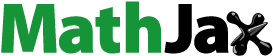
ABSTRACT
The provision of urban green spaces has been widely acknowledged to offer numerous benefits to individuals and communities. Recently, there has been a growing recognition of the importance of evaluating urban greenness from a human-centered perspective, partly due to the rapid development of eye-level greenness measurement. To provide a comprehensive overview of measurement approaches and topics related to eye-level urban greenness, we conducted a systematic review of 77 relevant papers. Our review revealed that street view images are the primarily utilized data source to quantify eye-level urban greenness in urban analysis. Additionally, simulated eye-level urban greenness represents a new frontier for the general public and urban planners, offering the potential to acquire universally applicable quantitative greenness data. Our findings further suggest that eye-level urban greenness has been explored across various domains, including physical behavior, public health, urban planning, human perception, and inequity. Such exploration has highlighted a significant association between eye-level urban greenness and individuals and communities. Our review generates new insights on the integration of urban greenness into urban planning and design, encouraging a greater focus on eye-level urban greenness as a critical component of urban environmental quality.
1. Introduction
Urban greenness typically refers to urban areas occupied by flora, including both natural and designed green areas (McMorris et al. Citation2015), ranging from small neighborhood parks and community gardens to large public parks and nature reserves. Green roofs are also implemented in some cities (Li and Yeung Citation2014; Shafique, Kim, and Rafiq Citation2018), which are essentially gardens or small parks built on the roofs of buildings for higher greenness provision and improvement of the attractiveness of the city. Coupled with rapid urbanization, urban greenness has been thoroughly involved in urban planning, design, and maintenance.
Urban greenness has gained significant attention in recent years due to the growing recognition of the benefits that greenness can provide to urban communities. There is a range of positive impacts on the urban environment, including improved air quality (Franchini and Mannucci Citation2018), reduced urban heat island effect (Watkins, Palmer, and Kolokotroni Citation2007), reduced noise pollution (Dzhambov and Dimitrova Citation2014), and increased biodiversity (Goddard et al. Citation2010). Besides, studies have shown that visible urban greenness can have significant positive impacts on both physical and mental health (Lu Citation2019; McMorris et al. Citation2015; Thomsen, Powell, and Monz Citation2018). For example, urban greenness provides opportunities for physical activity, social interaction, and recreation, (Chen et al. Citation2021; Twohig-Bennett and Jones Citation2018). Specifically, exposure to urban greenness has been linked to reduced stress levels, improved cognitive function, and lower rates of obesity, diabetes, and other chronic diseases (Fernández Núñez et al. Citation2022; Yang et al. Citation2021; Citation2019). In addition to the benefits to the urban environment and individuals who live in the urban zones, urban greenness can also have economic benefits for cities. For example, urban greenness can help to increase property values (Zheng et al. Citation2012), and attract businesses (Xie and Milcheva Citation2022) and tourists (Acuti et al. Citation2019). Hence, urban greenness constitutes a vital element of healthy, sustainable, and appealing cities. Initiatives to enhance the quantity and quality of urban greenness can potentially yield extensive benefits for both individuals and the environment.
Several methodologies are promoted to capture urban greenness, which can be grouped into two perspectives, i.e. the top-down perspective and the human perspective. The Normalized Difference Vegetation Index (NDVI) derived from remote sensing imagery is a popular way to capture urban greenness by providing comprehensive greenness distribution across large geographic areas and allowing for detailed analysis of vegetation patterns and trends over time (Abutaleb et al. Citation2021; Qian et al. Citation2015; Sadeh et al. Citation2021). Besides, there are other top-down approaches, such as proximity measures, counting the number of green spaces within a given walking distance, and the area of total greenery or canopy coverage (Labib, Lindley, and Huck Citation2020). However, the top-down measurement of urban greenness from a bird’s point of view falls short of reflecting the human perceived greenness in their daily routines (Chen et al. Citation2019). Thus, a new frontier of ‘eye-level urban greenness’ is fostered, pointing to the presence and visibility of vegetation at the eye level of pedestrians in urban areas (Labib, Huck, and Lindley Citation2021; Li et al. Citation2015). Eye-level greenness captures the visual exposure to greenness from a human perspective, which takes precedence over remote sensing imagery to explore the interaction of individuals or communities with urban greenness. Several studies found that, in certain scenarios, the urban greenness represented by NDVI has no statistically significant association with the greenness from human perception, while eye-level urban greenness shows significant associations with them (Helbich et al. Citation2019; Ordóñez et al. Citation2023). To present the eye-level view, the street view image was regarded as a prevalent supporter (Chen et al. Citation2023; Du et al. Citation2023). Besides, the model simulation provided another perspective to capture the eye-level urban greenness by mimicking the human view in an urban 3D environment (Wang et al. Citation2019; Yu et al. Citation2016).
As humans enter the new epoch of the Anthropocene (Malhi Citation2017), there has been a surge in attention to human-centered geography, which seeks to provide a comprehensive and integrated understanding of human behavior and urban science (Bennett et al. Citation2017). Several reviews were published in recent years to assess the association between urban greenness and urban systems or humans. For example, Bowler et al. (Citation2010) reviewed 47 studies that investigated cooling affection caused by urban greening interventions; Yang et al. (Citation2021) reviewed 40 systematic reviews to examine the beneficial associations of greenspace and human health outcomes, such as mortality and cardiometabolic factors. Shin et al. (Citation2020) identified 12 studies that explored the positive association between exposure to greenspace and sleep outcomes. In addition, some reviews also investigated the positive effects of urban greenness on mental health (Chen et al. Citation2021; Lakhani et al. Citation2019; Thomsen, Powell, and Monz Citation2018), outdoor activities (Lambert et al. Citation2019), and specific diseases (de Keijzer, Bauwelinck, and Dadvand Citation2020; Fernández Núñez et al. Citation2022; Yuan et al. Citation2021). It is imperative to note that prior reviews in this domain tended to focus on particular facets of the topic, as opposed to pursuing a holistic and exhaustive review. Besides, these reviews often failed to distinguish the disparities between eye-level and bird's-eye views of greenness. As attention to eye-level urban greenness continues to grow, it becomes increasingly essential to conduct a systematic review to understand the current progress and achievements in this domain. However, such a review remains under-examined in existing scholarship.
In this study, we conduct a comprehensive review of the measurement and benefits of eye-level urban greenness, aiming to better promote urban sustainability and understand human behavior and cognition. In summary, our research questions are threefold:
What are the methods and commonly used datasets employed for measuring eye-level urban greenness?
What are the applications of eye-level urban greenness in various domains?
What makes eye-level greenness unique, and what challenges arise from this uniqueness?
To address the above research questions, we conducted a comprehensive review of the literature on eye-level urban greenness, drawing on 77 highly relevant articles selected from 441 studies returned from the database in the preliminary round search. Our systematic selection criteria focus primarily on articles that explore the measurement of eye-level urban greenness and its potential to inform associations with various domains related to urban analysis. Our review identifies three major methods for calculating eye-level urban greenness, each of which is closely linked to the data source used, including street view images, field-taken photographs, and simulation. We further illustrate five key application domains for eye-level greenness, including physical behavior, public health, urban planning, human perception, and inequity. Finally, we discuss the unique characteristics of eye-level greenness along with its measurement limitations and initiate the debate regarding a human-centric agenda for urban greenness. The conceptual structure of this review is presented in .
2. Method
2.1. Article selection overview
In this study, we adhered to the established systematic review method (Biljecki and Ito Citation2021; Bowler et al. Citation2010) and utilized the Preferred Reporting Items for Systematic Reviews and Meta-Analyses (PRISMA) guidelines (Moher et al. Citation2009) to identify pertinent studies. Initially, we employed a set of relevant keywords to retrieve a preliminary list of papers. Following this, we screened these papers to exclude any non-relevant studies from the review. Subsequently, we focused on the identified and relevant papers to extract pertinent information. As the topic of eye-level urban greenness is relatively recent, we did not impose any specific timeframe restrictions in our literature search to ensure the diversity and comprehensiveness of the selected papers concerning eye-level urban greenness research. The PRISMA diagram for this review is depicted in . Further details of our method are elaborated upon in subsequent sections.
Figure 2. PRISMA flow diagram for selecting eligible studies, and means the number of papers The method is adapted from Moher et al. (Citation2009).

2.2. Search keywords
Following the query design and database selection of a systematic review conducted by Wang and Biljecki (Citation2022), we conducted a keyword search related to visible greenness within the topic section of the Web of Science database to identify the initial set of relevant papers. Our selection of keywords was guided by two primary considerations: the first focuses on the visual perspective, which included terms such as visibility, viewshed, and street view, among others; and the second emphasizes the presence of greenness, including greenspace, greenery, and vegetation. To refine our search and concentrate on eye-level greenness, we combined these two aspects into a single phrase. The final equation of keywords employed for this search is presented below:
TS= (“visible green*” OR “visual green*” OR “green* visibility” OR “viewshed green*” OR “eye level green*” OR “eye view green*” OR “visible vegetation” OR “viewshed vegetation” OR “vegetation visibility” OR “street level green*” OR “street view green*”)
2.3. Eligibility criteria
We carefully reviewed the abstracts of the papers in the initial list to identify relevant studies based on the following criteria: (1) the research was conducted within an urban context; (2) the paper is written in the English language; (3) the acquisition of greenness is only considered from the human perspective (e.g. studies that purely rely on satellite imageries for greenness quantification were excluded). Of the 441 publications identified, 77 were retained for further review. In our examination of the 77 papers, we observed an absence of review articles and qualitative research studies.
During the review process, we extracted information concerning the study region, data source, and topic of investigation from each paper to perform additional statistical analysis of the results. While we acknowledge the possibility of overlooking relevant articles, the large number and variety of papers could minimize significant bias (Biljecki and Ito Citation2021). Furthermore, we believe that our keywords are generally comprehensive. Not only have we reviewed the abstracts, but we have also examined the full text of most articles. As a result, we are confident that our findings are not significantly biased.
