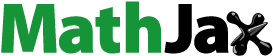
ABSTRACT
Armed conflicts in Africa have serious harmful impacts on human well-being. Existing evidence on whether economic aid (Official Finance, OF) reduced conflicts is diverse and contested. China has become one of the world’s largest overseas financiers. Taking the impacts of China’s OFs on conflict-related mortality risk (CRMR) as a case study is representative of addressing the issue. Combining the data of China’s OFs covering 52 African countries with armed conflict data from 2000 to 2017, we employ the instrumental variable and two-stage least squares estimation to explore the relationship between China’s OFs and CRMR. Our results indicate an 18% decrease (95% confidence interval (CI): 2–34%) in CRMR in a year after the OF commitment for a 1% increase in OF amounts. The OFs in social infrastructures (e.g. health, education and civil society) had greater positive impacts on the CRMR reduction than those in economic and production sectors. More pronounced effects of OFs are observed on small-scale state-based conflicts, in countries with low-level road infrastructures than their counterparts. Our findings give the references for policy-makers to optimize the pathway of the OFs for providing better relief to war-torn regions.
1. Introduction
Embroiled in conflicts in history (Alabi Citation2002), Africa is the most unstable and precarious continent in recent decades—an average of 28 conflicts per year since 1989 (Pettersson and Wallensteen Citation2015). Armed conflicts directly destroy physical environments and cause severe humanitarian disasters including injuries and deaths. They have been the leading concerns of food insecurity, poverty, and disruption of normal economic activities in Africa (Aremu Citation2010). To offset the large adverse socioeconomic consequences and alleviate hunger and suffering, substantial amounts of official finances (OFs), have been invested in Africa, especially the aid from the member countries of the Development Assistance Committee in the Organization for Economic Cooperation and Development (OECD-DAC) (Alesina and Dollar Citation2000; Loxley and Sackey Citation2008; Moss, Pettersson, and van de Walle Citation2006) and European Union (EU) (Bountagkidis, Fragkos, and Frangos Citation2015; Carbone Citation2013; Shiferaw and Hauck Citation2022). Research findings on any general, directional relationships existing between the OFs and armed conflicts are diverse and contested. Both positive and negative impacts of the OFs on conflicts have been documented, arising from multiple direct and indirect pathways. Several studies (Berman, Shapiro, and Felter Citation2008; Collier and Hoeffler Citation2002; Collier and Hoeffler Citation2004; Collier, Hoeffler, and Söderbom Citation2004; Crost, Felter, and Johnston Citation2014; Young and Findley Citation2011) suggest donor’s aid can be treated as a catalyst to promote economic growth, enhance attractiveness and solidarity of recipient government, bring tangible economic benefits to individuals, and improve local livelihoods, which safeguard the peace and stability of recipient countries. Negative impacts of the aid stem directly from the aid confiscations by armed groups, which exacerbate rather than defuse conflicts (Child Citation2019; Findley Citation2018; Nunn and Qian Citation2014), especially in the recipients with powerless governance, frequent corruption, and underdeveloped economies (Burnside and Dollar Citation1998; Nunn and Qian Citation2014). In that case, the limited aid may not be allocated to communities most in need (Nunn and Qian Citation2014; Roberts and Dionne Citation2013). To the best of our knowledge, the net effect of the OFs offered on armed conflicts in Africa has never been assessed over continental or multi-decadal scales.
A generalized, large-scale assessment of the effect of the OFs on armed conflicts in African countries is needed to help policy-makers to understand the contributions and disparities among assisted sectors, armed conflicts or countries, and develop practical mitigation strategies. In recent years, China has become one of the largest sources of global development finance (Ray et al. Citation2021), rehabilitating and developing large amounts of infrastructures in Africa through OFs (Bräutigam Citation2011). Taking the impacts of China’s OFs in Africa on conflict-related mortality risks (CRMR) as a case study is representative of addressing the above issues. The previous studies about the relationship between China’s OF and armed conflicts are contested (Banerjee, Duflo, and Qian Citation2020; Brautigam Citation2009; Dreher et al. Citation2016; Dreher et al. Citation2021; Gehring, Wong, and Kaplan Citation2018; Naim Citation2007; Onjala Citation2018), partly due to the unavailability of a comprehensive and high-resolution dataset of Chinese overseas development finance (AidData Citation2021; Dreher et al. Citation2021).
We have assembled China’s aid data from the AidData (AidData Citation2021), including 6,313 China’s OFs invested in 52 African countries from 2000 to 2017. The African armed conflict data come from Uppsala Conflict Data Program (UCDP), a reliable source used for many existing studies (Eck Citation2012; Halkia et al. Citation2020; Hegre et al. Citation2019; Hegre et al. Citation2021), recording 326,474 conflict deaths in the 52 African countries during 2000–2018. Based on the datasets, we investigate the effect of China’s OFs on CRMR in African recipients from 2000 to 2017. The main challenge in identifying the effects derives from reverse causality and joint determination (Nunn and Qian Citation2014). Moreover, there are concerns about dozens of potential confounders that affect both China’s OFs and conflicts, which would bias the estimates of the effect of China’s OFs. To overcome the challenges, we leverage the two-stage least square (2SLS) regression to conduct an instrumental variables (IVs) analysis (Dreher et al. Citation2021; Nunn and Qian Citation2014) for estimating the effect of China’s OFs on reducing CRMR in recipient countries. During the estimation, the country-fixed effects and year-fixed effects are employed to control for the time-invariant confounders specific to each recipient and change-over-time shocks which are common to all recipients (details are shown in Methods). Moreover, to confirm that the results are robust, we perform a battery of robustness checks to validate our results as plausible.
