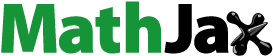
ABSTRACT
Remote sensing technology plays a critical role in evaluating large-scale ecological restoration efforts. However, previous studies tend to focus on specific aspects of ecological restoration, such as water conservation, vegetation restoration, or land rehabilitation. As a sustainable development demonstration city in China, Taiyuan has undertaken numerous ecological projects to mitigate environmental degradation caused by its long-standing energy-driven economic expansion. To comprehensively evaluate Taiyuan’s ecological restoration efforts, this study used remote sensing technology to assess its progress from the SDGs perspectives, such as water conservation (SDG 6.3.2), air quality improvement (SDG 11.6.2), and ecosystem preservation (SDG 6.6.1, 15.1.1, 15.3.1, 15.4.2). Firstly, ecological restoration indicators were selected from the aforementioned SDGs perspectives. Secondly, a new vegetation growth condition index (VGCI) was developed to accurately monitor changes in vegetation growth. Finally, Taiyuan’s ecological restoration efforts were evaluated based on the selected indicators. The results showed that Taiyuan has made significant progress in ecological restoration, including improved water and air quality, increased forest coverage, and restored mine’s vegetation, likely due to its ecological projects. This study provides a useful framework for evaluating ecological restoration efforts and advancing sustainability goals.
1. Introduction
To mitigate ecosystem degradation caused by global climate changes and human activities, the United Nations general assembly has announced the ‘United Nations Decade on Ecosystem Restoration (2021–2030)’ resolution (Waltham et al. Citation2020), which confirms ecological restoration as a critical nature-based approach for achieving Sustainable Development Goals (SDGs) (Chen et al. Citation2022). Ecological restoration has emerged as a paramount global priority for conserving biodiversity, combating desertification and land degradation, and mitigating the impacts of anthropogenic climate change (Aronson and Alexander Citation2013). This critical importance is widely acknowledged by international conventions (Convention on Biological Diversity Citation2012), intergovernmental platforms (Murcia et al. Citation2016), and policies that emphasize the urgent need for substantial action (De Pinto et al. Citation2020). The 2030 Agenda for Sustainable Development also calls for the protection and restoration of ecosystems, which states that ‘we are determined to conserve and sustainably use oceans and seas, freshwater resources, as well as forests, mountains and drylands and to protect biodiversity, ecosystems and wildlife’ (Resolution Citation2015). Therefore, many governments have developed a variety of ecosystem preservation and restoration projects to mitigate ecological damage and achieve SDGs (Cao Citation2011; Doren et al. Citation2009; Ning et al. Citation2022; Petursdottir et al. Citation2013; Wang, Song, et al. Citation2022). For example, the South Florida ecosystem restoration task group has announced the commencement of the multibillion- dollar Comprehensive Everglades Restoration Plan, which promotes biodiversity conservation and ecological restoration (SDG 15) in the Florida Everglades (Doren et al. Citation2009). Iceland has supported and subsidized the restoration of degraded pastures and improved land management. These measures have resulted in improving the state of damaged ecosystems and maintaining ecosystem health, and contributed to the realization of regional SDG15 (Petursdottir et al. Citation2013). China has made significant progress in ecological restoration and achieving SDGs by implementing a series of large-scale ecological projects since 1978, including the Three Norths Shelter Forest Program (TNSFP), Natural Forest Protection Project (NFPP), Combating Desertification Program (CDP), and Grain for Green Project (GGP) (Jia et al. Citation2014; Li et al. Citation2012; Wu et al. Citation2013). The outcomes of these initiatives are promising, leading to a substantial increase in global greenery by approximately 25% (Chen et al. Citation2019).
Taiyuan is one of the National Innovation-driven Demonstration Zones (NIDDZ) of China, which was established in 2016 as China’s key initiative to promote regional sustainable development (Wang, Yuan, and Lu Citation2020). Like many resource-based cities, Taiyuan has relied on energy resources to drive economic development, resulting in many environmental problems and ecosystem degradation (Zhang et al. Citation2020). In recent years, with the strong support of China, Taiyuan has actively carried out many ecological restoration projects to deal with ecological damage, and the progress of ecological restoration in Taiyuan as a result of implementation of ecological projects should be evaluated.
Water, air, land and biogenic factors are important components of an ecosystem, and there are complex interactions and mutual influences among them. For example, the efficiency of phytoremediation depends on plant species-specific metabolism, including air absorption and photosynthesis, diversity of soil microorganisms and heavy metal uptake (Wei et al. Citation2021). Therefore, the comprehensive evaluation of ecological restoration should involve water, air, land and biogenic factors, etc.
Remote sensing (RS) technology has been widely used in the evaluation of ecological restoration in large areas due to its characteristics of wide coverage and high temporal resolution (Chen et al. Citation2021; del Río-Mena et al. Citation2020; Feng et al. Citation2013; Xia et al. Citation2021). However, most current studies focus on a certain aspect of ecological restoration assessment, such as water environmental protection (Dekker and Hestir Citation2012; Devlin, Bricker, and Painting Citation2011; Ferreira et al. Citation2011; Park, Ruddick, and Lacroix Citation2010), vegetation restoration (Lin et al. Citation2020; Zhai et al. Citation2020), or land restoration (Li, Xu, and Li Citation2020; Liu et al. Citation2020; Zucca et al. Citation2015). For water environmental protection, some studies employ RS technology to measure water quality indicators. For example, Kapalanga et al. (Citation2021) developed a RS-based water quality monitoring algorithm to evaluate water quality in the Olushandja Dam, north-central Namibia, and Hasab et al. evaluated water quality in the marsh area of southern Iraq (Hasab et al. Citation2020). In addition, some studies have monitored vegetation dynamic changes based on RS. For example, Wang et al. (Wang, Han, et al. Citation2022) analyzed the impact of ecological restoration on the temporal and spatial distribution of Tamarix Forest in Changyi, China, and Guo et al. (Guo et al. Citation2022) evaluated the impact of climate change and ecological restoration policies on vegetation changes in the Three-River Headwaters region of China. Similarly, RS is often used to assess land degradation. For example, Michele et al. (de Oliveira et al. Citation2022) assessed the impact of land degradation and drought on terrestrial ecosystems in northeastern Brazil, and Dubovyk (Dubovyk Citation2017) reviewed the important role of RS in the assessment of land degradation and assessed land degradation in a region of Uzbekistan. These studies all concentrated on one aspect of ecological restoration, and there is a lack of a comprehensive evaluation method for ecological restoration.
In order to conduct a comprehensive evaluation of ecological restoration, this study specifically targets several SDGs that are closely linked to ecological restoration. These include SDG 6 (6.3.2, 6.6.1 for water protection), SDG 11 (11.6.2 for air purification), and SDG 15 (15.1.1, 15.3.1, 15.4.2 for land restoration and vegetation restoration). Additionally, SDG 15.4.1 proposes the MGCI as a tool for assessing the level of greenery in mountainous regions. However, a mere consideration of green cover area without taking into account the status of vegetation growth falls short in accurately evaluating the progress of greening efforts in such areas. Hence, there is a pressing need for an index that can holistically incorporate both the extent of green cover and the state of vegetation growth in mountainous regions in this study. By embracing the SDG framework, our study aims to comprehensively assess the ecological restoration endeavors in Taiyuan, with a specific focus on addressing key ecological issues. These include water pollution, air pollution, land degradation, and the destruction of mine’s vegetation. Finally, this study also discussed the causal relationship between Taiyuan’s ecological restoration and the conducted ecological projects. Through our evaluation, we seek to gain a deep and comprehensive understanding of the ongoing ecological restoration efforts in the region, thereby contributing to a more holistic approach towards environmental rejuvenation.
2. Materials and methods
2.1. Study area
Taiyuan of Shanxi Province, China, is located at 111°30′ E – 113°09′ E and 37°27′ N – 38°25′ N, covering an area of 6,911 km2 (). It is surrounded by Xishan (west) Mountain, Dongshan (east) Mountain and Beishan (north) Mountain. Taiyuan has a semiarid climate with an average annual temperature of approximately 8°C, annual rainfall of approximately 450 mm, and annual evaporation of 1045 mm over the last 40 years (Ma et al. Citation2011). Taiyuan is one of the first NIDDZ, which was established in 2016 as China’s key initiative to promote regional sustainable development at the municipal level, promote ecological restoration and implement the 2030 Agenda for Sustainable Development (Wang, Yuan, and Lu Citation2020). The prolonged dependence on energy resources for economic development in Taiyuan has given rise to a diverse array of ecological challenges faced by the city. First, Taiyuan’s water resources, water environment, and water ecology have been threatened after the high-intensity development of the resource-based economy. Second, Taiyuan’s energy supply structure is dominated by coal and other fossil fuels, which leads to air quality deterioration (Taiyuan Citation2021; Zhang et al. Citation2011). Finally, the Taiyuan Xishan coalfield is rich in mineral resources, making it one of the six coalfields in Shanxi Province. Its high-intensity coal mining not only causes a series of land subsidences but also damages the local ecological environment.