2.4. Research topics identification
In our analysis of eligible papers, we noted that apart from ten papers focused solely on the methodology to capture eye-level urban greenness, which is discussed separately in Section 3, the majority centered on investigating the applications of urban greenness in human or urban development. Consequently, we categorized the 77 papers based on their applied topics, following the delineation method employed by Biljecki and Ito (Citation2021). From our eligible papers, we identified five main interdisciplinary topic groups, each containing several sub-topics summarized in . Since one study may involve multiple interdisciplinary topics, we employed a flexible categorization approach. If an article is relevant to multiple topics, we assigned multiple tags to reflect its relevance to each respective topic.
Table 1. Thematic clusters and subtopics categories.
3. Results
3.1. Article statistics
The statistical information presented in encompasses the publication year, study area (limited to the country level), data sources utilized for calculating eye-level urban greenness, and the distribution of related topics. (a) demonstrates that eye-level urban greenness research first emerged in 2015 (Li et al. Citation2015) and has since gained increasing attention in recent years. (b) offers an overview of the various data sources employed in the reviewed papers, in which 79% (63) of studies used street view images from Google (23%), Baidu (29%), Tencent (26%), and Bing (1%) Maps. A considerable percentage of reviewed studies (13%) were conducted based on field experiment photographs. These field experiments adopt a similar approach for exploring eye-level urban greenness within a more specific context. Additionally, multi-source spatial data derived from satellite imagery constitutes a significant source of information used in 8% (6) of reviewed papers.
Figure 3. Statistics of the eligible papers. (a) The number of papers published per year; (b) data sources used to measure eye-level urban greenness and their associated percentages; (c) study region involved and the number of studies in these countries; (d) domains defined based on the outcome of this study and their associated percentages.

As demonstrated in (c), research on eye-level urban greenness has been conducted in eleven countries. However, there is a notable heterogeneity in the geographical distribution of these studies, with a majority focused on major cities in China and a few in the United States, while research in other countries remains scarce. Of the 77 studies reviewed, 58 were conducted in China, primarily in the eastern region, where economic hubs and universities are densely concentrated, rather than in the underrepresented western regions. This reveals significant research gaps in Africa, South America, and large portions of Europe and Asia. A possible explanation for this disparity could be the limited availability of street view imagery provided by major companies such as Google, Baidu, and Tencent Maps. Baidu and Tencent Maps predominantly serve as data sources for studies in China, whereas Google is primarily utilized for research in North America, Europe, Japan, and Hong Kong, China.
The thematic cluster in (d) sheds light on the current research interests within the field. Notably, the relationship between eye-level urban greenness and physical behavior (physical activity 20% and exposure 4%), human health (mental health 8% plus general health 16%), and urban planning (urban environment 8% and urban design 13%) has garnered significant attention. Some studies have simultaneously explored multiple research topics. For example, Han (Citation2017) discussed the effects of eye-level urban greenness on both physical activities and citizens’ emotions, while Xuan, Zhao, and Asce (Citation2022) examined the impacts from the perspectives of physical activities and urban planning. O’Regan, Hunter, and Nyhan (Citation2021) and Jimenez et al. (Citation2022) investigated the impacts of eye-level urban greenness on health and socioeconomic characteristics. Additionally, our literature review identified studies focusing on both mental health and physical activities (Liu et al. Citation2020), mental health and general health (Xiao et al. Citation2021; Zhang et al. Citation2021), as well as exposure and general health (Labib, Lindley, and Huck Citation2021a).
3.2. Measuring eye-level urban greenness: data sources and approaches
As discussed in the previous section, our review identifies three primary methods for assessing eye-level urban greenness, related to the data sources used in each study. Specifically, eye-level urban greenness derived from street view images typically employs techniques such as spectral and geometrical analysis or computer vision technologies for greenness extraction. The second method, utilizing field-taken photographs, involves field experiments that often include collecting photos from profile views and frequently incorporate surveys or questionnaires. These two methods both rely on photos as their primary data source. Model simulation, based on multi-source spatial data such as Light Detection and Ranging (LiDAR) point cloud, aerial photographs, and land use and land cover, represents another common approach for capturing eye-level urban greenness. It is important to note that each method has its advantages and drawbacks as summarized in , which necessitates careful evaluation.
Table 2. A brief summary of the identified three primary measurements of eye-level urban greenness.
In early studies, various approaches have been employed to measure eye-level urban greenness using street view images. Li et al. (Citation2015) utilized a straightforward unsupervised RGB classification method based on spectral features to extract green vegetation pixels from 360-degree panoramic street view images obtained from Google Street View. They further generated the street view green view index to quantify eye-level urban greenness. Kumakoshi et al. (Citation2020) utilized the same method as Li et al. (Citation2015) to calculate the eye-level urban greenness in Yokohama City, Japan. Other studies have also manually extracted green pixels based on RGB classification to represent urban greenness from street view images using software such as Photoshop (Jiao et al. Citation2021). With the advancements in computer vision technology, more sophisticated deep learning methods have been employed for greenness extraction. Deep learning models were trained to recognize vegetation based on shape and color in images. Subsequently, these models were utilized in their research to quantify and determine the proportion of vegetation, serving as an urban greenness index. For instance, Chen et al. (Citation2019) and Xia, Yabuki, and Fukuda (Citation2021) utilized the Pyramid Scene Parsing Network (PSPNet) (Zhao et al. Citation2017) for semantic segmentation of panoramic street view images, while other studies employed methods such as Fully Convolutional Network (Helbich et al. Citation2019; Jia and Zhang Citation2021; Jing et al. Citation2021; Liu et al. Citation2020; Ta et al. Citation2021; Wang et al. Citation2020; Citation2021; Wu et al. Citation2021; Yao et al. Citation2022), DeeplabV3 (Gou, Zhang, and Wang Citation2022; Jiang et al. Citation2022; Xiao et al. Citation2021; Zhang et al. Citation2021), and SegNet (Tang and Long Citation2019; Zhang and Dong Citation2018).
In field experiments, field-taken photographs are often combined with self-reported surveys to assess urban greenness. For example, Falfán et al. (Citation2018) collected a total of 120 360-degree photographs of two cities in Mexico to calculate the green view index. They found that human perception of urban greenness sometimes overestimated the actual situation, possibly due to limitations in the collection of horizontal 360-degree instead of global 360-degree photographs, which may result in incomplete capture of greenness. Field-taken photographs also have limitations in terms of scope, as they are time-consuming and cover only small areas. Zhang et al. (Citation2021a) conducted a field experiment on a university campus, collecting photographs and designing a questionnaire for staff and students to assess the impacts of greenness on emotions. Han (Citation2020; Citation2017) also conducted similar studies focusing on universities. Sun, Lu, and Sun (Citation2021) conducted a study utilizing street view imagery from Zhongshan Road in Qingdao, China, in order to address issues of inequality. In a separate investigation, Chen et al. (Citation2023) analyzed the greenness in manually captured photographs of a Chinese riverbed to explore the concept of visual harmony. Yang et al. (Citation2021a) employed images taken along primary pathways within a public park in Hong Kong, China, to assess park usage patterns. Similarly, Pearson et al. (Citation2020) implemented a comparable research design in a park in the United States, with the aim of correlating greenness to human health. Methods for extracting greenness from hand-taken photographs resemble those used for street view images, including pixel extraction based on spectral analysis (Chen et al. Citation2023; Pearson et al. Citation2020; Puppala et al. Citation2022; Sun, Lu, and Sun Citation2021; Zhang et al. Citation2021a), software such as Photoshop (Du et al. Citation2023), AutoCAD (Han Citation2020; Citation2017), or GIMP (Falfán et al. Citation2018), as well as deep learning techniques (Yang et al. Citation2021a).
Sun, Lu, and Sun (Citation2021) conducted a study utilizing street view imagery from Zhongshan Road in Qingdao, China, in order to address issues of inequality. In a separate investigation, Chen et al. (Citation2023) analyzed the greenness in manually captured photographs of a Chinese riverbed to explore the concept of visual harmony. Yang et al. (Citation2021a) employed images taken along primary pathways within a public park in Hong Kong, China, to assess park usage patterns. Similarly, Pearson et al. (Citation2020) implemented a comparable research design in a park in the United States, with the aim of correlating greenness to human health.
Geographic Information Systems (GIS) have become a critical tool for various modeling approaches in assessing simulated eye-level urban greenness. Viewshed analysis, a popular simulation method that focuses on the geographic area visible from a given location, has been extensively applied in urban planning (Sahraoui, Clauzel, and Foltête Citation2016; Yang, Putra, and Li Citation2007). However, its implementation in urban greenness research is relatively recent. Previous studies employing viewshed analysis for urban greenness have been constrained to smaller regions due to challenges in identifying appropriate observer locations. For example, Yu et al. (Citation2016) applied viewshed analysis to a building in Shanghai’s Lujiazui region, China, where they identified observation points on different floors to extract visible urban green spaces. Similarly, Wang et al. (Citation2019) conducted a study in Beijing, China, demonstrating the effectiveness of viewshed analysis in capturing actual green space views for residents on each floor. Schäffer et al. (Citation2020) expanded the application of viewshed analysis to quantify eye-level urban greenness from a residential home. To extend the applicability of simulated eye-level urban greenness, Labib, Huck, and Lindley (Citation2021), Labib, Lindley, and Huck (Citation2021a) integrated viewshed analysis with distance decay in Great Manchester, a large city in the United Kingdom. Distance decay accounted for the diminishing perceived prominence of features as the observer distance increased. In their simulation, observation points were generated citywide at an eye level of 1.7 m, enabling the model to provide comprehensive eye-level urban greenness estimates for the entire city.
