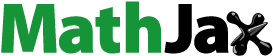
ABSTRACT
The terrestrial vegetation GPP of Qinghai Province is an important variable that characterizes the carbon cycling pattern. However, there is still a lack of a high-resolution GPP dataset for Qinghai Province. To address this issue, we processed all Landsat images of Qinghai from 1987 to 2021 using the GEE, and we combined multi-source auxiliary data to estimate GPP using the revised EC-LUE model. We compared our GPP dataset with flux observations to verify its accuracy. The results showed that our GPP dataset had a high correlation with the flux tower observations, with correlation coefficients of 0.984 at CF-AM site and 0.976 at CN-Ha2 site, respectively, and each site had an RMSE of and 12.986
, respectively. There are different deviations between our GPP dataset and the mainstream GPP datasets in various vegetation types, with the average correlation coefficient ranging from 0.431 to 0.943. By comparing with the flux observations and the related analysis, we demonstrated that our GPP dataset features better accuracy, higher spatial resolution, and more temporal coverage than mainstream GPP datasets. This study offers the first long-term high-resolution GPP dataset for Qinghai Province, and we believe that this dataset has important implications for ecological management and climate research.
KEYWORDS:
1. Introduction
Gross Primary Productivity (GPP) is the total amount of carbon dioxide () fixed by green plants through photosynthesis (Beer et al. Citation2010). It drives many ecosystem processes and plays a crucial role in global carbon balance (Wild et al. Citation2022). Estimating its spatiotemporal variability is essential for assessing the carbon storage capacity of terrestrial ecosystems, predicting climate change, and studying carbon balance mechanisms (Chen et al. Citation2017; Ye et al. Citation2021; Zhang et al. Citation2021).
The Qinghai Plateau, also known as Qinghai Province, covers approximately 30% of the Qinghai-Tibetan Plateau and has a wide variety of natural resources, such as grasslands, forests, and wetlands. It is a sensitive area to global climate change, as its unique geographical environment influences and is influenced by the climate and environment of surrounding areas, such as the westerlies and Asian monsoons (Yang et al. Citation2014). Accurately quantifying the vegetation GPP of Qinghai Province and elucidating its spatiotemporal patterns are therefore critical to unveiling carbon cycling processes in this climatically sensitive region. This will also further our understanding of how vegetation productivity responds to climate change, facilitating ecological conservation and sustainable development across the plateau (Jingbin et al. Citation2020; Lin, Chang, and Feng Citation2021; Ma et al. Citation2018).
The eddy covariance (EC) technique is a direct method to monitor the carbon fluxes between the atmosphere and land surface, but it has some limitations. First, the measured values only reflect the carbon flux state within a small area around the EC towers, which ranges from a few hundred meters to several kilometers. EC measurements require a flat terrain and homogeneous vegetation within the footprint. Giannico et al. (Citation2018) suggested that a high level of landscape heterogeneity would affect the accuracy of the flux measurements. Second, the number of flux tower sites in Qinghai Province is very limited. For example, only two sites in Qinghai Province are included in the FLUXNET2015 (Pastorello et al. Citation2020) dataset. The sparse distribution of flux tower sites leads to significant uncertainties when using the EC technique to monitor the spatial distribution and temporal trends of GPP. Therefore, researchers have applied remote sensing technology to estimate GPP by utilizing large-scale, spatially continuous land surface information (Lin, Li, and Liu Citation2018). Various GPP datasets have been developed to estimate global or regional GPP at different spatiotemporal scales using different data and models. For instance, Jiang et al. (Citation2021) used a machine learning model and a reflectance fill and filter algorithm with MODIS data to produce a higher resolution (250 m, daily) GPP dataset for the contiguous United States, which improved the temporal and spatial resolution of existing MODIS GPP products. He et al. (Citation2022) applied the Penman-Monteith-Leuning Version 2 water-carbon coupling model to estimate daily GPP and evapotranspiration data for China from 2000 to 2020, which provided valuable references for hydrological and ecological research. Zhang et al. (Citation2017) employed an improved VPM model with MODIS and NCEP reanalysis data to estimate global GPP from 2000 to 2016 at an 8-day and 500 m spatiotemporal resolution. However, these datasets generally suffer from the trade-off between temporal and spatial resolution. Most of the existing global or regional scale GPP datasets have low spatial resolution (He et al. Citation2022; Jiang et al. Citation2021; Ma et al. Citation2018; Wild et al. Citation2022; Zhang et al. Citation2017). This means that the GPP estimation values in regions with strong heterogeneity at local scales, such as croplands, wetlands, pastures, and regions with complex vegetation composition, will have significant statistical uncertainty and error (Zheng et al. Citation2018). Moreover, the coarse spatial resolution restricts the application of these datasets in these regions, as they cannot provide sufficient spatial information to effectively differentiate between different land cover boundaries.
As the longest-running medium to high-resolution earth observation satellite (Wulder et al. Citation2022), Landsat has enormous potential for estimating GPP at regional and global scales. Many scholars have used Landsat data to estimate GPP. For example, Gitelson et al. (Citation2012) found that the performance of MODIS GPP products in cropland areas was very poor due to the low spatial resolution and the lack of biome-specific parameters. Therefore, they used Landsat data to obtain some vegetation indices that represent chlorophyll content, and used these indices to estimate GPP. The results showed that the accuracy of Landsat-based GPP estimation was significantly improved, indicating that Landsat data can effectively handle the GPP estimation problem in areas with high heterogeneity. Knox et al. (Citation2017) also used Landsat data to estimate wetland GPP, showing that Landsat can assess the carbon exchange status at a low cost. Wolanin et al. (Citation2019) used Landsat 8 and Sentinel 2 data to estimate the GPP of C3 crops based on the radiative transfer model and machine learning algorithm. Even without using any local information corresponding to the flux sites, they were able to obtain good estimation results, demonstrating the potential of medium-to-high resolution earth observation satellites such as Landsat in mapping global crop productivity. These works have made great contributions to the field of productivity estimation at that time. However, they only applied Landsat data within a relatively small spatial range, mainly for model improvement or as auxiliary data. Applying Landsat data to obtain large-scale, spatially continuous GPP still faces many challenges, such as limited available observations due to cloud cover and the satellite's long repeat cycle, and large storage space and long processing times due to the high spatial resolution (30 m) of the data. With the emergence of Google Earth Engine (GEE), researchers have the possibility to address the aforementioned problems. He et al. (Citation2018) used GEE in conjunction with time-series reflectance information from Landsat and MODIS to establish a pixel-level linear relationship between MODIS and Landsat NDVI, successfully estimating high-accuracy GPP data at a regional scale with an 8-day interval and 30 m spatial resolution. Robinson et al. (Citation2018) also used Landsat data to produce a high-resolution GPP dataset covering the continental United States (CONUS). This is an innovative dataset that provides us with more spatial details of vegetation productivity on a large scale. However, this dataset is limited to the CONUS and does not cover Qinghai Province, which urgently needs vegetation productivity data. Therefore, we used GEE and a representative light use efficiency model revised EC-LUE (Yuan et al. Citation2007) to estimate the high spatiotemporal resolution GPP of Qinghai Province from 1987 to 2021, so that we can meet the demand for long-term and fine-resolution vegetation productivity monitoring in this region.