Figure 1. The geographical distribution map of Taiyuan and its implemented ecological projects. The base image is a Landsat-8 true-color compositing image.

In recent years, Taiyuan has been exploring and innovating the transformation of resource-based economy and the restoration of the natural environment, and has conducted a number of ecological projects () to address these ecological problems, including water, air quality management, land restoration, and vegetation restoration (Gaubatz and Hanink Citation2020). The geographical location of these ecological projects is shown in .
Table 1. Ecological projects related to ecosystem restoration conducted in Taiyuan in recent years.
These ecological projects were completed at the end of 2020, and their benefits to the ecological restoration of Taiyuan need to be assessed. In addition, due to the different implementation periods of ecological projects, this study selects different time periods to evaluate the impact of these ecological projects on the ecological restoration of Taiyuan such as water environmental protection (2015–2020), air purification (2015–2020), land restoration (2010–2020) and vegetation restoration (2010–2020). In addition, the mine restoration projects in Dongshan and Xishan (ECMPXD) are highly representative and have garnered significant attention. The ‘Xishan Model,’ as a prime example, has been featured in the ‘Progress Report on China’s Implementation of the 2030 Agenda for Sustainable Development. 2021’ and has been disseminated nationally and globally (Center for International Knowledge on Development Citation2021). Therefore, this study selected Xishan and Dongshan as the study areas for mine conducting the mine ecological assessment. Conversely, the majority of other projects encompass broader scopes, often spanning entire cities or extensive regions. Hence, the study chosen the entire city as the overarching study area for these more comprehensive projects.
2.2. Data sources and processing
Using RS data, this study comprehensively evaluates the ecological restoration of Taiyuan under the perspective of SDGs. illustrates the technical route adopted in this study. The SDGs related to ecological restoration, encompassing water protection (SDG 6.3.2), air purification (SDG 11.6.2), land restoration, and vegetation restoration (SDG 6.6.1, 15.1.1, 15.3.1, 15.4.2), were selected as the basis for evaluating Taiyuan’s ecological restoration. Evaluation indicators, including water transparency (Secchi Disk Depth, SDD) to reflect water quality, air pollutant quantities to indicate air pollution changes, land cover change matrix to assess land degradation and restoration, and a new concept of VGCI to represent vegetation growth condition, were employed in this study.
In this study, satellite RS data and its product, and air pollutant data were used to calculate the key indicators of ecological restoration assessment, which mainly include SDD, VGCI, ratio of annual days with good air, and land degradation and restoration.
Landsat multispectral data (https://earthexplorer.usgs.gov/) were selected for SDD, leaf area index (LAI) and fractional vegetation cover (FVC) estimation (see supplementary materials for details). The acquired Landsat data are all reflectance products (Level-2) with a spatial resolution of 30 m, and only mask extraction and image stitching are performed. The Landsat-8 data in 2015 and 2020 are mainly used for SDD estimation, and the Landsat-5 data in 2010 are used for LAI and FVC estimation.
Hourly air pollutant data provided by the China National Environmental Monitoring Centre (http://www.cnemc.cn/) from 2015 to 2020 were selected to analyze the variation characteristics of air pollutant quantities in Taiyuan. Based on the observation data of 1563 air pollutant observation stations in China, the distribution of air pollutants in China was obtained by Kriging interpolation, and then the average concentration of air pollution in Taiyuan was extracted.
Globeland30 land cover products (www.globeland30.org) from 2010 and 2020 () were selected to analyze land degradation and restoration in Taiyuan.
2.3. Methods
2.3.1. Ecological restoration evaluation indicators from the SDGs perspective
Ecological restoration synergistically contributes to the achievement of the SDGs (Yao et al. Citation2021), directly or indirectly supporting the achievement of 10 of the 17 SDGs (see supplementary materials for details). Among all the relevant SDGs, the goals that most rely on ecological restoration are SDG15 (life on land), SDG 14 (life below water), and SDG 6 (clean water & sanitation), followed by SDG 11 (sustainable cities & communities), SDG 2 (zero hunger) and SDG 1 (no poverty) (Resolution Citation2015; Wood et al. Citation2018; Yang et al. Citation2020; Yao et al. Citation2021; Yin et al. Citation2021).
Based on RS technology, this study focuses on securing water resources (SDG 6.3.2), improving air quality (SDG 11.6.2), combating desertification (SDG 6.6.1, 15.3.1), and protecting vegetation (SDG 15.1.1, 15.4.2) to construct the ecological restoration evaluation indicators under SDGs perspectives (). Among them, the SDD, air pollutant quantities, the area of land degradation, the area of forest and vegetation parameters were selected as the evaluation indicators of ecological restoration.
Table 2. Ecological restoration evaluation indicators from the SDGs perspective.
2.3.2. The Secchi Disk Depth estimation algorithm
At present, with economic development and population growth, Taiyuan has an increasing demand for water resources, leading to a significant threat to the realization of SDGs [66]. Fenhe Reservoir, Fenhe Reservoir 2, and Jinyang Lake were the three largest water bodies in Taiyuan (), and their water quality needs to be focused on. SDD is a quantitative measure of the transparency of a water body, or the visibility in the vertical direction (Duntley and Preisendorfer Citation1952), which has been widely applied in diver visibility and climate change research (Lee et al. Citation2015). Studies have shown that the visible band of Landsat-8 OLI data could accurately estimate SDD (Lee et al. Citation2016). Therefore, Landsat-8 data could be selected for SDD estimation of the main water bodies (Lee et al. Citation2015) in the proposed ecological restoration evaluation method. Equation (1) was used to estimate the SDD of the main water bodies (with an area greater than 1 km2) in Taiyuan.
(1)
(1) where
is the SDD, which represents the transparency of the water body.
is the attenuation coefficient of diffuse reflection at the transparent window of the water body in the visible light domain (410–665 nm).
is the reflectance corresponding to this wavelength (410–665 nm).
and
are the absorption and backscattering coefficients of water, respectively.
is the subsurface counterpart of
(Lee, Carder, and Arnone Citation2002). By modeling the radiation transfer function,
is a function of the ratio of
(Gordon et al. Citation1988).
is the absorption coefficient of pure water and is assumed to be a constant.
is the contribution from nonwater constituents and is estimated empirically from the
spectrum.
(= 0.089 sr−1) and
(= 0.125 sr−1) are model constants (Lee, Carder, and Arnone Citation2002).
and
are the backscattering coefficients of pure water and particles, respectively. Additionally, the
values for other wavelengths are estimated from a power-law function (Gordon and Morel Citation1983).
is estimated empirically from the
spectrum.
and
are model parameters, and their values are 0.005, 4.26, 0.52, 10.8, and 0.265, respectively.
(in degrees) is the solar zenith angle. The
(absorption) and
(backscattering) coefficients of pure water for Landsat-8 visible bands are shown in supplementary materials.
2.3.3. Statistical methods for characterizing air pollutant quantities
The concentrations of air pollutants such as PM2.5, PM10, CO, SO2, NO2 and O3 and the annual percentage of days with good air could be selected as the evaluation indicators for evaluating air quality. In addition, these six types of air pollutants were used to calculate the air quality index (AQI) (Ministry of Environmental Protection of China Citation2012b). Furthermore, the Chinese National Ambient Air Quality Standards (NAAQS) were selected as the air quality evaluation standard to calculate the annual percentage of days with good air quality (Ministry of Environmental Protection of China Citation2012a; Zhao et al. Citation2016), in which the AQI of optimal air was lower than level I, and the AQI of good air was higher than level I and lower than level II (see supplementary materials for details).
2.3.4. Land degradation and restoration patterns
Land degradation is defined by the United Nations Convention to Combat Desertification (UNCCD) and the Inter-agency and Expert Group on SDG Indicators (IAEG-SDGs) as ‘the reduction or loss of the biological or economic productivity and complexity of rain fed cropland, irrigated cropland, or range, pasture, forest and woodlands resulting from a combination of pressures, including land use and management practices’ (Steenmans Citation2017). The land degradation neutrality (LDN) target is regarded as an important measure to address global ecosystem degradation and achieve SDGs (Aronson et al. Citation2020; Cowie et al. Citation2018; Dubey et al. Citation2021; Lu et al. Citation2019).
