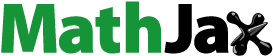
ABSTRACT
Effectively exploring the impacts of urban spatial structures on carbon dioxide emissions is important for achieving low-carbon goals. However, most previous studies have examined the impact of urban spatial structure on total carbon emissions based only on polycentricity. Fine-grained studies on subsectoral carbon emissions and other dimensions of urban spatial structure are lacking. Therefore, our study comprehensively explores the impact of urban dispersion and polycentricity on total carbon emissions and carbon emissions of four subsectors (industry, power, civilian, and transportation) from 2012 to 2017 while considering the effects of city size. Results reveal that the nighttime light data is useful for measuring urban spatial structure, and a polycentric, decentralized urban spatial structure correlates with the reduced total carbon emissions and transportation carbon emissions. Meanwhile, a decentralized urban spatial structure gives rise to lower industrial carbon emissions and civilian carbon emissions, whereas a multicenter urban spatial structure contributes to minimizing carbon emissions from power systems. However, in small and medium-sized cities, urban spatial structure differently affects the total carbon and transportation carbon emissions.
1. Introduction
Since the industrial revolution, the increasing greenhouse effect has accelerated global warming (Wang et al. Citation2014). According to Zhang et al. (Citation2021), from 1990 to 2014, global greenhouse gas emissions increased by about 15 billion tons, with carbon dioxide emissions considerably contributing to such an increase. China has inevitably experienced rapid industrialization and urbanization, which has caused high energy consumption and carbon dioxide emissions (Shi et al. Citation2018). Meanwhile, China has pledged to meet its peak carbon target by around 2030 (Yang et al. Citation2020). Therefore, the reduction of carbon emissions has become a primary task in China.
Similar to traditional technologies and strategies of energy efficiency enhancement, an appropriate urban spatial structure can effectively reduce carbon dioxide emissions (Lee and Lee Citation2014; Shi et al. Citation2023). Previous studies have focused on two aspects. First, the impact of polycentricity on carbon emissions is primarily explored. For example, Makido, Dhakal, and Yamagata (Citation2012) found that polycentric cities in Japan tend to reduce carbon emissions. Liu et al. (Citation2020) showed limited effects of polycentric structure on carbon emissions. Second, landscape indicators are often used to characterize urban spatial structure and determine its impact on carbon emissions. For instance, Shi et al. (Citation2020) demonstrated that complex and decentralized urban structures are positively correlated with carbon emissions, while cohesive and rule-based urban structures exhibit the opposite trend. She et al. (Citation2015) analyzed the geographic changes in 30 cities in the Yangtze River Delta from 1990–2015, revealing positive correlations between carbon emissions and factors, such as patch size, number of urban patches, maximum patch index, road network density, and average perimeter–area ratio. Bereitschaft and Debbage (Citation2013) examined 86 metropolitan areas in the United States in 2000 as the study area, concluding that urban continuity, urban form complexity, residential density, centrality, street accessibility, and Smart Growth America sprawl index were correlated with carbon emissions.
However, these studies only focused on the influence of urban spatial structures on total carbon emissions and the potential relation between urban spatial structures and carbon emissions of different sectors is still unknown.
In previous studies, the urban spatial structure was always measured from a single dimension of polycentricity (Garreau Citation1991). However, the urban spatial structure should contain two dimensions: polycentricity and dispersion (Li Citation2020). Polycentricity reflects the concentration of the population and employment in a small number of locations (i.e. main centers and subcenters), whereas the decentralized dimension represents the dispersion of employment and population outside the main center of a city (Burger and Meijers Citation2010). According to these two dimensions, the transformation of an urban spatial structure from a monocentric structure can be grouped into four potential patterns (). For example, if the transformation has diverse destinations that are scattered and not well developed, the urban spatial structure has high dispersion and low polycentricity (a). b represents an urban spatial structure with high polycentricity and dispersion, whereas c illustrates a monocentric urban spatial structure. When transformation has a specific destination that has developed well, the urban spatial structure becomes polycentric with low dispersion (d). Therefore, using the two dimensions simultaneously can help us better quantitatively understand the urban spatial structures (Li and Liu Citation2018; Zhu, Tu, and Li Citation2022).
Figure 1. Four potential transformation patterns of urban spatial structures (adapted from Burger and Meijers (Citation2010)).

Traditionally, two types of method are widely employed to quantify urban dispersion. The first is directly using statistical data to refer to the degree to which the population is clustered or concentrated in urban centers (Lee Citation2007), such as the number of counties (Hill Citation1974), room and dwelling density (Bramley and Power Citation2009; Glaeser and Kahn Citation2010), and job opportunity (Glaeser et al. Citation2001). Generally, these studies treated all urban centers equally, which is not consistent with reality as urban centers could have their own sizes of influence on surrounding areas. Therefore, the second type is calculating indices with the weights of each unit, including the Herfindahl–Hirschman Index (Hirschman Citation1964), the space Gini coefficient (Audretsch and Feldman Citation1996), the Ellison–Glaeser Index (Ellison and Glaeser Citation1994), and the DO Index (Duranton and Overman Citation2005). However, these indices could exaggerate the contribution of larger urban centers as they are calculated using the percentage sum of squares of contributions of each urban center, such as economy, population, or employment. Thus, the metropolitan fragmentation index (MFI) was proposed based on the percentage sum of the square root of a contribution instead of the percentage sum of the square of a contribution (Mitchell-Weaver, Miller, and Deal Citation2000). Because MFI has a better-balanced value range, we decided to use this index to quantify the urban dispersion herein.
The methods for measuring polycentricity focused on two main perspectives (Liu, Derudder, and Wang Citation2018). The first is the importance of individual center, which is traditionally measured from their population, employment, and gross domestic product (Sha et al. Citation2020) as well as the interactions among centers (Camagni and Capello Citation2004). Furthermore, the measurement of the balance of importance among the centers can be grouped into three types: the rank size approach (Burger and Meijers Citation2010), the comparison between the observed actual distribution and some ideal–typical benchmark scenarios (Hanssens et al. Citation2014), and a social network-based approach (Green Citation2007). However, previous methods only focused on one of the two perspectives. Thus, we adopted a novel polycentric indicator based on the number of centers and the above two aspects to comprehensively understand urban polycentricity (Amindarbari and Sevtsuk Citation2012).
In addition, although the MFI from Mitchell-Weaver, Miller, and Deal (Citation2000) and the polycentric metric from Amindarbari and Sevtsuk (Citation2012) performed well in representing urban dispersion and polycentricity, they highly relied on statistical data. These statistics often have a time lag and are incomplete in some backward areas, seriously limiting their usefulness. Therefore, an alternative data source is required to enhance the measurement of urban spatial structures.