The roles of the OFs invested in different sectors and countries might not be evenly distributed across armed conflicts. The 2SLS regression combined with the interaction term is conducted to identify the heterogeneous impacts of the OFs according to assisted sectors, scales and types of conflicts, as well as the characteristics of recipient countries. We finally examine whether China’s OFs cause negative consequences to the recipients, for instance, whether they have fueled autocracy, aggravated corruption or debts, or crowded out the projects financed by the countries belonging to OECD-DAC, which some scholars care about (Abdenur Citation2014; Dong and Fan Citation2017; Naim Citation2007; Onjala Citation2018; Wang and Ozanne Citation2000; Zhao Citation2014).
Our findings provide insights that can better contextualize how China’s OFs enhance the capacity to defuse armed conflicts. They also give policy-makers reference to optimize the pathway of China’s OFs for investigating the local effects of China’s aid on different recipients, and offer targeted relief to war-torn regions.
2. Methods
2.1. Data on armed conflicts
UCDP is the main provider of data on violent conflicts, systematically defining armed conflict and setting a global standard for conflict study. We obtain armed conflict data from the dataset named Georeferenced Event Dataset Global version 21.1 (https://ucdp.uu.se/downloads/) in UCDP. The dataset covers records about 326,474 deaths from violent conflicts in 52 African countries from 2000 to 2018. UCDP defines three types of violent conflicts: state-based armed conflicts, non-state conflicts, and one-sided violence. State-based armed conflicts indicate a disputed incompatibility involving government and/or territory, in which the use of armed force between two parties, one of which belongs to at least one state. Non-state conflicts refer to the use of armed forces between two organized armed groups, neither of which belong to a country. One-sided violence refers to the use of armed force against civilians in a country by a civic society or a formally organized group.
In addition, we obtained conflict deaths from the Armed Conflict Location and Event Data Project (ACLED), recording 427,117 conflict deaths in Africa from 2000 to 2018. ACLED provides a wide range of conflict types (Raleigh, Kishi, and Linke Citation2023), but its requirements for actor inclusion are loose and actors sometimes cannot be identified (Eck Citation2012; Raleigh et al. Citation2010). In comparison, UCDP strictly defines what actors (a state or armed group) are eligible for inclusion in various conflict categories (Eck Citation2012). The explicit identification of conflict actors helps in discerning the mechanism through which OFs influence CRMR. We employed the UCDP dataset in the baseline IV model, and the ACLED dataset was utilized to verify the robustness of our estimations.
2.2. Data of global Chinese development finance
The dataset records the known overseas projects supported by more than 300 Chinese government institutions from 2000 to 2017, covering 6,313 OFs worth 468.2 billion USD invested in Africa (AidData Citation2021). In particular, the data also include military aid to the recipients. In our study, the amount of annual OFs to each recipient is selected as the independent variable of interest.
2.3. Data of international students from recipient countries to China (IV)
We obtain the annual number of international students from recipients to China based on the Statistics of International Students Coming to China (Department of International Cooperation and Exchanges Citation2017). The dataset records a total of 337,551 international students from 46 African countries from 2000 to 2017.
2.4. Data of DHS household
The household data used are collected from Demographic and Health Surveys (DHSs, https://www.dhsprogram.com/data/) conducted from 2006 to 2017, covering a total of 239,865 surveyed families and 1,392,429 family members in 15 sub-Saharan African countries. We obtain the variables of wealth index combined, level of household wall materials (natural, rudimentary, or finished), level of floor material (natural, rudimentary, or finished), time to get water source, as well as whether the household has electricity, television, refrigerator, and car or truck from DHS dataset.
2.5. Baseline IV model
We choose the number of international students from recipients to China as the IV and employ two-stage least squares (2SLS) to explore the effect of OFs on CRMR. To eliminate the unobserved confounders, we include both country-, year-fixed effects, and a series of controls in the model. The first-stage and reduced-form regression of 2SLS using IVs are shown as follows.
First stage:
(1)
(1) Reduced form:
(2)
(2) where
is the amount of China’s OFs committed in t-1 year for country i,
represents the number of students studying in China from the recipient country i in t-1 years.
denotes the conflict deaths in country i in year t. We adopt the transformations of
,
, and
to explore linear trends on the logarithmic scale and avoid taking the logarithm of zero.
is the vector of country-year controls (please refer to Supplementary Note 1 for a detailed description of the controls and their sources).
and
denote the country-fixed effects, and
and
indicate year-fixed effects.
and
are error terms.
is the coefficient of interest, representing the change in the CRMR for a 1% increase in OF amounts. Notably, we undertake the cluster-robust standard error in regressions and it is clustered at the country level.
2.6. Robustness checks
We conducted multiple analyses to strengthen the confidence in the robustness of our estimations. First, we assess model robustness across the specifications by controlling for different fixed effects, including country + year (baseline specification), country + region-by-year fixed effects, and country-fixed effects + year trend. We elaborate on these three additional specifications in Supplementary Method 1.
