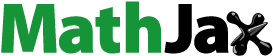
Abstract
Mining, an activity that dates to the earliest stages of human civilization, has played a vital role in fulfilling human requirements for an extended period of time. Nevertheless, it is worth noting that this particular activity accounts for a significant proportion, ranging from 4% to 7% of the overall world greenhouse gas emissions. These emissions mostly consist of carbon dioxide, methane, and nitrogen oxides, which are released because of fuel burning, on-site energy generation, and many other sources. The mining industry, as a constituent of industrial process and product use (IPPU) sector in Thailand, makes a substantial contribution to these emissions. Thailand is now engaged in the implementation of carbon sequestration strategies, specifically focusing on the reclamation and replanting of post-mining zones. This initiative aims to tackle the various issues associated with small-scale operations within the country’s mining sector. The objective of this study is to analyse the carbon sequestration potential of Thailand’s mining area through the utilization of satellite pictures obtained from LANDSAT 8 OLI and Geographic Information System (GIS) applications. This study assesses the present carbon storage capacity, establishes the appropriate carbon pricing, and investigates the potential for reforestation in mining areas inside the nation. Based on the findings, it is evident that the northern region of Thailand has the most substantial potential for carbon sequestration, mostly due to the presence of vegetated land. Furthermore, it is projected that the overall carbon sequestration capacity in Thailand will amount to 14.68 MtCO2e in 2023 and 28.02 MtCO2e in 2030.
Subject classification codes:
Introduction
Mining has played a crucial role in human history, but it also has a substantial impact on global greenhouse gas emissions [Citation1]. The metals and mining industry contributes between 4% and 7% of the overall world emissions of greenhouse gases (GHGs), emitting carbon dioxide, methane, and nitrogen oxides into the environment. Thailand is presently facing the repercussions of climate change, such as the escalation of sea levels and the detrimental effects on coastal areas and marine ecosystems. The nation’s carbon emissions have been consistently rising since the early 2000s, with the energy industry being the main source. From 2000 to 2018, there was a 55.88% increase in emissions, resulting in a total of 257.34 MtCO2eq. In 2010, the industrial process and product usage sectors contributed 10.74% of the total emissions. This sector experienced a growth rate of 2.12%. The mining industry significantly contributes to Thailand’s economic growth within this sector. The industry sector released a combined 40,118.18 gigagrams of carbon dioxide equivalent (GgCO2e) in 2018, with mining accounting for the majority of emissions at 51.28%. The main source of these emissions was the production of cement and aggregate, which contributed 19.36 MtCO2e. On the whole, mining in Thailand has a significant influence on the release of greenhouse gases, underscoring the necessity of implementing sustainable practices within the industry.
In the context of Thailand, the most viable approach entails the implementation of carbon sequestration via the reclamation and reforestation of post-mining regions. This is primarily due to the prevailing characteristics of the Thai mining sector, which predominantly consists of small-scale operations situated in remote areas. These types of mining operations constitute over 50% of cement material and aggregate production in the country [Citation2].
Forest productivity refers to the capacity of forests to mitigate CO2 emissions in the atmosphere by absorbing and storing CO2 in their organs through physiological processes. This method can stimulate the growth of the forest area over time. Therefore, monitoring the increased biomass content can be used to assess the efficiency of reforestation and reclamation efforts [3, 4]. The new post-mining ecosystems (PME) in the reclamation area can accumulate carbon in plants, soil organic matter (SOM), and other litter [Citation5] and have been widely reported to have significant potential for raising net carbon sequestration [6, 7].
Modern remote sensing approaches and integrated spatial analysis play an essential role in utilizing essential information such as topography, area, and current vegetation to support the improvement of carbon sequestration management in PMEs. The tools possess the capability to assess the degree of vegetation restoration at mining sites, thereby presenting a significant opportunity to enhance comprehension of mine site reclamation and its potential for sequestering carbon. In recent decades, there has been a growing utilization of remote sensing techniques to identify carbon sequestration and reforestation in mining areas [Citation8].
The study of [9, 10] illustrates how an integrated spatial analysis utilizing LIDAR and LANDSAT data can be utilized to estimate the dynamics of forest biomass over a mining region in Canada. The findings indicate that the approach successfully illustrates the tangible growth of biomass throughout the reforestation region. To investigate the coordination between vegetation structure and carbon sequestration function, ascertain the direction of network optimization, and propose optimization solutions. Ran et al. [Citation11] developed a topology and function coupling optimization model (TFCO). The findings illustrate the potential of this integrated approach in identifying the precise region along the Yellow River in China where substantial carbon sequestration can be improved.
Additionally, this integrated method can also be applied as an important tool in the estimation of carbon sequestration capacity in various reforestation regions, including the post-mining area. These methods are generally conducted using multispectral satellite image analysis and indices. According to Malik et al. [12], the estimation of above-ground biomass (AGB) based on the analysis using the normalized difference vegetation index (NDVI) in one specific area can be accomplished by various degrees of equations. The results demonstrate that the relationships from regression analysis, including linear, exponential, and polynomial equations, have a strong correlation with R2, ranging from 0.749 to 0.765.
Uttha et al. [Citation13] assess the AGB with measurements from the sampling plot using multiple linear regression analysis, which has the normalized difference vegetation index (NDVI), soil-adjusted vegetation index (SAVI), and fractional vegetation cover (FVC). The determined R2 for regression evaluation was 0.85, and the estimated AGB was 1,055.54 ktCO2e in the study area.
