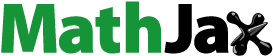
Abstract
The milk Fourier-transform infra-red spectrometry (FTIRS) can be used to predict the enteric methane emissions (EME) at population level. In this study, the variability in FTIRS predicted EME traits due to the breed of cow, farm, the production level of individual cows within herds, and their interactions were evaluated. A dataset obtained from milk recordings, which covered four breeds (Holstein, Brown Swiss, Simmental and Alpine Grey), 6,430 herds, 115,819 cows, and 1,759,706 test-day milk/spectra records was used. The herds were stratified into 5 production levels considering their average daily milk energy production; individual cows within herd were similarly stratified considering their individual production levels. The EME traits were predicted directly from milk FTIR spectra or indirectly from six informative milk FA predicted from milk spectra. The statistical model included, separately for each trait and method, breed, herd intensiveness level, cow production level, and their interactions, year, month, parity, and lactation stage. The direct and indirect methods yielded similar results in predicting CH4 yield per kg of DMI and CH4 intensity per kg of fat-protein corrected milk. The indirect method was reliable in predicting daily EME production per cow, the indirect one did not. EME was affected by the breed x herd production intensiveness interaction, and to a lesser degree, by the breed x cow production level interaction. A better understanding of the complex interactions influencing EME in dairy herds was achieved. This would be useful for the genetic improvement, the environmental certification of farms, and for setting prices in milk payment schemes.
Causes of variation of the FTIR predicted EME were studied at population level using data from 6,430 herds and 1,759,706 milk spectra records
Reliable daily EME estimates were achieved from 6 six informative fatty acids predicted from milk spectra
EME was influenced by the interaction breed × level of herd intensiveness and, to a lesser extent, by the individual production level
HIGHLIGHTS
Introduction
International treaties aimed at reducing the rate of increase in global warming over the coming decades have been signed by the majority of the world’s countries. The major interventions concern reducing dependency on fossil fuels, increasing the soil/forest carbon sink, and limiting CH4 emissions from domestic ruminants and landfill (Moumen et al. Citation2016). Enteric CH4 emissions (EME) are the main source of greenhouse gases from agriculture. The livestock sectors of several countries are currently reducing their yearly EME, and are actively contributing to contrast global climate warming by increasing production efficiency through genetic improvement, better nutrition and management practices, and improved animal health and welfare.
The gold standard method for EME quantification remains the calculation of material and energy balances using respiration chambers (Hristov et al. Citation2018), but it is not feasible at the population or large nucleus levels (de Haas et al. Citation2017). Proxies of daily CH4 emissions are based on analyses of exhaled gases sampled around the nostrils of animals as they access automatic feeding stations or automatic milking parlours (Difford et al. Citation2020). Other proxies of CH4 emissions are based on the milk fatty acid (FA) profiles, considering their relationships with rumen fermentation (van Engelen et al. Citation2015; van Gastelen and Dijkstra Citation2016; Bougouin et al. Citation2019). Both methodologies were used in large surveys carried out on commercial farms, although they are expensive and labour intensive.
Predictive equations obtained from selected informative milk FA analysed using gas chromatography (van Lingen et al. Citation2014) and used on commercial dairy farms (1,158 cows from 85 farms) yielded results that matched expectations with regards to the effects of dairy system, parity and lactation stage (Bittante et al. Citation2018), and the selected FA used and the EME traits obtained were heritable (Bittante and Cecchinato Citation2020). Among the equations published the two proposed by van Lingen et al. (Citation2014) for predicting CH4 yield (g CH4/DMI) and CH4 intensity (g CH4/kg CM, fat and protein corrected milk), were obtained from a meta-analysis that encompassed 30 different diets (eight experiments in two countries) comprising not only different feedstuffs and different forage:concentrate ratios, but also certain fat supplements sometimes used in commercial dairy farms.
To reduce costs and labor, and extend the possibility of EME prediction to population level, equations based on Fourier-transform infra-red (FTIR) spectra of milk were proposed (Negussie et al. Citation2017). The results were variable (Lassen et al. Citation2012; van Gastelen and Dijkstra Citation2016; Vanlierde et al. Citation2016) and raised debates over their scientific and practical value (Shetty et al. Citation2017; Wang and Bovenhuis Citation2019).