In this study, we leveraged Google Earth Engine to process the entire Landsat archive from 1987 to 2021 for Qinghai Province. The processed long-term Landsat-derived NDVI time series was used as input data, along with ERA5-Land reanalysis and auxiliary data. We employed the revised EC-LUE model to estimate vegetation GPP across Qinghai Province from 1987 to 2021. The main contributions of this work are: (1) We produced the first Landsat-based GPP dataset at 16-day temporal resolution and 30 m spatial resolution with a long time series (1987–2021) for Qinghai Province; and (2) We characterized the spatiotemporal variations in vegetation productivity across Qinghai Province based on the Landsat-derived GPP estimates.
2. Study area and data
2.1. Study area
Qinghai Province is located on the northeast Qinghai-Tibet Plateau, with an area of 724,000 . It spans from 89
E to 104
E and from 31
N to 40
N, and it has an average altitude of about 4000 m. Qinghai Province has a typical plateau continental climate, with long, cold winters and short, cool summers. The annual precipitation is low and unevenly distributed, mainly concentrated in summer and averaging between 156.6 and 669.1 mm. Temperatures range from 0.3
C to 7.2
C annually, with a large diurnal difference. Due to the influence of the Indian monsoon, Qinghai Province boasts world's most extensive and highest alpine meadows and grasslands (Li et al. Citation2016). The Province's unique alpine vegetation system, along with its vast glaciers and perennial snow cover, makes it an important ecological barrier and climate regulator in China ().
2.2. Data source
The datasets used for calculating GPP are presented in . For detailed information about each dataset, please refer to Sections 2.2.1 to 2.2.4.
Table 1. Input datasets used for generating GPP.
2.2.1. Time-series landsat data
We used all Landsat-5, Landsat-7, and Landsat-8 Collection 2 Level 2 surface reflectance data in Qinghai province from 1987 to 2021 in GEE to estimate the FPAR parameter required for generating GPP. The temporal and spatial resolution of Landsat are 16-day and 30 m, respectively. All Landsat images have been orthorectified and atmospherically corrected by the USGS. The surface reflectance data of Landsat 5, 7, and 8 were generated by the LEDAPS and LaSRC algorithms (Masek et al. Citation2006; Vermote et al. Citation2016), respectively. According to the information provided by the Landsat Science Product Guide, we used a scale of 0.0000275 and an offset of to convert the pixel values of surface reflectance products to actual surface reflectance. All pixels contaminated by cloud and cloud shadow were masked out using the QA band generated by the CFMASK algorithm (Foga et al. Citation2017). Since there are differences between different sensors, we used a conversion function to harmonize all Landsat data. Section 3.1 provides the details of this conversion function.
2.2.2. Meteorological and elevation data
The meteorological variables used in this study were obtained from the ERA5-Land daily reanalysis dataset in GEE, which provides high spatial resolution (0.1) land information. The 0.1-degree spatial resolution daily 2m air temperature (
), 2 m dew point temperature (
), and shortwave radiation (Srad) were used to calculate the temperature and moisture stress factors on maximum light use efficiency of vegetation in the surrounding environment. The Shuttle Radar Topography Mission (SRTM) digital elevation Version 4 data with a spatial resolution of 90 m was resampled to 0.1-degree resolution using bilinear interpolation. The resampled elevation data, along with
and
, was used to calculate the vapor pressure deficit (VPD). The specific calculation process can be seen in 3.2 Meteorological Data Processing.
2.2.3. Land cover and 
data
The land cover data used in this study is the 30 m spatial resolution land cover classification dataset (CLCD) developed by Yang and Huang (Citation2021). This dataset is the first high-resolution annual land cover dataset in China developed using machine learning and cloud computing platforms. Moreover, it provides higher overall accuracy than commonly used classification datasets such as MCD12Q1 and GlobeLand30.
The carbon dioxide() concentration data sourced from the Earth System Research Laboratory (ERSL) (https://gml.noaa.gov/ccgg/trends/global.html) was used to estimate the
stress factor on maximum light use efficiency.
2.2.4. Validation and reference data
This study collected GPP flux measurements from four eddy covariance sites obtained from the FLUXNET2015 dataset (https://fluxnet.org/data/fluxnet2015-dataset/) and ChinaFlux (http://www.chinaflux.org). These four sites are CN-Ha2 and CN-HaM from the FLUXNET2015 dataset, and CF-SHR and CF-AM from ChinaFlux. Table S1 provides detailed information about these sites. These datasets have been widely used for vegetation ecophysiological mechanism studies and various model training and validation (Wang et al. Citation2021; Zhou et al. Citation2021). Creators of the FLUXNET2015 dataset used unified processing method and quality control to obtain the data, which ensured the consistency and comparability of the data from different sites (Pastorello et al. Citation2020). Contributors of ChinaFlux also used standardized quality control and processing methods to ensure high confidence of the flux observation data (Chang et al. Citation2023). In the FLUXNET2015 site data, the average of the day-time (GPP_DT_VUT_REF) and night-time (GPP_NT_VUT_REF) partition methods generated GPP were selected as the flux GPP. In ChinaFlux, we used the difference between daily ecosystem respiration (RE) and net ecosystem exchange (NEE) to calculate GPP (Chang et al. Citation2023), as shown in Equation (Equation1(1)
(1) ). After calculating the 16-day cumulative values of the flux data at each site, we used all the data from CN-HaM and CF-SHR sites as training data, and the data from CN-Ha2 and CF-AM sites as independent validation data, to train and validate the model.
(1)
(1) To evaluate the consistency and difference between the GPP estimated by this study and the existing GPP datasets, we compared our estimation to three widely used GPP datasets: the MOD17A2H (version 006) dataset, the PML-V2 GPP dataset, and the GLASS-GPP dataset. The MOD17A2H dataset is a cumulative 8-day 500 m GPP dataset based on the light use efficiency model proposed by Monteith (Citation1972). It utilizes FPAR dataset (MOD15A2H), GMAO/NASA dataset, and biome look-up table to achieve near-real-time estimation of global GPP. This dataset is the most widely used dataset (Zhang et al. Citation2017). The PML-V2 GPP dataset is also an 8-day 500 m GPP dataset that used a water-carbon coupled model (referred to as PML-GPP). This dataset has been shown to have higher accuracy than other mainstream GPP products through its comparisons with 95 global flux sites. The GLASS (Global Land Surface Satellite) dataset (http://www.glass.umd.edu/GPP/AVHRR) is a global long-term land surface multi-parameter dataset. The dataset contains 8-day 0.05
GPP products (referred to as GLASS-GPP). With the above three datasets were selected as reference datasets, we accumulated the 8-day GPP estimates from these datasets over 16-day intervals to match the temporal resolution of the GPP estimates from this study. It should be noted that although these three GPP datasets are widely used, there are still some potential errors in them. Existing studies have shown that the MODIS GPP product has errors mainly caused by meteorological data and vegetation parameter data (Heinsch et al. Citation2006; Zhang et al. Citation2019), and the GLASS-GPP product, which also uses a light use efficiency model, is likely to have the same errors. The values of different versions of MODIS GPP products show significant inconsistencies in some regions, possibly due to the use of different algorithms (Peter and Messina Citation2019). The PML-GPP product is also affected by the MCD12 land cover data it uses, which increases the uncertainty of its model parameters and thus affects its estimation accuracy (Zhang et al. Citation2019). Therefore, these GPP datasets can only be used as references for our estimated GPP data, and the flux observation GPP will be used to validate our data.