Since the application of remote sensing is applicable, numerous studies have been conducted to determine the carbon sequestration potential and ecological relationship in the PMEs area [Citation14–17]. Thiteja et al. [Citation18] monitored the carbon storage of plantation forests in the PME area in the Northern Region of Thailand using a spatial regression model. The results indicate that the above-ground carbon storage (AGC) in biomass has increased to 75.55% of adjacent natural forest after 18 years of reforestation. Yang et al. [Citation14] combined field data and remote sensing techniques to create field data-driven maps of the tree-level landscape and determined that carbon storage rises in the initial/stable stages and decreases in the development stage during mining expansion and reclamation. The choice of restoration tree species significantly influenced carbon storage.
This study is conducted to illustrate the potential carbon sequestration of the whole Thai mining area based on the capability of this integrated spatial technique. The satellite image of Thailand from LANDSAT 8 OLI is assigned and analysed for gathering the NDVI value. The mining area was extracted from the land use amp of the Land Development Department of Thailand (LDD) using a GIS application. These areas are further estimated to determine their current baseline of carbon storage capacity using selected appropriate equations from relative standard deviation analysis (RSD) [Citation19]. Finally, the capacities are analysed to determine the carbon price and opportunities for mine reforestation in Thailand.
Methodology
The comprehensive methodology of this study is illustrated in . The study commences with a review of the relevant literature in order to establish an estimation formula suitable for spatial analysis over the mine in Thailand, covering both above-ground Biomass (AGB) and above-ground Carbon-stock (AGC). The selected equation is primarily determined by its proximity to Thailand’s mine-reforestation in terms of topography, location, and ecological characteristics. The GIS application assigns these equations in accordance with the results of the NDVI analysis using LANDSAT 8 OLI imagery captured during the vegetation culmination over mine -reforestation in Thailand (July–August) [Citation20]. The spatial precisions of the outcomes derived from the spatial analysis is subsequently assessed through the application of zonal spatial statistics. The essential formula for calculating AGC over the reforestation of the mine in Thailand is chosen based on the formula with the highest degree of precision. An in-depth analysis is conducted on the potential carbon sequestration of the mines, which are categorized by region, to assess their economic potential and propose the contribution of Thailand’s mining industry to the mitigation of domestic carbon emissions.
Site description
demonstrates the map of Thailand. It is situated in a tropical region of Southeast Asia bounded by longitudes E 7°22′ to E 105°37′ and latitudes N 5°37′ to N 20°27′, with a total area of 513,115 km2. According to the LDD, the land use in Thailand can be divided into six regions. The northern region is distinguished by mountainous and undulating terrain. The central region is a vast, low-lying plain with mountainous and rugged terrain, whereas the north-eastern region is a naturally elevated plain. The eastern region consists primarily of valleys and plains, with a few minor peaks. The southern region is a peninsula bordered on the west by the Andaman Sea and on the east by the South China Sea. Finally, the western region consists primarily of mountainous and rugged terrain. According to the classification, the mineral resources and industries of Thailand should be varied according to the differences in topographic and geological depositions; however, most of the mines that exist are aggregate materials for the cement and construction industries.
Data acquisition and preparation
The essential data for the estimation of carbon sequestration potential of Thai mine-reforestation area are gathered according to . These data are prepared and constructed as the input for further analysis in GIS application. The satellite images in this study area were acquired during the peak period of vegetation growth in Thailand (July–August) and featured a cloud coverage of less than 20% to minimize interferences in the calculation. The scenes of these images were captured, covering the entire territories of the coordinates zone WGS 84/UTM zone 47 N and WGS 84/UTM zone 48 N which can be representative of the whole territories of Thailand. The images were assigned for preprocessing, following the method of [21, 22] to reduce the errors from noise and defects associated with the sensors as well as improve feature identification for further analysis.
Table 1. Sources of data in this study.
Satellite image analysis
Since the estimation of carbon sequestration for the whole region of Thailand must be based on remote sensing and spatial analysis techniques,. The mutual basis of input must be considered. In this study, the Normalized difference vegetation index (NDVI), which is commonly used in monitoring of forestry and vegetation, are assigned and can be calculated using satellite images based on EquationEquation (1)(1)
(1)
(1)
(1)
where NIR and R indicate channel or band of LANDSAT 8 OLI, which are near-infrared and visible red, respectively. The AGB and AGC will be derived from the NDVI analysis result of the satellite image, taken during July to August 2023.The analysed image, obtained as raster layer, is further assigned for calculation in GIS. The value obtained from the analysis must range from −1 to 1. Since this study mainly focused on the carbon sequestration from active mine-reforestation, therefore, the area where NDVI are over 0.2, which refers to the existence of vegetation [23], are considered as inputs for further estimation.
Selection of estimation’s formula
The selection of an appropriate empirical method for estimating carbon sequestration in reforestation area is contingent upon the ecological parameters and the dominant species selected for plantation. When considering the entirety of the national region, it becomes impractical to accurately quantify AGB and AGC. Hence, the quantification of carbon sequestration can be accomplished by the utilization of certain formulas associated with remote sensing methodologies. In this study, a selection of 11 NDVI-based formulas, reported in the academic literature and notably relevant to reforestation in Thailand and similar climate zones, has been made and compared. The aforementioned formulas are displayed in . The selection is influenced by four key parameters: average temperature, average rainfall intensity, dominant vegetation type, and elevation. These equations are assigned in association with prescribed NDVI layer to obtain the layers for evaluation.