FTIRS predictions of EME were used in large surveys to quantify the effects of individual herds and of the parity and lactation stage of individual cows, and were found to have a genetic basis. FTIR spectrometry produced satisfactory results to predict the informative FA needed to use van Lingen et al.'s (Citation2014) equations for EME prediction (indirect approach: milk spectra → milk FA → EME) (Bittante and Cipolat-Gotet Citation2018). A direct approach, where EME was predicted directly from milk spectra was also proposed, and both direct and indirect predictions were found to have a genetic background (Bittante et al. Citation2020).
However, FTIR predictions of EME have not yet been studied at the population level with different breeds reared in herds under different dairy farming systems, and with animals stratified for different potential milk yields. A scientific question concerns the effect of daily milk yield on EME traits. It is important to understand whether differences in average milk yield among different herds and differences in milk yield among different cows within herd affect EME traits; whether these two effects are of the same magnitude, direction and pattern, and whether the differences are similar across different breeds or if there is some interaction. Aim of this research was to study FTIRS predictions of informative FA and EME traits at the population level based on a large dataset of milk infra-red spectra obtained during routine milk recording operations and to compare different dairy and dual-purpose breeds across different herd intensiveness and cow productivity levels.
Material and methods
The experimental design
The study was based on a dataset obtained from the milk recording systems of Italian dairy populations and did not include any direct handling of animals to take measurements or samples, and did not require authorisation by the University Ethical Committee. It drew on the entire historical database of milk recording and artificial insemination outcomes of the Breeders Federation of Alto Adige/Südtirol (Bolzano, Italy) in the northeastern Italy. This database was used in previous studies on the relationships between milk FTIR spectra and the fertility of dairy cows (Toledo-Alvarado et al. Citation2017, Citation2018a, Citation2018b, Citation2021), and on the prediction of the cheese-making ability and urea content of milk at the population level (Bittante et al. Citation2021; Bittante Citation2022).
Milk recording data and editing
We extracted all the data from cows of the four most common breeds in the province: two specialised dairy breeds, Holstein and Brown Swiss; and two dual-purpose breeds, Simmental and Alpine Grey. Multi-breed herds, i.e. with cows of two or more breeds, about one third of total, were treated as two or more single-breed herds.
A total of 1,898,994 test-day records were extracted. Only herds with more than five cows, and cows with more than five records were retained. After editing, 6,430 herds, 115,819 cows, 291,129 lactations and 1,759,706 test days/milk spectra remained for data analyses. Parity orders were grouped into first (95,049), second (74,976), third (55,780), fourth (33,578), and fifth and subsequent (31,746).
Test day milk recording data included daily milk yield. A milk sample with preservative added was transported to the laboratory for composition analysis.
FTIR spectra of milk samples
The milk samples collected were analysed with a MilkoScanTM FT+ 6000 (Foss A/S) on the basis of their FTIR spectra. The instrument and its operations are validated and certified according to ISO 9622:2013/IDF 141:2013 (ISO Citation2013) recently updated to ISO 21543:2020/IDF 201:2020 (ISO Citation2013, Citation2020; Niermöller and Holroyd Citation2019). The complete spectrum of every milk sample analysed was stored in the experimental database. A total of 1,060 absorbance values were recorded for each milk sample covering the infra-red wavenumbers ranging from 5,000/cm (corresponding to a wavelength of 2.0 μm) in the near-infra-red subdivision of the infra-red area, through the mid-infra-red to wave number 930/cm (corresponding to a wavelength of 10.8 μm) in the far infra-red subdivision.
EME traits and informative FA
For indirect prediction of the EME traits we used the equations developed by van Lingen et al. (Citation2014).
Methane yield per unit of dry matter intake:
where 16:0iso is iso-palmitic acid, 18:t10,+t11 is the sum of the isooleic and vaccenic acids, and 18:2c9,c12 is the α-linoleic acid of milk, all expressed as % of the sum of all milk FAs.
Methane intensity per unit of fat-protein corrected milk produced:
where 4:0 is butyric acid, and 18:1c9 is oleic acid, all expressed as % of the sum of all milk FAs.
In addition, daily CH4 production per cow was obtained from CH4 intensity.
Daily CH4 production per cow:
where dMY is the daily production of milk per cow corrected for fat and protein.