Second, we undertake an alternative specification by utilizing the inverse hyperbolic sine (arcsinh) transformation for the variables ,
and
in Equations (1)-(2). We compare the estimates from the alternative specification with those obtained from the baseline specification (using the logarithmic transformation).
Third, in response to the concern about changes in the estimated magnitude due to additional controls in the model, we compare the magnitudes and statistical significance of estimates before and after incorporating varying control variables in models, ranging from one to five. This comparison is also done across the four specifications aforementioned, including the baseline specification, two specifications including country + region by year-fixed effects and country-fixed effects + year trend, and the specification using arcsinh transformation. The five controls oil prices, amounts of military aids GDP per capita of China, drought severity in the 12 months in the year of OFs commitment, and recipient’s population. Therefore, considering combinations of different specifications and varying numbers of controls within each specification, we obtain a total of 20 regression estimates for comparison (4 specifications and 5 controls). To further address the concern of omitted variables, we introduced four additional controls about recipients (i.e. per capita GDP, unemployment rate, regimes, and corruption) in the model which already includes the five controls aforementioned, and examine if the model produces the robust estimates. The details about these four controls are listed in Supplementary Note 2.
Fourth, we also explored the impacts of OFs based on the conflict dataset from ACLED and compared the results with those obtained based on the UCDP dataset.
2.7. IV validation
For the IV to be valid, it should have a strong correlation with CRMR and satisfy the exclusion restriction. The necessary strong correlation is verified in the first-stage regressions (Kleibergen‒Paap F-statistics > 10). The exclusion restriction requires that the IV is independent of the confounders related to violent conflicts and only affects violent conflicts through the OFs. To verify the exclusion restriction of the IV is satisfied, we conduct a battery of exogeneity tests to examine whether the IV is uncorrelated with several confounders related to conflicts, i.e. international oil price, China per capita GDP, climatic factors in 12 months in the year of OFs commitment, recipients’ regime type, natural resource endowing, road density, GDP per capita, ethnic diversity, and proportion of the discriminated population (reflecting ethnic polarization). Additionally, the IV may be related to the amount of military aid and the recipient population. To address this concern, we control for these two variables in the IV model.
2.8. Heterogeneous effects across recipient countries
To explore the heterogeneity of China’s OFs in terms of several country characteristics of recipients, we include a binary dummy to construct the interaction term (also including direct effects) in the baseline IV model. This enables us to explore the heterogeneity brought by the corresponding characteristics, including ethnic issues (measured by ethnic diversity and proportion of the discriminated population), income level (measured by GDP per capita), natural resource endowment, democratization (measured by the democratic policy score), and road infrastructure level (measured by road density). Supplementary Note 3 provides details about the sources of these datasets. We defined the high-level countries (
) if the value that measures a recipient’s characteristic is above the mean of all recipients. Otherwise, they were taken as the low-level countries (
). With the addition of the interaction item, the reconstructed 2SLS model including first-stage and reduced-form regression is expressed as follows:
First stage:
(3)
(3) Reduced form:
(4)
(4) where
refers to a binary dummy variable. If this dummy variable is time-variant, we need to include the term
in Equations (3)–(4) which are not absorbed by fixed effects.
captures a differential effect on the OFs between the recipients with high-level country characteristics and their counterparts.
2.9. Effects of China’s OFs on OF amounts from other countries, recipients’ autocracy, corruption, and debt
To examine whether China’s OFs crowd out the OFs from other countries or facilitate autocracy (lower polity score), corruption, and debts in recipients, we take four indicators (measuring these items) as dependent variables and adopt the same regression specification as the baseline IVs model (with same independent variables, controls, and IVs included). The details about the used datasets are shown in Supplementary Note 4.
3. Results
3.1. Main results, robustness checks, and IV validation
As shown in (a), we obtain similar estimates from four specifications using different fixed effects and variable transformation, i.e. the specifications with country + year-fixed effects (baseline), country + region by year-fixed effects, country-fixed effects + year trend, and arcsinh transformations for variables. Moreover, the results with the inclusion of varying numbers (1 to 5) of controls in these four specifications show additional controls in the specification have little effect on the estimated magnitude. The estimate from baseline specifications with 5 controls indicates an 18% decrease in the CRMR a year after the OF commitment for every 1% increase of OF amounts (95% confidence interval (CI): 2-34%).
Figure 1. The estimated effects of OF amounts on CRMR and exogeneity checks of IV. a, Estimates from specifications with country + year fixed effects (FE), country + region-by-year fixed effects, country fixed effects + year trend, and arcsinh transformations for variables. We present five estimates (20 estimates in total) with the inclusion of varying (1 to 5) controls in each specification. The controls include oil prices (oil), amounts of military aid (military), GDP per capita of China (China GDP), drought severity in the 12 months in the year of OFs commitment (climate), and recipient’s population (population). Estimates are reported with standard errors clustered at the country level. Circles and lines denote the estimated effects of OFs and their 95% CIs. Kleibergen-paap F-statistics are listed in the estimates. b, P-values of estimates of the impact of IV on variables related to CRMR.