Estimation of above-ground carbon storage
As can be noticed in , the results of the calculation layers can be divided into two main groups, which are the AGC and AGB; therefore, the evaluation of the formulars should be accomplished under the same basis. The calculations for AGB are conducted according to , in the event that the results from the equations are in term of AGB. The AGB value from the selected equations are converted into AGC using raster calculations in GIS application based on [31], as can be seen in EquationEquation (2)(2)
(2) .
refers to the value of aboveground carbon stock at each cell of calculation layers, while represents the aboveground biomass of the vegetation at each cell of calculation. The unit of both variables are tCO2/ha/cell.
(2)
(2)
Table 2. Selected formular for this study based on significant characteristic of the reforestation in Thailand.
Relative standard deviation analysis
Due to the conversion of secondary data involved in estimating carbon sequestration from NDVI using various formulas, the result’s precision and repeatability must be considered. Precision denotes the degree of agreement between replicated and independent outcomes within predetermined parameters, whereas repeatability pertains to the accuracy of independent test results executed using the identical methodology [Citation32]. In order to assess the applicability of these equations for estimating the present conditions of the reforestation area in Thailand affected by mining, where direct validation is not possible, the standard relative deviation (%RSD) is utilized [Citation33]. This metric is appropriate for comparing the precision of various methods. The formula with the smallest percentage RSD indicates a higher level of estimation precision and consistency. The calculation parameters for determining the percent RSD in this investigation are illustrated in EquationEquation (3)(3)
(3) .
(3)
(3)
where stands for zonal standard deviation of the estimated results from the selected equation, while
refers to the value of zonal mean value of them. These parameters are retrieved using spatial statistical analysis based on GIS application.
Estimation of potential carbon sequestration
After the definition of appropriate estimation form, the calculations to determine the potential carbon sequestration of mine-reforestation area in Thailand are further conducted according to EquationEquations (13)–(15). This equationa set of is adapted from the certificated rules and guideline, established by Thailand Voluntary Emission Reduction Program (T-VER). The T-VER is prescribed rules and procedures for project development, GHGs emission reduction methodology, and certification of emission reduction credit [Citation34,Citation35].
(13)
(13)
(14)
(14)
(15)
(15)
(16)
(16)
where refers to belowground carbon stock (ton/ha/cell) at each cell of calculation layers.
and
are the total carbon stock (tCO2e) that can be accumulated from aboveground and belowground of the tree in the reforestation area. The potential carbon sequestration can be estimated based on the summation of aboveground and belowground carbon stock which is represented as
in Equation (6).
Economic potential of the mine reforestation area
The carbon stock of the entire ecosystem are can be found as stated in EquationEquation (17)(17)
(17) [Citation36,Citation37]. The current economic value of potential carbon sequestration in the mine-reforestation can be obtained by multiplying them with the current prices of carbon trade, as stated in EquationEquation (18)
(18)
(18) ; consequently,
represents the economic value of carbon sequestration in the monitored year. In this study, the
is the current economic value of 2023 (
), while
represents the price of carbon based on domestic voluntary carbon market which is commonly market for this stage of Thailand carbon policy at 79 THB per tCO2e [Citation38].
This study evaluates the value of carbon stock of Thai mine-reforestation for the year 2023 and forecasts these values for 2030. The purpose is to demonstrate the economic potential in form of value added of carbon sequestration that the subjected area may provide.
(17)
(17)
(18)
(18)
As for local market, rules regulation and standards are conducted, under the Thailand Voluntary Emission Reduction (T-VER). It is an initiative aimed at reducing emissions in Thailand by voluntary participation, established by the Thailand Greenhouse Gas Management Organization (TGO) with the aim of encouraging and assisting various sectors in voluntarily engaging in activities that reduce GHGs emissions. Participants in this program can generate and trade reduction units or carbon credits within the voluntary domestic market. According to the recommended standard, the reforested area where carbon credits can be accounted and monitored, can be divided into two major group based on their scale.
The first group is the reforested area which contain their territories, ranging from 0.048 to 1.6 km2 (30 to 1000 Rai in Thai unit). This type of area can assign the allometric formular to determine the increasing of carbon stock using EquationEquation (19)(19)
(19) .
(19)
(19)
where refers to the amount of increasing carbon in the monitoring period in unit of tCO2e. T stands for total number of trees in the unit area which is determined to be 1 tree/1 m2 which is the lowest density of common practice for mine reforestation of small-scale mining in Thailand. t is the total year for monitoring period while MAI represents the rate of increase in carbon storage of trees which is equal to 0.0095 tCO2e/plant/year.
For another major reforestation area, it is classified as the estimation scheme for large scale sites, containing over 1.6 km2. TGO recommend the applications of remote sensing technique to monitor and quantify the carbon sequestration in the monitored area, thus the exact method has not been established. Since there is no standard method for estimating of increased carbon stock for this type of area, the increase rate of carbon stock in this study is calculated, using EquationEquation (19)(19)
(19) . The MAI assigned in the estimation for this group is based on the normalized carbon stock rate of the Thai reforestation which is equal to 1.46 ktCO2e/km2/year [Citation39]. The economic potential of carbon stock in the reforestation area can be archived by the comparison between the equivalent value of current
in 2030 (
) and their increased value.