FTIR spectrometry of informative FAs and EME traits
Two datasets of EME traits predicted using the equations developed by Bittante and Cipolat-Gotet (Citation2018) were compiled:
Methane yield, CH4 intensity, and daily CH4 production were indirectly predicted (Indirect-IR) from the FTIR-predicted informative FA and the daily milk yields measured during milk recording using the aforementioned equations;
Methane yield, CH4intensity, and daily CH4 production were directly predicted (Direct-IR) from FTIR spectra using prediction equations of previous papers (Bittante and Cipolat-Gotet, Citation2018).
Prior to data analysis, each single wavenumber of the spectra was centred and standardised to a null mean and a unit sample variance. Mahalanobis distances were calculated using the Mahalanobis function in the R software (R Core Team 2015; R Foundation for Statistical Computing, Vienna, Austria). The spectra were centred to a null mean and standardised to a unit sample variance. The spectra were not subjected to any other mathematical pre-treatment.
Separate models were fitted for all the EME traits and for the informative FA. A Bayesian model (the BayesB model) was implemented in the BGLR package of the R software (Pérez and De Los Campos Citation2014). Details of the chemometric procedure used are described in Ferragina et al. (Citation2015), while details of the development of the FTIR equations for predicting informative FA and EME traits are described in Bittante and Cipolat-Gotet (Citation2018). The accuracy of the prediction equations was assessed by a training-testing procedure.
Accuracy of the FTIR prediction equations
A random training data set (80% of the total records) was used to build the equation, and a testing data set (20% of the total) was used as validation; the procedure was repeated 10 times for each trait. The coefficients of correlation between the measured and predicted traits (r) and the root mean square error of cross-validation (RMSECV) of the predicted traits (Bittante and Cipolat-Gotet Citation2018) were:
Informative FA for indirectly predicting EME traits:
4:0 butyric acid, r = 0.69; RMSECV = 0.66%;
16:0iso iso-palmitic acid, r = 0.55; RMSECV = 0.08%;
18:1t10 isooleic acid, r = 0.65; RMSECV = 0.07%;
18:1t11 vaccenic acid, r = 0.66; RMSECV = 0.28%;
18:1c9 oleic acid, r = 0.82; RMSECV = 1.85%;
18:2c9,c12 linoleic acid, r = 0.70; RMSECV = 0.44%;
Directly predicted EME traits:
CH4/DMI, CH4 yield (g/kg DM), r = 0.70; RMSECV = 1.18 g/kg;
CH4/CM, CH4 intensity (g/kg CM), r = 0.75; RMSECV = 1.17 g/kg;
dCH4, daily CH4 production (g/d/cow), r = 0.60; RMSECV = 86.0 g/d.
Stratification of herd intensiveness and cow productivity levels
Within breed, the herd average amount of milk energy produced daily by lactating cows was used as an indicator trait of herd ‘intensiveness level’. This is because it represents the cow’s net energy requirement for milk production as well as the animal’s major – and more variable – metabolic load. Unlike daily milk yield, energy output does not include the weight of water and ashes, but does include fat, protein and lactose in terms of their energy content. The herds were stratified into 5 categories of herd intensiveness level (HL) determined according to the average daily milk energy output (dMEO, MJ/d) yielded by all the lactating cows in the herd, as described in detail in a previous study using the same dataset (Bittante et al. Citation2021). Briefly, the net energy content (NEL) of milk was estimated by applying the following equation, recommended by NRC (Citation2001):
where NEL is the energy content of one kg milk.
The least squares means (LSM) of the dMEO of each herd were calculated separately for each breed after correcting the data for parity, class of days in milk, and year-season. The herd solutions were used to classify them into five dMEO levels: HL-1, herds < −1.5σ; HL-2, −1.5σ to −0.5σ; HL-3, −0.5σ to +0.5σ; HL-4, +0.5σ to +1.5σ; HL-5, > +1.5σ; where σ is the standard deviation of the herds’ LSM of dMEO within breed.
Similarly, within each breed and herd, the average milk energy produced daily by individual lactating cows was used as an indicator trait of the animal’s ‘productivity level’, i.e. the metabolic effort it requires for milk production. The model included the effects of parity, class of days in milk, and year-season. The milk production levels of the cows (CL) were stratified into five classes (CL-A to CL-E) according to the solutions of individual cows within HL.
The number of herds, cows and spectra available by breed, HL and CL are shown in Figure .