3. Methods
Generating accurate high-resolution GPP for the entire Qinghai Province faces two main challenges: (1) how to generate large-area high spatial-temporal resolution NDVI data under adverse factors such as cloud cover, which causes a large amount of missing data; and (2) how to accurately estimate the downward regulation scalars of temperature, moisture, and on light use efficiency (LUE). To address these challenges, we developed an effective framework for estimating GPP accurately. shows the specific framework for calculating GPP. The first step is to generate high resolution NDVI data, which involves the following procedures: calculating NDVI from Landsat surface reflectance data, masking out clouds, shadows, and snow using the QA band in the Landsat surface reflectance products, synthesizing 16-day NDVI data, and filling and reconstructing NDVI data. The second step is to use the reconstructed NDVI data and meteorological data as inputs for the revised EC-LUE model to obtain GPP data. The third step is to compare and validate the estimated GPP with other GPP datasets and flux tower observations.
3.1. NDVI filling and reconstruction
The vegetation index is a key input variable in the revised EC-LUE model, which reflects the vegetation's ability to absorb solar radiation through a linear transformation. We use Landsat surface reflectance data to calculate NDVI, which is defined as follows:
(2)
(2) where
represents the surface reflectance in the near-infrared band of the corresponding sensor of Landsat satellites, and
represents the surface reflectance in the red band of the corresponding sensor of Landsat satellites.
To ensure the consistency of the time series NDVI, we apply a simple transformation function (Roy et al. Citation2016) to adjust the performance differences among Landsat-5 TM, Landsat-7 ETM+, and Landsat-8 OLI sensors. This function is originally designed in consideration of differences between Landsat-7 ETM+ and Landsat-8 OLI sensors. Since Landsat-5 TM and Landsat-7 ETM+ sensors have similar performance (Claverie et al. Citation2015), we extend the function to Landsat-5 TM sensor as well. The specific transformation function is as follows:
(3)
(3) where
and
are the NDVI values calculated from Landsat-8 OLI and Landsat-5 TM or Landsat-7 ETM+ sensor data, respectively. One challenge we encountered was that the low temporal resolution of Landsat (about 16-day) and the atmospheric conditions limit the availability of Landsat-NDVI observations. To eliminate the effects of clouds and other factors on NDVI data, we first use the ‘QA_PIXEL’ band of Landsat surface reflectance products to evaluate the pixel quality and mask out clouds, shadows, and snow in the images. Then, we use the maximum value composite method to synthesize 16-day NDVI data for Qinghai Province starting from January 1st of each year. For the missing pixels caused by the masking process, we use the filling method proposed by Robinson et al. (Citation2017) to select ‘clear’ pixels from the past five years based on the ‘QA_PIXEL’ band, and we use the median composite method to synthesize them. We choose a time scale of five years because the time span of the El Nio-Southern Oscillation (ENSO) is about 5 years, and ENSO has a huge impact on the biosphere. Selecting NDVI filling values beyond five years may introduce more uncertainty to the NDVI reconstruction (Running and Zhao Citation2021). To further smooth the filled NDVI data and reduce the noise, we use the harmonic regression model (Wilson, Knight, and McRoberts Citation2018) to decompose the NDVI time series into a limited number of harmonic terms to model NDVI. For further understanding of this method, please consult the supporting material.
3.2. Meteorological data processing
To match the temporal resolution of other data, we computed the 16-day average of daily and
in ERA5-Land, and the 16-day sum of Srad. We resampled all meteorological data to a spatial resolution of 30 m using bilinear interpolation. Since ERA5-Land does not include VPD data, we calculated VPD using the following formula (Fang et al. Citation2022):
(4)
(4)
(5)
(5)
(6)
(6) where SVP and AVP are the saturated and actual vapor pressure in hPa, respectively.
and
are the dew point and air temperature in
C, respectively.
(7)
(7)
(8)
(8) where
represents the mean sea level air pressure (1013.25 hPa), and Z represents the elevation (m).
3.3. Revised EC-LUE model
This paper uses the revised EC-LUE model to estimate GPP. However, our implementation of EC-LUE has one major difference compared with the original model, when considering the constraints of temperature and moisture on the maximum light use efficiency of vegetation, we did not follow the the original model's method of directly taking the minimum value of the temperature and moisture scalars. Instead, we assumed that temperature and moisture jointly affect the maximum light use efficiency of vegetation, hence we multiplied the temperature scalar with the moisture scalar when computing LUE of vegetation. This is because Qinghai Province is located on the Qinghai-Tibet Plateau, where the vegetation growing season is short, and the temperature is low in spring and autumn. If the original model's calculation framework is used, it would easily result in zero values in certain periods of estimated GPP, in particular spring and autumn. However, by observing the temporal variation of GPP at flux sites in Qinghai Province, we found that the GPP in spring and autumn account for about 10% of the annual GPP. Therefore, we made some modifications to the model to better adapt to the local conditions.
(9)
(9) where FPAR is the fraction of absorbed photosynthetically active radiation, which is derived from the reconstructed NDVI; PAR is the photosynthetically active radiation (
), which is obtained by multiplying Srad by 0.45;
represents the maximum light use efficiency of vegetation (
).
,
, and
are the temperature, moisture, and carbon dioxide downward regulation scalars for
, which range from 0 to 1.
(10)
(10)
(11)
(11)
(12)
(12)
(13)
(13) where NDVI is the normalized difference vegetation index obtained from Landsat data; T represents the average air temperature (
C);
,
, and
are the minimum, maximum, and optimum air temperature for vegetation photosynthesis, respectively. The higher average elevation and plateau continental climate on the Qinghai Plateau may lead to significant differences in the values of
,
, and
compared to other regions. Therefore, following the settings in previous studies (Huang et al. Citation2019; Wang et al. Citation2012), we set
,
, and
to 0
C, 35
C, and 13
C, respectively.
represents the half-saturation coefficient of the vapor pressure deficit (kPa).
is the intercellular carbon dioxide concentration (ppm); φ is the compensation point of carbon dioxide in the absence of dark respiration, which can be calculated using the following formula (Bi et al. Citation2022).
(14)
(14)
(15)
(15)
(16)
(16)
(17)
(17)
(18)
(18)
(19)
(19)
(20)
(20) where
is the atmospheric
concentration (ppm); χ is the ratio of leaf-internal to ambient
; K is the Michaelis-Menten coefficient of Rubisco;
represents the water viscosity at 25
C (0.8903);
and
are the Michaelis-Menten constants of Rubisco for
and
, and
represents the partial pressure of
(21 kPa). R is the molar gas constant (8.314
). To optimize the maximum light use efficiency and
in the model, we used the Markov Chain-Monte Carlo method, which is widely applied to light use efficiency model parameter estimation. Based on previous experience (Bagnara et al. Citation2015), we set the prior distribution of the parameters as uniform distribution, and set the range of maximum light use efficiency and
to 0–10 (since in most literature, these two parameters are set within 0–10). We chose the Metropolis-Hastings algorithm as the MCMC sampler (see Yuan et al. (Citation2012) for a more detailed description). We used training data (CN-HaM and CF-SHR site data) corresponding to grassland and shrub vegetation cover types, so we only optimized the model parameters for these two vegetation types. We set the model parameters for other vegetation types based on the article by Yuan et al. (Citation2019). The classification system used in the CLCD dataset is different from that used in the article by Yuan et al. (Citation2019). In the CLCD dataset, vegetation is classified as cropland, forest, shrub, grassland, and wetland, while in the article by Yuan et al. (Citation2019), forests are classified as deciduous broadleaf forest, evergreen needleleaf forest, evergreen broadleaf forest, and mixed forest. We set the parameter values for these four types of forests to the average value for forests. The specific parameter values are shown in .