The economic potential of carbon stock in the reforestation area can be archived by the comparison between the inflated value of current carbon sequestration potential with their value in 2030 due to the increasing of carbon stock. To determine the equivalent value of the present carbon sequestration potential in 2030, the average inflation-rate of Thailand at 2.00% [Citation40] is assigned in EquationEquation (20)(20)
(20) , where r is the represent of average inflation rate and n refers to the total monitoring year. Consequently, EquationEquations (21)–(22) demonstrates the estimation scheme for the economic potential of carbon sequestration (
) in this study.
(20)
(20)
(21)
(21)
(22)
(22)
Results and discussion
Satellite image analysis result
The LANDSAT 8 OLI satellite images captured between July and August 2023 are merged and subjected to NDVI analysis. The outcome of the NDVI analysis applied with a GIS application is illustrated in . The presence of lush vegetation in the Northern and Southern regions of Thailand is evident. depicts the boundaries and localities of every active mining production area in Thailand, whereas illustrates the NDVI analysis results over the proposed mine-reforestation area in Thailand.
Estimation of above ground carbon stock
The process of selecting the proper formula as the primary method for calculation was undertaken. As previously specified, each of the obtained from each formula is subjected to spatial statistical analysis to determine its precision and repeatability in the estimation process. presents the findings obtained from the comparative analysis of the selected formulas. The estimation subsequently formula utilized in this work is determined based on Equation (7) because to its superior precision, as indicated by the results. The value of %RSD can be grouped as under 100% and over 100%. According to the study of [Citation33], the closer of %RSD to 100 is preferable. Corresponding to this comparison the average
in the proposed reforestation area of Thai mining is equal to 37.89 tCO2e/cell, based on its %RSD at 106.30%.
Table 3. Comparison between the estimated from each selected formular.
Spatial distribution of estimation results
The assessment of potential carbon sequestration of Thai mine-reforestation is carried out using the designated equation. The analysis initially focused on examining the estimated value in order to investigate the distribution of potential sequestration throughout different regions of Thailand, taking into account with variations in their characteristics based on location. The outcome of the estimation for each region is categorized into nine groups (0.00/0.00–59.24/59.24–69.36/69.36–78.75/78.75–87.42/87.42–95.37, 95.37–104.04/04.04–114.15/114.15–184.95) by the application of spatial clustering, utilizing Jenk’s natural break method [Citation41]. This approach enables the visualization of choropleth spatial data, effectively highlighting patterns while minimizing variation within each class [Citation42]. The clustering outcome is presented in . In accordance with the classification, it is evident that the regions in Thailand where the
values are equal to 0 exhibit the greatest contribution. This occurrence is observed in mining areas, where a significant portion of the land is devoid of any vegetative cover.
Table 4. Classification results from spatial distribution of in the study area.
Results of carbon sequestration potential in mine-reforestation area of Thailand
The estimation of is conducted, based on the results from the previous session, is presented in . Consistency to the clustering results, the northern region of Thailand also possesses the highest potential of carbon sequestration at t/cell. At this rate the total amount of carbon that can be contained in the reforestation of this region is equal to 8819.12 ktCO2e from the total area of 15.012 km2 (Obtained from total cell × cell’s resolution at 30 × 30 m2). For other regions, the centre and southern of Thailand are classified as the area with the second and third levels of carbon sequestration potential can be sensed with
equal to 5,515.96 and 3502.10 ktCO2e, respectively.
Results of economic potential of Thai mine-reforestation area
The estimation of economic potential of Thai mine-reforestation area is conducted according to the prescribed . According to the rules of TGO, the accountable area that can attend the domestic voluntary market are classified as can be followed in . The southern region of Thailand has the highest numbers of priceable area with 320 mines, while the lowest numbers located in the eastern part with only 21 accountable reforested mines.
Table 5. Numbers of accountable reforestation area from licensed-mine in Thailand.
In consistent with , the estimation of economic potential of mine-reforested area in Thailand are accomplished and summarized as established . At present, the northeastern region of G-I group is considered to be the highest potential area for gaining of economic benefits from carbon sequestration with the value expanding from 54.16 million Thai baht (MTHB) in 2023 to 143.74 baht in 2030 (+131.04%, compared to a 2% inflation rate). Conversely, it should be noted that the northern region of Thailand, possessing the highest contribution of carbon sequestration potential, contains the lowest economic potential at +97.54%. Consistent with the estimation results for G-I, the economic potential in the reforestation of G-II also demonstrates the same trend. The highest economic potential can be sensed in the northeastern region with 51.92% expansion, while the northern region expands only 8.94% of carbon stock value.
Table 6. Summarized data of economic potential from carbon sequestration in mine-reforestation area of Thailand.
The economic potential of the northern mine-reforestation area oppositely performed in the contrast trend with the potential carbon sequestration according to the increasing of carbon sequestration contribution within the accountable area. demonstrates the comparison between increasing of CSE and EP in 2030, where the northeastern region contains prior growth in all the monitoring parameters.
Discussion and suggestions
Integrated spatial and remote sensing for the determination of carbon sequestration
Integrated spatial technology between GIS and remote sensing is an effective tool for illustrating potential carbon sequestration in continental areas. In this study, the techniques play an important role in determining the potential of the mine-reforestation area for reducing carbon emissions. In Thailand, most of the mine-reforestation is regulated and reclaimed as deciduous forests with local species; therefore, the selected formula can give the most appropriate precision with a %RSD equal to 106.30 due to the similarity of the vegetative selection. Even supposing that the precision of the estimation can be statistically acceptable, there is some evidence of the high uncertainty of estimations, which contains a high value of the standard deviation at ±40.28 tCO2e.