Figure 1. Number of herds, cows and spectra collected by breed, herd intensiveness level class (HL) and cow production level class (CL). Five classes of daily milk energy output (dMEO, MJ/d) for each factor: HL-1 and CL-A <-1.5σ; HL-2 and CL-B -1.5σ to -0.5σ; HL-3 and CL-C -0.5σ to +0.5σ; HL-4 and CL-D + 0.5σ to +1.5σ; HL-5 and CL-E > +1.5σ.

Statistical analysis
The informative FA and EME traits were analysed using the following linear model:
,
,
where
is the response on the trait (FA and EME traits); µ is the general mean;
is the parity order (i = 1st, 2nd, 3rd, 4th or ≥ 5th);
is the category of DIM (j = 12 groups of 30 days each, with the last category open, max DIM = 450 d);
is the effect of Year (k = years 2010 to 2017);
is the effect of month (l = January to December); HLl is the Herd Level class (l = 1 to 5); CLn is the Cow Level class (n = 1 to 5);
is the effect of breed (o = Holstein, Brown Swiss, Simmental, and Alpine Grey);
is the effect of the interaction between Herd Level l and breed o;
is the effect of the interaction between Cow Level n and breed o; and
is the random residual
The models were fitted using the lm and aov functions in R. Details of the statistical models are given in a previous study on the same dataset (Bittante et al. Citation2021).
Results and discussion
Main sources of variation in milk yield and informative milk FA used for indirect EME predictions
The results of the analysis of variance carried out on the daily milk yield and the six FTIR-predicted informative FA included in the equations used for indirect prediction of EME traits are given in Table . Due to the very large amount of records, all the effects included in the statistical model were highly significant for every trait (p < 0.001). The relative importance of the various factors for each trait can be assessed from the size of the F-values. In accordance with the aims of this study, only the effects of breed, HL, CL, and their interactions are reported and discussed.
Table 1. Analysis of variance of milk yield and of the predicted informative fatty acids content: F-values of the factors included in the model of analysis (all the effects are highly significant for p < 0.001).
The average daily milk yield (23.2 ± 7.4 kg/d) is lower than, but with a similar variability to the values (24.5 ± 7.9 kg/d) reported in previous research by Bittante and Cipolat-Gotet (Citation2018) on direct and indirect FTIR predictions of EME traits, and in Lingen et al.'s (2014) research on fatty acid prediction of EME traits (28.9 ± 6.4 kg/d). The major source of variation in daily milk yield was breed of cow and HL, followed by CL and stage of lactation.
While oleic and linoleic acid are almost always included in the FA profiles of bovine milk, the other informative FA are found only when detailed FA profiles are analysed. The average values and SDs of the six FTIR-predicted informative FA considered here are similar to those found in previous research on detailed FA profiles of bovine milk analysed by gas chromatography (Heck et al. Citation2009; Pegolo et al. Citation2016). It should be noted that it is usually only the major milk FA that are predicted by FTIR, as those present in milk in small amounts generally have exhibit a modest level of prediction accuracy (Eskildsen et al. Citation2014; Ferrand-Calmels et al. Citation2014; Rovere et al. Citation2021).
The most important sources of variation in informative FA and in daily milk yield had different rankings. In neither case was breed of cow among the most important factors, despite being always significant. This is consistent with the results of previous analyses of milk FA, which revealed minor differences in the profiles across different breeds, with the exception of Jersey breed, not included here (Maurice-Van Eijndhoven et al. Citation2011; Vanbergue et al. Citation2017; Poulsen et al. Citation2012). Similar modest differences were found using FTIR predictions (Soyeurt et al. Citation2006; Maurice-Van Eijndhoven et al. Citation2013; Gottardo et al. Citation2017).
Stage of lactation was the most important source of variation for almost all the FA considered, except for the two 18:1t isomers (t10 and t11), which were more affected by parity (important also for oleic and iso-palmitic acids). The two 18:1t isomers and linoleic acid were also greatly affected by HL. The variation due to CL was less important than that due to HL (except in the case of butyric acid). The interactions between breed and both HL and CL, although less important than the main factors, were always highly significant. The LSMs of the major effects of breed of cow, HL and CL are therefore not shown in the figures, whereas the LSMs of the interactions are shown and discussed in the relevant chapters.