Nighttime light (NTL) remote sensing uses sensors to record artificial light emitted by cities and towns on the Earth’s surface (Elvidge et al. Citation1997). NTL datasets have been utilized to estimate gross the domestic product (Chen and Nordhaus Citation2011; Shi et al. Citation2014), carbon dioxide emissions (Shi et al. Citation2016), electricity consumption (Elvidge et al. Citation1997), postwar economic recovery assessment (Li et al. Citation2018; Li et al. Citation2018), housing vacancy rates (Chen et al. Citation2015), poverty (Yu et al. Citation2015), and economic development quality (Chen et al. Citation2023; Yang et al. Citation2019). NTL remote sensing data not only has wide coverage, high efficiency and objectivity but also can characterize the attributes of human economic activities; therefore, it can be applied to the extraction and research of urban spatial information and a lot of research results have been obtained from the analysis of urban spatial structures (Chen et al. Citation2017).
Herein, we aimed to measure the polycentricity and dispersion of urban spatial structures from NTL data and use a pooled regression model to reveal how urban spatial structures affect multisectoral carbon emissions within 96 cities in southeast China from 2012 to 2017. The objectives of this study are as follows: 1) to accurately quantify urban spatial structures from the two dimensions of polycentricity and aggregation using NTL data and 2) to study what urban spatial structures can reduce carbon emissions.
2. Study areas and data sources
2.1. Study areas
Herein, 96 cities in the southeastern region of China were selected to analyze the correlation between urban spatial structures and carbon emissions based on the following two main reasons (). First, coastal towns develop considerably faster than inland cities because of their natural advantage of being open to the outside world. This rapid development causes rapid economic growth and thus a sharp increase in carbon dioxide emissions. Therefore, the task of reducing carbon dioxide emissions is more challenging in coastal areas than in other regions. Second, China’s southeast region includes cities with diverse functions, such as resource-intensive cities (e.g. Shanghai, Shenzhen, and Guangzhou) and tourism service cities (e.g. Jingdezhen, Jiujiang, and Huangshan). Notably, because the carbon emission data in Hainan Province were incomplete, we excluded the Hainan Province from this study.
2.2. Data sources
NPP-VIIRS-like NTL data were used to calculate the urban dispersion and polycentricity of urban spatial structures and can be obtained from https://doi.org/10.7910/DVN/YGIVCD (Chen et al. Citation2021). This dataset overcomes the limitation that DMSP-OLS stable NTL data (2000–2012) and Suomi NPP-VIIRS NTL data (2013–2018) cannot be directly used together for long-term analysis. In addition, the spatial resolution of the class NPP-VIIRS NTL data reached 500 m and was validated for accuracy.
Urban extent data from harmonized NTLs (Zhao et al. Citation2022) were used to integrate with the NPP-VIIRS-like NTL data for the measurement of urban dispersion. This dataset fits well with the traditional global urban boundaries derived from impervious surface area. This dataset was obtained from https://doi.org/10.6084/m9.figshare.16602224.
The LandScan high-resolution global population dataset was adopted to identify urban centers for further polycentricity measurements. The LandScan population dataset captures the average population distribution over 24 h, with a grid resolution of approximately 1 km (30″ × 30″). The dataset can be freely accessed from the US Department of Energy’s Oak Ridge National Laboratory (https://landscan.ornl.gov/).
Carbon emission data were derived from the Multi-resolution Emission Inventory for China (MEIC) model (Li et al. Citation2017; Zheng et al. Citation2018). The MEIC model contains data on the total carbon emissions and carbon emissions in four subsectors: industry, power, civilian, and transportation. Meanwhile, the dataset covers the whole of mainland China and has a spatial resolution of 0.25° × 0.25°. Because the dataset was available from 2008 to 2017 and the growth rate of China’s emissions first decelerated in 2012, we set the study period to 2012–2017(Zheng et al. Citation2019) ().
2.3. Control variables
Carbon emissions may be influenced by factors other than urban spatial structure; therefore, four control variables were set up herein to better explore the impact of urban spatial structure on carbon emissions. Based on previous studies (Shi et al. Citation2019; Shi et al. Citation2020), this study included several control variables such as gross domestic product per capita (GDP), normalized difference vegetation index (NDVI), number of patents (TEC), and total nighttime light intensity (TNL) in the regression model. (1) GDP represents the level of economic development of the city, and rapid economic growth may lead to a sharp increase in carbon emissions. (2) NDVI, a natural environmental factor, affects carbon concentration through the absorption of carbon dioxide by plants. (3) TEC mainly represents the innovation level of the city through scientific and technological innovation and can develop new energy sources, thereby reducing carbon emissions (You and Chen Citation2022). (4) TNL primarily characterizes human activities and is closely related to carbon emissions (Chen et al. Citation2017).
Data on GDP and TEC were collected from the Statistical Yearbook of Chinese Cities (2012–2017). NDVI data were obtained from the Resource and Environmental Science and Data Center of the Chinese Academy of Sciences (https://www.resdc.cn/). Annual NDVI data were used in this study, which mainly used the maximum value in the monthly NDVI from January to December of each year. The data covered the period from 1998 to 2019. The spatial resolution of this data was 1 km, which met the accuracy requirement of this study.
3. Methodology
As shown in , we quantified the urban polycentricity and dispersion of urban spatial structures using NPP-VIIRS-like NTL data and investigated their impacts on subsectoral carbon emissions using a pooled regression model. This comprised three components. (1) Urban dispersion of each city was calculated using the MFI based on the total night light intensity within the urban extents. (2) The urban polycentricity was calculated according to the results of the urban center identification from LandScan gridded population data and the exploratory spatial data analysis (ESDA) method at the city level. (3) The pooled model was used to explore the effects of urban polycentricity and dispersion on subsectoral carbon emissions.
Figure 4. Framework of the methodology. GDP: gross domestic product per capita, NDVI: normalized difference vegetation index; TEC: number of patents, TNL: total nighttime light intensity, ICE: industrial carbon emissions, PCE: power carbon emissions, CCE: civilian carbon emissions, and TCE: transportation carbon emissions.

3.1. Measuring urban spatial structures from NTL data
3.1.1. Measurement of urban dispersion
Urban dispersion was calculated using the MFI method from Mitchell-Weaver, Miller, and Deal (Citation2000). The detailed expressions are as follows:
(1)
(1)
(2)
(2) where
(i = 1, 2, … , n, where n is the number of urban extents in the urban area) represents the luminance value in the urban extent,
represents the proportion of the luminance value in the ith urban extent block to the total urban extent blocks, and I is the fragmentation index, where a larger value of I means that the city has a higher level of urban dispersion. The range of I varies in different cities as its maximum value is controlled by the number of urban extents of each city, which makes the degree of dispersion based on I not comparable between any two cities. Therefore, the Min–Max normalization method was applied to make the degree of dispersion comparable across cities (Panda and Jana Citation2015), using equation 3.