Moreover, as shown in Supplementary Figure 2a, we produce similar estimates when including four additional controls in the baseline IV model which already has 5 controls (oil + military + China GDP + climate + population). Similarly, replacing the UCDP dataset with the ACLED dataset seldom changes the estimated effects of OFs in terms of magnitudes and statistical significance (Supplementary Figure 2b).
For the validity of IV, all the Kleibergen‒Paap F-statistics displayed next to the estimates ((a)) are greater than 10, which verifies the strong relationship between IV and CRMR. Furthermore, the exclusion restriction of IV is satisfied due to the observed statistically nonsignificant impact of IV (P-values > 0.05) on the factors related to conflicts including oil price, China GDP, drought severity in 12 months in the year of OFs commitment, the proportion of the discriminated population, natural resource, democratic policy score, and road density.
3.2. Heterogeneity across assisted sectors
Disparate allocation of the OFs to different sectors might play heterogeneous roles in mitigating armed conflicts. Sector 1 is related to social infrastructure and services (e.g. education and health). OFs for Sector 2 are dedicated to economic infrastructure and services. Sector 3 pertains to production. Other sectors encompass a variety of contents, including multi-sector, debt, and humanitarian aid (please refer to Supplementary Table 2 for more details). As shown in (a), the OFs allocated to Sector 1 have a pronounced effect on CRMR, i.e. about a 27% decrease in CRMR (95% CI: 1-53%) with every 1% amount increase. In comparison, we do not find the significant impact of OFs from Sector 2, Sector 3, and other sectors on the CRMR (P > 0.05).
Figure 2. Heterogeneous effects of OFs across assisted sectors. a, Separate estimates of the OF impact on CRMR across sectors (Sector 1, Sector 2, Sector 3, and other sectors). b, Heterogeneity effects of OFs across supported sectors on the several household socioeconomic factors from DHSs. c, Average amounts per OFs (Million USD) by sectors and by types during 2000–2017. The circle and line denote the estimated effect of OFs and its 95% CI. d–g, The number of China’s OFs invested in Sectors 1, 2, 3 and other sectors (from d to g) of the African recipient countries during 2000–2017.
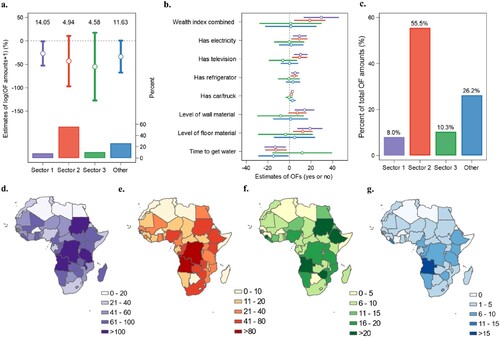
This significant effect from Sector 1 can be explained by the improvement of local well-being and socioeconomic status, which is a critical factor in reducing CRMR. As shown in (b), we find that DHS households within a 50 km distance to the location of Sector-1 OFs have a higher socioeconomic status compared to households located beyond 50 km. The household socioeconomic status is measured by the wealth index combined (an index that completely measures household wealth in DHS), access to electricity, ownership of a television and refrigerator, possession of a car or truck, the quality of wall and floor materials, and the time taken to reach a water source. While investing directly in economic infrastructure and services (Sector 2) can also enhance the socioeconomic status of households, its impact is smaller than Sector-1 OFs, particularly when considering the wealth index combined. Moreover, the amount of Sector-1 OFs takes up only 8% of the total amounts ((d)), but has more in the recipient countries. (d–g) illustrates the spatial distribution of OFs invested in different assisted sectors. We observed that OFs of all kinds of sectors tend to be invested in Central and East Africa (e.g. Angola). In particular, th largest numbers of Sector-1 OFs (>100) are observed in five recipients (Sudan, DR Congo, Angola, Tanzania, and Malawi).
3.3. Heterogeneity across armed conflicts
African recipients often experience armed conflicts with different types (i.e. state-based conflicts, non-state conflicts, and one-sided violence) and scales (large scale (≥1000 deaths in a year) and small scale (<1000 deaths in a year)), which may lead to the heterogenous impact of OFs. Due to the colinearity between CRMR and conflict scales, we use a new dependent variable, i.e. the incidence of conflicts with a specific type and scale. As shown in (a), we observe that OFs significantly only affect the incidence of small-scale state-based conflicts, which is decreased by 2% (95% CI: 0–3%) with an additional 1% amount of OFs. Moreover, there is no discernible influence of OFs on the incidence of conflicts with other types and scales.
Figure 3. Heterogeneity across armed conflict. a, Heterogeneous effect of OFs on the CRMR across armed conflict with different scales (i.e. state-based conflict, non-state conflict, and one-sided violence) and types (large-scale (conflict deaths ≥ 1000) and small-scale conflict (conflict deaths < 1000)). The circle and line denote the estimated effect of OFs and its 95% CI. b, The proportion of each type and scale of conflicts in all samples (including the sample without conflicts). c, Proportion of the number of OFs received by each type of receiving agency (defined by AidData). These types are broadly divided into government (purple colour) and other agencies (green colour). d-f, Annual death tolls led by the state-based conflict (d), non-state conflict (f), and one-sided violence (e) in African countries during 2000–2017.