Main sources of variation in EME traits
The results of the analysis of variance carried out on the EME traits predicted directly by FTIR spectra (Direct-IR) and indirectly through prediction of the informative FA(Indirect-IR) are given in Table . Also, for EME traits, due to the large amount of data available, all the effects included in the statistical model were highly significant (p < 0.001) for every trait. In accordance with the aims of this study, only the effects of breed of cow, HL, CL, and their interactions are shown and discussed.
Table 2. Analysis of variance of the enteric CH4 yield, intensity and production predicted directly from milk FTIR spectra or through prediction of informative fatty acids: F-values of the factors included in the model of analysis (all the effects are highly significant for p < 0.001).
The means and SDs of Direct-IR and Indirect-IR CH4 yield were very similar, as were those of Direct-IR and Indirect-IR CH4 intensity. Note that all these predictions were based on FTIR spectra information, the difference being that in the case of Indirect-IR the spectra were used to predict the informative FA that, in turn, were used to estimate the EME traits. The mean values of these two traits (CH4 yield and intensity) in both versions (Direct-IR and Indirect-IR) were very similar but the SDs were lower than those of the population used for calibrating the FTIR equations (21.3 ± 1.6 g/kg DMI, and 14.2 ± 1.8 g/kg CM; Bittante and Cipolat-Gotet Citation2018), and those used for defining the equations based on FA (van Lingen et al. Citation2014).
Methane yield and intensity were both particularly affected by stage of lactation, but also by year of calving and HL.
The results for daily CH4 production differ from the previously cited EME traits because the Direct-IR and Indirect-IR predictions yielded very different results and were affected by different sources of variability. It is worth noting that while the Direct-IR daily CH4 production was predicted directly and solely from FTIR spectra, the reference values used for calibrating the prediction equation were obtained by multiplying the daily milk yield by the CH4 intensity per kg of fat-protein corrected milk of each cow (Bittante and Cipolat-Gotet Citation2018). Chemometric methods are required to extrapolate from the milk FTIR spectra (which constitute substantially qualitative information) the amount of milk produced daily by the cows and the amount of CH4 released into the atmosphere per kg of milk. Using FTIR spectrometry to predict quantitative information is expected to be difficult, which explains the fact that Direct-IR CH4 production was the EME trait prediction with the lowest correlation with the reference data (r = 0.60).
Indirect-IR daily CH4 production was, in contrast, obtained from the cows’ daily milk yield measured during milk recording (quantitative information), whereas FTIR spectra alone were used to predict CH4 intensity per kg of corrected milk (r = 0.75) (qualitative information).
This explains the very different means and SDs obtained for Direct-IR and Indirect-IR daily CH4 production and for the ANOVA. That the ranking of the major sources of variation for Indirect-IR CH4 production is much more similar to daily milk yield (Table ) than to the other EME traits (Table ) was expected. Breed was the most important factor affecting Indirect-IR CH4 production (and daily milk yield), followed by HL then CL.
Effects of herd intensiveness level and cow productivity levels by breed of cow on informative milk FA
In our database, breed of cow was the most important source of variability in milk yield, which ranged on average from 26.8 kg/d in the Holstein cows to 15.6 kg/d in the Alpine Grey cows, with Brown Swiss and Simmental intermediate (Figure ). Note that this study was carried out in an Alpine region, where there is a variety of dairy farming systems, ranging from the very traditional to the modern (Schiavon et al. Citation2019; Berton et al. Citation2020). The differences in the average milk yields of the various breeds therefore reflect not only different genetic backgrounds but also different farming systems. Previous research on the same area which compared six breeds of cow reared in mixed herds (Stocco et al. Citation2018) found a difference within herd between Holstein and Alpine Grey cows of 8.0 kg/d, as opposed to the 11.2 kg/d found here across herds. However, the variability among farms was also very large within breed, which is why we included HL in the statistical model. Note that this is a within-breed classification factor and that it is not corrected for the different environmental conditions in which the different breeds are raised. To make the significance of the HL within breed clearer, we plotted all the LSMs of the investigated traits (y axis) against the actual daily milk yield of each breed and the Herd or CLs (Figure ).
Figure 3. Milk fat, butyric acid (4:0) and iso-palmitic acid (16:0 iso), in % of total fatty acids (LSM ± CI), by breed, herd intensiveness class level or cow production class level plotted against actual cow yield (kg/d).

