate change on wetlands. The results of optimal wetland zoning can comprehensively reflect the regional natural geographic characteristics, provide a basis for the regional division of the TP, and serve biodiversity protection and nature reserve construction.
Disclosure statement
No potential conflict of interest was reported by the author(s).
Data availability statement
The data and code used in this study dataset are available by contacting the corresponding author.
Additional information
Funding
References
- Braswell, Anna E., and James B. Heffernan. 2019. “Coastal Wetland Distributions: Delineating Domains of Macroscale Drivers and Local Feedbacks.” Ecosystems 22 (6): 1256–1270. https://doi.org/10.1007/s10021-018-0332-3.
- Brauer, Markus, and John J. Curtin. 2018. “Linear Mixed-Effects Models and the Analysis of Nonindependent Data: A Unified Framework to Analyze Categorical and Continuous Independent Variables That Vary Within-Subjects and/or Within-Items.” Psychological Methods 23 (3): 389–411. https://doi.org/10.1037/met0000159.
- Cao, Shixiong, and Junze Zhang. 2015. “Political Risks Arising from the Impacts of Large-Scale Afforestation on Water Resources of the Tibetan Plateau.” Gondwana Research 28 (2): 898–903. https://doi.org/10.1016/j.gr.2014.07.002.
- Chatterjee, Kajal, Abhirup Bandyopadhyay, Amitava Ghosh, and Samarjit Kar. 2015. “Assessment of Environmental Factors Causing Wetland Degradation, Using Fuzzy Analytic Network Process: A Case Study on Keoladeo National Park, India.” Ecological Modelling 316: 1–13. https://doi.org/10.1016/j.ecolmodel.2015.07.029.
- Chen, Lei, Yong Gao, Di Zhu, Yihong Yuan, and Yu Liu. 2019. “Quantifying the Scale Effect in Geospatial Big Data Using Semi-Variograms.” PLoS ONE 14 (11): e0225139.
- Chen, Huai, Qiuan Zhu, Changhui Peng, Ning Wu, Yanfen Wang, Xiuqing Fang, Yongheng Gao, et al. 2013. “The Impacts of Climate Change and Human Activities on Biogeochemical Cycles on the Qinghai-Tibetan Plateau.” Global Change Biology 19 (10): 2940–2955. https://doi.org/10.1111/gcb.12277.
- Chmura, Gail L., Shimon C. Anisfeld, Donald R. Cahoon, and James C. Lynch. 2003. “Global Carbon Sequestration in Tidal, Saline Wetland Soils.” Global Biogeochemical Cycles 17 (4): 1111.
- Cohen, Matthew J, Irena F Creed, Laurie Alexander, Nandita B Basu, Aram JK Calhoun, Christopher Craft, Ellen D’Amico, et al. 2016. “Do Geographically Isolated Wetlands Influence Landscape Functions?” Proceedings of the National Academy of Sciences 113 (8): 1978–1986. https://doi.org/10.1073/pnas.1512650113.
- Datta, Anupam, Shayak Sen, and Yair Zick. 2016. “Algorithmic Transparency via Quantitative Input Influence: Theory and Experiments with Learning Systems.” In 2016 IEEE Symposium on Security and Privacy (SP), 598–617. IEEE.
- Didan, Kamel, Armando Barreto Munoz, and Alfredo Huete. 2015. “MODIS Vegetation Index User’s Guide (MOD13 Series).”.
- Duan, Anmin, and Zhixiang Xiao. 2015. “Does the Climate Warming Hiatus Exist Over the Tibetan Plateau?” Scientific Reports 5 (1): 13711. https://doi.org/10.1038/srep13711.
- Farr, Tom G., and Mike Kobrick. 2000. “Shuttle Radar Topography Mission Produces a Wealth of Data.” Eos, Transactions American Geophysical Union 81 (48): 583–585. https://doi.org/10.1029/EO081i048p00583.