(3)
(3) where Inor is the normalized value of I. IMax and IMin are the maximum and minimum values of I, respectively.
3.1.2. Measurement of urban polycentricity
The measurement of urban polycentricity developed by Amindarbari and Sevtsuk (Citation2012) requires the identification of urban centers. Herein, we used ESDA to detect the urban centers from LandScan gridded population data (Li and Liu Citation2018). First, we calculated the local Moran’s I index based on the LandScan population dataset. We considered the population grid identified as a high–high cluster (HH) as the urban center. Then, the extracted HH grids were aggregated under the criterion of rook contiguity to obtain potential urban centers. Finally, potential urban centers with an area exceeding 2 km2 and a population higher than 50,000 were selected as the final urban centers (Li, Xiong, and Wang Citation2019; Sha et al. Citation2020).
The degree of intracity polycentricity (PC) from Amindarbari and Sevtsuk (Citation2012) contained three dimensions: the number of urban centers (N), the degree of agglomeration (R), and the degree of balance of urban centers (HI), as shown in equation (4). The three dimensions are specified as follows: (1) cities with more urban centers have a more substantial level of polycentricity than cities with fewer urban centers; (2) the more urban elements are clustered in urban centers, the higher the level of polycentricity, where the number of urban elements is measured by the intensity of luminosity in this study; and (3) the more balanced the power among urban centers within a city, the higher the level of urban polycentricity, where the degree of center balance is calculated based on the entropy index by Limtanakool, Schwanen, and Dijst (Citation2009). The calculation of the degree of polycentricity can be expressed as
(4)
(4)
(5)
(5) where
represents the total luminous value in the ith urban center; N represents the number of urban centers. As this formula is valid only for polycentric cities, we arbitrarily set the PC value for monocentric cities as 0.
3.2. Developing the pooled regression model
The pooled regression model was adopted to analyze the effects of urban spatial structures on total and subsectoral carbon emissions because of its two advantages. First, it has a particular time dimension, so it can solve the problem of insufficient sample size to a certain extent (Hsiao Citation2014). Second, it can reduce the mutual influence between independent variables and weaken the influence of multicollinearity (Fang, Wang, and Li Citation2015). The specific formula is as follows:
(6)
(6) In addition to two urban spatial structure indicators, four indicators (GDP, TEC, NDVI, and NTL) were selected as control variables.
represents the carbon dioxide emissions of the ith city in the tth year, α represents the intercepts of all individuals, β1–β6 represents regression parameters for each independent variable, GDP represents the GDP per capita, TEC represents the level of technological innovation, and ϵ is the random error.
4. Results
4.1. Trends in urban dispersion and urban polycentricity
In the southeast region, the urban spatial structure is moving toward dispersion and polycentricity. Over the years, the median line for urban dispersion and urban polycentricity has been on the rise (). This implies that cities in the southeast region, in general, have experienced a dispersion of population and urban factors from the urban centers, along with improved equitable distribution of these factors between the main centers and subcenters. Our empirical results are similar to those of previous studies (Angel and Blei Citation2016).
As depicted in (a and b), the degree of urban dispersion in southeast China increased from 2012 to 2017 in most cities, which means more and more cities in this region moved toward a decentralized spatial structure, especially in the east of Guangxi Province and west of Fujian Province. These changes corresponded to the urban master plan introduced in the area. For example, Chizhou, a city in Anhui Province, experienced a rapid increase in urban dispersion as it has a particular focus on renowned tourist destinations in the ‘Chizhou City Master Plan (2009–2030).’ Chizhou has a natural landscape and human resources, especially in suburban areas, such as Jiuhua Mountain and Guliujiang. The development of these regions made them tourist centers and substantially increased urban dispersion. Furthermore, efficient communication technology facilitates interactions between central business districts (CBDs) and suburbs located farther from CBDs, which also accelerates urban dispersion (Arribas-Bel and Sanz-Gracia Citation2014; Tao, Sheng, and Wen Citation2023). However, a minority of cities are moving toward an aggregated urban spatial structure. These cities exhibit lower economic development levels compared to their southeast counterparts, which is consistent with the results from Wang (Citation2022). These cities usually struggle with limited land resources and insufficient infrastructure. In these cities, urban planners and developers tend to build more compact urban spatial structures to maximize land use efficiency (Ding Citation2013; Yan et al. Citation2021), and citizens prefer to live in urban centers for high accessibility to public services (Ojo Citation2020).
Figure 6. Spatial distribution of (a and b) urban dispersion and (c and d) polycentricity in 2012 and 2017. a(1), c(1), c(2), c(3), and c(4) represent Chizhou, Heyuan, Jieyang, Xiamen, and Suzhou, respectively.

Meanwhile, we found that polycentricity had an increasing trend similar to that of urban dispersion (c and d). First, more cities had a polycentricity value higher than 0, which meant the number of polycentric cities increased significantly from 2012 to 2017. Second, the urban centers in each city experienced a balanced development, according to the increment of the polycentricity value in most cities.
The formation of urban polycentricity is influenced by physical geography and human behavior (Krugman Citation1993). First, the topography of southeast China is complex and dominated by hills and mountains, making it impossible to concentrate the population in a single center and thus increasing the number of urban centers in the cities in this topography (e.g. Heyuan and Jieyang). Second, most cities in southeast China have high economic levels and population concentrations, making them experience agglomeration diseconomies. Therefore, these cities (e.g. Xiamen and Suzhou) tend to be polycentric to alleviate the pressure on the main center and weaken the agglomeration diseconomies (Sun and Lv Citation2020).
4.2. Effects of polycentricity and urban dispersion on total and subsectoral carbon dioxide emissions
We entered the indicators of 96 cities in southeast China between 2012 and 2017 as samples in a pooled regression model. First, we ensured that there was no colinearity between any two variables using the variance inflation factor (VIF) method and tolerance values (). Then, all variables were inputted into the pooled regression model, and the results showed that the total carbon emissions (OCEs) had the highest R2 value of 0.861 when fitting with urban spatial structures. For the carbon emissions of four subsectors (industry, power, civilian, and transportation), industrial carbon emissions (ICEs) and transportation carbon emissions (TCEs) had more important relationships with urban spatial structures (with R2 values of 0.800 and 0.768, respectively) than those of power carbon emissions (PCEs) and civilian carbon emissions (CCEs), whose R2 values were 0.418 and 0.467, respectively.