3.3. Application domains of eye-level greenness
3.3.1. Physical behavior
Physical activities have garnered significant attention due to their positive impacts on individuals and communities. Numerous studies have demonstrated that natural outdoor environments positively impact community attachment and participation (Liu et al. Citation2020), while eye-level urban greenness has been found to reduce sedentary behavior and encourage outdoor activities (Zhang et al. Citation2023). This urban feature has also been shown to increase the walkability of cities, thereby promoting walking and jogging behavior (Chen et al. Citation2022; Han Citation2017; Sun, Lu, and Sun Citation2021; Xuan, Zhao, and Asce Citation2022). Further studies have explored the impact of eye-level urban greenness on walking behavior in different settings. For example, Yang et al. (Citation2022) conducted a survey in Shanghai, China, and found that the provision of eye-level urban greenness in workplaces contributed to walking behaviors, including commuting, utilitarian, and recreational walking. Similarly, Lu, Sarkar, and Xiao (Citation2018) and Lu (Citation2018) reported that eye-level greenness and the availability of parks were positively linked to walking behavior and longer walking duration. However, the influence of eye-level urban greenness on older adults in Hong Kong, China, was found to be inconsistent with previous findings. Zang et al. (Citation2023) revealed that eye-level urban greenness had a positive effect on walking propensity among seniors within a certain range, but it turned out to have a negative effect on elderly walking beyond that range. Apart from walking, bicycling is also a common physical activity among residents (Wang et al. Citation2020). Existing studies have compared the impacts of the overhead view and eye-level urban greenness on bicycling. Chen et al. (Citation2020), Gao et al. (Citation2021), and Yu et al. (Citation2022) analyzed big data from shared bicycle companies and concluded that eye-level urban greenness had a favorable influence on cycling, while overhead green coverage did not. A similar conclusion was drawn from resident survey data by Lu et al. (Citation2019). We observe that the influence of urban greenness, particularly eye-level urban greenness, on physical activities has been extensively studied, and it is clear that this urban feature has the potential to promote physical activities, improve community health, and enhance the quality of life in urban areas.
Research conducted by Ye et al. (Citation2019) in Singapore showed that daily exposure to eye-level urban greenness, considering both greenness and accessibility, provides equivalent benefits to city residents. The study employed spatial design network analysis, using street networks obtained from OpenStreetMap, to quantify exposure to urban greenness. Labib, Lindley, and Huck (Citation2021a) developed a composite greenspace exposure index using a simulation approach that indicated a close association between greenness exposure and human health outcomes. Furthermore, Zhang and Hu (Citation2022) conducted a comprehensive analysis in Osaka, Japan, using an adjacency matrix and the Floyd-Warshall algorithm to calculate the best path for green view. The results provide guidance for maximizing urban greenness utilization by residents and visitors through both intuitive and objective analyses. Together, these studies highlight exposure to urban greenness as a key factor influencing human physical behavior. By considering accessibility and utilizing advanced analytical techniques, it is possible to optimize the design and placement of urban green spaces, thereby enhancing the benefits they provide to the communities.
3.3.2. Public health
The assessment of health outcomes typically involves the use of self-reported surveys or health records as primary data sources. O’Regan, Hunter, and Nyhan (Citation2021) reported that an increase in the interquartile range of eye-level urban greenness was associated with a 2.78% increase in self-reported good or very good health. The positive influence of eye-level urban greenness on thermal sensation and comfort vote of elderly adults was also observed (Du et al. Citation2023). However, comprehensive community-level surveys conducted in China and Singapore proposed no significant relationship between neighborhood greenness and residents’ general health (Xiao et al. Citation2021; Zhang, Tan, and Richards Citation2021b). It has been found that higher eye-level urban greenness was associated with longer sleep duration in children during mid-childhood or early adolescence. However, it is worth noting that higher exposure to eye-level urban greenness may promote the transmission of Covid-19 because the high rate of urban greenness can block the sunlight which contributes to suppressing the transmission of the virus (Zhang et al. Citation2021). Therefore, more attention requires from urban management during a pandemic.
In addition to examining general health outcomes, various specific health issues have been explored to investigate the health benefits of eye-level urban greenness. For instance, Labib, Lindley, and Huck (Citation2021a) found that a 10% increase in eye-level urban greenness exposure was associated with a one-year lower premature mortality rate. Yao et al. (Citation2022) also noted that mortality rates of ischemic heart diseases are inversely associated with eye-level urban greenness. Certain studies aimed to identify the mediating factors between eye-level urban greenness and health outcomes. Xiao et al. (Citation2021a) reported that higher eye-level urban greenness was associated with reduced body weight, and air pollution may partially mediate this relationship. Furthermore, greater greenness exposure was linked to better lung function when air pollution was present at high levels (Yu et al. Citation2021). Wang et al. (Citation2022) found that higher provision of eye-level urban greenness was associated with lower cardiovascular diseases, but none of the mediators, such as air pollution, physical exercise, or body mass index (BMI), could explain the associations. Studies also showed that the observed effects of eye-level urban greenness on various physical health outcomes were more significant in females than males (Wang et al. Citation2022; Xiao et al. Citation2021a), while male patients with ischemic heart disease could benefit more from eye-level urban greenness (Yao et al. Citation2022).
As for mental health, previous research has demonstrated that eye-level urban greenness can facilitate stress recovery (Zhang, Tan, and Richards Citation2021b). Xiao et al. (Citation2021) have uncovered a more robust link between eye-level urban greenness and mental health than physical health in a Chinese community. Helbich et al. (Citation2019) administered a survey of the shortened Geriatric Depression Scale to elderly individuals in China and found that eye-level urban greenness has a favorable effect on depression. However, further research is needed to confirm causality in this association. Using the World Health Organization Well-Being Index (WHO-5) assessment, Wang et al. (Citation2021) employed structural equation models to identify stress, social cohesion, physical activity, and life satisfaction as potential mediators of the association between eye-level urban greenness and WHO-5 results. Liu et al. (Citation2020) stated that eye-level urban greenness has a positive impact on mental health through both direct and indirect effects, which enhance neighborhood connections and participation. Furthermore, Wang et al. (Citation2021a) emphasized the importance of the quality, rather than quantity, of eye-level urban greenness in recreational areas for its favorable impact on mental health.
3.3.3. Urban planning
Urban design and scenic protection policies are fundamental to urban planning. Eye-level urban greenness has become a crucial factor in understanding green exposure in complex urban landscapes (Larkin and Hystad Citation2018). Recent studies have revealed the link between eye-level urban greenness and environmental features. Zhu et al. (Citation2022) integrated geospatial data with 17 environmental features to highlight the strong role of spatial distribution and landscape composition on the physical appearance of eye-level urban greenness. Chen et al. (Citation2023) demonstrated that eye-level urban greenness could enhance visual harmony and increase public acceptance of changes in the streaming landscape. The presence of points of interest (POI) is significantly associated with eye-level urban greenness, as Gou, Zhang, and Wang (Citation2022) found that hospitals tend to increase eye-level urban greenness while subway stations tend to decrease it. The density of POI and eye-level urban greenness serve as critical indicators of walking behavior, outweighing urban arrangement characteristics such as street accessibility and functional mixing degree (Xuan, Zhao, and Asce Citation2022).
The quality of eye-level urban greenness has become a critical factor for urban designers and planners due to the fact that it can directly reflect urban greenness acquisition from a human view. Jiang et al. (Citation2022) discovered a mismatch between vitality and quality in the streetscape, where certain vital places experience low visual quality. Tang and Long (Citation2019) evaluated the visual quality of two types of urban areas, Hutongs and historical protection blocks, in Beijing, China, and found that Hutongs exhibited inferior visual quality. This highlights the need for careful consideration of urban greenness in areas with lower visual quality. Yang et al. (Citation2021a) further emphasized the importance of green quality by demonstrating that people are more attracted to high-quality parks rather than just a large quantity of green spaces. This finding supports the notion that sufficient green provision for each resident is a critical consideration in the planning process. Even though parks are popular green space designed by the government to provide eye-level urban greenness, daily exposure to street-level or eye-level greenness is higher than exposure to a park (Zhu et al. Citation2022). It is therefore important to integrate eye-level urban greenness into urban landscape policies and management. Gu, Chen, and Dai (Citation2019) suggest that a quantitative approach to studying urban greenness can provide guidance in ensuring sufficient green provision for each resident. Dong, Zhang, and Zhao (Citation2018) demonstrated that eye-level urban greenness derived from street view images can highly represent the quantity of urban greenness along roads. Integrating eye-level urban greenness into transportation planning is a trending research area for exploring dynamic greenness exposure (Wu et al. Citation2019). Balancing and integrating qualitative and quantitative eye-level urban greenness in urban design strategies is a significant challenge that needs to be addressed.
The enhancement of the urban environment through eye-level urban greenness has been widely recognized. Wang et al. (Citation2022a) found that eye-level urban greenness could explain 2.3% of the air quality variations in the summer and 3.6% in the winter, based on the pooled data analysis at the national level in China. The potential of eye-level urban greenness in reducing air pollution was also demonstrated by Deng et al. (Citation2023) in their study of Xiamen Island, where increasing urban greenness, especially around parking lots, was recommended to mitigate air pollution. Similarly, eye-level urban greenness can reduce traffic noise, as Nourmohammadi et al. (Citation2021) suggested that increasing residential greenness in densely populated areas can effectively reduce the negative impact of traffic noise exposure. With the emergence of big data, eye-level urban greenness measurement provides a new basis for urban greenness infrastructure management. Xiao et al. (Citation2021b) proposed that regulating eye-level urban greenness exposure could be achieved by focusing on landscape configuration and large tree conservation.