The attributes of satellite images, which serve as the primary input for remote sensing computations, provide an explanation for these uncertainties. Numerous factors, including atmospheric conditions and spatial resolution, have the potential to introduce interference into the data. The acquisition of valuable information regarding the Earth’s surface by optical satellites is impeded at multiple levels due to the detrimental effects of cloud cover and humidity [Citation43]. In this study, the cloud coverage in all images is controlled to be less than 20%, which is commonly recommended in similar type of studies [Citation44–46], thus the effects of them in generating of uncertainty still remains. Additionally, higher spatial resolution imagery significantly improves the structural information of vegetation, which in turn enables more complex NDVI analyses [Citation47]. As a result, it can reduce uncertainty in the reforestation of complex areas of mine by generating greater differentiation among various types of vegetation and land cover.
Due to these constraints and the need for systematic control over the use of remote sensing in carbon stock accreditation, unavoidable uncertainties will persist. Presently, the utilization of multispectral unmanned aerial vehicles (UAVs) offers numerous benefits that can mitigate these adverse consequences. Maintaining a high spatial resolution of up to 5 m2, it is capable of gathering targeted imagery in the same manner as an operational satellite, oblivious to cloud cover. The rapid deployment, cost-effectiveness, and ability to capture precise and detailed data are all facilitated by these capabilities, which are crucial for precise carbon sequestration in the mine-reforestation region [Citation48]. According to these advantages, advocating this technique in carbon accreditation can effectively increase the reliability of the recommended procedures of TGO.
Carbon sequestration potential in the mine-reforestation area of Thailand
Using integrated spatial analysis, the potential carbon sequestration in the reforestation area of Thai mines is computed and illustrated at the regional level. It has been ascertained that the total CO2e of Thailand is 19,187.20 ± 496.91 kt. The northern region of Thailand exhibits the greatest potential among all areas, with the central and southern regions following suit in terms of vegetation abundance, as indicated by the distribution of their respective NDVI values.
The NDVI values, which indicate the amount of reflectance from vegetation biomass [Citation22], can be used to assess the current reforestation status during the mining lifecycle, particularly in the southern region. The mines in this area, which are currently in operation, often undermine reforestation efforts and instead prioritize meeting the demand for aggregates in the region. In contrast, in the northern region of the country, where there are numerous mines which are in the last stages, reforestation activities are encouraged to facilitate their closure plans. As a result, the current levels of carbon sequestration in this area are the highest compared to other regions.
In spite of the dense vegetation cover in the region of undergoing reforestation, the values are categorized as low to medium tier (59.24–87.42 tCO2e/cell), in comparison with the values observed in all other rankings of spatial clustering. This phenomenon was a result of the vegetation in the active mine being typically juvenile (0–10 years old), which is also common for all other regions from this study.
While the actual carbon sequestration value in the reforestation areas falls short of initial expectations, the findings nonetheless indicate significant potential for post-mining development in these places. The mines contain a total area of 193.63 km2 that is devoid of vegetation and classified as under-operating with a 0 value of This unvegetated land can be potentially developed utilizing a combination of reforestation techniques and other technologies, such as Carbon Capture and Storage (CCS). Regarding traditional reforestation methods, it is evident that expanding the reforested area has the potential to significantly enhance carbon sequestration capabilities. However, the extent of this expansion is inherently limited by regulations, economic considerations related to the reclaimed value of the land, and societal attitudes. Consequently, the current levels of carbon uptake remain insufficient. The methods developed by the CCS offer the potential to improve the quantity of carbon sequestration within the given restrictions.
Based on information provided by the Department of Primary Industries and Mines (DPIM), it has been reported that there are currently 325 active mines engaged in the production of construction cement and aggregates. Consequently, the implementation of Carbon Capture and Storage (CCS) measures in the unvegetated regions is expected to yield advantageous outcomes. One novel technique that can enhance the potential for carbon sequestration is the process of Carbon Dioxide Mineralization. This process involves the interaction of carbon dioxide with rocks, resulting in its transformation into stable minerals. These minerals serve as a permanent storage medium for carbon dioxide, effectively preventing its release into the atmosphere. Assuming that the proposed method will exhibit a high level of effectiveness, it becomes imperative to emphasize the significance of fostering awareness and implementing regulations that enable the integration of accommodating technology in post-mining areas.
Economic potential of Thai mine-reforestation area
The estimation of economic potential of Thai mine-reforestation area is prescribed. Even though the calculation of CSE and EP must be separately conducted, the results demonstrate the same trend for both divisions. The Northeastern region of Thailand, which processes the highest numbers of accreditable area according to the rules of TGO at 80.97 km2, can yield up to 691.93 MTHB in 2030 (See ANNEX I for more information). Since the mines in this region is located over the deposition of igneous bed, so the reforestation area is commonly composed of shrub and dry deciduous forest with lower NDVI value, resulting in a contrast between carbon sequestration and economic potential. This is the best evidence for the development of the non-vegetated area that can increase the economic value up to 131.04% and 51.92% for G-I and G-II, respectively.