- Gall, Heather E, Jeryang Park, Ciaran J Harman, James W Jawitz, P. Suresh, and C. Rao. 2013. “Landscape Filtering of Hydrologic and Biogeochemical Responses in Managed Catchments.” Landscape Ecology 28 (4): 651–664. https://doi.org/10.1007/s10980-012-9829-x.
- Guo, Jiangang, Jinfeng Wang, Chengdong Xu, and Yongze Song. 2022. “Modeling of Spatial Stratified Heterogeneity.” GIScience & Remote Sensing 59 (1): 1660–1677. https://doi.org/10.1080/15481603.2022.2126375.
- Hu, Shengjie, Zhenguo Niu, Yanfen Chen, Lifeng Li, and Haiying Zhang. 2017. “Global Wetlands: Potential Distribution, Wetland Loss, and Status.” Science of The Total Environment 586 (May): 319–327. https://doi.org/10.1016/j.scitotenv.2017.02.001.
- Jacoby, William G. 2000. “Loess: A Nonparametric, Graphical Tool for Depicting Relationships between Variables.” Electoral Studies 19 (4): 577–613. https://doi.org/10.1016/S0261-3794(99)00028-1.
- Kang, ShiChang, YongJun Zhang, DaHe Qin, JiaWen Ren, QiangGong Zhang, Bjorn Grigholm, and Paul A. Mayewski. 2007. “Recent Temperature Increase Recorded in an Ice Core in the Source Region of Yangtze River.” Chinese Science Bulletin 52 (6): 825–831. https://doi.org/10.1007/s11434-007-0140-1.
- Kuang, Xingxing, and Jiu Jimmy Jiao. 2016. “Review on Climate Change on the Tibetan Plateau During the Last Half Century.” Journal of Geophysical Research: Atmospheres 121 (8): 3979–4007. https://doi.org/10.1002/2015JD024728.
- Li, Yang, Zhengyang Hou, Liqiang Zhang, Ying Qu, Guoqing Zhou, Jintai Lin, Jingwen Li, and Ke Huang. 2023a. “Long-Term Spatio-Temporal Changes of Wetlands in Tibetan Plateau and Their Response to Climate Change.” International Journal of Applied Earth Observation and Geoinformation 121 (July): 103351. https://doi.org/10.1016/j.jag.2023.103351.
- Li, Yang, Zhengyang Hou, Liqiang Zhang, Changqing Song, Shilong Piao, Jintai Lin, Shushi Peng, et al. 2023b. “Rapid Expansion of Wetlands on the Central Tibetan Plateau by Global Warming and El Niño.” Science Bulletin 68 (5): 485–488. https://doi.org/10.1016/j.scib.2023.02.021.
- Lundberg, Scott M., Gabriel Erion, Hugh Chen, Alex DeGrave, Jordan M. Prutkin, Bala Nair, Ronit Katz, Jonathan Himmelfarb, Nisha Bansal, and Su-In Lee. 2020. “From Local Explanations to Global Understanding with Explainable AI for Trees.” Nature Machine Intelligence 2 (1): 56–67. https://doi.org/10.1038/s42256-019-0138-9.
- Lundberg, Scott M, and Su-In Lee. 2017. “A Unified Approach to Interpreting Model Predictions.” In Advances in Neural Information Processing Systems 30: 4765–4774.
- Luo, Peng, Yongze Song, Xin Huang, Hongliang Ma, Jin Liu, Yao Yao, and Liqiu Meng. 2022. “Identifying Determinants of Spatio-Temporal Disparities in Soil Moisture of the Northern Hemisphere Using a Geographically Optimal Zones-Based Heterogeneity Model.” ISPRS Journal of Photogrammetry and Remote Sensing 185 (March): 111–128. https://doi.org/10.1016/j.isprsjprs.2022.01.009.
- Luo, Peng, Yongze Song, and Peng Wu. 2021. “Spatial Disparities in Trade-Offs: Economic and Environmental Impacts of Road Infrastructure on Continental Level.” GIScience & Remote Sensing 58 (5): 756–775. https://doi.org/10.1080/15481603.2021.1947624.