3.3.4. Human perception
Higher urban density has been shown to have a negative effect on life satisfaction, particularly among elderly groups, due to a decrease in community cohesion. However, this negative impact can be mitigated by incorporating eye-level urban greenness (He et al. Citation2022). Research has shown that higher provisions of eye-level urban greenness tend to stimulate higher life satisfaction by reducing the fear of crime (Jing et al. Citation2021) and explaining 18% of the variation in life satisfaction indicators such as safety, liveliness, and beauty perception (Larkin et al. Citation2021). Limited greenness exposure can still lead to lower life satisfaction, and this association is also related to the composition of the residential family and the presence of school-age children (Wu et al. Citation2021). Eye-level urban greenness has also been found to have a positive impact on travel satisfaction, although the extent of its contribution varies depending on travel mode, duration, and purpose (Ta et al. Citation2021). Moreover, the satisfaction of running influenced by eye-level urban greenness can be affected by the number of traffic nodes and traffic lights (Huang, Jiang, and Yuan Citation2022). Furthermore, the effect of eye-level urban greenness on human life satisfaction varies across different places, with its impact on improving human life satisfaction being significant at residences but lower at the workplace (Wu et al. Citation2022) or a less significant impact (Wu et al. Citation2021a).
In addition, emotions are increasingly recognized as profoundly impacting public health and overall quality of life (Zhang et al. Citation2021a). Recent studies have shown that eye-level urban greenness can positively influence emotions. Wang et al. (Citation2022b) analyzed social media microblog data and found a positive association between higher exposure to eye-level urban greenness and lower levels of negative emotions, particularly during non-work time. Similarly, Han (Citation2017) conducted a survey on college students after a 15-minute walk or jog and concluded that eye-level urban greenness had a strong positive impact on human emotions, explaining 58% of the variance. This highlights the potential of incorporating nature into human-centric science and designing urban landscapes that promote positive emotions (Han Citation2020). In addition, Tao et al. (Citation2022) found that combining eye-level urban greenness with sky visibility has a significant positive effect on public sentiment. A study by Huang et al. (Citation2022a) echoes the findings from Tao et al. (Citation2022) by proving that increasing eye-level urban greenness can effectively enhance the positive emotions of visitors. Overall, the above evidence points out that integrating eye-level urban greenness into landscape design can have multiple benefits for residents, including promoting positive emotions and enhancing the overall quality of life.
3.3.5. Inequity
The equitable distribution of eye-level urban greenness has garnered considerable interest in human-centric science, as access to such green spaces may vary significantly within a city. Studies have revealed inequality in greenness distribution, with lower income and education individuals experiencing less exposure to eye-level urban greenness (O’Regan, Hunter, and Nyhan Citation2021). For instance, in Los Angeles, poor neighborhoods have 5% less average eye-level urban greenness exposure than other neighborhoods (Sun et al. Citation2021a). However, socioeconomically disadvantaged groups stand to benefit more from increased greenness within their neighborhoods (Wu et al. Citation2021a). To address this issue, urban planners and designers must ensure the delivery of street greenness in these neighborhoods (Wang, Feng, and Pearce Citation2022c). Moreover, the association between eye-level urban greenness and sleep varies based on different racial or socioeconomic groups. For example, Jimenez et al. (Citation2022) discovered that higher greenness is linked to poorer sleep in neighborhoods with low socioeconomic groups, while it is linked to better sleep in neighborhoods with high socioeconomic groups. Wang et al. (Citation2021b) also found that neighborhood socioeconomic status is associated with both the quantity and quality of eye-level urban greenness, with quality being more sensitive. Therefore, more studies should investigate the provision qualities of urban greenness.
The economic benefits of eye-level urban greenness are also substantial. Greater exposure to greenness is often linked to higher economic benefits (Jia and Zhang Citation2021), contributing to residents’ welfare and an increase in house prices at both global and local scales (Ye et al. Citation2019a). Yang et al. (Citation2021b) found that high exposure to eye-level urban greenness resulted in a transaction premium of 8.9% to 10.5% and a rent premium of 5.6% to 7.8% compared to low exposure in a study based on office transactions in New York City. In Beijing, homebuyers are willing to pay more for private housing estates with higher eye-level urban greenness (Zhang and Dong Citation2018). The above findings underscore the need for policymakers, urban planners, and designers to consider the social, economic, and environmental benefits of green spaces in developing sustainable and equitable urban environments that enhance the quality of life for all residents.
4. Discussion
4.1. Uniqueness of eye-level urban greenness
Eye-level urban greenness has been increasingly incorporated in research on the environmental, health, and perceptual benefits of green spaces in urban environments. This is because eye-level observations can provide unique information that remote sensing data cannot capture, such as small-scale details such as individual trees, bushes, and patches of greenery, as well as provide context regarding the quality of greenness. These observations offer a more inclusive perspective by incorporating human experiences and perceptions, which remote sensing data cannot reveal. Previous studies have demonstrated that eye-level urban greenness can mimic human perception of greenness, and its quality and quantity are positively associated with various human health outcomes, such as reduced stress, improved cognitive function, and better sleep quality (Jiang et al. Citation2022; Tang and Long Citation2019; Yang et al. Citation2021). Furthermore, eye-level observations can reveal the social and cultural values associated with green spaces, which is important for understanding how these spaces contribute to human well-being and the overall livability of urban environments (Larkin and Hystad Citation2018; Wang et al. Citation2021).
Despite the advantages of eye-level observations, most studies have relied solely on remote sensing data to investigate its effects on human-related associations. While remote sensing methods provide valuable information about urban greenness from a top-down perspective, we believe incorporating eye-level observations can offer a more nuanced understanding of the distribution, quality, and social values of green spaces in urban environments. According to the findings of Barona et al. (Citation2023), eye-level urban greenness exhibited a stronger positive association with human satisfaction concerning urban trees compared to the top-down perspective. Therefore, we argue that the integration of eye-level urban greenness in future research and urban planning should be considered as a key strategy to promote the environmental, health, and perceptual benefits of green spaces in cities.
4.2. Challenges in measuring eye-level urban greenness
Measuring eye-level urban greenness in large-scale areas requires innovative and efficient methods. Two methods that have been used are street view images and simulation, each with its unique advantages and limitations. Street view images provide a means to incorporate human perception from a profile view into urban science, enabling accurate representation of the scene as seen by a person. Recent advances in deep learning and computer vision technologies have facilitated the extraction of urban greenness from street view images, making it an excellent data source for measuring eye-level urban greenness. However, the spatial coverage and temporal range of street view images vary greatly, and there are limitations in coverage in areas where vehicles cannot access them. For example, human activities are not confined solely to streets; individuals frequently inhabit parks, indoor spaces, and a range of other outdoor settings. In such contexts, employing 360-degree imagery to compute eye-level greenness might not be pertinent. A study by Kim and Jang (Citation2023) examined the spatial coverage and temporal range of Google Street View images in 45 small- and medium-sized cities in the United States and found that 45% of commute routes lacked adequate coverage. Although efforts to incorporate crowdsourcing street view images (e.g. from Mapillary) have been made, the coverage limitation still exists and requires further investigation. Besides, it is important to note that certain proprietary image providers, such as Google, have restrictions on the extraction of information from their images. Extracting data under Fair Usage Policy might come with costs and legal issues, potentially limiting scalability for teams with financial constraints.
In comparison, simulation provides a comprehensive assessment of eye-level urban greenness by creating a 3D scene that simulates a person standing at an observer location and calculating the greenness ratio in all directions. This approach offers a solid and scientific basis for future large-scale and even nationwide measurements of urban greenness. Simulated greenness estimation can be easily accessed using commonly available open-source geospatial data such as LiDAR point cloud or satellite-based earth observations, expanding the coverage of greenness assessment to areas that are not only street view accessible but also human-accessible. The advantages of the simulation make large-scale, timely, and full coverage estimation of greenness feasible. However, the simulation approach faces challenges related to the high computational demand, which increases exponentially for larger geographic areas. Therefore, optimizing computational efficiency is desired to enable large-scale and accurate simulation of urban greenness. Cimburova and Blumentrath (Citation2022) developed a useful GIS tool based on the simulation method to accelerate the computational speed and enlarge the scope of the simulation application to more practitioners. Besides, Brinkmann, Kremer, and Walker (Citation2022) also applied a prototype strategy to speed up the capitation efficiency from 11.5 days to 73 min. Therefore, we believe the simulated approach has great potential to provide a more comprehensive representation of eye-level urban greenness with evolving acceleration techniques.
Yan et al. (Citation2023) verified that the simulation has a robust performance in replacing or alternating the street view image by conducting a field experiment to collect 360-degree panoramas. Torkko et al. (Citation2023) also claimed that the use of both street view images and simulation approaches can complement each other and provide more accurate and comprehensive measures of eye-level urban greenness in large-scale areas. We argue that future research should focus on further exploring the capabilities of street view images and simulation approaches for measuring eye-level urban greenness in large-scale areas. This includes addressing coverage limitations and optimizing computational efficiency. By integrating these methods into urban science, researchers and urban planners can develop more comprehensive and accurate assessments of the benefits of urban greenness for human health and well-being, supporting evidence-based decision-making for urban planning and design.
4.3. Toward a human-centric agenda for urban greenness
Urban green spaces are becoming increasingly important as cities continue to expand and develop both in population and areas. The diversity of greenery at eye level in urban environments is vast, with a variety of plant species. We argue that future research needs to focus on the distinct advantages and disadvantages of each type of greenery and how their combination can contribute to creating the most effective urban green spaces. To address this need, standardized metrics for measuring and comparing greenness across various cities and landscapes should be developed. The standardization of measurement methodologies would allow researchers and practitioners in the fields of urban ecology, landscape architecture, and other related fields to communicate and share findings effectively. Collaborative efforts can drive the development of a comprehensive framework for measuring and comparing eye-level urban greenness, which should take into account the unique characteristics of each type of greenery and their interactions, as well as the cultural, social, and economic factors that influence green space planning and management. The benefits of such a framework are manifold. It can help identify areas where additional greenery is needed and guide the development of policies that promote creating and maintaining high-quality urban green spaces.