The Good Practice Guidance (GPG) document provides guidance on how to calculate the extent of land degradation for reporting on SDG 15.3.1: the proportion of land that is degraded over the total land area (Sims et al. Citation2021). The land cover change matrix provided by the GPG () shows the mutual conversion relationship between land cover types and discriminates whether the conversion relationship is restoration, degradation or stable. Since China is advancing the ‘Conversion of Cropland to Forest and Grassland Program’ (Xu et al. Citation2004), this study classifies ‘agricultural withdrawal’ as restoration and ‘agricultural expansion’ as degradation.
Table 3. The land cover change matrix used in this study.
2.3.5. The vegetation growth condition index
The Mountain Green Cover Index (MGCI) measures changes in the area of green vegetation in mountain areas (forest, shrubs and pasture land, and cropland), which can identify the status of mountain environment conservation to measure progress toward SDG Target 15.4. In the Metadata of SDG 15.4.2, the MGCI was defined as the ratio of the green vegetation areas to the mountain areas (Equation 2) of each country (Bian et al. Citation2022).
(2)
(2) where FA, SA, PA and CA are the forest, shrub, pastureland and cropland areas in a mountain area, respectively. LA is the total mountain area for each study area.
However, the MGCI can only reflect the changes in the green vegetation area and does not consider the changes in vegetation growth condition caused by natural vegetation growth or changed vegetation types. The LAI and FVC are two indicators for evaluating vertical and horizontal vegetation growth condition, respectively. LAI represents the total leaf area of vegetation per unit area, whereas FVC depicts the coverage of vegetation on the ground (Jia et al. Citation2016; Wang et al. Citation2020). Therefore, LAI and FVC are combined in this study to construct the new vegetation growth condition index (VGCI) to practically reflect the vegetation growth condition, which is defined as the leaf area possessed by a unit area of vegetation, expressed as Equation (3).
(3)
(3) where GA and LA are the green area (forest, shrub, pastureland and cropland) and the total mountain area for each study area, respectively. LAI and FVC are the mean values of LA.
Meanwhile, back propagation neural networks (BPNNs) (Foody Citation1999) have been employed as an efficient approach to predict crucial vegetation variables such as LAI and FVC (Baret et al. Citation2007). Therefore, BPNNs were selected to build the LAI and FVC estimation algorithm from Landsat-8 (Landsat-5) data based on MATLAB. The PROSAIL model simulations (Jia et al. Citation2016; Wang et al. Citation2020), which approximated the physical relationships between reflectance and FVC (or LAI) with a wide variety of land surface conditions, were used to generate the training samples for the BPNNs in this study. Green, red, and NIR band reflectance from the Landsat-8 data were the inputs of the BPNNs. The output was the corresponding LAI and FVC. The hidden layer’s node was set to 6. The activation functions in the hidden and output layers of the BPNNs were set to ‘sigmoid’ and ‘linear’, respectively. The Levenberg-Marquardt minimization algorithm was used to calibrate the synaptic coefficients because it had an efficient convergence capacity (Jia et al. Citation2015; Ngia and Sjoberg Citation2000). The learning dataset was randomly divided into two parts: 90% was used to train the BPNNs, while the remaining 10% was utilized to test hyper-specialization throughout the training process. In the training procedure, the performance criterion used mean squared error as an indication was set to 0.005. The training of BPNNs was finally finished after 414 iterations when the performance of the training achieved the intended goal.
3. Results
3.1. SDD estimation of the main water bodies in Taiyuan
Based on the Landsat-8 SDD estimation algorithm (Equation 1), this study estimated the SDD of these three major water bodies and other main water bodies (area greater than 1 km2) in Taiyuan () for 2015 and 2020 (). The main water bodies in Taiyuan present low SDD values of 27.62 and 24.89 cm for 2015 and 2020 (), respectively, which indicates that the main water bodies in Taiyuan are turbidites and continues to decline. In addition, compared with 2015, the SDD of Fenhe Reservoir and Fenhe Reservoir 2 in 2020 decreased by 7.54 and 3.34 cm, respectively, which is not a positive outcome regarding water environment protection in Taiyuan. It is commendable that the SDD of Jinyang Lake has been significantly improved from 27.07 cm in 2015 to 45.08 cm in 2020, indicating that the water quality has been greatly improved.
Similarly, the spatial distribution map of SDD indicates that the SDD of Fenhe Reservoir and Fenhe Reservoir 2 generally decreased from 2015 to 2020, while the central area of Jinyang Lake experienced a significant increase in SDD. As a result, the transparency of Taiyuan’s main water bodies remains poor overall. However, unlike other water bodies, the transparency of Jinyang Lake has been significantly improved.
3.2. Variation characteristics of air pollutant quantities in Taiyuan
The annual mean value of the air pollution concentration was generated to analyze the air quality in Taiyuan from 2015 to 2020 (). The ratio of annual days with good air (RAGA) is helpful in evaluating the overall air quality and identifying the major pollutants that cause air pollution ().
From 2015 to 2020, the annual average concentrations of CO and SO2 decreased, and RAGA increased year by year, which indicates that Taiyuan has achieved good performance in the control of CO and SO2 emissions. Distinct from CO and SO2, the annual average concentrations of PM2.5, PM10, and NO2 show a gradually improving trend after reaching the worst at the end of 2017. In addition, the RAGA values of PM2.5, PM10 and O3 have always been at a low level, which are the three major pollutants that cause poor air quality in Taiyuan. Fortunately, the RAGA values of PM2.5 and PM10 have increased significantly since 2018. However, the RAGA value of O3 has continued to decline, and it has gradually become the main culprit of air pollution in Taiyuan, which should be considered.
In conclusion, the overall air quality of Taiyuan has improved from 2015 to 2020. The concentration of air pollutants has decreased, and the RAGA has increased. However, the concentration of O3 is high and increasing gradually.
3.3. Variation status of land degradation and restoration in Taiyuan
Based on GlobeLand30 land cover data () and land cover transfer matrix (), this study generated the regional distribution map of land degradation and restoration (), mutual conversion between land cover types () and the area changes of water-related land types and forest area () in Taiyuan from 2010 to 2020.
Figure 9. Mutual conversion between land cover types in Taiyuan from 2010 to 2020 (unit km2). (Note: the two types of land cover types connected by a curve indicate that there is mutual conversion between them. For example, the brown curve represents the interconversion between impervious surface and cropland. The narrower end of impervious surface and the wider end of cropland means that a larger area of cropland is converted into impervious surface, that is, urban expansion occurs.)

Table 4. SDG indicators of land types in Taiyuan from 2010 to 2020.
As shown in , from 2010 to 2020, land restoration areas in Taiyuan were mainly concentrated in the southwestern (region A of ) and central (region B of ) regions of Yangqu County, which may be related to afforestation activities. Due to the active restoration of ecology in Taiyuan, the forest area has increased significantly, making the ratio of forest area to land increased from 27.5% to 30.1% (). In addition, the land degradation areas were mainly concentrated in western Luofan County (region C of ), southwestern Gujiao County (region D of ), and southeastern Taiyuan (region E of ). The land degradation in regions C and D may be related to mining, of which region C is the Sigou Village mining area of Jianshan Iron Mine Company of Tisco, region D is the Malan mining area, and region E may be caused by urban expansion. In addition, the area of water-related land types in Taiyuan decreased from 6417.35 km2 in 2010 to 6186.98 km2 in 2020 () because of urban expansion and mining. To some extent, Taiyuan has achieved great performances in ecological restoration, with a significant increase in forest area, making 3.68% of the total area of land restored (). However, at the same time, mining and urban expansion have also resulted in the occupation of some cropland and grasslands, resulting in land degradation of 5.18% of the total area. The area of land degradation is higher than the area of land restoration, which means Taiyuan has not realized land degradation neutrality from 2010 to 2020, and still needs to implement ecological restoration.
As shown in , actions of forest protection, such as afforestation and returning cropland to forest, have significantly restored the land, contributed to the prevention and control of land desertification, and increased the forest area of Taiyuan (). Meanwhile, Taiyuan is also accompanied by urban expansion and mining () with cropland degrading to artificial surfaces, which leads to an increase in urban area and a decrease in cropland area.
3.4. The changes of vegetation growth condition in Xishan and Dongshan of Taiyuan
This study utilized Landsat satellite remote sensing data inversion from 2010 to 2020 to derive the spatial distribution maps of LAI () and FVC () for Xishan and Dongshan. However, it is important to note that the data for the years 2011 and 2012 could not be obtained successfully due to various factors such as cloud cover. Apart from the unknown value in 2011 and 2012, the study indicates a consistent increase in LAI values for both Xishan and Dongshan over the years. This upward trend suggests a gradual improvement in vegetation growth. Furthermore, similar to the trend observed in LAI values, the FVC of both Xishan and Dongshan is also exhibiting a yearly increase. This consistent upward trend in FVC suggests an overall greening trend in the study area.