Table 1. Relations between urban spatial structures and carbon emissions.
As shown in , the degrees of both dispersion and polycentricity had an important negative effect on OCEs and TCEs. The degree of dispersion negatively impacted ICEs and CCEs, whereas the polycentricity level could considerably reduce PCEs. Specifically, a 1% increase in urban dispersion reduced OCEs by 0.085%, ICEs by 0.134%, CCEs by 0.126%, and TCEs by 0.049%. Simultaneously, a 1% increase in polycentricity can reduce OCEs by 0.064%, PCEs by 0.071%, and TCEs by 0.099%.
4.3. Impacts of city size on the correlation between urban spatial structure and carbon emissions
Owing to the differences in the development and size of cities in southeast China, the following question arises: do small and medium-sized cities require distinct spatial planning strategies to reduce carbon emissions compared to the larger cities? To answer this, a pooled regression model was adopted again excluding 11 large cities (such as Shanghai, Shenzhen, Xiamen, Ningbo, and six provincial capitals).
In contrast to the findings in , the urban spatial structure of the small and medium-sized cities affects the OCEs and TCEs differently. First, the polycentricity of small and medium-sized cities no longer exhibited a significant reduction in OCEs. Second, the negative effects of the dispersion and polycentricity on the TCEs within these cities were diminished.
4.4. Validation and robustness analysis
Verifying the capacity of NTL data in measuring urban spatial structure is imperative. We used traditional measurements (Mitchell-Weaver, Miller, and Deal Citation2000) as the reference and found that the urban dispersion and polycentricity degrees, derived from NTL data in this study, exhibited high accuracy. The R2 value of each year exceeded 0.8 and reached up to 0.976, as shown in . This validation affirms the viability of the NTL data as an alternative proxy for measuring urban spatial structure in terms of urban dispersion and polycentricity ().
Figure 7. Scatter plots of urban (a–f) dispersion and (g–l) polycentricity based on NTL data and traditional built-up area and urban center area from 2012 to 2017.

Table 2. Relations between urban spatial structures and carbon emissions in small and medium-sized cities.
In addition, considering potential time lags in the influence of urban spatial structure on carbon emissions and to mitigate potential endogeneity concerns (Cooper et al. Citation2020), urban dispersion and urban polycentricity degrees were lagged by one period. The results presented in reveal that the effects of urban dispersion and polycentricity on total and subsectoral carbon emissions align with those in , indicating the robustness of the results of this study.
Table 3. Relations between lag-phase urban spatial structure and carbon emissions.
5. Discussion
5.1. What type of urban spatial structure is conducive to reducing OCEs?
According to the results shown in , the higher the degree of dispersion and polycentricity, the lower the OCE let off. Specifically, a polycentric urban structure can accelerate the population migration from the main urban center to subcenters, which can considerably reduce the OCEs in the main center but slightly increase the carbon emissions in the subcenters (Sun, Han, and Li Citation2020). Because of decentralized urban spatial structures, more urban ecological corridors are created to speed up the diffusion of carbon emissions (Guo et al. Citation2007) and cause positive spatial spillovers on carbon emissions of its surrounding regions (Wu et al. Citation2023).
We also found that the impact of urban dispersion on total urban carbon emissions is much stronger than that of urban polycentricity, according to the coefficients of urban dispersion and polycentricity in model (1) (). A higher urban polycentricity means urban centers have a balanced development, which implies that each urban center has similar spillover effects on carbon emissions to surrounding urban centers. In other words, spillover effects are repulsive; subsequently, the reduction of carbon emissions of the polycentric structures seems weakened.
5.2. What type of urban spatial structure is conducive to reducing subsectoral carbon emissions?
Herein, we discussed the effects of urban spatial structures on four subsectoral carbon emissions. For instance, we revealed that decentralized urban spatial structures can considerably reduce ICEs and CCEs. There are two reasons for this finding. First, dispersed factories and settlements lead to dispersed sources of carbon emissions, facilitating the diffusion of carbon dioxide to other urban areas and reducing the local carbon dioxide concentration. Second, decentralized urban spatial structures are good for forming urban ecological corridors, which can help absorb considerable amounts of carbon dioxide (Guo et al. Citation2007) and introduce fresh air into urban interiors to reduce the concentration of carbon dioxide (Fan et al. Citation2022).
The results of model (3) in show that urban spatial structures cannot distinctly affect PCEs as its R2 of 0.418 is the lowest among those of all the models. However, we found that polycentric cities can have lower carbon emissions from power systems. Polycentric urban structure involves the emergence of new urban centers within the existing urban areas. The influx of talent often accompanies technological advancements, accelerating transformations in energy structures, such as the adoption of natural gas and solar energy (Chen et al. Citation2022; Junfeng et al. Citation2021). As usual, these new energy structures let off less carbon emissions than those of the traditional coal energy in old urban areas (Vazquez Hernandez, Gonzalez, and Fernandez-Blanco Citation2019). For example, the Lishui Urban Master Plan (2013–2030) clearly proposes the setting of Bihu and Dagangtou towns as subcenters, where the gasification rate must reach 90% in their gas engineering plan.
A decentralized and polycentric urban structure is beneficial for reducing TCEs. There are two main reasons for this. On the one hand, a decentralized urban structure can considerably alleviate traffic jams and consequently reduce exhaust emissions (Jung, Kang, and Kim Citation2022). Moreover, a polycentric urban structure implies that these subcenters are comparatively advanced, boasting well-established infrastructures. Consequently, such a spatial layout can lower the frequency of intercenter mobility. Moreover, because of the shorter commuting distances, residents tend to switch from private vehicles to public transportation (Ismiyati and Hermawan Citation2018). Therefore, polycentric urban spatial structures can considerably reduce TCEs. An empirical study conducted by Yang et al. (Citation2012) focused on the 50 largest metropolitan areas in the United States and found that the spatial distribution of high-density areas, similar to our urban centers, played a critical role in reducing commuting times while reducing the carbon emissions from transportation.
5.3. Optimal urban spatial structures for subsectoral carbon emissions reduction across different city sizes
First, the limited capacity of polycentricity in reducing OCE in small and medium-sized cities can be attributed to their insufficient population density. Polycentric urban spatial structures are effective in reducing carbon emissions within a certain population density range (Han, Sun, and Zhang Citation2020). Large cities generally exhibit high population density, particularly their main centers, which gathers a large number of people, ultimately causing polycentric urban structures to alleviate the concentration of pollution in the main center (Wu et al. Citation2022). In contrast, small and medium-sized cities feature low population densities, and their main centers lack strong agglomeration effects, diminishing the effects of polycentric urban structures on carbon emission reduction (Shi et al. Citation2023). Second, the reasons for the attenuation of the negative effects of decentralization and polycentricity on TCE in small and medium-sized cities differ. Traditionally, traffic congestion is less prevalent in small and medium-sized cities compared to large cities, limiting the potential for improved traffic conditions through urban decentralization. Therefore, urban decentralization exhibits a limited effect on TCEs. Moreover, the urban centers in small and medium-sized cities usually exhibit imbalances between job opportunities and housing, resulting in most routine commuting being intercenter (Burgalassi and Luzzati Citation2015), making it challenging for polycentricity to directly reduce TCEs in such regions.