Recent research has highlighted the positive impact of urban green spaces on human health and physical activity. However, the significance of individual perceptions and emotions toward urban green spaces has been predominantly overlooked. Thus, it is imperative to adopt a more comprehensive approach that encompasses the holistic benefits of greenery, including its impact on emotional well-being, inequality, and the economy. Related research should be undertaken across diverse countries and regions to ensure global consistency in progress. Eye-level urban greenness is a fundamental component of green infrastructure, which provides ecosystem services in urban areas. Integrating eye-level urban greenness into broader green infrastructure planning and design can optimize its potential benefits. Furthermore, incorporating community input into the planning and design of urban green spaces is critical to ensure they align with the needs and preferences of local residents. Urban design strategies are pivotal in determining the amount and quality of greenery at the street level. Hence, future research should examine the efficacy of different urban design strategies in promoting eye-level greenness and identifying methods to maximize greenery in both quality and quantity. Such research can inform urban planners and policymakers on how to create more sustainable and livable cities that prioritize the well-being of their citizens.
Even though the concept of visual exposure in our study serves as a proxy, offering a valuable starting point for understanding the potential positive effects of urban greenness on human satisfaction and well-being, it is important to distinguish that our study primarily focuses on visual exposure to green space, recognizing that this aspect may not encompass the full range of exposure types relevant to human health. Visual exposure to greenery, as captured by eye-level greenness, provides a valuable dimension of experiencing urban nature. However, it is crucial to acknowledge that it does not necessarily equate to other forms of exposure, such as the physical benefits of shade, air quality improvement, or the psychological impact of sensory engagement with green spaces. Future investigations may explore the interplay between visual exposure and other forms of exposure to green space and their respective contributions to human health and well-being. This nuanced understanding could provide a more comprehensive view of the multifaceted benefits of urban greenery, taking into account both the visual and non-visual dimensions of exposure.
4.4. Limitations of this study
Our study acknowledges specific limitations pertaining to our research methodology. Primarily, our literature search was executed using the Web of Science database, a renowned repository for academic publications. While comprehensive, it does not encapsulate all available research; databases like Scopus might house exclusive articles absent from our analysis. We understand the inherent disparities that can emerge from utilizing different databases. Furthermore, the potential for oversight of pertinent articles due to keyword discrepancies or terminological variations is recognized. Even with a meticulous approach to search terms, it is conceivable that some germane papers eluded our search. Acknowledging the fallibility of any single search approach, there could be supplementary studies pertinent to our topic that were omitted. To address these constraints in subsequent research, we advocate for multi-database searches, enriched by diverse search terms and synonyms, ensuring a more exhaustive literature review. We also suggest that scholars consult alternative data sources to augment the breadth of their literary exploration on the topic.
5. Conclusion
Urban greenness offers an extensive array of benefits for individuals and communities, encompassing enhanced health, well-being, and participation in physical activities. The significance of assessing urban greenness from a person-centered standpoint, as opposed to a bird's-eye view, has gained increasing recognition in human-centric research. In order to deliver an exhaustive synopsis of measurement techniques and pertinent topics within the existing literature, we conducted a systematic review of 77 scholarly articles. Our principal findings reveal that street view imagery is the primary data source for quantifying eye-level urban greenness in urban studies. Meanwhile, simulated eye-level urban greenness emerges as a cutting-edge prospect for the public and urban planners, offering a promising opportunity for quantifying greenness exposure at various scales. Moreover, eye-level urban greenness has been investigated across various disciplines, including physical and mental health, urban planning, human perception, social inequality, and economic impact. This underscores a noteworthy correlation between eye-level urban greenness and the well-being of individuals and communities. Our systematic review offers holistic perspectives on integrating urban greenness into urban planning and design, advocating for a heightened emphasis on eye-level urban greenness as a crucial element of urban environmental quality. Upcoming research endeavors ought to scrutinize the effectiveness of various urban design approaches in fostering eye-level greenness and pinpointing techniques to optimize green spaces in urban settings and develop more sustainable and habitable cities.
Disclosure statement
No potential conflict of interest was reported by the author(s).
Correction Statement
This article has been corrected with minor changes. These changes do not impact the academic content of the article.
References
- Abutaleb, K., M. Freddy Mudede, N. Nkongolo, and S. W. Newete. 2021. “Estimating Urban Greenness Index Using Remote Sensing Data: A Case Study of an Affluent vs Poor Suburbs in the City of Johannesburg.” The Egyptian Journal of Remote Sensing and Space Science 24 (3): 343–351. https://doi.org/10.1016/J.EJRS.2020.07.002.
- Acuti, D., L. Grazzini, V. Mazzoli, and G. Aiello. 2019. “Stakeholder Engagement in Green Place Branding: A Focus on User-generated Content.” Corporate Social Responsibility and Environmental Management 26 (2): 492–501. https://doi.org/10.1002/CSR.1703.
- Barona, C. O., S. M. Labib, L. Chung, and T. Conway. 2023. “Satisfaction with Urban Trees Associates with Tree Canopy Cover and Tree Visibility Around the Home.”
- Bennett, N. J., R. Roth, S. C. Klain, K. Chan, P. Christie, D. A. Clark, G. Cullman, et al. 2017. “Conservation Social Science: Understanding and Integrating Human Dimensions to Improve Conservation.” Biological Conservation 205:93–108. https://doi.org/10.1016/j.biocon.2016.10.006.
- Biljecki, F., and K. Ito. 2021. “Street View Imagery in Urban Analytics and GIS: A Review.” Landscape and Urban Planning 215:104217. https://doi.org/10.1016/j.landurbplan.2021.104217.
- Bowler, D. E., L. Buyung-Ali, T. M. Knight, and A. S. Pullin. 2010. “Urban Greening to Cool Towns and Cities: A Systematic Review of the Empirical Evidence.” Landscape and Urban Planning 97 (3): 147–155. https://doi.org/10.1016/j.landurbplan.2010.05.006.
- Brinkmann, S. T., D. Kremer, and B. B. Walker. 2022. “Modelling Eye-level Visibility of Urban Green Space: Optimising City-wide Point-based Viewshed Computations Through Prototyping.” AGILE: GIScience Series 3:27. https://doi.org/10.5194/agile-giss-3-27-2022.
- Chen, Yiyong, Yu Chen, W. Tu, and X. Zeng. 2020. “Is Eye-level Greening Associated with the Use of Dockless Shared Bicycles?” Urban Forestry & Urban Greening 51:126690. https://doi.org/10.1016/j.ufug.2020.126690.
- Chen, J. C., X. R. Fan, J. Q. Fan, X. Z. Lai, G. L. Li, and F. B. Li. 2023. “Visual Harmony of the Proportion of Water and Greenery in Urban Streams: Baxi Stream, Yongan City, China.” Water 15:341–341. https://doi.org/10.3390/w15020341.
- Chen, L., Y. Lu, Y. Ye, Y. Xiao, and L. Yang. 2022. “Examining the Association between the Built Environment and Pedestrian Volume Using Street View Images.” Cities 127:103734. https://doi.org/10.1016/j.cities.2022.103734.
- Chen, X., Q. Meng, D. Hu, L. Zhang, and J. Yang. 2019. “Evaluating Greenery Around Streets Using Baidu Panoramic Street View Images and the Panoramic Green View Index.” Forests 10:1109. https://doi.org/10.3390/f10121109.
- Chen, K., T. Zhang, F. Liu, Y. Zhang, and Y. Song. 2021. “How does Urban Green Space Impact Residents’ Mental Health: A Literature Review of Mediators.” International Journal of Environmental Research & Public Health 18. https://doi.org/10.3390/IJERPH182211746/S1.
- Cimburova, Z., and S. Blumentrath. 2022. “Viewshed-based Modelling of Visual Exposure to Urban greenery–An Efficient GIS Tool for Practical Planning Applications.” Landscape and Urban Planning 222:104395. https://doi.org/10.1016/j.landurbplan.2022.104395.
- de Keijzer, C., M. Bauwelinck, and P. Dadvand. 2020. “Long-term Exposure to Residential Greenspace and Healthy Ageing: A Systematic Review.” Current Environmental Health Reports 7 (1): 65–88. https://doi.org/10.1007/s40572-020-00264-7.
- Deng, X., F. Gao, S. Liao, and S. Li. 2023. “Unraveling the Association between the Built Environment and Air Pollution from a Geospatial Perspective.” Journal of Cleaner Production 386:135768. https://doi.org/10.1016/j.jclepro.2022.135768.
- Dong, R., Y. Zhang, and J. Zhao. 2018. “How Green are the Streets Within the Sixth Ring Road of Beijing? An Analysis Based on Tencent Street View Pictures and the Green View Index.” International Journal of Environmental Research and Public Health 15:1367. https://doi.org/10.3390/ijerph15071367.
- Du, M., B. Hong, C. Gu, Y. Li, and Y. Wang. 2023. “Multiple Effects of Visual-Acoustic-thermal Perceptions on the Overall Comfort of Elderly Adults in Residential Outdoor Environments.” Energy and Buildings 283:112813. https://doi.org/10.1016/j.enbuild.2023.112813.
- Dzhambov, A. M., and D. D. Dimitrova. 2014. “Urban Green Spaces’ Effectiveness as a Psychological Buffer for the Negative Health Impact of Noise Pollution: A Systematic Review.” Noise and Health 16 (70): 157. https://doi.org/10.4103/1463-1741.134916.
- Falfán, I., C. A. Muñoz-Robles, M. Bonilla-Moheno, and I. MacGregor-Fors. 2018. “Can you Really See ‘Green’? Assessing Physical and Self-reported Measurements of Urban Greenery.” Urban Forestry & Urban Greening 36:13–21. https://doi.org/10.1016/j.ufug.2018.08.016.
- Fernández Núñez, M. B., L. Campos Suzman, R. Maneja, A. Bach, O. Marquet, I. Anguelovski, and P. Knobel. 2022. “The Differences by Sex and Gender in the Relationship between Urban Greenness and Cardiometabolic Health: A Systematic Review.” Journal of Urban Health 99 (6): 1054–1067. https://doi.org/10.1007/s11524-022-00685-9.
- Franchini, M., and P. M. Mannucci. 2018. “Mitigation of air Pollution by Greenness: A Narrative Review.” European Journal of Internal Medicine 55:1–5. https://doi.org/10.1016/j.ejim.2018.06.021.