This study encountered challenges in obtaining vegetation greening area, leading to a limited dataset for calculating the VGCI for Xishan and Dongshan. As a result, only Landsat data from three specific years, namely 2010, 2015, and 2020, were utilized for this purpose. shows the bar chart of various vegetation indices in Xishan and Dongshan in 2010, 2015 and 2020. The MGCI of Xishan was 0.8 in 2010, which means that the green area accounts for 80% of the total area, and decreased to 0.78 in 2015 and 0.76 in 2020, respectively. The proportion of green area gradually decreased, and about 2% of the total land area was converted to non-green land every 5 years. Similarly, the MGCI of Dongshan was 0.96 in 2010, and decreased to 0.9 in 2015 and 0.84 in 2020, respectively. The proportion of green area is also gradually decreasing, and about 6% of the total land area is converted to non-green land every 5 years. The MGCI deceasing of Xishan and Dongshan from 2010 to 2020, indicates that the green area is slowly decreasing and the area occupied by vegetation is gradually shrinking. According to the land cover data (), the vegetation restoration of Xishan and Dongshan from 2010 to 2020 was not significant, and there was no obvious behavior of returning cropland to forest and grassland. In addition, urban expansion occupied some cropland areas (), resulting in partial vegetation degradation and land degradation. As shown in , the impervious surface areas of Xishan and Dongshan increased by 8.7 and 10 km2, respectively, which is why the MGCI of Xishan and Dongshan decreased year by year ().
Figure 12. Change of various vegetation indices in Xishan (a) and Dongshan (b) in 2010, 2015 and 2020.

Figure 13. Land cover (a, b, c, d) and land degradation or restoration(e, f) distribution maps of Xishan and Dongshan in 2010 and 2020.

Table 5. Land cover area of Xishan and Dongshan in 2010 and 2020.
Unlike MGCI, the FVC of Xishan was 0.5 in 2010, and increased to 0.66 in 2015 and 0.72 in 2020, respectively. Similarly, the FVC of Dongshan also increased from 0.46 in 2010 to 0.68 in 2020. The continuous increase of FVC represents a significant improvement in the ability of vegetation to cover the land surface. In addition, the LAI of Xishan and Dongshan also increased. The LAI of Xishan increased from 1.17 in 2010 to 2.05 in 2015 and 2.66 in 2020, indicating a significant increase in the total leaf area. Similarly, Dongshan’s LAI has also increased from 1 in 2010 to 1.84 in 2015 and 2.27 in 2020, showing an obvious growth trend.
In 2010, the VGCI of Xishan was 1.91, indicating that the vegetation leaf area of Xishan was 1.91 times the vegetation coverage area, that is, the leaves in the vegetation area could cover 1.91 layers of the area. The VGCI increased to 2.4 in 2015 and 2.9 in 2020, showing a clear growth trend. The vegetation growth condition in 2020 has generally improved, and about a new layer of leaves has grown compared to 2010. Similar to Xishan, the VGCI of Dongshan increased from 1.93 in 2010 to 2.61 in 2015 and then to 2.9 in 2020, and the growth condition of vegetation has also improved significantly.
4. Discussion
4.1. Advantages of the vegetation growth condition index
MGCI, the indicator of SDGs 15.4.2, is defined as the area ratio of all green plants (forests, shrubs, woodlands, pastures, and farmland) in the mountain. In 2017, MGCI was chosen for inclusion in the SDG 15 indicator system as a significant indicator of the level of environmental protection in mountainous areas by the International Mountain Science Committee (Bian et al. Citation2022), which would provide information of green vegetation cover changes in mountainous areas. The MGCI, however, is only able to represent changes in the green vegetation area and does not take into account vegetation growth condition changes due to natural vegetation growth or vegetation type changes. Therefore, a new VGCI concept (Equation 3) defining the leaf area possessed by a unit area of vegetation is proposed to practically characterize the vegetation growth condition in this study.
Theoretically, VGCI represents the leaf area per unit area of vegetation coverage region (with at least one leaf covering the ground), and its value is always greater than or equal to 1. In Equation (3), represents the total leaf area of the study area, while
represent the area of the ground covered by vegetation in the study area. VGCI as ratio of the two represents the number of leaf layers per unit area of vegetation, which can reflect the growth condition of vegetation to a certain extent (the greater the number of leaf layers, the greater the VGCI, which means that the vegetation grows more luxuriant). Therefore, the VGCI can reflect the growth of vegetation by taking considering the natural growth of vegetation and changes in vegetation types.
Compared with MGCI, the VGCI can more accurately reflect the vegetation growth conditions. For example, the MGCI of Xishan has declined from 0.8 in 2010 to 0.76 in 2020, indicating that its green area is decreasing. In contrast, the obvious increase of VGCI in Xishan and Dongshan from 2010 to 2020 indicates that vegetation growth has a clear improvement trend, which is consistent with the results of existing studies (Qi, Hu, and Zhao Citation2021; Zhao et al. Citation2020). Taking Xishan as an example, the VGCI of Xishan increased from 1.93 in 2010 to 2.9 in 2020, and the increased leaf area accounted for 97% of the vegetation coverage area, indicating that the growth of the vegetation area in Xishan greatly improved. Therefore, the case study in Taiyuan’s ecological restoration assessment indicates that the proposed VGCI can accurately reflect the real growth of vegetation.
In addition, compared with FVC and LAI, VGCI also has advantages in evaluating vegetation growth. LAI reflects the total leaf area of vegetation per unit area, whereas FVC represents the coverage of vegetation on the ground (Jia et al. Citation2016; Wang et al. Citation2020). However, the increase of FVC and LAI cannot strictly represent that the vegetation growth condition is getting better. FVC reflects the ability of vegetation to cover the surface, and an increase in FVC represents an increase in the area of the ground covered by vegetation. It is not only the growth of vegetation that will lead to an increase in FVC, but also an increase in the number of green plants. Similarly, LAI is the total leaf area of vegetation on a unit area of land, and an increase in LAI may be the result of better growth of vegetation or an increase in the number of green plants. In addition, the possible change relationship between FVC, LAI and VGCI and their possible causes are shown in supplementary materials. Therefore, although FVC and LAI are also indicators of vegetation growth condition, the circumstances that cause changes in FVC and LAI are usually complex. It is sometimes difficult to judge the changes of vegetation growth condition only by referring to the changes of FVC and LAI. In contrast, VGCI can better reflect the vegetation growth condition.
4.2. Benefits and prospects of ecological projects in Taiyuan
In this study, the evaluation indicators of ecological restoration from the perspective of SDGs were applied to comprehensively evaluate the ecological restoration process in Taiyuan, focusing on water resource management, air quality improvement, land degradation and restoration, and mining vegetation restoration. Based on the evaluation results, it can be indicated that Taiyuan has significantly restored many aspects of its ecosystem, such as improving water quality in Jinyang Lake, improving air quality, achieving land degradation neutrality, and restoring vegetation in Xishan and Dongshan. In addition, Taiyuan has conducted a series of ecological projects which have played an important role for the ecological restoration.
According to the Taiyuan Environmental Status Bulletin (Lai et al. Citation2023), the water quality of Jinyang Lake after comprehensive treatment has improved yearly, which is consistent with the results assessed in this study. In addition, under the background that the SDDs of other major water bodies in Taiyuan were in various degrees of decline, the SDD of Jinyang Lake has a significant improvement trend, which may be closely related to the Jinyang Lake Comprehensive Management Project. Therefore, as a possible result of the project’s successful implementation, Jinyang Lake’s water quality and ecological function have been greatly improved, which can be a model for the improvement of other water bodies in Taiyuan, as well as a reference for the water environment and water ecology improvements in other regions.
Several studies have shown that the air quality of Taiyuan has improved gradually in recent years (Liang, Gao, and Liu Citation2022; Wang, Wen, et al. Citation2022; Zhang, Zheng, and Wang Citation2022). Especially after 2018, the concentration of air pollutants decreased significantly, and RAGA increased significantly (Yan et al. Citation2019), which is highly consistent with the results of this study. In fact, Taiyuan started rolling out the Green Transformation Project of Promoting Production and Living Energy Use (GTPPLEU) in 2017 to enhance its air quality. During the implementation of the GTPPLEU project, the government adopted central heating, an electricity supply instead of coal burning, and a gas supply instead of coal burning to adjust the energy supply structure of Taiyuan and has increased the use of clean energy and changed the energy supply structure, which reduces the concentration of air pollutants in Taiyuan and solves the impact of coal-fired heating on the atmospheric environment in winter. Therefore, there are reasons to believe that the large decrease in air pollutant concentrations in Taiyuan since 2018 may be related to GTPPLEU.