5.4. Study limitations and future research directions
Although we have demonstrated that urban spatial structures can have important impacts on total urban carbon emissions and four subsectoral carbon emissions, there are still some limitations in this study. First, because the current carbon emission data in China did not cover recent years, we could not empirically illustrate how urban spatial structures currently affect carbon emissions. However, once we get sufficient carbon emission data, we can quickly make an empirical analysis as we have proposed an efficient framework. Second, this study only analyzed the impact of urban spatial structure on carbon emissions within a six-year period, without accounting for inter-annual variations, a topic that warrants future investigation. In addition, the effects of urban spatial structures on OCEs are diverse at different spatial scales (Shi et al. Citation2020) but the effects on subsectoral carbon emissions at county or region scales remain unknown.
6. Conclusion
This study quantified urban dispersion and urban polycentricity using the NTL data. Subsequently, a pooled regression analysis was performed on the samples from 2012 to 2017 in 96 cities in southeastern China to analyze how urban spatial structures impact carbon emissions, especially subsectoral carbon emissions, while considering the effects of city scale. From 2012 to 2017, we concluded that decentralized and polycentric structures benefit the reduction of OCEs and TCEs. A decentralized urban structure is conducive to reducing ICEs and CCEs, whereas a polycentric urban structure gives rise to lower PCEs. Moreover, we found that polycentricity in small and medium-sized cities exhibited no correlation with OCEs. Additionally, neither polycentricity nor decentralization in these cities influenced TCEs.
The relations between subsectoral carbon emissions and urban spatial structures can provide valuable insights for each city. First, for industrial cities, industries should be dispersed and the size of industrial parks should be limited. This strategy can better reduce ICEs. Second, for cities with good transportation, the infrastructure within each urban center should be well-designed and constructed. This can help reduce intercenter commuting and consequently cut TCEs.
Disclosure statement
No potential conflict of interest was reported by the author(s).
Data availability statement
The NPP-VIIRS-like NTL data are freely available and downloadable from https://doi.org/10.7910/DVN/YGIVCD. Carbon emission data were derived from the Multi-resolution Emission Inventory for China (MEIC) model.
Additional information
Funding
References
- Amindarbari, Reza, and Andres Sevtsuk. 2012. “Measuring Growth and Change in Metropolitan Form.” Sciences 104 (17): 7301–7306.
- Angel, Shlomo, and Alejandro M. Blei. 2016. “The Spatial Structure of American Cities: The Great Majority of Workplaces are no Longer in CBDs, Employment Sub-centers, or Live-Work Communities.” Cities 51: 21–35. https://doi.org/10.1016/j.cities.2015.11.031.
- Arribas-Bel, Daniel, and Fernando Sanz-Gracia. 2014. “The Validity of the Monocentric City Model in a Polycentric Age: US Metropolitan Areas in 1990, 2000 and 2010.” Urban Geography 35 (7): 980–997. https://doi.org/10.1080/02723638.2014.940693.
- Audretsch, David, and Maryann Feldman. 1996. “R-D Spillovers and the Geography of Innovation and Production.” American Economic Review 86: 630–640.
- Bereitschaft, Bradley, and Keith Debbage. 2013. “Urban Form, Air Pollution, and CO2 Emissions in Large U.S. Metropolitan Areas.” The Professional Geographer 65 (4): 612–635. https://doi.org/10.1080/00330124.2013.799991.
- Bramley, Glen, and Sinéad Power. 2009. “Urban Form and Social Sustainability: The Role of Density and Housing Type.” Environment and Planning B: Planning and Design 36 (1): 30–48. https://doi.org/10.1068/b33129.
- Burgalassi, David, and Tommaso Luzzati. 2015. “Urban Spatial Structure and Environmental Emissions: A Survey of the Literature and Some Empirical Evidence for Italian NUTS 3 Regions.” Cities 49: 134–148. https://doi.org/10.1016/j.cities.2015.07.008.
- Burger, Martijn, and Evert Meijers. 2010. “Spatial Structure and Productivity in US Metropolitan Areas.” Environment and Planning A: Economy and Space 42 (6): 1383–1402. https://doi.org/10.1068/a42151.
- Camagni, Roberto, and Roberta Capello. 2004. Urban Dynamics and Growth: Advances in Urban Economics. Contributions to Economic Analysis, edited by R. Capello and P. Nijkamp, 495–529. Amsterdam; Oxford: Elsevier
- Chen, Xi, and William Nordhaus. 2011. “Using Luminosity Data as a Proxy for Economic Statistics.” Proceedings of the National Academy of Sciences 108 (21): 8589–8594. https://doi.org/10.1073/pnas.1017031108
- Chen, Zuoqi, Bailang Yu, Yingjie Hu, Chang Huang, Kaifang Shi, and Jianping Wu. 2015. “Estimating House Vacancy Rate in Metropolitan Areas Using NPP-VIIRS Nighttime Light Composite Data.” IEEE Journal of Selected Topics in Applied Earth Observations and Remote Sensing 8 (5): 2188–2197. https://doi.org/10.1109/JSTARS.2015.2418201.
- Chen, Zuoqi, Bailang Yu, Yong Li, Qiusheng Wu, Bin Wu, Yan Huang, Siyuan Wu, et al. 2022. “Assessing the Potential and Utilization of Solar Energy at the Building-scale in Shanghai.” Sustainable Cities and Society 82: 103917. https://doi.org/10.1016/j.scs.2022.103917.
- Chen, Zuoqi, Bailang Yu, Wei Song, Hongxing Liu, Qiusheng Wu, Kaifang Shi, and Jianping Wu. 2017. “A New Approach for Detecting Urban Centers and Their Spatial Structure with Nighttime Light Remote Sensing.” IEEE Transactions on Geoscience and Remote Sensing 55: 6305–6319. https://doi.org/10.1109/TGRS.2017.2725917
- Chen, Zuoqi, Bailang Yu, Chengshu Yang, Yuyu Zhou, Shenjun Yao, Xingjian Qian, Congxiao Wang, Bin Wu, and Jianping Wu. 2021. “An Extended Time Series (2000–2018) of Global NPP-VIIRS-like Nighttime Light Data from a Cross-Sensor Calibration.” Earth System Science Data 13: 889–906. https://doi.org/10.5194/essd-13-889-2021.