- Gao, F., S. Li, Z. Tan, X. Zhang, Z. Lai, and Z. Tan. 2021. “How is Urban Greenness Spatially Associated with Dockless Bike Sharing Usage on Weekdays, Weekends, and Holidays?” ISPRS International Journal of Geo-Information 10:238. https://doi.org/10.3390/ijgi10040238.
- Goddard, M. A., A. J. Dougill, and T. G. Benton. 2010. “Scaling up from gardens: biodiversity conservation in urban environments.” Trends in ecology and evolution 25 (2): 90–98.
- Gou, A., C. Zhang, and J. Wang. 2022. “Study on the Identification and Dynamics of Green Vision Rate in Jing’an District, Shanghai Based on Deeplab V3 + Model.” Earth Science Informatics 15 (1): 163–181. https://doi.org/10.1007/s12145-021-00691-6.
- Gu, W., Y. Chen, and M. Dai. 2019. “Measuring Community Greening Merging Multi-Source Geo-data.” Sustainability 11:1104. https://doi.org/10.3390/su11041104.
- Han, K. T. 2017. “The Effect of Nature and Physical Activity on Emotions and Attention While Engaging in Green Exercise.” Urban Forestry & Urban Greening 24:5–13. https://doi.org/10.1016/j.ufug.2017.03.012.
- Han, K. T. 2020. “The Effect of Environmental Factors and Physical Activity on Emotions and Attention While Walking and Jogging.” 52:619–641. https://doi.org/10.1080/00222216.2020.1788474.
- He, D., J. Miao, Y. Lu, Y. Song, L. Chen, and Y. Liu. 2022. “Urban Greenery Mitigates the Negative Effect of Urban Density on Older Adults’ Life Satisfaction: Evidence from Shanghai, China.” Cities 124:103607. https://doi.org/10.1016/j.cities.2022.103607.
- Helbich, M., Y. Yao, Y. Liu, J. Zhang, P. Liu, and R. Wang. 2019. “Using Deep Learning to Examine Street View Green and Blue Spaces and their Associations with Geriatric Depression in Beijing, China.” Environment International 126:107–117. https://doi.org/10.1016/j.envint.2019.02.013.
- Huang, D., B. Jiang, and L. Yuan. 2022. “Analyzing the Effects of Nature Exposure on Perceived Satisfaction with Running Routes: An Activity Path-based Measure Approach.” Urban Forestry & Urban Greening 68:127480. https://doi.org/10.1016/j.ufug.2022.127480.
- Huang, S., J. Zhu, K. Zhai, Y. Wang, H. Wei, Z. Xu, and X. Gu. 2022a. “Do Emotional Perceptions of Visible Greeneries Rely on the Largeness of Green Space? A Verification in Nanchang, China.” Forests 13:1192. https://doi.org/10.3390/f13081192.
- Jia, J., and X. Zhang. 2021. “A Human-scale Investigation into Economic Benefits of Urban Green and Blue Infrastructure Based on Big Data and Machine Learning: A Case Study of Wuhan.” Journal of Cleaner Production 316:128321. https://doi.org/10.1016/j.jclepro.2021.128321.
- Jiang, Y., Y. Han, M. Liu, and Y. Ye. 2022. “Street Vitality and Built Environment Features: A Data-informed Approach from Fourteen Chinese Cities.” Sustainable Cities and Society 79:103724. https://doi.org/10.1016/j.scs.2022.103724.
- Jiao, J., J. Rollo, B. Fu, and C. Liu. 2021. “Exploring Effective Built Environment Factors for Evaluating Pedestrian Volume in High-Density Areas: A New Finding for the Central Business District in Melbourne, Australia.” Land 10:655. https://doi.org/10.3390/land10060655.
- Jimenez, M. P., E. Suel, S. L. Rifas-Shiman, P. Hystad, A. Larkin, S. Hankey, A. C. Just, S. Redline, E. Oken, and P. James. 2022. “Street-view Greenspace Exposure and Objective Sleep Characteristics among Children.” Environmental Research 214:113744. https://doi.org/10.1016/j.envres.2022.113744.
- Jing, F., L. Liu, S. Zhou, J. Song, L. Wang, H. Zhou, Y. Wang, and R. Ma. 2021. “Assessing the Impact of Street-view Greenery on Fear of Neighborhood Crime in Guangzhou, China.” International Journal of Environmental Research and Public Health 18:311. https://doi.org/10.3390/ijerph18010311.
- Kim, J., and K. M. Jang. 2023. “An Examination of the Spatial Coverage and Temporal Variability of Google Street View (GSV) Images in Small- and Medium-Sized Cities: A People-based Approach.” Computers, Environment and Urban Systems 102:101956. https://doi.org/10.1016/j.compenvurbsys.2023.101956.
- Kumakoshi, Y., S. Y. Chan, H. Koizumi, X. Li, and Y. Yoshimura. 2020. “Standardized Green View Index and Quantification of Different Metrics of Urban Green Vegetation.” Sustainability 12:7434. https://doi.org/10.3390/su12187434.
- Labib, S. M., J. J. Huck, and S. Lindley. 2021. “Modelling and Mapping Eye-level Greenness Visibility Exposure Using Multi-source Data at High Spatial Resolutions.” Science of the Total Environment 755:143050. https://doi.org/10.1016/j.scitotenv.2020.143050.
- Labib, S. M., Sarah Lindley, and Jonny J. Huck. 2020. “Spatial Dimensions of the Influence of Urban Green-blue Spaces on Human Health: A Systematic Review.” Environmental Research 180:108869. https://doi.org/10.1016/j.envres.2019.108869.
- Labib, S. M., S. Lindley, and J. J. Huck. 2021a. “Estimating Multiple Greenspace Exposure Types and their Associations with Neighbourhood Premature Mortality: A Socioecological Study.” Science of The Total Environment 789:147919. https://doi.org/10.1016/j.scitotenv.2021.147919.
- Lakhani, A., M. Norwood, D. P. Watling, H. Zeeman, and E. Kendall. 2019. “Using the Natural Environment to Address the Psychosocial Impact of Neurological Disability: A Systematic Review.” Health & Place 55:188–201. https://doi.org/10.1016/j.healthplace.2018.12.002.
- Lambert, A., J. Vlaar, S. Herrington, and M. Brussoni. 2019. “What is the Relationship between the Neighbourhood Built Environment and Time Spent in Outdoor Play? A Systematic Review.” International Journal of Environmental Research and Public Health 16:3840. https://doi.org/10.3390/ijerph16203840.
- Larkin, A., X. Gu, L. Chen, and P. Hystad. 2021. “Predicting Perceptions of the Built Environment Using GIS, Satellite and Street View Image Approaches.” Landscape and Urban Planning 216:104257. https://doi.org/10.1016/j.landurbplan.2021.104257.
- Larkin, A., and P. Hystad. 2018. “Evaluating Street View Exposure Measures of Visible Green Space for Health Research.” Journal of Exposure Science & Environmental Epidemiology 29 (4): 447–456. https://doi.org/10.1038/s41370-018-0017-1.
- Li, W. C., and K. K. A. Yeung. 2014. “A Comprehensive Study of Green Roof Performance from Environmental Perspective.” International Journal of Sustainable Built Environment 3 (1): 127–134. https://doi.org/10.1016/j.ijsbe.2014.05.001.
- Li, X., C. Zhang, W. Li, R. Ricard, Q. Meng, and W. Zhang. 2015. “Assessing Street-level Urban Greenery Using Google Street View and a Modified Green View Index.” Urban Forestry & Urban Greening 14 (3): 675–685. https://doi.org/10.1016/j.ufug.2015.06.006.
- Liu, Y., R. Wang, Y. Lu, Z. Li, H. Chen, M. Cao, Y. Zhang, and Y. Song. 2020. “Natural Outdoor Environment, Neighbourhood Social Cohesion and Mental Health: Using Multilevel Structural Equation Modelling, Streetscape and Remote-Sensing Metrics.” Urban Forestry & Urban Greening 48:126576. https://doi.org/10.1016/j.ufug.2019.126576.
- Lu, Y. 2018. “The Association of Urban Greenness and Walking Behavior: Using Google Street View and Deep Learning Techniques to Estimate Residents’ Exposure to Urban Greenness.” International Journal of Environmental Research and Public Health 15:1576. https://doi.org/10.3390/ijerph15081576.
- Lu, Y. 2019. “Using Google Street View to Investigate the Association between Street Greenery and Physical Activity.” Landscape and Urban Planning 191:103435. https://doi.org/10.1016/j.landurbplan.2018.08.029.
- Lu, Y., C. Sarkar, and Y. Xiao. 2018. “The Effect of Street-level Greenery on Walking Behavior: Evidence from Hong Kong.” Social Science & Medicine 208:41–49. https://doi.org/10.1016/j.socscimed.2018.05.022.
- Lu, Y., Y. Yang, G. Sun, and Z. Gou. 2019. “Associations between Overhead-view and Eye-level Urban Greenness and Cycling Behaviors.” Cities 88:10–18. https://doi.org/10.1016/j.cities.2019.01.003.
- Malhi, Y. 2017. “The Concept of the Anthropocene.” Annual Review of Environment and Resources 42:77–104. https://doi.org/10.1146/ANNUREV-ENVIRON-102016-060854.
- McMorris, O., P. J. Villeneuve, J. Su, and M. Jerrett. 2015. “Urban Greenness and Physical Activity in a National Survey of Canadians.” Environmental Research 137:94–100. https://doi.org/10.1016/j.envres.2014.11.010.
- Moher, D., A. Liberati, J. Tetzlaff, D. G. Altman, D. Altman, G. Antes, D. Atkins, et al. 2009. “Preferred Reporting Items for Systematic Reviews and Meta-analyses: The PRISMA Statement.” PLoS Medicine 6:e1000097. https://doi.org/10.1371/journal.pmed.1000097.