Moreover, the northern region of Thailand, characterized by an abundance of vegetated areas, may offer the greatest potential for carbon sequestration but is devoid of economic value-adding potential. Because of the higher basic proportions utilized in the division, this region is unable to increase EP to the same extent as other regions. This may also bear resemblance to the attribute of aged vegetation, which fails to accumulate carbon in comparison to its youthful counterparts. According to this study, the rate of carbon sequestration is relatively low in the northern region due to the fact that some of the mines has already reclaimed the area as evergreen forest, resulting in a low rate of accreditable sequestered carbon [Citation49]. These evergreen forests are classified as protected area according to the regulation; therefore, the area cannot be accredited after the reclamation.
The economic potential of mine-reforestation in Thailand can therefore be estimated; consequently, additional research is required. The economic model for the expansion of the voluntary carbon credit market should be implemented in order to develop the study’s findings. Thai IPPU can benefit from the precise estimation to develop effective mitigation and reduction policies for greenhouse gas emissions, as well as generate financial gains for stakeholders in the sector.
Developing a policy for mine-reforestation area in Thailand
Although the mining industry is intimately linked to worldwide trends and awareness regarding its primary contribution to greenhouse gas emissions, Thailand’s mining sector does not yet have defined legislation for carbon management and reduction. Based on the mineral acts of Thailand, it is mandated that the reclamation of all types of mines necessitates registration, accompanied by a fee of 21.25 THB/m2/y. This requirement poses a significant challenge to the extension of reforestation efforts since the cost associated with mine reclamation limits the allocation of resources towards reforestation endeavours. This situation has the potential to result in a decline in the global compatibilities of the IPPU, together with the deterioration of the environment.
In order to address this unfavourable circumstance, it may be advisable to implement a policy and management framework that links the reimbursement for reclamation fees to the carbon sequestration potential of the mine. This approach could serve as a viable strategy for restoring the reforestation rate. This notion is further substantiated by the existing T-VER delivery modalities that can be tailored to the specific characteristics of the Thai mining industry. The implementation of collaborative concessions among small mines within a group might render the region eligible for accreditation registration. This is facilitated by the utilization of T-VER, which permits projects with similar activities and operational durations to be registered collectively under a single entity. This can help mitigate the degradation of environmental quality that arises from unregulated operational practices in certain small-scale mining operations. The T-VER also provided the capability for implementing programs of activity (PoA) that offer support to groups within the same industry. The PoA consists of GHGs-reduction programs that are accompanied by a comprehensive plan and include sub-projects implemented across numerous locations and time periods. It also represents a viable option for the remaining non-accreditable mine to generate value inside the domestic carbon market.
Notwithstanding the existence of reforestation alternatives for each sector of the mining industry, excellent governance in both the public and private spheres remains the most critical factor in establishing effective mitigation. The promotion of mining reclamation as a carbon reservoir should be conducted with a strong emphasis on benefit coordination and cooperation. In order to foster compatibility between Thailand’s mining industry and the IPPU sector within a sustainable global society, it is imperative for the Department of Primary Industries and Mining, the Royal Forest Department, the TGO, and other relevant authorities to collaboratively review and amend their respective rules and regulations.
Conclusion
Thailand has witnessed a consistent rise in awareness regarding the reduction of greenhouse gas emissions since the 2010s. However, concrete data regarding the sector’s potential in terms of its role and participation remains scarce, primarily due to geographical complexities and characteristics. The potential of spatial distribution can be effectively established by the integration of spatial analysis and remote sensing, allowing for descriptive analysis. The findings suggest that mine reforestation in the northern region of Thailand exhibits the greatest capacity for carbon sequestration, amounting to 19,187.20 ktCO2e. This can be attributed to the substantial presence of vegetated land in the area. On the contrary, the economic performance of the region is comparatively lower than that of the northeastern region, which exhibits a significant increase in economic value of up to 131.04% from the aforementioned data. This occurs due to the characteristic of the area that reclaimed as evergreen forests, which means that the reclamation activities are cannot be extended in the future. Although the method may have a strong capacity for displaying and quantifying carbon sequestration in both continental and remote areas, it is necessary to obtain more exact information and inputs in order to improve the accuracy of the calculations. Based on the study, policymakers may benefit from these potentials by working with stakeholders to collaboratively revise rules, roles, and regulations in order to enhance the viability and competitiveness of Thailand’s mining industry in the context of a sustainable global society.
Disclosure statement
I have no conflicts of interest to disclose.
Data availability statement
The data that supports the findings of this study is available from the corresponding author upon reasonable request.
Additional information
Funding
References
- Ulrich S, Trench A, and Hagemann S. Gold mining greenhouse gas emissions, abatement measures, and the impact of a carbon price. J Cleaner Prod. 2022;340:130851. doi: 10.1016/j.jclepro.2022.130851.
- DPIM Department of Primary Industries and Mines. (n.d.). Public relation management (DPIM) SYSTEM. Available from: https://www.dpim.go.th/images/landing/indexwebnew.php.
- Heriansyah IK, Miyakuni T, Kato Y, et al. Growth characteristics and biomass accumulations of acacia mangium under different management practices in Indonesia. J Trop Sci. 2007;19(4):226–235.
- Somprasong K, Hutayanon T, Jaroonpattanapong P. Using carbon sequestration as a remote-monitoring approach for reclamation’s effectiveness in the open pit coal mine: a case study of Mae Moh, Thailand. Energies. 2023;17(1):231. doi: 10.3390/en17010231.
- Ussiri DA, Lal R. Carbon sequestration in reclaimed mine soils. Crit Rev Plant Sci. 2005;24(3):151–165. doi: 10.1080/07352680591002147.