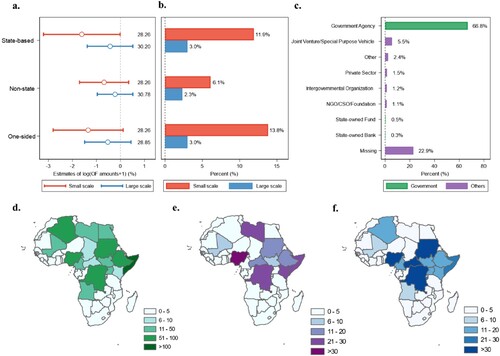
In terms of conflict types, state-based conflicts reflect how friendly between two nations or between a nation and an armed group. Our outcomes suggest OFs alleviate tensions between governments or foster trust between armed groups and the government. In comparison, we do not observe a discernible impact of OFs on non-state conflicts among armed groups (without government intervention) and one-sided violence targeting civilians. The possible explanation is that over 66% of the OFs are received by government agencies ((c)) and it may positively influence inter-government relations and the relations between government or armed groups.
Considering the conflict scale, our findings predominantly suggest that the involvement of OFs has a mitigating effect on small-scale conflicts, while its impact on larger conflicts remains limited. This is partly attributed to the smaller proportion of larger conflicts within the sample. Additionally, in regions characterized by uncontrolled turmoil and fragmentation, such as Somalia, Sudan, Ethiopia, DR Congo, and Syria ((d–f)), the improvements in livelihoods or economics brought about by OFs may face challenges in exerting significant influence.
3.4. Mechanism’s exploration and potential risks of OFs
To clarify what mechanism by which OFs reduce CRMR, we aim to identify what recipient characteristics can amplify the OFs’ effects on CRMR reduction. Here, we examined four variables, i.e. the proportion of discriminated populations (reflecting ethnic polarization), natural resource endowment, road density, and unemployment rate. (a) presents that road density significantly affects the impact of OFs on CRMR, and the effects of the other three factors are weak. Specifically, the countries with low-level road density (below the average road density) experience a 21% (95% CI: 3-39%) decrease in CRMR after receiving OFs. Countries with high-level road density show little impact on CRMR after receiving OFs. This suggests that China’s OFs can exert significant influence in countries with poor infrastructure. It also implies that the effect of OFs is likely manifested through the improvement of infrastructure, thereby reducing domestic vulnerability and diminishing CRMR. From (c–e), we observe that the substantial OFs are invested in Angola and Sudan which have a poor road infrastructure (although not the poorest). Nevertheless, many Western and Eastern African countries, including Niger, Burkina Faso, Somalia, Mauritania, and Senegal, exhibit low road density coupled with limited Chinese investments. Therefore, there is a need for more OFs in these countries to alleviate local vulnerabilities and diminish the likelihood of violent conflicts.
Figure 4. Heterogeneity across country characteristic of recipients. a, Effect of China’s OFs between recipients with high-level and low-level country characteristics. The circle and line denote the estimated effect of OFs and its 95% CI, and standard errors are clustered at the country level. b, P-values for estimated effects of OFs on the amounts of other official flows (from all OECD donors, USA, UK, Germany, and Japan), as well as on regime types (authoritarian or democratic), corruption, and debt of recipients in the year of and the lagged year of OFs commitment. c, Distribution of total OF amounts (million USD) in Africa from 2000 to 2017. d, Average road density of countries in Africa from 2000 to 2017. e, Bivariate choropleth maps combining two variables presented in c–d.
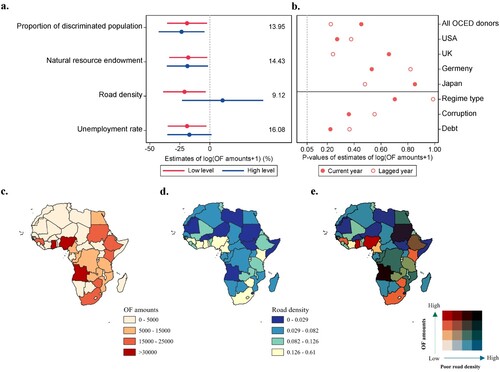
In addition, the substantial influx of OFs from China may pose potential risks to recipient countries, including crowding out the OFs from other donors or aggravating the recipients’ autocracy, corruption (measured by Corruption Perceptions Index), and debts. As shown in (b), there were no significant changes in the amounts of the OFs from other donators (i.e. all OFs from OECD countries and the individual OFs from the USA, UK, Germany, and Japan) as the recipients obtained China’s OFs, highlighting that the effect of China’s OFs in Africa did not crowd out the aids of other countries. Our outcomes also showed that there were little effects (P-values > 0.05) for China’s OFs on fuelling the autocracy, corruption, and debts of recipients in the year or the following year of OF commitments.
4. Discussion
Based on the datasets of comprehensive China’s OFs and armed conflicts in 46 African countries from 2000 to 2017, we have quantified the relationship between China’s OFs and CRMR reduction in African recipient countries. To address the concerns arising from potential biases, we employed the IV analysis combined with several robustness checks. Our outcomes highlight that China’s OFs are conductive in mitigating CRMR, and suggest that China’s OFs are a beneficial complement to Western economic aid (Humphrey and Michaelowa Citation2019). Despite marked contributions of the aid to averting CRMR from 2000 to 2017 across Africa, substantial differences persist among assisted sectors, armed conflicts, and countries, and the identification of these differences helps us to clarify the mechanism of OFs influencing CRMR.