- Nourmohammadi, Z., T. Lilasathapornkit, M. Ashfaq, Z. Gu, and M. Saberi. 2021. “Mapping Urban Environmental Performance with Emerging Data Sources: A Case of Urban Greenery and Traffic Noise in Sydney, Australia.” Sustainability 13:605. https://doi.org/10.3390/su13020605.
- Ordóñez, C., S. M. Labib, L. Chung, and T. M. Conway. 2023. “Satisfaction with Urban Trees Associates with Tree Canopy Cover and Tree Visibility Around the Home.” NPJ Urban Sustainability 3 (1): 37. https://doi.org/10.1038/s42949-023-00119-8.
- O’Regan, A. C., R. F. Hunter, and M. M. Nyhan. 2021. “‘Biophilic Cities’: Quantifying the Impact of Google Street View-derived Greenspace Exposures on Socioeconomic Factors and Self-Reported Health.” Environmental Science & Technology 55 (13): 9063–9073. https://doi.org/10.1021/acs.est.1c01326.
- Pearson, A. L., K. A. Pfeiffer, J. Gardiner, T. Horton, R. T. Buxton, R. F. Hunter, V. Breeze, and T. McDade. 2020. “Study of Active Neighborhoods in Detroit (StAND): Study Protocol for a Natural Experiment Evaluating the Health Benefits of Ecological Restoration of Parks.” BMC Public Health 20 (1): 1–14. https://doi.org/10.1186/s12889-019-7969-5.
- Puppala, H., J. P. Tamvada, B. Kim, and P. R. T. Peddinti. 2022. “Enhanced Green View Index.” MethodsX 9:101824. https://doi.org/10.1016/j.mex.2022.101824.
- Qian, Y., W. Zhou, W. Yu, and S. T. A. Pickett. 2015. “Quantifying Spatiotemporal Pattern of Urban Greenspace: New Insights from High Resolution Data.” Landscape Ecology 30 (7): 1165–1173. https://doi.org/10.1007/s10980-015-0195-3.
- Sadeh, M., M. Brauer, R. Dankner, N. Fulman, and A. Chudnovsky. 2021. “Remote Sensing Metrics to Assess Exposure to Residential Greenness in Epidemiological Studies: A Population Case Study from the Eastern Mediterranean.” Environment International 146:106270. https://doi.org/10.1016/j.envint.2020.106270.
- Sahraoui, Y., C. Clauzel, and J. C. Foltête. 2016. “Spatial Modelling of Landscape Aesthetic Potential in Urban-Rural Fringes.” Journal of Environmental Management 181:623–636. https://doi.org/10.1016/j.jenvman.2016.06.031.
- Schäffer, B., M. Brink, F. Schlatter, D. Vienneau, and J. M. Wunderli. 2020. “Residential Green is Associated with Reduced Annoyance to Road Traffic and Railway Noise but Increased Annoyance to Aircraft Noise Exposure.” Environment International 143:105885. https://doi.org/10.1016/j.envint.2020.105885.
- Shafique, M., R. Kim, and M. Rafiq. 2018. “Green Roof Benefits, Opportunities and Challenges – A Review.” Renewable and Sustainable Energy Reviews 90:757–773. https://doi.org/10.1016/j.rser.2018.04.006.
- Shin, J. C., K. V. Parab, R. An, and D. S. Grigsby-Toussaint. 2020. “Greenspace Exposure and Sleep: A Systematic Review.” Environmental Research 182:109081. https://doi.org/10.1016/j.envres.2019.109081.
- Sun, Y., W. Lu, and P. Sun. 2021. “Optimization of Walk Score Based on Street Greening – A Case Study of Zhongshan Road in Qingdao.” International Journal of Environmental Research and Public Health 18:1277. https://doi.org/10.3390/ijerph18031277.
- Sun, Yi, X. Wang, J. Zhu, L. Chen, Y. Jia, J. M. Lawrence, L. hua Jiang, X. Xie, and J. Wu. 2021a. “Using Machine Learning to Examine Street Green Space Types at a High Spatial Resolution: Application in Los Angeles County on Socioeconomic Disparities in Exposure.” Science of The Total Environment 787:147653. https://doi.org/10.1016/j.scitotenv.2021.147653.
- Ta, N., H. Li, Y. Chai, and J. Wu. 2021. “The Impact of Green Space Exposure on Satisfaction with Active Travel Trips.” Transportation Research Part D: Transport and Environment 99:103022. https://doi.org/10.1016/j.trd.2021.103022.
- Tang, J., and Y. Long. 2019. “Measuring Visual Quality of Street Space and its Temporal Variation: Methodology and its Application in the Hutong Area in Beijing.” Landscape and Urban Planning 191:103436. https://doi.org/10.1016/j.landurbplan.2018.09.015.
- Tao, J., M. Yang, J. Wu, J. Tao, M. Yang, and J. Wu. 2022. “Coupling Coordination Evaluation of Lakefront Landscape Spatial Quality and Public Sentiment.” Land 11:865. https://doi.org/10.3390/land11060865.
- Thomsen, J. M., R. B. Powell, and C. Monz. 2018. “A Systematic Review of the Physical and Mental Health Benefits of Wildland Recreation.” Journal of Park and Recreation Administration 36:123–148. https://doi.org/10.18666/JPRA-2018-V36-I1-8095.
- Torkko, J., A. Poom, E. Willberg, and T. Toivonen. 2023. “How to Best Map Greenery from a Human Perspective? Comparing Computational Measurements with Human Perception.” Frontiers in Sustainable Cities 5:1160995. https://doi.org/10.3389/frsc.2023.1160995.
- Twohig-Bennett, C., and A. Jones. 2018. “The Health Benefits of the Great Outdoors: A Systematic Review and Meta-analysis of Greenspace Exposure and Health Outcomes.” Environmental Research 166:628–637. https://doi.org/10.1016/j.envres.2018.06.030.
- Wang, J., and F. Biljecki. 2022. “Unsupervised machine learning in urban studies: A systematic review of applications.” Cities 129: 103925.
- Wang, R., M. H. E. M. Browning, X. Qin, J. He, W. Wu, Y. Yao, and Y. Liu. 2022b. “Visible Green Space Predicts Emotion: Evidence from Social Media and Street View Data.” Applied Geography 148:102803. https://doi.org/10.1016/j.apgeog.2022.102803.
- Wang, R., P. Dong, G. Dong, X. Xiao, J. Huang, L. Yang, Y. Yu, and G. H. Dong. 2022. “Exploring the Impacts of Street-Level Greenspace on Stroke and Cardiovascular Diseases in Chinese Adults.” Ecotoxicology and Environmental Safety 243:113974. https://doi.org/10.1016/j.ecoenv.2022.113974.
- Wang, R., Z. Feng, and J. Pearce. 2022c. “Neighbourhood Greenspace Quantity, Quality and Socioeconomic Inequalities in Mental Health.” Cities 129:103815. https://doi.org/10.1016/j.cities.2022.103815.
- Wang, R., Z. Feng, J. Pearce, Y. Liu, and G. Dong. 2021. “Are Greenspace Quantity and Quality Associated with Mental Health through Different Mechanisms in Guangzhou, China: A Comparison Study Using Street View Data.” Environmental Pollution 290:117976. https://doi.org/10.1016/j.envpol.2021.117976.
- Wang, R., Z. Feng, J. Pearce, Y. Yao, X. Li, and Y. Liu. 2021b. “The Distribution of Greenspace Quantity and Quality and Their Association with Neighbourhood Socioeconomic Conditions in Guangzhou, China: A New Approach Using Deep Learning Method and Street View Images.” Sustainable Cities and Society 66:102664. https://doi.org/10.1016/j.scs.2020.102664.
- Wang, R., Z. Feng, J. Pearce, S. Zhou, L. Zhang, and Y. Liu. 2021a. “Dynamic Greenspace Exposure and Residents’ Mental Health in Guangzhou, China: From Over-Head to Eye-level Perspective, from Quantity to Quality.” Landscape and Urban Planning 215:104230. https://doi.org/10.1016/j.landurbplan.2021.104230.
- Wang, W., Z. Lin, L. Zhang, T. Yu, P. Ciren, and Y. Zhu. 2019. “Building Visual Green Index: A Measure of Visual Green Spaces for Urban Building.” Urban Forestry & Urban Greening 40:335–343. https://doi.org/10.1016/j.ufug.2018.04.004.
- Wang, R., Y. Lu, X. Wu, Y. Liu, and Y. Yao. 2020. “Relationship between Eye-level Greenness and Cycling Frequency Around Metro Stations in Shenzhen, China: A Big Data Approach.” Sustainable Cities and Society 59:102201. https://doi.org/10.1016/j.scs.2020.102201.
- Wang, W., P. Tian, J. Zhang, E. Agathokleous, L. Xiao, T. Koike, H. Wang, and X. He. 2022a. “Big Data-Based Urban Greenness in Chinese Megalopolises and Possible Contribution to air Quality Control.” Science of The Total Environment 824:153834. https://doi.org/10.1016/j.scitotenv.2022.153834.
- Watkins, R., J. Palmer, and M. Kolokotroni. 2007. “Increased Temperature and Intensification of the Urban Heat Island: Implications for Human Comfort and Urban Design.” Built Environment 33:85–96. https://doi.org/10.2148/benv.33.1.85.
- Wu, W., W. Y. Chen, Y. Yun, F. Wang, and Z. Gong. 2022. “Urban Greenness, Mixed Land-use, and Life Satisfaction: Evidence from Residential Locations and Workplace Settings in Beijing.” Landscape and Urban Planning 224:104428. https://doi.org/10.1016/j.landurbplan.2022.104428.
- Wu, J., L. Cheng, S. Chu, N. Xia, and M. Li. 2019. “A Green View Index for Urban Transportation: How Much Greenery do we View While Moving Around in Cities?” International Journal of Sustainable Transportation 14:972–989. https://doi.org/10.1080/15568318.2019.1672001.