3.4. Data validation and comparison
To verify the accuracy of the estimated GPP in this study, we calculated the root mean square error (RMSE), bias, and correlation coefficient (r) between the estimated GPP and the flux tower observed GPP. Similarly, we computed the RMSE, bias, and r between PML-GPP, GLASS-GPP, and MOD17A2H GPP and flux tower observed GPP for comparative analysis with our estimation. We also calculated the statistical metrics between the estimated GPP and the existing GPP products to evaluate their consistency. To match the different spatial resolutions of the GPP data, we resampled the high spatial resolution images using bilinear interpolation to the low spatial resolution before calculating these statistical metrics. When compared with PML-GPP and MOD17A2H, we resampled the estimated 30 m GPP to 500 m, and when compared with GLASS-GPP, we resampled the estimated 30 m GPP to 0.05 (about 5566 m). The formulas for calculating these three statistical metrics are shown below:
(21)
(21) where
represents the estimated GPP; ρ represents the flux tower observed GPP;
represents the covariance between the estimated GPP and the flux observation GPP;
and
are the variance of the estimated GPP and the flux tower observed GPP, respectively.
(22)
(22)
(23)
(23) where m represents the total number of observations; i represents the sequence number of the observation;
and
respectively represent the estimated GPP and the observed GPP of the i-th sample point.
Table 2. Revised EC-LUE model parameters for different vegetation types.
4. Results
4.1. GPP comparison and validation
4.1.1. Validation against fluxnet GPP
We evaluated the accuracy of the estimated GPP in this study (referred to as ) by comparing it with flux tower observations and MOD17A2H, PML-GPP, and GLASS-GPP datasets. shows that most of the
data points at a 16-day time step are close to the 1:1 line with the flux tower observations, indicating a high level of agreement between the two. At the CF-AM site,
has the second highest r (0.984) and slightly higher RMSE (11.960
) than PML-GPP, followed by MOD17A2H and GLASS-GPP, which have similar r values (around 0.98) but higher RMSE values. At the CN-Ha2 site,
has a slightly lower r (0.976) than the other three datasets. The RMSE is 12.986
, higher than PML-GPP and GLASS-GPP, but lower than the MOD17A2H dataset. Overall,
has the second highest correlation coefficient (0.978) and almost the lowest RMSE (12.312
). illustrates the time series data of remote sensing GPP datasets and flux tower observations. From the figure, we can see that
exhibits a consistent seasonal variation with the flux tower observations. Except for MOD17A2H, which underestimates GPP at both sites, there are relatively small differences among the different datasets. Overall,
demonstrates similar accuracy to the other datasets. The high correlation coefficient and low RMSE indicate a small deviation between
and the flux tower observations.
4.1.2. Comparison of different GPP datasets
The limited number of flux tower sites in Qinghai Province introduces some uncertainties in validation. Therefore, we also compared with other datasets for different vegetation types to quantitatively assess the correlation and differences between
and the reference datasets (). We found that
exhibits strong linear relationships with the other three datasets in most vegetation types. Specifically, in grassland types,
has the highest average r (0.943) with PML-GPP, followed by MOD17A2H (0.891) and GLASS-GPP (0.868). The r values are concentrated, indicating high similarity between
-derived grassland productivity and the other datasets for the period of 2001-2018, with low RMSE and Bias suggesting minimal deviation. Overall, in the Shrub type,
has lower r values with the other datasets, particularly with the MOD17A2H dataset, which has an average r of only 0.431. Both RMSE and Bias are higher for the MOD17A2H dataset compared to the PML-GPP and GLASS-GPP datasets. Among all reference datasets,
shows the highest correlation and the least difference with PML-GPP. Specifically, the r values between PML-GPP and
range from 0.671 to 0.943 across different vegetation types, all higher than those of MOD17A2H and GLASS-GPP datasets. The RMSE and Bias range from 123.750 to 281.739
and −102.935 to 121.518
, respectively, which are also the lowest compared to the other two reference datasets. Overall,
exhibits high consistency with flux tower observations and reference datasets, indicating its high accuracy and suitability for applications.
Figure 5. Statistical metrics of the annual cumulative compared to PML-GPP (PML), MOD17A2H (MOD), and GLASS-GPP (GLASS) from 2001 to 2018. These statistics were calculated based on 10,000 randomly generated sample points within each vegetation type.

We also compared with other remote sensing GPP datasets for different regions and seasons in Qinghai Province. As shown in ,
maintains consistent quarterly variations with the reference datasets in most regions, particularly in the northeastern, eastern, and southeastern parts of Qinghai Province. In the western part of the Sanjiangyuan region, where precipitation is low and vegetation is sparse, GPP values are also low, resulting in differences between
and the reference datasets in that area. Overall,
shows high correlation with PML-GLASS, MOD17A2H, and GLASS-GPP, with average r values of 0.879, 0.901 and 0.839, respectively. Due to the differences in input data, model structures, and model parameterization methods, there may be variations in the magnitude of GPP estimated by different models (Zheng et al. Citation2018). Therefore, a better approach is to compare the interannual variations of different GPP anomalies (). We found that all GPP datasets exhibit consistent interannual trends during the period from 1987 to 2021. From this figure, we can observe that each dataset shows a continuous increase in GPP in Qinghai.
4.2. Spatial and temporal distribution patterns of GPP in qinghai province
We analyzed the seasonal variations of in Qinghai Province. As shown in ,
exhibits strong seasonal fluctuations. During the summer months (June to August), which are characterized by suitable temperature and rainfall for vegetation growth, GPP reaches its peak, accounting for the highest proportion (87.11%) of the annual value. In contrast, during the winter months (December to February), characterized by extremely low temperature and rainfall, vegetation photosynthetic capacity is severely weakened, resulting in near-zero GPP. GPP values in spring and autumn are similar, accounting for 6.14% and 6.75% of the annual value, respectively. From 1987 to 2021, the average annual GPP in Qinghai Province was 424.179
. Over the course of 35 years, the annual total GPP shows a decreasing trend during the years of 1987 and 1992, followed by gradual increases in 1993–2005, 2006–2015, and a rapid increase after 2015. The peak GPP value was observed in 2020, reaching 537.64
. Overall, GPP exhibited an increasing trend with a growth rate of 4.42
(
).
Figure 8. Distribution image of 16-day GPP in Qinghai Province. Due to the varying number of days in each year from 1987 to 2021, an index is used to represent the regional average cumulative GPP within each 16-day period of the year in Qinghai Province. For example, in the year 1987, a grid with an index of 1 represents the cumulative GPP regional average from January 1, 1987, to January 16, 1987, in Qinghai Province.