The OFs invested in the sector related to social infrastructures (health, education, and civil society) make an outstanding contribution to mitigating CRMR compared with sectors related to economics and production. Consistent with the existing study (Young and Findley Citation2011), our finding highlights the importance of the aid related to people’s livelihoods, health and education in reducing CRMR. Many violent conflicts in Africa stem from inequality and poverty. Limited resources and inadequate infrastructure erode confidence in the governance capabilities of government, making individuals susceptible to militant ideologies and recruitments. Improvements in social infrastructure have a pronounced and direct impact on rebuilding government credibility and fostering the domestic populace. Our household-level results from DHS indicate that OFs for social infrastructure significantly influence socioeconomic status and household wealth compared to direct economic and production investment (i.e. Sector 2 and Sector 3). The financial assistance directed to economic growth in African recipients is usually unavailable for disadvantaged populations (Nunn and Qian Citation2014; Roberts and Dionne Citation2013), especially in recipients with serious social inequalities and frequent corruption (Burnside and Dollar Citation1998). The findings emphasize that some African countries cannot keep regional stabilities only by focusing on economic growth or production without improving human development. If the sustainable growths in African countries are to be accelerated, policies or assistance to improve human development should precede or at least complement economic-growth-oriented policies (Guillon and Mathonnat Citation2020; Lindahl and Krueger Citation2001; Suri et al. Citation2011).
Furthermore, our findings indicate that OF investments mitigate state-based conflicts (one of the parties belongs to at least one state or government). This is because China’s OFs predominantly are received by government agencies, and these investments probably contribute to fostering the trust of armed groups or another country towards the recipient’s government. Notably, we observed that in countries with weak infrastructure, the impact of CRMR is particularly discernible. This suggests that China’s OFs play a pivotal role in addressing vulnerabilities within national infrastructure.
Our results also exclude some potential risks associated with OFs, as mentioned in prior studies, including the rise of authoritarianism (Jackson and Rosberg Citation1985), corruption (Gnangnon Citation2021), and increased debt (Djimeu Citation2018) in recipient countries. This exclusion of risks may be due to China’s tendency to invest aid in regions with great stability and manageability. Additionally, China often dispatches personnel to assist in infrastructure construction and the implementation of OFs, reducing the risk of fund misappropriation.
The above findings have important implications for targeted aid to mitigate entrenched armed conflicts in Africa. They offer scientific evidence for proactive planning and management to address the concerns about social security and stability of the recipients, and unlock the potential of China’s overseas development finance for sustainable development in Africa (Yang et al. Citation2021).
We acknowledge several limitations in this study. First, no evidence from our results supports that China’s OFs worsen the governance of the recipients, namely, not having made them more authoritarian, corrupt or indebted. However, these are macro-evaluations from the country level, and the specific effects of China’s aid on local governance require more county- or state-scale indicators. Second, some records in a part of China’s OFs are missing in the AidData, such as the amount paid, actual starting date and ending date, which may inevitably cause estimation biases. Additionally, the CRMR may be underestimated because the conflicts that occurred in remote areas might be undocumented according to the report of UCDP (Pettersson and Öberg Citation2021).
Supplemental Material
Download MS Word (1.7 MB)Disclosure statement
No potential conflict of interest was reported by the authors.
Data availability statement
The data that support the findings of this study are available from the corresponding author upon request.
Additional information
Funding
References
- Abdenur, Adriana Erthal. 2014. “China and the BRICS Development Bank: Legitimacy and Multilateralism in South–South Cooperation.” IDS Bulletin 45 (4): 85–101. https://doi.org/10.1111/1759-5436.12095.
- AidData. 2021. “China’s Public Diplomacy Dashboard Dataset, Version 2.0.” http://china-dashboard.aiddata.org.
- Alabi, David T. 2002. “Emerging Trends and Dimensions of the Rwandan Crisis.” African Journal of International Affairs and Development 7 (1): 40–65.
- Alesina, Alberto, and David Dollar. 2000. “Who Gives Foreign Aid to Whom and Why?” Journal of Economic Growth 5 (1): 33–63. https://doi.org/10.1023/A:1009874203400.
- Aremu, Johnson Olaosebikan. 2010. “Conflicts in Africa: Meaning, Causes, Impact and Solution.” African Research Review 4 (4): 549–560.
- Banerjee, Abhijit, Esther Duflo, and Nancy Qian. 2020. “On the Road: Access to Transportation Infrastructure and Economic Growth in China.” Journal of Development Economics 145: 102442. https://doi.org/10.1016/j.jdeveco.2020.102442.
- Berman, Eli, Jacob N Shapiro, and Joseph H Felter. 2008. “Can Hearts and Minds Be Bought? The Economics of Counterinsurgency in Iraq.” National Bureau of Economic Research Working Paper Series. Vol. No. 14606. https://doi.org/10.3386/w14606.
- Bountagkidis, Georgios K, Konstantinos C Fragkos, and Christos C Frangos. 2015. “EU Development Aid Towards Sub-Saharan Africa: Exploring the Normative Principle.” Social Sciences 4 (1): 85–116. https://doi.org/10.3390/socsci4010085.
- Brautigam, Deborah. 2009. The Dragon’s Gift: The Real Story of China in Africa. Oxford: Oxford University Press.