- Chen, Zuoqi, Siyi Yu, Xiaojun You, Chengshu Yang, Congxiao Wang, Juan Lin, Wenting Wu, and Bailang Yu. 2023. “New Nighttime Light Landscape Metrics for Analyzing Urban-Rural Differentiation in Economic Development at Township: A Case Study of Fujian Province, China.” Applied Geography 150: 102841. https://doi.org/10.1016/j.apgeog.2022.102841.
- Cooper, Brian, Nathan Eva, Forough Zarea Fazlelahi, Alexander Newman, Allan Lee, and Martin Obschonka. 2020. “Addressing Common Method Variance and Endogeneity in Vocational Behavior Research: A Review of the Literature and Suggestions for Future Research.” Journal of Vocational Behavior 121, https://doi.org/10.1016/j.jvb.2020.103472.
- Ding, Chengri. 2013. “Building Height Restrictions, Land Development and Economic costs.” Land Use Policy 30 (1): 485–495. https://doi.org/10.1016/j.landusepol.2012.04.016.
- Duranton, Gilles, and Henry G. Overman. 2005. “Testing for Localization Using Micro-Geographic Data.” The Review of Economic Studies 72 (4): 1077–1106. https://doi.org/10.1111/0034-6527.00362.
- Ellison, Glenn, and Edward L. Glaeser. 1994. “Geographic Concentration in U.S. Manufacturing Industries: A Dartboard Approach.” Journal of Political Economy 105: 889–927. https://doi.org/10.1086/262098
- Elvidge, Christopher D, Kimberly E Baugh, Eric A Kihn, Herbert W Kroehl, and Ethan R Davis. 1997a. “Mapping City Lights with Nighttime Data from the DMSP Operational Linescan System.” Photogrammetric Engineering and Remote Sensing 63 (6): 727–734.
- Elvidge, Christopher D., Kimberly E. Baugh, Eric A. Kihn, Herbert W. Kroehl, Ethan R. Davis, and C. W. Davis. 1997b. “Relation Between Satellite Observed Visible-Near Infrared Emissions, Population, Economic Activity and Electric Power Consumption.” International Journal of Remote Sensing 18 (6): 1373–1379. https://doi.org/10.1080/014311697218485
- Fan, Mingyue, Zhaolin Gu, Weijun Li, Dian Zhou, and Chuck Wah Yu. 2022. “Integration of a Large Green Corridor with an Underground Complex – a Low Carbon Building Solution for Urban Climate Revival.” Indoor and Built Environment 31 (4): 872–877. https://doi.org/10.1177/1420326X211067607.
- Fang, Chuanglin, Shaojian Wang, and Guangdong Li. 2015. “Changing Urban Forms and Carbon Dioxide Emissions in China: A Case Study of 30 Provincial Capital Cities.” Applied Energy 158: 519–531. https://doi.org/10.1016/j.apenergy.2015.08.095
- Garreau, Joel. 1991. Edge City: Life on the New Frontier. New York: Doubleday.
- Glaeser, Edward L., and Matthew E. Kahn. 2010. “The Greenness of Cities: Carbon dioxide Emissions and Urban Development.” Journal of Urban Economics 67 (3): 404–418. https://doi.org/10.1016/j.jue.2009.11.006
- Glaeser, Edward L., Matthew E. Kahn, Richard Arnott, and Christopher Mayer. 2001. “Decentralized Employment and the Transformation of the American City.” Brookings-Wharton Papers on Urban Affairs, 1–63. https://doi.org/10.1353/urb.2001.0008.
- Green, Nick. 2007. “Functional Polycentricity: A Formal Definition in Terms of Social Network Analysis.” Urban Studies 44 (11): 2077–2103. https://doi.org/10.1080/00420980701518941.
- Guo, Rongchao, Changhong Miao, Xuexin Li, and Deguang Chen. 2007. “Eco-spatial Structure of Urban Agglomeration.” Chinese Geographical Science 17 (1): 28–33. https://doi.org/10.1007/s11769-007-0028-7.
- Han, Shuaishuai, Bindong Sun, and Tinglin Zhang. 2020. “Mono- and Polycentric Urban Spatial Structure and PM2.5 Concentrations: Regarding the Dependence on Population Density.” Habitat International 104, https://doi.org/10.1016/j.habitatint.2020.102257.
- Hanssens, Heidi, Ben Derudder, Stefan Van Aelst, and Frank Witlox. 2014. “Assessing the Functional Polycentricity of the Mega-City-Region of Central Belgium Based on Advanced Producer Service Transaction Links.” Regional Studies 48 (12): 1939–1953. https://doi.org/10.1080/00343404.2012.759650
- Hill, Richard. 1974. “Separate and Unequal: Governmental Inequality in the Metropolis.” American Political Science Review 68 (4): 1557–1568. https://doi.org/10.2307/1959941
- Hirschman, Albert O. 1964. “The Paternity of an Index.” The American Economic Review 54 (5): 761.
- Hsiao, Cheng. 2014. Analysis of Panel Data. 3 ed., Econometric Society Monographs. Cambridge: Cambridge University Press.
- Ismiyati, I., and F. Hermawan. 2018. “Lifestyle as an Influential Factor to Urban Mobility Transport: a Case Study of Semarang City, Indonesia.” IOP Conference Series: Earth and Environmental Science 123, https://doi.org/10.1088/1755-1315/123/1/012020.
- Junfeng, Wang, Nana Li, Mengdi Huang, Yue Zhao, and Yuanbo Qiao. 2021. “The Challenges of Rising Income on Urban Household Carbon Emission: Do Savings Matter?” Journal of Cleaner Production 326: 129295. https://doi.org/10.1016/j.jclepro.2021.129295.
- Jung, Meen Chel, Mingyu Kang, and Sunghwan Kim. 2022. “Does Polycentric Development Produce Less Transportation Carbon Emissions? Evidence from Urban Form Identified by Night-time lights Across US Metropolitan Areas.” Urban Climate 44, https://doi.org/10.1016/j.uclim.2022.101223.
- Krugman, Paul. 1993. “First Nature, Second Nature, and Metropolitan Location.” Journal of Regional Science 33 (2): 129–144. https://doi.org/10.1111/j.1467-9787.1993.tb00217.x.
- Lee, Bumsoo. 2007. ““Edge” or “Edgeless” Cities? Urban Spatial Structure in US Metropolitan Areas, 1980 to 2000.” Journal of Regional Science 47 (3): 479–515. https://doi.org/10.1111/j.1467-9787.2007.00517.x
- Lee, Sungwon, and Bumsoo Lee. 2014. “The Influence of Urban form on GHG Emissions in the U.S. Household Sector.” Energy Policy 68: 534–549. https://doi.org/10.1016/j.enpol.2014.01.024.