As illustrates, GPP in Qinghai Province exhibits a clear spatial heterogeneity. The eastern and southeastern regions of Qinghai Lake and the southern region of the Sanjiangyuan area have high GPP values. In the northeastern part of Qinghai Province, such as the Chaidamu Desert region, and the western part of the Sanjiangyuan area, GPP is extremely low due to factors such as scarce precipitation and severe soil salinization. Generally, all GPP datasets decreases gradually from south to north and increases from west to east. Regarding the temporal trend of GPP changes, GPP in Qinghai Province showed a general increase from 1987 to 2021, where 90.47% of the vegetation areas feature increasing GPP. The areas with the most pronounced GPP increase are near Qinghai Lake-Qilian Mountains, the agricultural and pastoral production areas in the western part of Qinghai Province, and the southern and southeastern regions of the Sanjiangyuan area, with a rate of up to 20 . However, some areas in the central and southern parts of the Sanjiangyuan region exhibited a slight decreasing trend in GPP. The increasing trend of GPP from our observation is consistent with previous studies (Shen et al. Citation2015; Wei et al. Citation2022; Zhong et al. Citation2019), indicating that vegetation productivity in Qinghai Province, located on the Qinghai-Tibet Plateau, has been continuously increasing due to the warming and humidification of the plateau and the prolongation of the vegetation growing season.
5. Discussion
5.1. Advancements and uncertainties
In this paper, we developed the first long-term, high-resolution 16-day GPP dataset, named , so that we can aid Qinghai Province in addressing the need for accurate monitoring of vegetation productivity in the context of climate change. Compared to reference GPP datasets,
featured the highest correlation and smaller deviations with flux tower observations. Moreover,
provided vegetation productivity information at a higher spatial resolution, and exhibited a high level of spatiotemporal consistency with the reference datasets. The higher spatial resolution of
allows us to observe vegetation changes at finer scales, as (e) illustrates, the values of
are significantly higher than those of other datasets, indicating that
can accurately capture the productivity changes of small patches of green vegetation within the vast Mugetan sandy land. In the data processing procedure, we employed transformation functions, data filling, and smoothing algorithms to adjust and reconstruct the time-series NDVI, accounting for the effects of atmospheric conditions, aerosols, and sensor variations on Landsat imagery. We also integrated the effects of hydro-thermal conditions and atmospheric
concentration on vegetation light-use efficiency by using the revised EC-LUE model, achieving accurate estimation of GPP in Qinghai.
Figure 10. Annual GPP of Mugetan sandy land in different spatial resolutions in 2017: (a) 0.05; (b) 500 m; (c) 500 m; (d) 30 m. (e) represents the 16-day GPP time series data at the black dots in (a–d).

Despite the significant improvement in accuracy and the provision of long-term consistent high-resolution GPP observations, still has some limitations. Firstly, the input data used for GPP generation has inherent uncertainty. For example, Landsat NDVI can mitigate the effects of topography, atmosphere, and BRDF, but there are some known issues with Landsat products in the CFMASK algorithm used in this product struggles to identify thin clouds, and the detection of cloud shadows has not been fully validated (Foga et al. Citation2017), leading to some uncertainties in the time series NDVI. To minimize these errors, we employed data filling and smoothing algorithms. Secondly, the 30 m resolution landcover used in this paper is an annual data, but due to insufficient Landsat data, there was only one image before 1990, which nominally belongs to 1985. Therefore, we had to use the same landcover data for producing the GPP for 1987–1989, which may result in inaccurate classification information. This kind of error may also propagate to the GPP through the biome-specfic parameters in the revised EC-LUE model. Thirdly, the structure of revised EC-LUE model only considers the effects of hydro-thermal conditions and atmospheric
concentration on vegetation photosynthesis. Other factors affecting photosynthesis, such as soil nitrogen, potassium content and other soil properties, can be added to the model. However, due to the difficulty of obtaining soil properties and their mismatches of temporal coverage with the
, this limitation can only be improved by conducting research.
5.2. Future and benefits
In this paper, we successfully used tens of thousands of Landsat images over more than 30 years to map high-resolution vegetation productivity in Qinghai Province, which demonstrates the reliability of our proposed GPP processing workflow. Moreover, our work also provides useful reference for future high-resolution vegetation productivity estimation at continental and even global scale. Currently, there are many methods to monitor vegetation change information at high resolution, such as the widely used spatiotemporal fusion algorithms (Gao et al. Citation2006; Zhu et al. Citation2010) and the filtering methods with gap-filling and fusion (Cao et al. Citation2022; Chen et al. Citation2021). However, these methods have some drawbacks that are hard to overcome. First of all, these methods usually require fusing high spatial and low temporal resolution data with low spatial and high temporal resolution data. Generally speaking, low spatial and high temporal resolution data are chosen from MODIS or VIIRS data, since data with longer temporal coverage such as GIMMS NDVI have a huge difference in spatial resolution (about 8000 m) with Landsat (30 m), Sentinel (10 m) and other high spatial resolution data, which may lead to high uncertainty. The consequence of choosing MODIS and VIIRS data is that these methods cannot extend the observation period of high-resolution data to pre-2000. In the GPP processing workflow proposed in this paper, only Landsat satellite data is used, which is the longest global satellite observation record (Wulder et al. Citation2022). This allows us to extend the high-resolution GPP observation period to the 1980s, which is crucial for some climate change research and ecological management in climate-sensitive areas. Apart from the limitations discussed before, these methods are usually very time-consuming, such as spatiotemporal fusion algorithms. Although some scholars have successfully implemented this algorithm on the GEE platform (Nietupski et al. Citation2021), due to the low-end server cluster as the underlying foundation of GEE, and the limitation of the amount of data that can be passed into a single server in these clusters (Gorelick et al. Citation2017), GEE cannot effectively execute spatiotemporal fusion algorithms, and the computing efficiency is slow. The parallel processing mode of GEE itself makes the pixel-by-pixel calculation process used in this paper very efficient. This feature allows us to extend the processing workflow in this paper to any location in the world, so that we can help people quickly and conveniently obtain high-resolution GPP data.
6. Conclusion
We developed a long-term (1987–2021) and high spatiotemporal resolution (16-day, 30 m) GPP dataset for Qinghai Province using the Google Earth Engine (GEE) platform. By integrating multiple data sources, vegetation index filling and smoothing algorithms, and revised EC-LUE model, our estimates agreed well with flux tower observations, with a high average correlation coefficient of 0.978 and the second lowest RMSE of 12.312
. This indicates a small deviation and consistent temporal variation between
and flux tower observations. Since flux tower sites are limited in Qinghai Province, we compared
with reference datasets under different vegetation types. Overall,
showed the highest correlation coefficients (0.868–0.943), lowest RMSE (123.750–276.978
), and bias (−61.776–193.949
) in grassland areas, which constitute the majority of Qinghai Province. These results demonstrate the high consistency of the
estimates with the reference datasets in grassland type. The validation results indicate that our estimated GPP for Qinghai Province is similar in accuracy to existing GPP datasets.
We analyzed the estimated GPP and found that vegetation productivity in Qinghai Province had a fluctuating growth over the past 35 years, with a growth rate of 4.42 (p<0.05). Approximately 90% of the vegetation areas exhibited an increasing trend in GPP. Qinghai Province's GPP had clear seasonality and spatial consistency, influenced by factors such as altitude and hydro-thermal conditions. Summer accounted for approximately 87% of the annual GPP, and most of the GPP was concentrated in the eastern and southeastern regions of Qinghai Province, where hydro-thermal conditions were favorable. In contrast, the GPP values were extremely low in the large desert areas of western and northwestern Qinghai Province.