Similarly, studies show that with the strengthening of Taiyuan’s regional ecological management, the overall ecological quality of Taiyuan has been improved, and land degradation has been alleviated (Yang and Liu Citation2019; Zhao et al. Citation2021). Although urban expansion has taken up a large area of farmland, Taiyuan has also been actively promoting afforestation and returning farmland to forest, increasing the forest area by about 180 km2 from 2010 to 2020. The evaluation results of land degradation and restoration show that there was significant land restoration in the southwestern (region A of ) and central (region B of ) regions of Yangqu County from 2010 to 2020, and the areas where land restoration occurred were the same as the project areas of Yangqu County Afforestation Project (YCAP) and Taiyuan Beishan Volunteer Tree Planting Base (TBVTP). Therefore, the land restoration in Yangqu County is probably related to the afforestation projects such as YCAP and TBVTP.
As for mine’s vegetation restoration, relevant studies (Qi, Hu, and Zhao Citation2021; Zhao et al. Citation2020) have shown that by actively promoting the implementation of comprehensive ecological management of mines (Ecological Comprehensive Management Project of Xishan and Dongshan, ECMPXD) in Taiyuan in recent years, the vegetation of Xishan and Dongshan has a significant trend of improvement. The assessment of VGCI showed that the vegetation growth conditions of the two mountain areas were significantly improved, and the vegetation growth was significantly improved, which is consistent with previous studies (Qi, Hu, and Zhao Citation2021; Zhao et al. Citation2020). In addition, compared with the MGCI, the proposed VGCI can better reflect the actual vegetation growth condition.
Although Taiyuan’s ecological projects have contributed to its ecological restoration, there are still many challenges in its implementation. First, the water quality of the main water bodies in Taiyuan is still deteriorating. The Jinyang Lake governance model should be promoted to manage other major water bodies. Second, O3 has become the main air pollutant in Taiyuan. It is necessary to focus on the rise in O3 concentration, speed up the improvement of oxygen pollution control shortcomings, promote the coordinated emission reduction of nitrogen oxides (NOx) and volatile organic compounds (VOCs) (Council Citation1992; Wang et al. Citation2017), and promote the realization of SDG11.1 goals. Finally, urban expansion and mining are the main causes of land degradation in Taiyuan. Taiyuan should not only focus on economic development but also consider vegetation restoration methods such as afforestation and returning cropland to forests to ensure the planned and targeted sustainable restoration of vegetation and promote the realization of SDG15.3.
4.3. Advantages and limitations of the ecological restoration evaluation indicators
In this study, the evaluation indicators for ecological restoration were constructed based on several SDGs (SDG 6, 11, and 15) related to ecological restoration, which included a wide range of topics, such as water environmental protection, urban air pollution purification, land restoration, and vegetation restoration. Correspondingly, the SDD, air pollutant quantities, the area of land degradation, the area of forest and vegetation parameters were selected as the evaluation indicators of ecological restoration. Compared with previous studies (Lin et al. Citation2020; Park, Ruddick, and Lacroix Citation2010; Zucca et al. Citation2015) that focus on one aspect of ecological restoration, the evaluation indicators include more aspects of ecological restoration from the perspective of SDGs, and can conduct a relatively comprehensive assessment of water, air, land, and vegetation.
In this study, given that the research period encompasses 2010–2020, we were unable to utilize Sentinel-2 data publicly released after 2015. Therefore, this study mainly used data from only Landsat series. However, it is suggested that future studies should focus on experimenting with other satellite datasets such as Copernicus, Radar (Schulte to Bühne and Pettorelli Citation2018) or Lidar (Moudrý et al. Citation2019; Moudrý et al. Citation2021). Furthermore, the relationship between the components of ecological restoration is not yet clear in this study, and there is still a need for a comprehensive index to evaluate the benefits of ecological restoration. Overall, the ecological restoration evaluation indicators proposed in this study applies RS technology to the comprehensive evaluation of the ecological restoration process, and provides a case reference for the use of RS to evaluate regional ecological restoration and regional sustainable development, which has certain theoretical and practical significance.
5. Conclusion
Under the SDGs perspective, the evaluation indictors for ecological restoration were proposed for Taiyuan’s ecological restoration assessment. The following conclusions can be drawn: (1) Taiyuan has made great progress in ecological restoration in recent years, such as the improvement of water quality of Jinyang lake, the control of air pollution, the increase of forest coverage and the restoration of mine’s vegetation; (2) the proposed VGCI is sensitive to vegetation growth conditions and can accurately evaluate the vegetation growth condition; (3) ecological projects might play a positive role in Taiyuan’s ecological restoration. The improvement of water quality in Jinyang lake may be related to the implementation of Jinyang Lake Comprehensive Management Project. In conclusion, this study provides a comprehensive evaluation of Taiyuan’s ecological restoration from a sustainable development perspective. The evaluation results underscore the significant improvement in Taiyuan’s ecological restoration, which can be attributed to the proactive implementation of ecological engineering measures.
Supplemental Material
Download ()Acknowledgments
The Landsat dataset was obtained online (https://earthexplorer.usgs.gov/). The hourly air pollutant data was provided by the China National Environmental Monitoring Centre (http://www.cnemc.cn/). The land cover data was provided by the National Geomatics Center of China (http://www.ngcc.cn/ngcc/). We would like to thank Yuanyuan Chen from Beijing Forestry University, China, for helping to improve the quality of this manuscript. We also thank the editors and anonymous reviewers for their comments and suggestions that improved this paper.
Data availability statement
Data available on request.
Disclosure statement
No potential conflict of interest was reported by the author(s).
Additional information
Funding
References
- Aronson, James, and Sasha Alexander. 2013. “Ecosystem Restoration is Now a Global Priority: Time to Roll up our Sleeves.” Restoration Ecology 21 (3): 293–296. https://doi.org/10.1111/rec.12011.
- Aronson, James, Neva Goodwin, Laura Orlando, Cristina Eisenberg, and Adam T. Cross. 2020. “A World of Possibilities: Six Restoration Strategies to Support the United Nation’s Decade on Ecosystem Restoration.” Restoration Ecology 28 (4): 730–736. https://doi.org/10.1111/rec.13170.
- Baret, Frédéric, Olivier Hagolle, Bernhard Geiger, Patrice Bicheron, Bastien Miras, Mireille Huc, Béatrice Berthelot, et al. 2007. “LAI, fAPAR and fCover CYCLOPES Global Products Derived from VEGETATION: Part 1: Principles of the Algorithm.” Remote Sensing of Environment 110 (3): 275–286. https://doi.org/10.1016/j.rse.2007.02.018.
- Bian, Jinhu, Ainong Li, Xi Nan, Guangbin Lei, and Zhengjian Zhang. 2022. “Dataset of the Mountain Green Cover Index (SDG15. 4.2) Over the Economic Corridors of the Belt and Road Initiative for 2010-2019.” Big Earth Data 6 (1): 77–89. https://doi.org/10.1080/20964471.2021.1941571.
- Cao, Shixiong. 2011. “Impact of China’s Large-Scale Ecological Restoration Program on the Environment and Society in Arid and Semiarid Areas of China: Achievements, Problems, Synthesis, and Applications.” Critical Reviews in Environmental Science and Technology 41 (4): 317–335. https://doi.org/10.1080/10643380902800034.
- CBD (Convention on Biological Diversity). 2012. “UNEP/CBD/COP Decision XI/16.” Ecosystem Restoration.
- Center for International Knowledge on Development. 2021. Progress Report on China’s Implementation of the 2030 Agenda for Sustainable Development. Beijing: Center for International Knowledge on Development.
- Chen, Chi, Taejin Park, Xuhui Wang, Shilong Piao, Baodong Xu, Rajiv K. Chaturvedi, Richard Fuchs, Victor Brovkin, Philippe Ciais, and Rasmus Fensholt. 2019. “China and India Lead in Greening of the World Through Land-Use Management.” Nature Sustainability 2: 122–129. https://doi.org/10.1038/s41893-019-0220-7.
- Chen, Ang, Xiuchun Yang, Jian Guo, Xiaoyu Xing, Dong Yang, and Bin Xu. 2021. “Synthesized Remote Sensing-Based Desertification Index Reveals Ecological Restoration and its Driving Forces in the Northern Sand-Prevention Belt of China.” Ecological Indicators 131: 108230. https://doi.org/10.1016/j.ecolind.2021.108230.
- Chen, Dengshuai, Qiqi Zhao, Penghui Jiang, and Manchun Li. 2022. “Incorporating Ecosystem Services to Assess Progress Towards Sustainable Development Goals: A Case Study of the Yangtze River Economic Belt, China.” Science of the Total Environment 806 (Pt 3): 151277. https://doi.org/10.1016/j.scitotenv.2021.151277.