- Li, Yingcheng. 2020. “Towards Concentration and Decentralization: The Evolution of Urban Spatial Structure of Chinese Cities, 2001–2016.” Computers, Environment and Urban Systems 80, https://doi.org/10.1016/j.compenvurbsys.2019.101425.
- Li, Yingcheng, and Xingjian Liu. 2018. “How did Urban Polycentricity and Dispersion Affect Economic Productivity? A Case Study of 306 Chinese Cities.” Landscape and Urban Planning 173: 51–59. https://doi.org/10.1016/j.landurbplan.2018.01.007.
- Li, Meng, Huan Liu, Guannan Geng, Chaopeng Hong, Fei Liu, Yu Song, Dan Tong, et al. 2017. “Anthropogenic Emission Inventories in China: A Review.” National Science Review 4 (6): 834–866. https://doi.org/10.1093/nsr/nwx150.
- Li, Xi, Shanshan Liu, Michael Jendryke, Deren Li, and Chuanqing Wu. 2018a. “Night-Time Light Dynamics During the Iraqi Civil War.” Remote Sensing 10 (6), https://doi.org/10.3390/rs10060858.
- Li, Yingcheng, Weiting Xiong, and Xingping Wang. 2019. “Does Polycentric and Compact Development Alleviate Urban Traffic Congestion? A Case Study of 98 Chinese Cities.” Cities 88: 100–111. https://doi.org/10.1016/j.cities.2019.01.017.
- Li, Xi, Lixian Zhao, Wenjun Han, Bouarouri Faouzi, Prosper Washaya, Xubing Zhang, Huazhong Jin, and Chuanqing Wu. 2018b. “Evaluating Algeria’s Social and Economic Development Using a Series of Night-time Light Images Between 1992 to 2012.” International Journal of Remote Sensing 39 (23): 9228–9248. https://doi.org/10.1080/01431161.2018.1510560.
- Limtanakool, Narisra, Tim Schwanen, and Martin Dijst. 2009. “Developments in the Dutch Urban System on the Basis of Flows.” Regional Studies 43 (2): 179–196. https://doi.org/10.1080/00343400701808832.
- Liu, Xingjian, Ben Derudder, and Mingshu Wang. 2018. “Polycentric Urban Development in China: A Multi-scale Analysis.” Environment and Planning B: Urban Analytics and City Science 45: 953–972.
- Liu, Kai, Mingyue Xue, Mengjie Peng, and Chengxin Wang. 2020. “Impact of Spatial Structure of Urban Agglomeration on Carbon Emissions: An Analysis of the Shandong Peninsula, China.” Technological Forecasting and Social Change 161, https://doi.org/10.1016/j.techfore.2020.120313.
- Makido, Yasuyo, Shobhakar Dhakal, and Yoshiki Yamagata. 2012. “Relationship Between Urban Form and CO2 Emissions: Evidence from Fifty Japanese Cities.” Urban Climate 2: 55–67. https://doi.org/10.1016/j.uclim.2012.10.006.
- Mitchell-Weaver, Clyde, David Miller, and Ronald Deal. 2000. “Multilevel Governance and Metropolitan Regionalism in the USA.” Urban Studies 37 (5-6): 851–876. https://doi.org/10.1080/00420980050011127
- Ojo, A. E. 2020. “The Socio-economic Drivers of Public Infrastructures Development in Nigeria.” International Journal of Critical Infrastructures 16 (4): 328–341. https://doi.org/10.1504/IJCIS.2020.112063
- Panda, Sanjaya, and Prasanta Jana. 2015. “Efficient Task Scheduling Algorithms For Heterogeneous Multi-Cloud Environment.” The Journal of Supercomputing 71 (4): 1505–1533. https://doi.org/10.1007/s11227-014-1376-6.
- Sha, W., Y. Chen, J. Wu, and Z. Wang. 2020. “Will Polycentric Cities Cause More CO(2) Emissions? A Case Study of 232 Chinese Cities.” Journal of Environmental Sciences 96: 33–43. https://doi.org/10.1016/j.jes.2020.04.025.
- She, Qian-nan, Wen-xiao Jia, Chen Pan, Xi-yang Zhu, Fang Yang, M. Liu, and Wei-ning Xiang. 2015. “Spatial and Temporal Variation Characteristics of Urban Forms’ Impact on Regional Carbon Emissions in the Yangtze River Delta.” Chinese Journal of Population Resources and Environment 25: 44–51.
- Shi, Kaifang, Yun Chen, Bailang Yu, Tingbao Xu, Zuoqi Chen, Rui Liu, Linyi Li, and Jianping Wu. 2016. “Modeling Spatiotemporal CO2 (carbon dioxide) Emission Dynamics in China from DMSP-OLS Nighttime Stable Light Data Using Panel Data Analysis.” Applied Energy 168: 523–533. https://doi.org/10.1016/j.apenergy.2015.11.055.
- Shi, Kaifang, Guifen Liu, Yuanzheng Cui, and Yizhen Wu. 2023. “What Urban Spatial Structure is More Conducive to Reducing Carbon Emissions? A Conditional Effect of Population Size.” Applied Geography 151, https://doi.org/10.1016/j.apgeog.2022.102855.
- Shi, K., T. Xu, Y. Li, Z. Chen, W. Gong, J. Wu, and B. Yu. 2020. “Effects of Urban Forms on CO(2) Emissions in China from a Multi-perspective Analysis.” Journal of Environmental Management 262: 110300. https://doi.org/10.1016/j.jenvman.2020.110300.
- Shi, Kaifang, Qingyuan Yang, Guangliang Fang, Bailang Yu, Zuoqi Chen, Chengshu Yang, and Jianping Wu. 2018. “Evaluating Spatiotemporal Patterns of Urban Electricity Consumption Within Different Spatial Boundaries: A Case Study of Chongqing, China.” Energy 167, https://doi.org/10.1016/j.energy.2018.11.022.
- Shi, Kaifang, Bailang Yu, Yixiu Huang, Yingjie Hu, Bing Yin, Zuoqi Chen, Liujia Chen, and Jianping Wu. 2014. “Evaluating the Ability of NPP-VIIRS Nighttime Light Data to Estimate the Gross Domestic Product and the Electric Power Consumption of China at Multiple Scales: A Comparison with DMSP-OLS Data.” Remote Sensing 6 (2): 1705–1724. https://doi.org/10.3390/rs6021705.