Compared with existing datasets, this GPP dataset has some advantages or features. For example, our dataset is the first high resolution GPP dataset for Qinghai Province. Although some researchers have estimated high-resolution GPP using GEE (such as He et al. (Citation2018); Robinson et al. (Citation2018)), these datasets usually do not cover Qinghai Province, and our dataset has higher accuracy and reliability in Qinghai Province because we used independent site data located in Qinghai Province for training and validation. Secondly, our dataset has a longer time coverage, and the dataset is housed in GEE, which is convenient for researchers to analyze it. In summary, our GPP dataset can provide reliable observations of long-term GPP in Qinghai Province at a finer scale.
Supplemental Material
Download MS Word (18.3 KB)Disclosure statement
No potential conflict of interest was reported by the author(s).
Data availability statement
The GPP dataset of this study are openly available in https://code.earthengine.google.com/3443ab7c12cf04448eda52376a44cc07
Additional information
Funding
References
- Bagnara, Maurizio, Matteo Sottocornola, Alessandro Cescatti, Stefano Minerbi, Leonardo Montagnani, Damiano Gianelle, and Federico Magnani. 2015. “Bayesian Optimization of a Light Use Efficiency Model for the Estimation of Daily Gross Primary Productivity in a Range of Italian Forest Ecosystems.” Ecological Modelling 306:57–66. https://doi.org/10.1016/j.ecolmodel.2014.09.021.
- Beer, Christian, Markus Reichstein, Enrico Tomelleri, Philippe Ciais, Martin Jung, Nuno Carvalhais, and Christian Rödenbeck. 2010. “Terrestrial Gross Carbon Dioxide Uptake: Global Distribution and Covariation with Climate.” Science 329 (5993): 834–838. https://doi.org/10.1126/science.1184984.
- Bi, Wenjun, Wei He, Yanlian Zhou, Weimin Ju, Yibo Liu, Yang Liu, Xiaoyu Zhang, Xiaonan Wei, and Nuo Cheng. 2022. “A Global 0.05 Dataset for Gross Primary Production of Sunlit and Shaded Vegetation Canopies From 1992 to 2020.” Scientific Data 9 (1): 213. https://doi.org/10.1038/s41597-022-01309-2.
- Cao, Ruyin, Zichao Xu, Yang Chen, Jin Chen, and Miaogen Shen. 2022. “Reconstructing High-Spatiotemporal-Resolution (30 m and 8-Days) NDVI Time-Series Data for the Qinghai–Tibetan Plateau From 2000–2020.” Remote Sensing14 (15): 3648. https://doi.org/10.3390/rs14153648.
- Chang, Xiaoqing, Yanqiu Xing, Weishu Gong, Cheng Yang, Zhen Guo, Dejun Wang, Jiaqi Wang, et al. 2023. “Evaluating Gross Primary Productivity Over 9 ChinaFlux Sites Based on Random Forest Regression Models, Remote Sensing, and Eddy Covariance Data.” Science of The Total Environment 875:162601. https://doi.org/10.1016/j.scitotenv.2023.162601.
- Chen, Yang, Ruyin Cao, Jin Chen, Licong Liu, and Bunkei Matsushita. 2021. “A Practical Approach to Reconstruct High-quality Landsat NDVI Time-series Data by Gap Filling and the Savitzky–Golay Filter.” ISPRS Journal of Photogrammetry and Remote Sensing 180:174–190. https://doi.org/10.1016/j.isprsjprs.2021.08.015.
- Chen, Min, Rashid Rafique, Ghassem R. Asrar, Ben Bond-Lamberty, Philippe Ciais, Fang Zhao, and Christopher P. O. Reyer. 2017. “Regional Contribution to Variability and Trends of Global Gross Primary Productivity.” Environmental Research Letters 12 (10): 105005. https://doi.org/10.1088/1748-9326/aa8978.
- Claverie, Martin, Eric F. Vermote, Belen Franch, and Jeffrey G. Masek. 2015. “Evaluation of the Landsat-5 TM and Landsat-7 ETM+ Surface Reflectance Products.” Remote Sensing of Environment 169:390–403. https://doi.org/10.1016/j.rse.2015.08.030.
- Fang, Zhongxiang, Wenmin Zhang, Martin Brandt, Abdulhakim M. Abdi, and Rasmus Fensholt. 2022. “Globally Increasing Atmospheric Aridity Over the 21st Century.” Earth's Future 10 (10): e2022EF003019. https://doi.org/10.1029/2022EF003019.
- Foga, Steve, Pat L. Scaramuzza, Song Guo, Zhe Zhu, Ronald D. Dilley Jr, Tim Beckmann, Gail L. Schmidt, et al. 2017. “Cloud Detection Algorithm Comparison and Validation for Operational Landsat Data Products.” Remote Sensing of Environment194:379–390. https://doi.org/10.1016/j.rse.2017.03.026.
- Gao, Feng, Jeff Masek, Matt Schwaller, and Forrest Hall. 2006. “On the Blending of the Landsat and MODIS Surface Reflectance: Predicting Daily Landsat Surface Reflectance.” IEEE Transactions on Geoscience and Remote Sensing 44 (8): 2207–2218. https://doi.org/10.1109/TGRS.2006.872081.
- Giannico, Vincenzo, Jiquan Chen, Changliang Shao, Zutao Ouyang, Ranjeet John, and Raffaele Lafortezza. 2018. “Contributions of Landscape Heterogeneity Within the Footprint of Eddy-covariance Towers to Flux Measurements.” Agricultural and Forest Meteorology 260:144–153. https://doi.org/10.1016/j.agrformet.2018.06.004.
- Gitelson, Anatoly A., Yi Peng, Jeffery G. Masek, Donald C. Rundquist, Shashi Verma, Andrew Suyker, John M. Baker, Jerry L. Hatfield, and Tilden Meyers. 2012. “Remote Estimation of Crop Gross Primary Production with Landsat Data.” Remote Sensing of Environment 121:404–414. https://doi.org/10.1016/j.rse.2012.02.017.
- Gorelick, Noel, Matt Hancher, Mike Dixon, Simon Ilyushchenko, David Thau, and Rebecca Moore. 2017. “Google Earth Engine: Planetary-scale Geospatial Analysis for Everyone.” Remote Sensing of Environment 202:18–27. https://doi.org/10.1016/j.rse.2017.06.031.
- He, Mingzhu, John S. Kimball, Marco P. Maneta, Bruce D. Maxwell, Alvaro Moreno, Santiago Beguería, and Xiaocui Wu. 2018. “Regional Crop Gross Primary Productivity and Yield Estimation Using Fused Landsat-MODIS Data.” Remote Sensing 10 (3): 372. https://doi.org/10.3390/rs10030372.
- He, Shaoyang, Yongqiang Zhang, Ning Ma, Jing Tian, Dongdong Kong, and Changming Liu. 2022. “A Daily and 500 m Coupled Evapotranspiration and Gross Primary Production Product Across China During 2000–2020.” Earth System Science Data 14 (12): 5463–5488. https://doi.org/10.5194/essd-14-5463-2022.