- Wu, W., Y. Yao, Y. Song, D. He, and R. Wang. 2021a. “Perceived Influence of Street-level Visible Greenness Exposure in the Work and Residential Environment on Life Satisfaction: Evidence from Beijing, China.” Urban Forestry & Urban Greening 62:127161. https://doi.org/10.1016/j.ufug.2021.127161.
- Wu, W., Y. Yun, J. Zhai, Y. Sun, G. Zhang, and R. Wang. 2021. “Residential Self-selection in the Greenness-wellbeing Connection: A Family Composition Perspective.” Urban Forestry & Urban Greening 59:127000. https://doi.org/10.1016/j.ufug.2021.127000.
- Xia, Y., N. Yabuki, and T. Fukuda. 2021. “Development of a System for Assessing the Quality of Urban Street-level Greenery Using Street View Images and Deep Learning.” Urban Forestry & Urban Greening 59:126995. https://doi.org/10.1016/j.ufug.2021.126995.
- Xiao, Y., S. Miao, Y. Zhang, H. Chen, and W. WU. 2021. “Exploring the Health Effects of Neighborhood Greenness on Lilong Residents in Shanghai.” Urban Forestry & Urban Greening 66:127383. https://doi.org/10.1016/j.ufug.2021.127383.
- Xiao, X., R. Wang, L. D. Knibbs, B. Jalaludin, J. Heinrich, I. Markevych, M. Gao, et al. 2021a. “Street View Greenness is Associated with Lower Risk of Obesity in Adults: Findings from the 33 Chinese Community Health Study.” Environmental Research 200:111434. https://doi.org/10.1016/j.envres.2021.111434.
- Xiao, L., W. Wang, Z. Ren, Y. Fu, H. Lv, and X. He. 2021b. “Two-city Street-View Greenery Variations and Association with Forest Attributes and Landscape Metrics in NE China.” Landscape Ecology 36:1261–1280. https://doi.org/10.1007/s10980-021-01210-0.
- Xie, L., and S. Milcheva. 2022. “ESG Investors and Local Greenness: Evidence from Infrastructure Deals.” SSRN Electronic Journal. https://doi.org/10.2139/SSRN.4264934.
- Xuan, W., L. Zhao, and S. M. Asce. 2022. “Research on Correlation between Spatial Quality of Urban Streets and Pedestrian Walking Characteristics in China Based on Street View Big Data.” Journal of Urban Planning and Development 148 (4): 05022035. https://doi.org/10.1061/(ASCE)UP.1943-5444.0000888.
- Yan, J., R. Naghedi, X. Huang, Siqin Wang, Junyu Lu, and Yang Xu. 2023. “Evaluating Simulated Visible Greenness in Urban Landscapes: An Examination of a Midsize US City.” Urban Forestry & Urban Greening 87:128060. https://doi.org/10.1016/j.ufug.2023.128060.
- Yang, Y., Y. Lu, H. Yang, L. Yang, and Z. Gou. 2021a. “Impact of the Quality and Quantity of Eye-level Greenery on Park Usage.” Urban Forestry & Urban Greening 60:127061. https://doi.org/10.1016/j.ufug.2021.127061.
- Yang, B. Y., I. Markevych, M. S. Bloom, J. Heinrich, Y. Guo, L. Morawska, S. C. Dharmage, et al. 2019. “Community Greenness, Blood Pressure, and Hypertension in Urban Dwellers: The 33 Communities Chinese Health Study.” Environment International 126:727–734. https://doi.org/10.1016/j.envint.2019.02.068.
- Yang, P. P. J., S. Y. Putra, and W. Li. 2007. “Viewsphere: A GIS-Based 3D Visibility Analysis for Urban Design Evaluation.” Environment and Planning B: Planning and Design 34 (6): 971–992. https://doi.org/10.1068/b32142.
- Yang, J., H. Rong, Y. Kang, F. Zhang, and A. Chegut. 2021b. “The Financial Impact of Street-level Greenery on New York Commercial Buildings.” Landscape and Urban Planning 214:104162. https://doi.org/10.1016/j.landurbplan.2021.104162.
- Yang, H., Q. Zhang, M. Helbich, Y. Lu, D. He, D. Ettema, and L. Chen. 2022. “Examining Non-linear Associations between Built Environments Around Workplace and Adults’ Walking Behaviour in Shanghai, China.” Transportation Research Part A: Policy and Practice 155:234–246. https://doi.org/10.1016/j.tra.2021.11.017.
- Yang, B. Y., T. Zhao, L. X. Hu, M. H. E. M. Browning, J. Heinrich, S. C. Dharmage, B. Jalaludin, et al. 2021. “Greenspace and Human Health: An Umbrella Review.” The Innovation 2 (4): 100164. https://doi.org/10.1016/j.xinn.2021.100164.
- Yao, Y., C. Xu, H. Yin, L. Shao, and R. Wang. 2022. “More Visible Greenspace, Stronger Heart? Evidence from Ischaemic Heart Disease Emergency Department Visits by Middle-Aged and Older Adults in Hubei, China.” Landscape and Urban Planning 224:104444. https://doi.org/10.1016/j.landurbplan.2022.104444.
- Ye, Y., D. Richards, Y. Lu, X. Song, Y. Zhuang, W. Zeng, and T. Zhong. 2019. “Measuring Daily Accessed Street Greenery: A Human-Scale Approach for Informing Better Urban Planning Practices.” Landscape and Urban Planning 191:103434. https://doi.org/10.1016/j.landurbplan.2018.08.028.
- Ye, Y., H. Xie, J. Fang, H. Jiang, and D. Wang. 2019a. “Daily Accessed Street Greenery and Housing Price: Measuring Economic Performance of Human-scale Streetscapes via New Urban Data.” Sustainability 11:1741. https://doi.org/10.3390/su11061741.
- Yu, H., L. W. Hu, Y. Zhou, Z. Qian, M. Schootman, M. H. LeBaige, Y. Zhou, et al. 2021. “Association between Eye-level Greenness and Lung Function in Urban Chinese Children.” Environmental Research 202:111641. https://doi.org/10.1016/j.envres.2021.111641.
- Yu, Y., Y. Jiang, N. Qiu, H. Guo, X. Han, and Y. Guo. 2022. “Exploring Built Environment Factors on E-bike Travel Behavior in Urban China: A Case Study of Jinan.” Frontiers in Public Health 10. https://doi.org/10.3389/FPUBH.2022.1013421.
- Yu, S., B. Yu, W. Song, B. Wu, J. Zhou, Y. Huang, J. Wu, F. Zhao, and W. Mao. 2016. “View-based Greenery: A Three-Dimensional Assessment of City Buildings’ Green Visibility Using Floor Green View Index.” Landscape and Urban Planning 152:13–26. https://doi.org/10.1016/j.landurbplan.2016.04.004.
- Yuan, Y., F. Huang, F. Lin, Pengyi Zhu, and Pengli Zhu. 2021. “Green Space Exposure on Mortality and Cardiovascular Outcomes in Older Adults: A Systematic Review and Meta-analysis of Observational Studies.” Aging Clinical and Experimental Research 33 (7): 1783–1797. https://doi.org/10.1007/s40520-020-01710-0.
- Zang, P., H. Qiu, K. Chen, H. Zhang, F. Xian, J. Mi, H. Guo, Y. Qiu, and K. Liao. 2023. “The Built Environment’s Nonlinear Effects on the Elderly’s Propensity to Walk.” Researchgate.net. https://doi.org/10.3389/fevo.2023.1103140.
- Zhang, Y., N. Chen, W. Du, Y. Li, and X. Zheng. 2021. “Multi-source Sensor Based Urban Habitat and Resident Health Sensing: A Case Study of Wuhan, China.” Building and Environment 198:107883. https://doi.org/10.1016/j.buildenv.2021.107883.
- Zhang, Y., and R. Dong. 2018. “Impacts of Street-Visible Greenery on Housing Prices: Evidence from a Hedonic Price Model and a Massive Street View Image Dataset in Beijing.” ISPRS International Journal of Geo-Information 7:104. https://doi.org/10.3390/ijgi7030104.
- Zhang, J., and A. Hu. 2022. “Analyzing Green View Index and Green View Index Best Path Using Google Street View and Deep Learning.” Journal of Computational Design and Engineering 9:2010–2023. https://doi.org/10.1093/JCDE/QWAC102.
- Zhang, T., B. Huang, Y. Yan, Y. Lin, H. Wong, S. Y. S. Wong, and R. Y. N. Chung. 2023. “Street-View and Traditional Greenness Metrics with Adults' Sitting Time in High-Density Living in Hong Kong: Comparing Associations, Air Pollution and Noise Roles, and Population Heterogeneity.” Science of The Total Environment 870: 161778.
- Zhang, T., M. Su, B. Hong, C. Wang, and K. Li. 2021a. “Interaction of Emotional Regulation and Outdoor Thermal Perception: A Pilot Study in a Cold Region of China.” Building and Environment 198:107870. https://doi.org/10.1016/j.buildenv.2021.107870.
- Zhang, L., P. Y. Tan, and D. Richards. 2021b. “Relative Importance of Quantitative and Qualitative Aspects of Urban Green Spaces in Promoting Health.” Landscape and Urban Planning 213:104131. https://doi.org/10.1016/j.landurbplan.2021.104131.
- Zhao, H., J. Shi, X. Qi, X. Wang, and J. Jia. 2017. “Pyramid Scene Parsing Network.” In Proceedings of the IEEE conference on computer vision and pattern recognition, 2881–2890.
- Zheng, S., J. Wu, M. E. Kahn, and Y. Deng. 2012. “The Nascent Market for “Green” Real Estate in Beijing.” European Economic Review 56 (5): 974–984. https://doi.org/10.1016/j.euroecorev.2012.02.012.
- Zhu, J., L. Qiu, Y. Su, Q. Guo, T. Hu, H. Bao, J. Luo, et al. 2022. “Disentangling the Effects of the Surrounding Environment on Street-side Greenery: Evidence from Hangzhou.” Ecological Indicators 143:109153. https://doi.org/10.1016/j.ecolind.2022.109153.