- Cowie, Annette L., Barron J. Orr, Victor M. Castillo Sanchez, Pamela Chasek, Neville D. Crossman, Alexander Erlewein, Geertrui Louwagie, et al. 2018. “Land in Balance: The Scientific Conceptual Framework for Land Degradation Neutrality.” Environmental Science & Policy 79: 25–35. https://doi.org/10.1016/j.envsci.2017.10.011.
- Dekker, Arnold G., and Erin L. Hestir. 2012. Evaluating the Feasibility of Systematic Inland Water Quality Monitoring with Satellite Remote Sensing. Canberra, Australia: Commonwealth Scientific Industrial Research Organization.
- del Río-Mena, Trinidad, Louise Willemen, Ghirmay Tsegay Tesfamariam, Otto Beukes, and Andy Nelson. 2020. “Remote Sensing for Mapping Ecosystem Services to Support Evaluation of Ecological Restoration Interventions in an Arid Landscape.” Ecological Indicators 113: 106182. https://doi.org/10.1016/j.ecolind.2020.106182.
- de Oliveira, Michele L., Carlos A. C. dos Santos, Gabriel de Oliveira, Madson T. Silva, Bernardo B. da Silva, John E. de B. L. Cunha, Anderson Ruhoff, and Celso A. G. Santos. 2022. “Remote Sensing-Based Assessment of Land Degradation and Drought Impacts Over Terrestrial Ecosystems in Northeastern Brazil.” Science of the Total Environment 835: 155490. https://doi.org/10.1016/j.scitotenv.2022.155490.
- De Pinto, Alessandro, Nicola Cenacchi, Richard Robertson, Ho-Young Kwon, Timothy Thomas, Jawoo Koo, Salome Begeladze, and Chetan Kumar. 2020. “The Role of Crop Production in the Forest Landscape Restoration Approach—Assessing the Potential Benefits of Meeting the Bonn Challenge.” Frontiers in Sustainable Food Systems 4: 61. https://doi.org/10.3389/fsufs.2020.00061.
- Devlin, Michelle, Suzanne Bricker, and Suzanne Painting. 2011. “Comparison of Five Methods for Assessing Impacts of Nutrient Enrichment Using Estuarine Case Studies.” Biogeochemistry 106 (2): 177–205. https://doi.org/10.1007/s10533-011-9588-9.
- Doren, Robert F., Joel C. Trexler, Andrew D. Gottlieb, and Matthew C. Harwell. 2009. “Ecological Indicators for System-Wide Assessment of the Greater Everglades Ecosystem Restoration Program.” Ecological Indicators 9 (6): S2–S16. https://doi.org/10.1016/j.ecolind.2008.08.009.
- Dubey, Pradeep Kumar, Ajeet Singh, Apoorva Raghubanshi, and P. C. Abhilash. 2021. “Steering the Restoration of Degraded Agroecosystems During the United Nations Decade on Ecosystem Restoration.” Journal of Environmental Management 280: 111798. https://doi.org/10.1016/j.jenvman.2020.111798.
- Dubovyk, Olena. 2017. “The Role of Remote Sensing in Land Degradation Assessments: Opportunities and Challenges.” European Journal of Remote Sensing 50 (1): 601–613. https://doi.org/10.1080/22797254.2017.1378926.
- Duntley, Seibert Q., and R. W. Preisendorfer. 1952. “The Visibility of Submerged Objects.” Visibility Lab., Mass. Inst. Tech pp. 74.
- Feng, Xiaoming, Bojie Fu, Nan Lu, Yuan Zeng, and Bingfang Wu. 2013. “How Ecological Restoration Alters Ecosystem Services: An Analysis of Carbon Sequestration in China’s Loess Plateau.” Scientific Reports 3: 2846. https://doi.org/10.1038/srep02846.
- Ferreira, João G., Jesper H. Andersen, Angel Borja, Suzanne B. Bricker, Jordi Camp, Margarida Cardoso da Silva, Esther Garcés, et al. 2011. “Overview of Eutrophication Indicators to Assess Environmental Status Within the European Marine Strategy Framework Directive.” Estuarine, Coastal and Shelf Science 93 (2): 117–131. https://doi.org/10.1016/j.ecss.2011.03.014.
- Foody, Giles M. 1999. “The Significance of Border Training Patterns in Classification by a Feedforward Neural Network Using Back Propagation Learning.” International Journal of Remote Sensing 20 (18): 3549–3562. https://doi.org/10.1080/014311699211192.
- Gaubatz, Piper, and Dean Hanink. 2020. “Learning from Taiyuan: Chinese Cities as Urban Sustainability Laboratories.” Geography and Sustainability 1 (2): 118–126. https://doi.org/10.1016/j.geosus.2020.06.004.
- General Assembly Resolution. 2015. “Transforming Our World: The 2030 Agenda for Sustainable Development.” UN Doc. A/RES/70/1 (September 25, 2015).
- Gordon, Howard R., Otis B. Brown, Robert H. Evans, James W. Brown, Raymond C. Smith, Karen S. Baker, and Dennis K. Clark. 1988. “A Semianalytic Radiance Model of Ocean Color.” Journal of Geophysical Research: Atmospheres 93 (D9): 10909–10924. https://doi.org/10.1029/JD093iD09p10909.
- Gordon, Howard R., and André Y. Morel. 1983. Remote Assessment of Ocean Color for Interpretation of Satellite Visible Imagery: A Review. Berlin, Germany: Springer-Verlag.
- Guo, Biyun, Jushang Wang, Venkata Subrahmanyam Mantravadi, Li Zhang, and Guangzhe Liu. 2022. “Effect of Climate and Ecological Restoration on Vegetation Changes in the “Three-River Headwaters” Region Based on Remote Sensing Technology.” Environmental Science and Pollution Research 29 (11): 16436–16448. https://doi.org/10.1007/s11356-021-16927-3.
- Hasab, Hashim Ali, Hussain A. Jawad, Hayder Dibs, Hussain Musa Hussain, and Nadhir Al-Ansari. 2020. “Evaluation of Water Quality Parameters in Marshes Zone Southern of Iraq Based on Remote Sensing and GIS Techniques.” Water, Air, & Soil Pollution 231 (4). https://doi.org/10.1007/s11270-020-04531-z.
- Jia, Xiaoqing, Bojie Fu, Xiaoming Feng, Guohua Hou, Yu Liu, and Xiaofeng Wang. 2014. “The Tradeoff and Synergy Between Ecosystem Services in the Grain-for-Green Areas in Northern Shaanxi, China.” Ecological Indicators 43: 103–113. https://doi.org/10.1016/j.ecolind.2014.02.028.
- Jia, Kun, Shunlin Liang, Xingfa Gu, F. Baret, Xiangqin Wei, Xiaoxia Wang, Yunjun Yao, Linqing Yang, and Yuwei Li. 2016. “Fractional Vegetation Cover Estimation Algorithm for Chinese GF-1 Wide Field View Data.” Remote Sensing of Environment 177: 184–191. https://doi.org/10.1016/j.rse.2016.02.019.
- Jia, Kun, Shunlin Liang, Xiangqin Wei, Qiangzi Li, Xin Du, Bo Jiang, Yunjun Yao, Xiang Zhao, and Yuwei Li. 2015. “Fractional Forest Cover Changes in Northeast China from 1982 to 2011 and its Relationship with Climatic Variations.” IEEE Journal of Selected Topics in Applied Earth Observations and Remote Sensing 2.
- Kapalanga, Taimi S., Zvikomborero Hoko, Webster Gumindoga, and Loyd Chikwiramakomo. 2021. “Remote-sensing-based Algorithms for Water Quality Monitoring in Olushandja Dam, North-Central Namibia.” Water Supply 21 (5): 1878–1894. https://doi.org/10.2166/ws.2020.290.
- Lai, Kefu, Huahu Xu, Jun Sheng, and Yuzhe Huang. 2023. “Hour-by-Hour Prediction Model of Air Pollutant Concentration Based on EIDW-Informer—A Case Study of Taiyuan.” Atmosphere 14 (8): 1274. https://doi.org/10.3390/atmos14081274.
- Lee, ZhongPing, Kendall L. Carder, and Robert A. Arnone. 2002. “Deriving Inherent Optical Properties from Water Color: A Multiband Quasi-Analytical Algorithm for Optically Deep Waters.” Applied Optics 41 (27): 5755–5772. https://doi.org/10.1364/AO.41.005755.