- Luo, Peng, Yongze Song, Di Zhu, Junyi Cheng, and Liqiu Meng. 2023. “A Generalized Heterogeneity Model for Spatial Interpolation.” International Journal of Geographical Information Science 37 (3): 634–659. https://doi.org/10.1080/13658816.2022.2147530.
- Mitsch, William J., Blanca Bernal, Amanda M. Nahlik, Ülo Mander, Li Zhang, Christopher J. Anderson, Sven E. Jørgensen, and Hans Brix. 2013. “Wetlands, Carbon, and Climate Change.” Landscape Ecology 28 (4): 583–597. https://doi.org/10.1007/s10980-012-9758-8.
- Moshir Panahi, Davood, Georgia Destouni, Zahra Kalantari, and Bagher Zahabiyoun. 2022. “Distinction of Driver Contributions to Wetland Decline and Their Associated Basin Hydrology Around Iran.” Journal of Hydrology: Regional Studies 42 (August): 101126.
- Muñoz-Sabater, Joaquín, Emanuel Dutra, Anna Agustí-Panareda, Clément Albergel, Gabriele Arduini, Gianpaolo Balsamo, Souhail Boussetta, et al. 2021. “ERA5-Land: A State-of-the-Art Global Reanalysis Dataset for Land Applications.” Earth System Science Data 13 (9): 4349–4383. https://doi.org/10.5194/essd-13-4349-2021.
- Nie, Yong, and Ainong Li. 2011. “Assessment of Alpine Wetland Dynamics from 1976–2006 in the Vicinity of Mount Everest.” Wetlands 31 (5): 875–884. https://doi.org/10.1007/s13157-011-0202-7.
- Russi, Daniela. 2013. “Paper Citation: Russi D., Ten Brink P., Farmer A., Badura T., Coates D., Förster J., Kumar R. and Davidson N.(2013) The Economics of Ecosystems and Biodiversity for Water and Wetlands. IEEP, London and Brussels; Ramsar Secretariat, Gland.”.
- Shapley, Lloyd S. 1953. “A Value for N-Person Games.” Princeton University Press Princeton: 307-317.
- Song, Yongze, Jinfeng Wang, Yong Ge, and Chengdong Xu. 2020. “An Optimal Parameters-Based Geographical Detector Model Enhances Geographic Characteristics of Explanatory Variables for Spatial Heterogeneity Analysis: Cases with Different Types of Spatial Data.” GIScience & Remote Sensing 57 (5): 593–610. https://doi.org/10.1080/15481603.2020.1760434.
- Song, Yongze, and Peng Wu. 2021. “An Interactive Detector for Spatial Associations.” International Journal of Geographical Information Science 35 (8): 1676–1701. https://doi.org/10.1080/13658816.2021.1882680.
- Tian, Aohua, Tingting Xu, Jay Gao, Chang Liu, and Letao Han. 2023. “Multi-Scale Spatiotemporal Wetland Loss and Its Critical Influencing Factors in China Determined Using Innovative Grid-Based GWR.” Ecological Indicators 149 (May): 110144.
- Truong, Charles, Laurent Oudre, and Nicolas Vayatis. 2020. “Selective Review of Offline Change Point Detection Methods.” Signal Processing 167 (February): 107299.
- Wang, Rui, Min He, and Zhenguo Niu. 2020. “Responses of Alpine Wetlands to Climate Changes on the Qinghai-Tibetan Plateau Based on Remote Sensing.” Chinese Geographical Science 30 (2): 189–201. https://doi.org/10.1007/s11769-020-1107-2.
- Wang, Chao, Le Ma, Yan Zhang, Nengcheng Chen, and Wei Wang. 2022. “Spatiotemporal Dynamics of Wetlands and Their Driving Factors Based on PLS-SEM: A Case Study in Wuhan.” Science of The Total Environment 806 (February): 151310.