- Shi, Kaifang, Bailang Yu, Yuyu Zhou, Yun Chen, Chengshu Yang, Zuoqi Chen, and Jianping Wu. 2019. “Spatiotemporal Variations of CO2 Emissions and their Impact Factors in China: A Comparative Analysis Between the Provincial and Prefectural Levels.” Applied Energy 233: 170–181. https://doi.org/10.1016/j.apenergy.2018.10.050.
- Sun, Bindong, Shuaishuai Han, and Wan Li. 2020. “Effects of the Polycentric Spatial Structures of Chinese City Regions on CO2 Concentrations.” Transportation Research Part D: Transport and Environment 82, https://doi.org/10.1016/j.trd.2020.102333.
- Sun, Tieshan, and Yongqiang Lv. 2020. “Employment Centers and Polycentric Spatial Development in Chinese Cities: A Multi-scale Analysis.” Cities 99, https://doi.org/10.1016/j.cities.2020.102617.
- Tao, Miaomiao, Mingyue Selena Sheng, and Le Wen. 2023. “How Does Financial Development Influence Carbon Emission Intensity in the OECD Countries: Some Insights from the Information and Communication Technology Perspective.” Journal of Environmental Management 335: 117553. https://doi.org/10.1016/j.jenvman.2023.117553.
- Vazquez Hernandez, C., J. Serrano Gonzalez, and R. Fernandez-Blanco. 2019. “New Method to Assess the Long-Term Role of Wind Energy Generation in Reduction of CO(2) Emissions - Case Study of the European Union.” Journal of Cleaner Production 207: 1099–1111. https://doi.org/10.1016/j.jclepro.2018.09.249.
- Wang, Hsi-Chuan. 2022. “Prioritizing compactness for a better quality of life: The case of U.S. cities.” Cities 123, https://doi.org/10.1016/j.cities.2022.103566.
- Wang, Shaojian, Chuanglin Fang, Haitao Ma, Yang Wang, and Jing Qin. 2014. “Spatial Differences and Multi-Mechanism of Carbon Footprint Based on GWR Model in Provincial China.” Journal of Geographical Sciences 24 (4): 612–630. https://doi.org/10.1007/s11442-014-1109-z.
- Wu, Yizhen, Chuanlong Li, Kaifang Shi, Shirao Liu, and Zhijian Chang. 2022. “Exploring the Effect of Urban Sprawl on Carbon Dioxide Emissions: An Urban Sprawl Model Analysis from Remotely Sensed Nighttime Light Data.” Environmental Impact Assessment Review 93, https://doi.org/10.1016/j.eiar.2021.106731.
- Wu, Yizhen, Kaifang Shi, Yuanzheng Cui, Shirao Liu, and Lili Liu. 2023. “Differentiated Effects of Morphological and Functional Polycentric Urban Spatial Structure on Carbon Emissions In China: An Empirical Analysis From Remotely Sensed Nighttime Light Approach.” International Journal of Digital Earth 16 (1): 532–551. https://doi.org/10.1080/17538947.2023.2176558.
- Yan, H., K. Wang, T. Lin, G. Q. Zhang, C. G. Sun, X. Y. Hu, and H. Ye. 2021. “The Challenge of the Urban Compact Form: Three-Dimensional Index Construction and Urban Land Surface Temperature Impacts.” Remote Sensing 13 (6), https://doi.org/10.3390/rs13061067.
- Yang, Jiawen, Steven French, James Holt, and Xingyou Zhang. 2012. “Measuring the Structure of U.S. Metropolitan Areas, 1970–2000.” Journal of the American Planning Association 78 (2): 197–209. https://doi.org/10.1080/01944363.2012.677382.
- Yang, Mian, Yaru Hou, Qiang Ji, and Dayong Zhang. 2020. “Assessment and Optimization of Provincial CO2 Emission Reduction Scheme in China: An Improved ZSG-DEA Approach.” Energy Economics 91: 104931. https://doi.org/10.1016/j.eneco.2020.104931.
- Yang, Chengshu, Bailang Yu, Zuoqi Chen, Wei Song, Yuyu Zhou, Xia Li, and Jianping Wu. 2019. “A Spatial-Socioeconomic Urban Development Status Curve from NPP-VIIRS Nighttime Light Data.” Remote Sensing 11 (20): 2398. https://doi.org/10.3390/rs11202398.
- You, X., and Z. Chen. 2022. “Interaction and Mediation Effects of Economic Growth and Innovation Performance on Carbon Emissions: Insights from 282 Chinese Cities.” Science of The Total Environment 831: 154910. https://doi.org/10.1016/j.scitotenv.2022.154910.
- Yu, Bailang, Kaifang Shi, Yingjie Hu, Chang Huang, Zuoqi Chen, and Jianping Wu. 2015. “Poverty Evaluation Using NPP-VIIRS Nighttime Light Composite Data at the County Level in China.” IEEE Journal of Selected Topics in Applied Earth Observations and Remote Sensing 8 (3): 1217–1229. https://doi.org/10.1109/JSTARS.2015.2399416.
- Zhang, Jingxiao, Weixing Jin, Guoliang Yang, Hui Li, Yongjian Ke, and Simon Philbin. 2021. “Optimizing Regional Allocation of CO2 Emissions Considering Output Under Overall Efficiency.” Socio-economic Planning Sciences 77: 101012. https://doi.org/10.1016/j.seps.2021.101012.
- Zhao, Min, Changxiu Cheng, Yuyu Zhou, Xuecao Li, Shi Shen, and Changqing Song. 2022. “A Global Dataset of Annual Urban Extents (1992–2020) From Harmonized Nighttime Lights.” Earth System Science Data 14 (2): 517–534. https://doi.org/10.5194/essd-14-517-2022.
- Zheng, Jiali, Zhifu Mi, D’Maris Coffman, Yuli Shan, Dabo Guan, and Shouyang Wang. 2019. “The Slowdown in China’s Carbon Emissions Growth in the New Phase of Economic Development.” One Earth 1 (2): 240–253. https://doi.org/10.1016/j.oneear.2019.10.007.
- Zheng, Bo, Dan Tong, Meng Li, Fei Liu, Chaopeng Hong, Guan-nan Geng, Haiyan Li, et al. 2018. “Trends in China’s Anthropogenic Emissions Since 2010 as the Consequence of Clean Air Actions.” Atmospheric Chemistry and Physics, 18 (19): 14095–14111.
- Zhu, Kai, Manya Tu, and Yingcheng Li. 2022. “Did Polycentric and Compact Structure Reduce Carbon Emissions? A Spatial Panel Data Analysis of 286 Chinese Cities from 2002 to 2019.” Land 11 (2): 185. https://doi.org/10.3390/land11020185