- Lee, ZhongPing, Shaoling Shang, Chuanmin Hu, Keping Du, Alan Weidemann, Weilin Hou, Junfang Lin, and Gong Lin. 2015. “Secchi Disk Depth: A New Theory and Mechanistic Model for Underwater Visibility.” Remote Sensing of Environment 169: 139–149. https://doi.org/10.1016/j.rse.2015.08.002.
- Lee, Zhongping, Shaoling Shang, Lin Qi, Jing Yan, and Gong Lin. 2016. “A Semi-Analytical Scheme to Estimate Secchi-Disk Depth from Landsat-8 Measurements.” Remote Sensing of Environment 177: 101–106. https://doi.org/10.1016/j.rse.2016.02.033.
- Li, Miaomiao, Antian Liu, Chunjing Zou, Wenduo Xu, Hideyuki Shimizu, and Kaiyun Wang. 2012. “An Overview of the “Three-North” Shelterbelt Project in China.” Forestry Studies in China 14 (1): 70–79. https://doi.org/10.1007/s11632-012-0108-3.
- Li, Hengkai, Feng Xu, and Qin Li. 2020. “Remote Sensing Monitoring of Land Damage and Restoration in Rare Earth Mining Areas in 6 Counties in Southern Jiangxi Based on Multisource Sequential Images.” Journal of Environmental Management 267: 110653. https://doi.org/10.1016/j.jenvman.2020.110653.
- Liang, Wenting, Xuedong Gao, and Hao Liu. 2022. “Air Quality Prediction Model Based on Grey Interval.” In 2022 IEEE 25th International Conference on Computer Supported Cooperative Work in Design (CSCWD), Hangzhou, China, 2022, 553–55.
- Lin, Xingna, Jianzhi Niu, Ronny Berndtsson, Xinxiao Yu, Linus Zhang, and Xiongwen Chen. 2020. “NDVI Dynamics and Its Response to Climate Change and Reforestation in Northern China.” Remote Sensing 12 (24): 4138. https://doi.org/10.3390/rs12244138.
- Liu, Qingfu, Qing Zhang, Yongzhi Yan, Xuefeng Zhang, Jianming Niu, and Jens-Christian Svenning. 2020. “Ecological Restoration is the Dominant Driver of the Recent Reversal of Desertification in the Mu Us Desert (China).” Journal of Cleaner Production 268: 122241. https://doi.org/10.1016/j.jclepro.2020.122241.
- Lu, Yonglong, Yueqing Zhang, Xianghui Cao, Chenchen Wang, Yichao Wang, Meng Zhang, C. Ferrier Robert, et al. 2019. “Forty Years of Reform and Opening Up: China’s Progress Toward a Sustainable Path.” Science Advances 5 (8): eaau9413. https://doi.org/10.1126/sciadv.aau9413.
- Ma, Rui, Yanxin Wang, Ziyong Sun, Chunmiao Zheng, Teng Ma, and Henning Prommer. 2011. “Geochemical Evolution of Groundwater in Carbonate Aquifers in Taiyuan, Northern China.” Applied Geochemistry 26 (5): 884–897. https://doi.org/10.1016/j.apgeochem.2011.02.008.
- Ministry of Environmental Protection of China. 2012a. Ambient Air Quality Standards (Document GB 3095–2012). Beijing: Ministry of Environmental Protection of the People’s Republic of China.
- Ministry of Environmental Protection of China. 2012b. Technical Regulation on Ambient Air Quality Index (on Trial) (Document HJ 633–2012). Beijing: Ministry of Environmental Protection of the People’s Republic of China.
- Moudrý, Vítězslav, Kateřina Gdulová, Michal Fogl, Petr Klápště, Rudolf Urban, Jan Komárek, Lucie Moudrá, Martin Štroner, Vojtěch Barták, and Milič Solský. 2019. “Comparison of Leaf-off and Leaf-on Combined UAV Imagery and Airborne LiDAR for Assessment of a Post-Mining Site Terrain and Vegetation Structure: Prospects for Monitoring Hazards and Restoration Success.” Applied Geography 104: 32–41. https://doi.org/10.1016/j.apgeog.2019.02.002.
- Moudrý, Vítězslav, Lucie Moudrá, Vojtěch Barták, Vladimír Bejček, Kateřina Gdulová, Markéta Hendrychová, David Moravec, Petr Musil, Duccio Rocchini, and Karel Šťastný. 2021. “The Role of the Vegetation Structure, Primary Productivity and Senescence Derived from Airborne LiDAR and Hyperspectral Data for Birds Diversity and Rarity on a Restored Site.” Landscape and Urban Planning 210: 104064. https://doi.org/10.1016/j.landurbplan.2021.104064.
- Murcia, Carolina, Manuel R. Guariguata, Ángela Andrade, Germán Ignacio Andrade, James Aronson, Elsa Matilde Escobar, Andrés Etter, Flavio H. Moreno, Wilson Ramírez, and Elena Montes. 2016. “Challenges and Prospects for Scaling-up Ecological Restoration to Meet International Commitments: Colombia as a Case Study.” Conservation Letters 9 (3): 213–220. https://doi.org/10.1111/conl.12199.
- National Research Council. 1992. Rethinking the Ozone Problem in Urban and Regional Air Pollution. National Academies Press.
- Ngia, L. S. H., and J. Sjoberg. 2000. “Efficient Training of Neural Nets for Nonlinear Adaptive Filtering Using a Recursive Levenberg-Marquardt Algorithm.” IEEE Transactions on Signal Processing 48 (7): 1915–1927. https://doi.org/10.1109/78.847778.
- Ning, Xiaogang, Ning Zhu, Yafei Liu, and Hao Wang. 2022. “Quantifying Impacts of Climate and Human Activities on the Grassland in the Three-River Headwater Region After Two Phases of Ecological Project.” Geography and Sustainability 3 (2): 164–176. https://doi.org/10.1016/j.geosus.2022.05.003.
- Park, Young-Je, Kevin Ruddick, and Geneviève Lacroix. 2010. “Detection of Algal Blooms in European Waters Based on Satellite Chlorophyll Data from MERIS and MODIS.” International Journal of Remote Sensing 31 (24): 6567–6583. https://doi.org/10.1080/01431161003801369.
- Petursdottir, Thorunn, Olafur Arnalds, Susan Baker, Luca Montanarella, and Ása L. Aradóttir. 2013. “A Social-Ecological System Approach to Analyze Stakeholders’ Interactions within a Large-Scale Rangeland Restoration Program.” Ecology and Society 18 (2), https://doi.org/10.5751/es-05399-180229.
- Qi, Danning, Zhengjun Hu, and Shangmin Zhao. 2021. “Long-term NDVI Spatio-Temporal Variation Trend and its Response to Climate in Xishan Coalfield, Shanxi Province.” Bulletin of Surveying and Mapping 0 (9): 98–102,107. https://doi.org/10.13474/j.cnki.11-2246.2021.0282.
- Schulte to Bühne, Henrike, and Nathalie Pettorelli. 2018. “Better Together: Integrating and Fusing Multispectral and Radar Satellite Imagery to Inform Biodiversity Monitoring, Ecological Research and Conservation Science.” Methods in Ecology and Evolution 9 (4): 849–865. https://doi.org/10.1111/2041-210X.12942.
- Sims, N. C., G. J. Newnham, J. R. England, J. Guerschman, S. J. D. Cox, S. H. Roxburgh, R. A. Viscarra Rossel, S. Fritz, and I. Wheeler. 2021. Good Practice Guidance. SDG Indicator 15.3.1, Proportion of Land That is Degraded Over Total Land Area. Version 2.0. Bonn, Germany: United Nations Convention to Combat Desertification.
- Steenmans, Katrien. 2017. “United Nations Convention to Combat Desertification 1994.” In Elgar Encyclopedia of Environmental Law, edited by Michael Faure, 49–59. Cheltenham, UK: Edward Elgar Publishing.
- Taiyuan, Statistics Bureau. 2021. “Taiyuan Statistics Bureau (Taiyuan SB), 2021.” Taiyuan National Economy and Social Development Bulletin 2020.
- Waltham, Nathan J., Michael Elliott, Shing Yip Lee, Catherine Lovelock, Carlos M. Duarte, Christina Buelow, Charles Simenstad, Ivan Nagelkerken, Louw Claassens, and Colin K. C. Wen. 2020. “UN Decade on Ecosystem Restoration 2021–2030—What Chance for Success in Restoring Coastal Ecosystems?” Frontiers in Marine Science 7: 71. https://doi.org/10.3389/fmars.2020.00071.