- Heinsch, Faith Ann, Maosheng Zhao, Steven W. Running, John S. Kimball, Ramakrishna R. Nemani, Kenneth J. Davis, and Paul V. Bolstad. 2006. “Evaluation of Remote Sensing Based Terrestrial Productivity From MODIS Using Regional Tower Eddy Flux Network Observations.” IEEE Transactions on Geoscience and Remote Sensing 44 (7): 1908–1925. https://doi.org/10.1109/TGRS.2005.853936.
- Huang, Mengtian, Shilong Piao, Philippe Ciais, Josep Peñuelas, Xuhui Wang, Trevor F. Keenan, and Shushi Peng. 2019. “Air Temperature Optima of Vegetation Productivity Across Global Biomes.” Nature Ecology & Evolution 3 (5): 772–779. https://doi.org/10.1038/s41559-019-0838-x.
- Jiang, Chongya, Kaiyu Guan, Genghong Wu, Bin Peng, and Sheng Wang. 2021. “A Daily, 250 m and Real-time Gross Primary Productivity Product (2000–present) Covering the Contiguous United States.” Earth System Science Data 13 (2): 281–298. https://doi.org/10.5194/essd-13-281-2021.
- Jingbin, Zhu, He Huidan, Li Hongqin, Zhang Fawei, Li Yingnian, Yang Yongsheng, Zhang Guangru, Wang Chunyu, and Luo Fanglin. 2020. “Effect of Growing Season Degree Days on Gross Primary Productivity and Its Variation Characteristics in Alpine Wetland of the Qinghai-Tibetan Plateau.” Acta Ecologica Sinica 40 (24): 8958–8965.
- Knox, Sara Helen, Iryna Dronova, Cove Sturtevant, Patricia Y. Oikawa, Jaclyn Hatala Matthes, Joseph Verfaillie, and Dennis Baldocchi. 2017. “Using Digital Camera and Landsat Imagery with Eddy Covariance Data to Model Gross Primary Production in Restored Wetlands.” Agricultural and Forest Meteorology 237:233–245. https://doi.org/10.1016/j.agrformet.2017.02.020.
- Li, Ruicheng, Tianxiang Luo, Thomas Mölg, Jingxue Zhao, Xiang Li, Xiaoyong Cui, Mingyuan Du, and Yanhong Tang. 2016. “Leaf Unfolding of Tibetan Alpine Meadows Captures the Arrival of Monsoon Rainfall.” Scientific Reports 6 (1): 20985. https://doi.org/10.1038/srep20985.
- Lin, Xiao-ding, Le Chang, and Dan Feng. 2021. “Remote-sensing Estimation of Vegetation Gross Primary Productivity and Its Spatiotemporal Changes in Qinghai Province From 2000 to 2019.” Acta Prataculturae Sinica 30 (6): 16.
- Lin, Shangrong, J. Li, and Q. Liu. 2018. “Overview on Estimation Accuracy of Gross Primary Productivity with Remote Sensing Methods.” Journal of Remote Sensing 22:234–254.
- Ma, Minna, Wenping Yuan, Jie Dong, Fawei Zhang, Wenwen Cai, and Hongqin Li. 2018. “Large-scale Estimates of Gross Primary Production on the Qinghai-Tibet Plateau Based on Remote Sensing Data.” International Journal of Digital Earth 11 (11): 1166–1183. https://doi.org/10.1080/17538947.2017.1381192.
- Masek, Jeffrey G., Eric F. Vermote, Nazmi E. Saleous, Robert Wolfe, Forrest G. Hall, Karl Fred Huemmrich, Feng Gao, Jonathan Kutler, and Teng-Kui Lim. 2006. “A Landsat Surface Reflectance Dataset for North America, 1990–2000.” IEEE Geoscience and Remote Sensing Letters 3 (1): 68–72. https://doi.org/10.1109/LGRS.2005.857030.
- Monteith, John L. 1972. “Solar Radiation and Productivity in Tropical Ecosystems.” Journal of Applied Ecology 9 (3): 747–766. https://doi.org/10.2307/2401901.
- Nietupski, Ty C., Robert E. Kennedy, Hailemariam Temesgen, and Becky K. Kerns. 2021. “Spatiotemporal Image Fusion in Google Earth Engine for Annual Estimates of Land Surface Phenology in a Heterogenous Landscape.” International Journal of Applied Earth Observation and Geoinformation99:102323. https://doi.org/10.1016/j.jag.2021.102323.
- Pastorello, Gilberto, Carlo Trotta, Eleonora Canfora, Housen Chu, Danielle Christianson, You-Wei Cheah, and Cristina Poindexter. 2020. “The FLUXNET2015 Dataset and the ONEFlux Processing Pipeline for Eddy Covariance Data.” Scientific Data 7 (1): 1–27. https://doi.org/10.1038/s41597-020-0534-3.
- Peter, Brad G., and Joseph P. Messina. 2019. “Errors in Time-series Remote Sensing and An Open Access Application for Detecting and Visualizing Spatial Data Outliers Using Google Earth Engine.” IEEE Journal of Selected Topics in Applied Earth Observations and Remote Sensing 12 (4): 1165–1174. https://doi.org/10.1109/JSTARS.4609443.
- Robinson, Nathaniel P., Brady W. Allred, Matthew O. Jones, Alvaro Moreno, John S. Kimball, David E. Naugle, Tyler A. Erickson, and Andrew D. Richardson. 2017. “A Dynamic Landsat Derived Normalized Difference Vegetation Index (NDVI) Product for the Conterminous United States.” Remote Sensing 9 (8): 863. https://doi.org/10.3390/rs9080863.
- Robinson, Nathaniel P., Brady W. Allred, William K. Smith, Matthew O. Jones, Alvaro Moreno, Tyler A. Erickson, David E. Naugle, and Steven W. Running. 2018. “Terrestrial Primary Production for the Conterminous United States Derived From Landsat 30 M and MODIS 250 m.” Remote Sensing in Ecology and Conservation 4 (3): 264–280. https://doi.org/10.1002/rse2.2018.4.issue-3.
- Roy, David P., V. Kovalskyy, H. K. Zhang, Eric F. Vermote, L. Yan, S. S. Kumar, and A. Egorov. 2016. “Characterization of Landsat-7 to Landsat-8 Reflective Wavelength and Normalized Difference Vegetation Index Continuity.” Remote Sensing of Environment 185:57–70. https://doi.org/10.1016/j.rse.2015.12.024.
- Running, S., and M. Zhao. 2021. “MODIS/Terra Net Primary Production Gap-Filled Yearly L4 Global 500m SIN Grid V061.” NASA EOSDIS Land Processes DAAC.
- Shen, Miaogen, Shilong Piao, Su-Jong Jeong, Liming Zhou, Zhenzhong Zeng, Philippe Ciais, and Deliang Chen. 2015. “Evaporative Cooling Over the Tibetan Plateau Induced by Vegetation Growth.” Proceedings of the National Academy of Sciences 112 (30): 9299–9304. https://doi.org/10.1073/pnas.1504418112.
- Vermote, Eric, Chris Justice, Martin Claverie, and Belen Franch. 2016. “Preliminary Analysis of the Performance of the Landsat 8/OLI Land Surface Reflectance Product.” Remote Sensing of Environment185:46–56. https://doi.org/10.1016/j.rse.2016.04.008.