- Bräutigam, Deborah. 2011. “Aid ‘With Chinese Characteristics’: Chinese Foreign Aid and Development Finance Meet the OECD-DAC Aid Regime.” Journal of International Development 23 (5): 752–764. https://doi.org/10.1002/jid.1798.
- Burnside, Craig, and David Dollar. 1998. Aid, the Incentive Regime, and Poverty Reduction. Washington DC: World Bank Publications.
- Carbone, Maurizio. 2013. “Between EU Actorness and Aid Effectiveness: The Logics of EU Aid to Sub-Saharan Africa.” International Relations 27 (3): 341–355. https://doi.org/10.1177/0047117813497300.
- Child, Travers Barclay. 2019. “Conflict and Counterinsurgency Aid: Drawing Sectoral Distinctions.” Journal of Development Economics 141: 102245. https://doi.org/10.1016/j.jdeveco.2018.06.003.
- Collier, Paul, and Anke Hoeffler. 2002. “AID, Policy and Peace: Reducing the Risks of Civil Conflict.” Defence and Peace Economics 13 (6): 435–450. https://doi.org/10.1080/10242690214335.
- Collier, Paul, and Anke Hoeffler. 2004. “Aid, Policy and Growth in Post-Conflict Societies.” European Economic Review 48 (5): 1125–1145. https://doi.org/10.1016/j.euroecorev.2003.11.005.
- Collier, Paul, Anke Hoeffler, and Måns Söderbom. 2004. “On the Duration of Civil War.” Journal of Peace Research 41 (3): 253–273. https://doi.org/10.1177/0022343304043769.
- Crost, Benjamin, Joseph Felter, and Patrick Johnston. 2014. “Aid Under Fire: Development Projects and Civil Conflict.” American Economic Review 104 (6): 1833–1856. https://doi.org/10.1257/aer.104.6.1833.
- Department of International Cooperation and Exchanges. 2017. Statistics of International Students Coming to China. Beijing: Ministry of Education.
- Djimeu, Eric W. 2018. “The Impact of the Heavily Indebted Poor Countries Initiative on Growth and Investment in Africa.” World Development 104: 108–127. https://doi.org/10.1016/j.worlddev.2017.11.002.
- Dong, Yan, and Cijun Fan. 2017. “The Effects of China’s Aid and Trade on Its ODI in African Countries.” Emerging Markets Review 33: 1–18. https://doi.org/10.1016/j.ememar.2017.09.003.
- Dreher, Axel, Andreas Fuchs, Roland Hodler, Bradley Parks, Paul A. Raschky, and Michael J. Tierney. 2016. “Aid on Demand: African Leaders and the Geography of China’s Foreign Assistance.” Centro Studi Luca d’Agliano Development Studies Working Paper No. 400. https://doi.org/10.2139/ssrn.2900351.
- Dreher, Axel, Andreas Fuchs, Bradley Parks, Austin Strange, and Michael J Tierney. 2021. “Aid, China, and Growth: Evidence from a New Global Development Finance Dataset.” American Economic Journal: Economic Policy 13 (2): 135–174. https://doi.org/10.1257/pol.20180631.
- Eck, Kristine. 2012. “In Data We Trust? A Comparison of UCDP GED and ACLED Conflict Events Datasets.” Cooperation and Conflict 47 (1): 124–141. https://doi.org/10.1177/0010836711434463. http://www.jstor.org/stable/45084688.
- Findley, Michael G. 2018. “Does Foreign Aid Build Peace?” Annual Review of Political Science 21 (1): 359–384. https://doi.org/10.1146/annurev-polisci-041916-015516.
- Gehring, Kai S, Melvin H L Wong, and Lennart Kaplan. 2018. Aid and Conflict at the Subnational Level: Evidence from World Bank and Chinese Development Projects in Africa. Heidelberg: University of Heidelberg, Department of Economics. http://hdl.handle.net/10419/207635.
- Gnangnon, Sèna Kimm. 2021. “Aid for Trade and Inflation: Exploring the Trade Openness, Export Product Diversification and Foreign Direct Investment Channels.” Australian Economic Papers 60 (4): 563–593. https://doi.org/10.1111/1467-8454.12219.
- Guillon, Marlène, and Jacky Mathonnat. 2020. “What Can We Learn on Chinese Aid Allocation Motivations from Available Data? A Sectorial Analysis of Chinese Aid to African Countries.” China Economic Review 60: 101265. https://doi.org/10.1016/j.chieco.2019.01.004.
- Halkia, Matina, Stefano Ferri, Marie K Schellens, Michail Papazoglou, and Dimitrios Thomakos. 2020. “The Global Conflict Risk Index: A Quantitative Tool for Policy Support on Conflict Prevention.” Progress in Disaster Science 6: 100069. https://doi.org/10.1016/j.pdisas.2020.100069.
- Hegre, Håvard, Marie Allansson, Matthias Basedau, Michael Colaresi, Mihai Croicu, Hanne Fjelde, Frederick Hoyles, et al. 2019. “ViEWS: A Political Violence Early-Warning System.” Journal of Peace Research 56 (2): 155–174. https://doi.org/10.1177/0022343319823860.