- Wang, Jin, Ping Han, Yanhua Zhang, Jinyu Li, Linxu Xu, Xue Shen, Zhigang Yang, Sisi Xu, Guangxue Li, and Feiyong Chen. 2022. “Analysis on Ecological Status and Spatial-Temporal Variation of Tamarix Chinensis Forest Based on Spectral Characteristics and Remote Sensing Vegetation Indices.” Environmental Science and Pollution Research 29 (25): 37315–37326. https://doi.org/10.1007/s11356-022-18678-1.
- Wang, Bing, Kun Jia, Xiangqin Wei, Mu Xia, Yunjun Yao, Xiaotong Zhang, Duanyang Liu, and Guofeng Tao. 2020. “Generating Spatiotemporally Consistent Fractional Vegetation Cover at Different Scales Using Spatiotemporal Fusion and Multiresolution Tree Methods.” ISPRS Journal of Photogrammetry and Remote Sensing 167: 214–229. https://doi.org/10.1016/j.isprsjprs.2020.07.006.
- Wang, Xin, Jinling Song, Zhiqiang Xiao, Jing Wang, and Fangze Hu. 2022. “Desertification in the Mu Us Sandy Land in China: Response to Climate Change and Human Activity from 2000 to 2020.” Geography and Sustainability 3 (2): 177–189. https://doi.org/10.1016/j.geosus.2022.06.001.
- Wang, Yang, Yanping Wen, Yang Cui, Lili Guo, Qiusheng He, Hongyan Li, and Xinming Wang. 2022. “Substantial Changes of Chemical Composition and Sources of Fine Particles During the Period of COVID-19 Pandemic in Taiyuan, Northern China.” Air Quality, Atmosphere & Health 15 (1): 47–58. https://doi.org/10.1007/s11869-021-01082-y.
- Wang, Tao, Likun Xue, Peter Brimblecombe, Yun Fat Lam, Li Li, and Li Zhang. 2017. “Ozone Pollution in China: A Review of Concentrations, Meteorological Influences, Chemical Precursors, and Effects.” Science of the Total Environment 575: 1582–1596. https://doi.org/10.1016/j.scitotenv.2016.10.081.
- Wang, Yichao, Jingjing Yuan, and Yonglong Lu. 2020. “Constructing Demonstration Zones to Promote the Implementation of Sustainable Development Goals.” Geography and Sustainability 1 (1): 18–24. https://doi.org/10.1016/j.geosus.2020.02.004.
- Wei, Zihan, Quyet Van Le, Wanxi Peng, Yafeng Yang, Han Yang, Haiping Gu, Su Shiung Lam, and Christian Sonne. 2021. “A Review on Phytoremediation of Contaminants in air, Water and Soil.” Journal of Hazardous Materials 403: 123658. https://doi.org/10.1016/j.jhazmat.2020.123658.
- Wood, Sylvia L. R., Sarah K. Jones, Justin A. Johnson, Kate A. Brauman, Rebecca Chaplin-Kramer, Alexander Fremier, Evan Girvetz, et al. 2018. “Distilling the Role of Ecosystem Services in the Sustainable Development Goals.” Ecosystem Services 29: 70–82. https://doi.org/10.1016/j.ecoser.2017.10.010.
- Wu, Zhitao, Jianjun Wu, Jinghui Liu, Bin He, Tianjie Lei, and Qianfeng Wang. 2013. “Increasing Terrestrial Vegetation Activity of Ecological Restoration Program in the Beijing–Tianjin Sand Source Region of China.” Ecological Engineering 52: 37–50. https://doi.org/10.1016/j.ecoleng.2012.12.040.
- Xia, Mu, Kun Jia, Wenwu Zhao, Shiliang Liu, Xiangqin Wei, and Bing Wang. 2021. “Spatio-temporal Changes of Ecological Vulnerability Across the Qinghai-Tibetan Plateau.” Ecological Indicators 123: 107274. https://doi.org/10.1016/j.ecolind.2020.107274.
- Xu, Zhigang, Michael T Bennett, Ran Tao, and Jintao Xu. 2004. “China’s Sloping Land Conversion Program Four Years on: Current Situation and Pending Issues.” International Forestry Review 6 (3-4): 317–326.
- Yan, Shi-Ming, Yan Wang, Wei Guo, Ying Li, and Feng-Sheng Zhang. 2019. “[Characteristics, Transportation, Pathways, and Potential Sources of Air Pollution During Autumn and Winter in Taiyuan].” Huan jing ke xue= Huanjing kexue 40 (11): 4801–4809. https://doi.org/10.13227/j.hjkx.201904140.
- Yang, Na, and Renhui Liu. 2019. “Study on Land Use Status and Land Ecosystem Service Value in Taiyuan City.” In ICCREM 2019: Innovative Construction Project Management and Construction Industrialization, edited by Yaowu Wang, Mohamed Al-Hussein, and Geoffrey Q. P. Shen, 931–944. Banff, Canada: American Society of Civil Engineers Reston, VA.
- Yang, Siqi, Wenwu Zhao, Yanxu Liu, Francesco Cherubini, Bojie Fu, and Paulo Pereira. 2020. “Prioritizing Sustainable Development Goals and Linking Them to Ecosystem Services: A Global Expert’s Knowledge Evaluation.” Geography and Sustainability 1 (4): 321–330. https://doi.org/10.1016/j.geosus.2020.09.004.
- Yao, Ying, Bojie Fu, Yanxu Liu, Yijia Wang, and Shuang Song. 2021. “The Contribution of Ecosystem Restoration to Sustainable Development Goals in Asian Drylands: A Literature Review.” Land Degradation & Development 32 (16): 4472–4483. https://doi.org/10.1002/ldr.4065.
- Yin, Caichun, Wenwu Zhao, Francesco Cherubini, and Paulo Pereira. 2021. “Integrate Ecosystem Services Into Socio-Economic Development to Enhance Achievement of Sustainable Development Goals in the Post-Pandemic era.” Geography and Sustainability 2 (1): 68–73. https://doi.org/10.1016/j.geosus.2021.03.002.
- Zhai, Xiaohui, Xiaolei Liang, Changzhen Yan, Xuegang Xing, Haowei Jia, Xiaoxu Wei, and Kun Feng. 2020. “Vegetation Dynamic Changes and Their Response to Ecological Engineering in the Sanjiangyuan Region of China.” Remote Sensing 12: 4035. https://doi.org/10.3390/rs12244035.
- Zhang, Daisheng, Kristin Aunan, Hans Martin Seip, and Haakon Vennemo. 2011. “The Energy Intensity Target in China’s 11th Five-Year Plan Period—Local Implementation and Achievements in Shanxi Province.” Energy Policy 39 (7): 4115–4124. https://doi.org/10.1016/j.enpol.2011.03.085.
- Zhang, Mei, Zhiping Li, Min Xu, Jianwei Yue, Zongwei Cai, Ken Kin Lam Yung, and Ruijin Li. 2020. “Pollution Characteristics, Source Apportionment and Health Risks Assessment of Fine Particulate Matter During a Typical Winter and Summer Time Period in Urban Taiyuan, China.” Human and Ecological Risk Assessment: An International Journal 26 (10): 2737–2750. https://doi.org/10.1080/10807039.2019.1684184.
- Zhang, Xi, Jiayu Zheng, and Ligang Wang. 2022. “Can the Relationship between Atmospheric Environmental Quality and Urban Industrial Structure Adjustment Achieve Green and Sustainable Development in China? A Case of Taiyuan City.” Energies 15 (9): 3402. https://doi.org/10.3390/en15093402.
- Zhao, Fuqiang, Yue Ma, Fengming Xi, Lun Yang, and Jing Sun. 2020. “Evaluating the Sustainability of Mine Rehabilitation Programs in China.” Restoration Ecology 28 (5): 1061–1066. https://doi.org/10.1111/rec.13183.
- Zhao, Bingtao, Yaxin Su, Shushen He, Mei Zhong, and Guomin Cui. 2016. “Evolution and Comparative Assessment of Ambient Air Quality Standards in China.” Journal of Integrative Environmental Sciences, 1–18. https://doi.org/10.1080/1943815X.2016.1150301.
- Zhao, Weijun, Tingting Yan, Xue Ding, Shuzhen Peng, Henan Chen, Yanchao Fu, and Ze Zhou. 2021. “Response of Ecological Quality to the Evolution of Land Use Structure in Taiyuan During 2003 to 2018.” Alexandria Engineering Journal 60 (1): 1777–1785. https://doi.org/10.1016/j.aej.2020.11.026.
- Zucca, Claudio, Weicheng Wu, Leonarda Dessena, and Maurizio Mulas. 2015. “Assessing the Effectiveness of Land Restoration Interventions in Dry Lands by Multitemporal Remote Sensing – A Case Study in Ouled DLIM (Marrakech, Morocco).” Land Degradation & Development 26 (1): 80–91. https://doi.org/10.1002/ldr.2307.