- Wang, Yipu, Rui Li, Jiheng Hu, Yuyun Fu, Jiawei Duan, and Yuanxi Cheng. 2021. “Daily Estimation of Gross Primary Production Under All Sky Using a Light Use Efficiency Model Coupled with Satellite Passive Microwave Measurements.” Remote Sensing of Environment 267:112721. https://doi.org/10.1016/j.rse.2021.112721.
- Wang, Xufeng, Mingguo Ma, Xin Li, Yi Song, Junlei Tan, Guanghui Huang, and Wenping Yu. 2012. “Comparison of Remote Sensing Based GPP Models At An Alpine Meadow Site.” Yaogan Xuebao- Journal of Remote Sensing 16 (4): 751–763.
- Wei, Yanqiang, Haiyan Lu, Jinniu Wang, Xufeng Wang, and Jian Sun. 2022. “Dual Influence of Climate Change and Anthropogenic Activities on the Spatiotemporal Vegetation Dynamics Over the Qinghai-Tibetan Plateau From 1981 to 2015.” Earth's Future 10 (5): e2021EF002566. https://doi.org/10.1029/2021EF002566.
- Wild, Benjamin, Irene Teubner, Leander Moesinger, Ruxandra-Maria Zotta, Matthias Forkel, Robin van der Schalie, Stephen Sitch, and Wouter Dorigo. 2022. “VODCA2GPP–a New, Global, Long-term (1988–2020) Gross Primary Production Dataset From Microwave Remote Sensing.” Earth System Science Data 14 (3): 1063–1085. https://doi.org/10.5194/essd-14-1063-2022.
- Wilson, Barry T., Joseph F. Knight, and Ronald E. McRoberts. 2018. “Harmonic Regression of Landsat Time Series for Modeling Attributes From National Forest Inventory Data.” ISPRS Journal of Photogrammetry and Remote Sensing 137:29–46. https://doi.org/10.1016/j.isprsjprs.2018.01.006.
- Wolanin, Aleksandra, Gustau Camps-Valls, Luis Gómez-Chova, Gonzalo Mateo-García, Christiaan van der Tol, Yongguang Zhang, and Luis Guanter. 2019. “Estimating Crop Primary Productivity with Sentinel-2 and Landsat 8 Using Machine Learning Methods Trained with Radiative Transfer Simulations.” Remote Sensing of Environment 225:441–457. https://doi.org/10.1016/j.rse.2019.03.002.
- Wulder, Michael A., David P. Roy, Volker C. Radeloff, Thomas R. Loveland, Martha C. Anderson, David M. Johnson, and Sean Healey. 2022. “Fifty Years of Landsat Science and Impacts.” Remote Sensing of Environment 280:113195. https://doi.org/10.1016/j.rse.2022.113195.
- Yang, Jie, and Xin Huang. 2021. “The 30 m Annual Land Cover Dataset and Its Dynamics in China From 1990 to 2019.” Earth System Science Data 13 (8): 3907–3925. https://doi.org/10.5194/essd-13-3907-2021.
- Yang, Kun, Hui Wu, Jun Qin, Changgui Lin, Wenjun Tang, and Yingying Chen. 2014. “Recent Climate Changes Over the Tibetan Plateau and Their Impacts on Energy and Water Cycle: A Review.” Global and Planetary Change 112:79–91. https://doi.org/10.1016/j.gloplacha.2013.12.001.
- Ye, Xuchun, Xiaoxia Yang, Fuhong Liu, Juan Wu, and Jia Liu. 2021. “Spatio-temporal Variations of Land Vegetation Gross Primary Production in TheYangtze River Basin and Correlation with Meteorological Factors.” Acta Ecologica Sinica 41 (17): 1–11.
- Yuan, Wenping, Shunlin Liang, Shuguang Liu, Ensheng Weng, Yiqi Luo, David Hollinger, and Haicheng Zhang. 2012. “Improving Model Parameter Estimation Using Coupling Relationships Between Vegetation Production and Ecosystem Respiration.” Ecological Modelling 240:29–40. https://doi.org/10.1016/j.ecolmodel.2012.04.027.
- Yuan, Wenping, Shuguang Liu, Guangsheng Zhou, Guoyi Zhou, Larry L. Tieszen, Dennis Baldocchi, and Christian Bernhofer. 2007. “Deriving a Light Use Efficiency Model From Eddy Covariance Flux Data for Predicting Daily Gross Primary Production Across Biomes.” Agricultural and Forest Meteorology143 (3-4): 189–207. https://doi.org/10.1016/j.agrformet.2006.12.001.
- Yuan, Wenping, Yi Zheng, Shilong Piao, Philippe Ciais, Danica Lombardozzi, Yingping Wang, and Youngryel Ryu. 2019. “Increased Atmospheric Vapor Pressure Deficit Reduces Global Vegetation Growth.” Science Advances 5 (8): eaax1396. https://doi.org/10.1126/sciadv.aax1396.
- Zhang, Yongqiang, Dongdong Kong, Rong Gan, Francis H. S. Chiew, Tim R. McVicar, Qiang Zhang, and Yuting Yang. 2019. “Coupled Estimation of 500 m and 8-day Resolution Global Evapotranspiration and Gross Primary Production in 2002–2017.” Remote Sensing of Environment222:165–182. https://doi.org/10.1016/j.rse.2018.12.031.
- Zhang, Xinzhu, Hesong Wang, Hao Yan, and Jinlong Ai. 2021. “Analysis of Spatio-temporal Changes of Gross Primary Productivity in China From 2001 to 2018 Based on Romote Sensing.” Acta Ecologica Sinica 41 (16): 6351–6362.
- Zhang, Yao, Xiangming Xiao, Xiaocui Wu, Sha Zhou, Geli Zhang, Yuanwei Qin, and Jinwei Dong. 2017. “A Global Moderate Resolution Dataset of Gross Primary Production of Vegetation for 2000–2016.” Scientific Data 4 (1): 1–13.
- Zheng, Yi, Li Zhang, Jingfeng Xiao, Wenping Yuan, Min Yan, Tong Li, and Zhiqiang Zhang. 2018. “Sources of Uncertainty in Gross Primary Productivity Simulated by Light Use Efficiency Models: Model Structure, Parameters, Input Data, and Spatial Resolution.” Agricultural and Forest Meteorology263:242–257. https://doi.org/10.1016/j.agrformet.2018.08.003.
- Zhong, Lei, Yaoming Ma, Yongkang Xue, and Shilong Piao. 2019. “Climate Change Trends and Impacts on Vegetation Greening Over the Tibetan Plateau.” Journal of Geophysical Research: Atmospheres 124 (14): 7540–7552. https://doi.org/10.1029/2019JD030481.
- Zhou, Hao, Xu Yue, Yadong Lei, Tianyi Zhang, Chenguang Tian, Yimian Ma, and Yang Cao. 2021. “Responses of Gross Primary Productivity to Diffuse Radiation At Global FLUXNET Sites.” Atmospheric Environment 244:117905. https://doi.org/10.1016/j.atmosenv.2020.117905.
- Zhu, Xiaolin, Jin Chen, Feng Gao, Xuehong Chen, and Jeffrey G. Masek. 2010. “An Enhanced Spatial and Temporal Adaptive Reflectance Fusion Model for Complex Heterogeneous Regions.” Remote Sensing of Environment 114 (11): 2610–2623. https://doi.org/10.1016/j.rse.2010.05.032.