- Hegre, Håvard, Curtis Bell, Michael Colaresi, Mihai Croicu, Frederick Hoyles, Remco Jansen, Maxine Ria Leis, et al. 2021. “ViEWS2020: Revising and Evaluating the ViEWS Political Violence Early-Warning System.” Journal of Peace Research 58 (3): 599–611. https://doi.org/10.1177/0022343320962157.
- Humphrey, Chris, and Katharina Michaelowa. 2019. “China in Africa: Competition for Traditional Development Finance Institutions?” World Development 120: 15–28. https://doi.org/10.1016/j.worlddev.2019.03.014.
- Jackson, Robert H, and Carl G Rosberg. 1985. “Democracy in Tropical Africa: Democracy Versus Autocracy in African Politics.” Journal of International Affairs 38 (2): 293–305. http://www.jstor.org/stable/24356915.
- Lindahl, Mikael, and Alan B Krueger. 2001. “Education for Growth: Why and for Whom?” Journal of Economic Literature 39 (4): 1101–1136. https://doi.org/10.1257/jel.39.4.1101
- Loxley, John, and Harry A Sackey. 2008. “Aid Effectiveness in Africa.” African Development Review 20 (2): 163–199. https://doi.org/10.1111/j.1467-8268.2008.00181.x.
- Moss, Todd J., Gunilla Pettersson, and Nicolas van de Walle. 2006. “An Aid-Institutions Paradox? A Review Essay on Aid Dependency and State Building in Sub-Saharan Africa.” Center for Global Development Working Paper. https://doi.org/10.2139/ssrn.860826.
- Naim, Moises. 2007. “Rogue Aid.” Foreign Policy 159: 96.
- Nunn, Nathan, and Nancy Qian. 2014. “US Food Aid and Civil Conflict.” American Economic Review 104 (6): 1630–1666. https://doi.org/10.1257/aer.104.6.1630.
- Onjala, Joseph. 2018. “China’s Development Loans and the Threat of Debt Crisis in Kenya.” Development Policy Review 36 (S2): O710–O728. https://doi.org/10.1111/dpr.12328.
- Pettersson, Therése, and Magnus Öberg. 2021. “Organized Violence, 1989–2020.” Journal of Peace Research 58 (4): 597–613. https://doi.org/10.1177/0022343320934986.
- Pettersson, Therése, and Peter Wallensteen. 2015. “Armed Conflicts, 1946–2014.” Journal of Peace Research 52 (4): 536–550. https://doi.org/10.1177/0022343315595927.
- Raleigh, Clionadh, Roudabeh Kishi, and Andrew Linke. 2023. “Political Instability Patterns Are Obscured by Conflict Dataset Scope Conditions, Sources, and Coding Choices.” Humanities and Social Sciences Communications 10 (1): 74. https://doi.org/10.1057/s41599-023-01559-4.
- Raleigh, Clionadh, Rew Linke, Håvard Hegre, and Joakim Karlsen. 2010. “Introducing ACLED: An Armed Conflict Location and Event Dataset.” Journal of Peace Research 47 (5): 651–660. https://doi.org/10.1177/0022343310378914.
- Ray, Rebecca, Kevin P Gallagher, William Kring, Joshua Pitts, and B. Alexander Simmons. 2021. “Geolocated Dataset of Chinese Overseas Development Finance.” Scientific Data 8 (1): 241. https://doi.org/10.1038/s41597-021-01021-7.
- Roberts, Tyson, and Kim Yi Dionne. 2013. “Aid Effectiveness and Allocation: Evidence from Malawi.” In ASA 2013 Annual Meeting Paper.
- Shiferaw, Lidet Tadesse, and Volker Hauck. 2022. “The War in Ukraine: Implications for the Africa-Europe Peace and Security Partnership.” The Strategic Review for Southern Africa 44 (1 SE-Special Focus: Africa and the war in Europe): 93–109. https://doi.org/10.35293/srsa.v44i1.4071.
- Suri, Tavneet, Michael A Boozer, Gustav Ranis, and Frances Stewart. 2011. “Paths to Success: The Relationship Between Human Development and Economic Growth.” World Development 39 (4): 506–522. https://doi.org/10.1016/j.worlddev.2010.08.020.
- Wang, Xiaobing, and Adam Ozanne. 2000. “Two Approaches to Aid in Africa: China and the West.” In Prepared for the International Conference “Ten Years Of ‘War Against Poverty’: What Have We Learned Since, 2010–2020.
- Yang, Hongbo, B. Alexander Simmons, Rebecca Ray, Christoph Nolte, Suchi Gopal, Yaxiong Ma, Xinyue Ma, and Kevin P Gallagher. 2021. “Risks to Global Biodiversity and Indigenous Lands from China’s Overseas Development Finance.” Nature Ecology & Evolution 5 (11): 1520–1529. https://doi.org/10.1038/s41559-021-01541-w.
- Young, Joseph K, and Michael G Findley. 2011. “Can Peace Be Purchased? A Sectoral-Level Analysis of Aid’s Influence on Transnational Terrorism.” Public Choice 149 (3-4): 365. https://doi.org/10.1007/s11127-011-9875-y.
- Zhao, Suisheng. 2014. “A Neo-Colonialist Predator or Development Partner? China’s Engagement and Rebalance in Africa.” Journal of Contemporary China 23 (90): 1033–1052. https://doi.org/10.1080/10670564.2014.898893.