- Tripathi N, Singh RS, Nathanail CP. Mine spoil acts as a sink of carbon dioxide in the dry tropical environment of India. Sci Total Environ. 2014; 468–469:1162–1171. doi: 10.1016/j.scitotenv.2013.09.024.
- Shrestha RK, Lal R. Ecosystem C budgeting and soil C sequestration in reclaimed mine soil. Environ Int. 2006;32(6):781–796. doi: 10.1016/j.envint.2006.05.001.
- Jacinthe PA, Lal R. Tillage effects on C sequestration and microbial biomass in reclaimed farmland soils of southwestern Indiana. Soil Science Soc Am J. 2008;73(2):605–613. doi: 10.2136/sssaj2008.0156.
- McKenna PB, Lechner AM, Phinn S, et al. Remote sensing of mine site rehabilitation for ecological outcomes: a global systematic review. Remote Sens. 2020;12(21):3535. doi: 10.3390/rs12213535.
- Badreldin N, Sanchez-Azofeifa A. Estimating forest biomass dynamics by integrating multi-temporal landsat satellite images with ground and airborne LiDAR data in the coal valley mine, Alberta, Canada. Remote Sens. 2015;7(3):2832–2849. doi: 10.3390/rs70302832.
- Ran L, Lu XX, and Xin Z. Erosion-induced massive organic carbon burial and carbon emission in the Yellow River basin. China Biogeosci. 2014;11(4):945–959. doi: 10.3390/rs70302832.
- Malik, A. D., Nasrudin, A., Withaningsih, S., Parikesit,. Vegetation stands biomass and carbon stock estimation using NDVI-Landsat 8 imagery in a mixed garden in Rancakalong, Sumedang, Indonesia. IOP Conf Ser Earth Environ Sci. 2023;1211(1):012015. doi: 10.1088/1755-1315/1211/1/012015.
- Uttha T, Buasri N, Prasertsri N, et al. Assessment of above-ground biomass in Phu Pha Wua Forest Park, Kalasin Province, Thailand. Int J Innovat Eng Sci Res. 2017;4(6):74–80.
- Yang G, Su C, Zhang H, et al.: Tree-level landscape transitions and changes in carbon storage throughout the mine life cycle. Sci Total Environ. 2023;905:166896. doi: 10.1016/j.scitotenv.2023.166896.
- Hu J, Ye B, Bai Z, et al. Remote sensing monitoring of vegetation reclamation in the Antaibao open-pit mine. Remote Sens. 2022;14(22):5634. doi: 10.3390/rs14225634.
- Yuan M., Ouyang J., Zheng S., et al. Research on the ecological effect assessment method of ecological restoration of open-pit coal mines in alpine regions. Int J Environ Res Public Health. 2022;19(13):7682. doi: 10.3390/ijerph19137682.
- Larekeng SH, Nursaputra M, Hamzah AS, Mustari AS, Arif AR, Ambodo AP, Lawang Y, and Ardiansyah A. An assessment of high carbon stock and high conservation value approaches in mining areas. IOP Conf. Ser.: Earth Environ. Sci. 2021;807(2):022034. IOP Publishing. doi: 10.1088/1755-1315/807/2/022034.
- Thiteja S., Khamyong S., Boontun A., et al. Using the normalized difference vegetation index (NDVI) to assess the carbon storage of plantations in the Zinc-Mined Mae Tao watershed, Mae Sod District, Tak Province. Burapha Sci J. 2020;8:51–63.
- Somprasong K, Assawadithalerd M. Integrated spatial approaches for long-term monitoring of cadmium contamination caused by rainfall erosion: a case study of overland sediment in Mae sot, Thailand. Phys Chem Earth Parts A/B/C. 2021;121:102961. doi: 10.1016/j.pce.2020.102961.
- Hutayanon T, Somprasong K. Application of integrated spatial analysis and NDVI for tree monitoring in reclamation area of coal mine. Environ Sci Pollut Res. 2023;1–11. doi: 10.1007/s11356-023-28910-1.
- Liang L, Di L, Zhang L, et al. Estimation of crop LAI using hyperspectral vegetation indices and a hybrid inversion method. Remote Sens. Environ. 2015;165:123–134. doi: 10.1016/j.rse.2015.04.032.
- Choudhary, K., Shi, W., Boori, MS, and Corgne, S. (2018). Agriculture phenology monitoring using NDVI time series based on remote sensing satellites: a case study of Guangdong, China. Opt Mem Neural Networks 2019;28:204–214. doi: 10.3103/S1060992X19030093.
- Wessels KJ, Prince SD, Zambatis N, et al. Relationship between herbaceous biomass and 1‐km2 advanced very high-resolution radiometer (AVHRR) NDVI in Kruger National Park, South Africa. Int J Remote Sens. 2006;27(5):951–973. doi: 10.1080/01431160500169098.
- Mey CB, Gore ML. Biodiversity conservation and carbon sequestration in agroforestry systems of the Mbalmayo Forest Reserve. J. Environ. Sci. 2021;37:91–103.
- Bindu G, Rajan P, Jishnu ES, et al. Carbon stock assessment of mangroves using remote sensing and geographic information systems. Egypt J Remote Sens Space Sci. 2020;23(1):1–9. doi: 10.1016/j.ejrs.2018.04.006.