- Wang, Jinfeng, and Chengdong Xu. 2017. “Geodetector: Principle and Prospective.” Acta Geographica Sinica 72 (1): 116–134.
- Were, David, Frank Kansiime, Tadesse Fetahi, Ashley Cooper, and Charles Jjuuko. 2019. “Carbon Sequestration by Wetlands: A Critical Review of Enhancement Measures for Climate Change Mitigation.” Earth Systems and Environment 3 (2): 327–340. https://doi.org/10.1007/s41748-019-00094-0.
- Wu, Jianguo. 2004. “Effects of Changing Scale on Landscape Pattern Analysis: Scaling Relations.” Landscape Ecology 19 (2): 125–138. https://doi.org/10.1023/B:LAND.0000021711.40074.ae.
- Xi, Yi, Shushi Peng, Philippe Ciais, and Youhua Chen. 2020. “Future Impacts of Climate Change on Inland Ramsar Wetlands.” Nature Climate Change 11 (1): 45–51. https://doi.org/10.1038/s41558-020-00942-2.
- Xu, Ting, Baisha Weng, Denghua Yan, Kun Wang, Xiangnan Li, Wuxia Bi, Meng Li, Xiangjun Cheng, and Yinxue Liu. 2019. “Wetlands of International Importance: Status, Threats, and Future Protection.” International Journal of Environmental Research and Public Health 16 (10): 1818. https://doi.org/10.3390/ijerph16101818.
- Xue, Zhenshan, Xianguo Lyu, Zhike Chen, Zhongsheng Zhang, Ming Jiang, Kun Zhang, and Yonglei Lyu. 2018. “Spatial and Temporal Changes of Wetlands on the Qinghai-Tibetan Plateau from the 1970s to 2010s.” Chinese Geographical Science 28 (6): 935–945. https://doi.org/10.1007/s11769-018-1003-1.
- Yao, Tandong, Xiaodong Liu, Ninglian Wang, and Yafeng Shi. 2000. “Amplitude of Climatic Changes in Qinghai-Tibetan Plateau.” Chinese Science Bulletin 45 (13): 1236–1243. https://doi.org/10.1007/BF02886087.
- Zhang, Guoqing, Tandong Yao, Hongjie Xie, Kun Yang, Liping Zhu, C. K. Shum, Tobias Bolch, Shuang Yi, Simon Allen, and Liguang Jiang. 2020. “Response of Tibetan Plateau Lakes to Climate Change: Trends, Patterns, and Mechanisms.” Earth-Science Reviews 208: 103269. https://doi.org/10.1016/j.earscirev.2020.103269.
- Zhang, Wenjiang, Yonghong Yi, Kechao Song, John S. Kimball, and Qifeng Lu. 2016a. “Hydrological Response of Alpine Wetlands to Climate Warming in the Eastern Tibetan Plateau.” Remote Sensing 8 (4): 336. https://doi.org/10.3390/rs8040336.
- Zhang, Zhen, Niklaus E. Zimmermann, Jed O. Kaplan, and Benjamin Poulter. 2016b. “Modeling Spatiotemporal Dynamics of Global Wetlands: Comprehensive Evaluation of a New Sub-Grid TOPMODEL Parameterization and Uncertainties.” Biogeosciences (online) 13 (5): 1387–1408. https://doi.org/10.5194/bg-13-1387-2016.
- Zhao, Zhilong, Yili Zhang, Linshan Liu, Fenggui Liu, and Haifeng Zhang. 2015. “Recent Changes in Wetlands on the Tibetan Plateau: A Review.” Journal of Geographical Sciences 25 (7): 879–896. https://doi.org/10.1007/s11442-015-1208-5.
- Zhu, Yanxin, and Yanfang Sang. 2018. “Spatial variability in the seasonal distribution of precipitation on the Tibetan Plateau.” Progress in Geography 37 (11): 1533–1544. https://doi.org/10.18306/dlkxjz.2018.11.009.