- Laosuwan T, Uttaruk Y. Carbon sequestration assessment of the orchards using satellite data. J Ecol Eng. 2017; 18(1):11–17. doi: 10.12911/22998993/66257.
- Situmorang JP, Sugianto, S. Estimation of carbon stock stands using EVI and NDVI vegetation index in the production forest of Lembah Soleawah Sub-district, Aceh, Indonesia. Aceh Int J Sci Technol. 2016; 5(3):126–139. doi: 10.13170/aijst.5.3.5836.
- Ali N, Saad M, Ali A, et al. Assessment of aboveground biomass and carbon stock of subtropical pine forests in Pakistan. J. Sci. 2023;69(7):287–304. doi: 10.17221/125/2022-JFS.
- Khaple AK, Devagiri GM, Veerabhadraswamy N, et al. Vegetation biomass and carbon stock assessment using a geospatial approach. In: Shit PK, Pourghasemi HR, Adhikary PP, Bhunia GS, Sati VP, editors. Forest resources resilience and conflicts. Amsterdam: Elsevier. 2021. p. 77–91. doi: 10.1016/B978-0-12-822931-6.00006-X.
- Banerjee K, Sahoo CK, and Paul R. Assessment and modelling of vegetation biomass in a major bauxite mine in the Eastern Ghats, India. Model Earth Syst Environ. 2021;7(4):2341–2361. doi: 10.1007/s40808-020-01004-4.
- Ravindranath NH, Ostwald M. Carbon inventory methods: handbook for greenhouse gas inventory, carbon mitigation, and roundwood production projects. Dordrecht: Springer, 2007.
- Pryseley A., Mintiens K., Knapen K., et al. Estimating precision, repeatability, and reproducibility from Gaussian and non-Gaussian data: a mixed model approach. Appl. Stat. 2010;37(10):1729–1747. doi: 10.1080/02664760903150706.
- Somprasong K, Chaiwiwatworakul P. Estimation of potential cadmium contamination using an integrated RUSLE, GIS, and remote sensing technique in a remote watershed area: a case study of the Mae Tao basin, Thailand. Environ Earth Sci. 2015;73(8):4805–4818. doi: 10.1007/s12665-014-3766-8.
- Bunjongsiri K. The overview of the carbon credit market in Thailand. Sau J Sci Technol. 2019; 5(2):1–9.
- Kauffman JB, Heider C, Cole TG, et al. Ecosystem carbon stocks of micronesian mangrove forests. Wetlands. 2011;31(2):343–352. doi: 10.1007/s13157-011-0148-9.
- Kauffman JB, Donato DC. Protocols for the measurement, monitoring, and reporting of structure, biomass, and carbon stocks in mangrove forests. Bogor, Indonesia: Cifor, 2012.
- Lichaikul N, Chidthaisong A, Havey NW, et al. Carbon stock and net CO2 emission in tropical upland soils under different land uses. Kasetsart J Nat Sci. 2006;40:382–394.
- Blondes MS, Merrill MD, Anderson ST, et al. Carbon dioxide mineralization feasibility in the United States. Scientific Investigations Report—US Geological Survey, 2019 (2018–5079).
- Daripada Sektor PGRH. The development of a prototype project for carbon storage and greenhouse gas emission reduction in Thailand’s agricultural sector. Sains Malaysiana. 2019;48(10):2083–2092. doi: 10.17576/jsm-2019-4810-03.
- Bank of Thailand, Monetary Policy Report 1/2023, 2023.
- Ke C, He S, Qin Y. Comparison of the natural breaks method and frequency ratio dividing attribute intervals for landslide susceptibility mapping. Bull Eng Geol Environ. 2023;82(10):384. doi: 10.1007/s10064-023-03392-0.
- Lin Y. A comparison study on natural and head/tail breaks involving digital elevation models. 2013.
- Li Z, Shen H, Weng Q, et al. Cloud and cloud shadow detection for optical satellite imagery: features, algorithms, validation, and prospects. ISPRS J Photogramm Remote Sens. 2022;188:89–108. doi: 10.1016/j.isprsjprs.2022.03.020.
- Awad MM. FlexibleNet is a new lightweight convolutional neural network model for estimating carbon sequestration qualitatively using remote sensing. Remote Sens. 2023;15(1):272. doi: 10.3390/rs15010272.
- Gudelė GM, Visockienė JS. Application of remote sensing for monitoring carbon farming: a review. Mokslas-Lietuvos Ateitis/Science-Future of Lithuania. 2023;15. doi: 10.3846/mla.2023.19396.
- Jia X, Han H, Feng Y, et al. Scale-dependent and driving relationships between spatial features and carbon storage and sequestration in an urban park in Zhengzhou, China. Sci Total Environ. 2023;894:164916. doi: 10.1016/j.scitotenv.2023.164916.
- Liu M, Yu T, Gu X, et al. The impact of spatial resolution on the classification of vegetation types in highly fragmented planting areas based on unmanned aerial vehicle hyperspectral images. Remote Sensing. 2020;12(1):146. doi: 10.3390/rs12010146.
- Biney JK, Saberioon M, Borůvka L, et al. Exploring the suitability of uas-based multispectral images for estimating soil organic carbon: comparison with proximal soil sensing and spaceborne imagery. Remote Sensing. 2021;13(2):308. doi: 10.3390/rs13020308.
- Thailand Greenhouse Gas Management Organization (TGO). Calculation for Carbon Sequestration in tree 3/2023.