The first plot in Figure reports the 20 LSMs of the milk fat content (in %) of each of the four breeds and at each of the five HLs. Here, milk fat serves to compare the pattern of total lipid content of the milk with the proportion of the informative FA in the same fat. Large differences between the four breeds in terms of milk fat content were evident (compare the medians, i.e. the third value of each curve). All breeds exhibited similar but not identical patterns of increasing HL. All patterns increased curvilinearly with small differences between the first three HLs and increasingly large differences moving to the fourth and especially the fifth HLs. The increase from first to fifth HL was greater for the Holstein breed than for the Brown Swiss, with the two dual-purpose breeds intermediate (the interaction between breed and HL was highly significant, data not shown). No overlap in the relationships between milk fat content and daily milk yield in the different breeds was found. However, the Alpine Greys and Holsteins had a common trajectory, which could be interpreted as the difference in fat content of these two breeds being dependent mainly on their different milk yields. Simmental, and especially Brown Swiss cows had different parallel trajectories.
The effect of CL within breed had similarities with the HL effect, but also some differences (second plot of Figure ). The rankings and the median values of the four breeds were obviously the same, as the two plots are based on the same dataset. The increase in milk fat with increasing CL was, in this case, almost linear and the difference between the first and last classes of each breed was smaller than for HL. The fact that both HL and CL were favourably associated with the increase in milk fat content was because these classes were based not simply on milk yield, but on the daily output of net energy. So, the classes represent an estimation of the ability of the cows (herds) to produce valuable nutrients, and not simply water excretion, and also quantify the cows’ metabolic burden for lactation.
The informative FA exhibited patterns that were very different from each other and also from total milk fat content. The butyric acid content of milk fat (Figure , 3rd and 4th plots) increased curvilinearly for all breeds at the CL and for Holsteins and Brown Swiss at the HL, but in an opposite pattern to that of milk fat, increasing rapidly from the lower to the central classes then much less so to the higher classes. The curves in part overlap because the average butyric acid content differed significantly in the four breeds, although the differences were not relevant. Note that butyric acid was included in the equation for estimating CH4 intensity per kg milk with a negative sign (van Lingen et al. Citation2014), which means that the increase in this fatty acid with increasing Herd and CLs results in a favourable effect of herd intensiveness and cow production, reducing the EME of lactating cows per kg of milk produced.
Iso-palmitic acid (Figure 3rd, 5th and 6th plots), in contrast, decreased with increasing Herd and CLs. The median values of the breeds were similar, but the decrease was much greater at the HL than at the CL, and in Holsteins and Simmentals than in Brown Swiss and Alpine Greys. Note that iso-palmitic acid is the only one of the six informative FA with positive correlations with both CH4 yield and intensity (van Lingen et al. Citation2014). This means that the decrease in the proportion of iso-palmitic acid in milk at increasing Herd and CLs along with the increase in butyric acid leads to a decrease in CH4 emitted per unit of DMI and per unit of milk produced. A positive association between iso-palmitic acid in milk and EME was also found by Rico et al. (Citation2016), and positive associations between palmitic acid and EME were found by several other authors (Chilliard et al. Citation2009; Dijkstra et al. Citation2011), as was the case for many saturated FA and especially those derived by mammary de novo synthesis (Chilliard et al. Citation2009).
Isooleic acid (18:1 trans10) increased with HL, steeply in the case of Alpine Grey herds, moderately in Brown Swiss and Simmental herds, and very modestly and curvilinearly in Holstein herds (1st and 2nd plots in Figure ). The effect of CL on this fatty acid was smaller and almost the opposite (decreasing, especially in Holsteins and Simmentals). This milk fatty acid was also correlated with a decreased CH4 yield and intensity (van Lingen et al. Citation2014), like several other isomers of oleic acid (Chilliard et al. Citation2009; Mohammed et al. Citation2011). It is worth noting that isooleic acid had a favourable effect on the carbon footprint at increasing levels of dairy farm intensiveness, but an unfavourable effect at increasing cow production levels within farm (Figure , 1st and 2nd plots).
Figure 4. Milk isooleic acid (18:1 trans10), vaccenic (18:1 trans11), oleic (18:1 cis9), and linoleic acid (18:2 cis9,cis12), in % of total fatty acids (LSM ± CI), by breed, herd intensiveness class level or cow production class level plotted against actual cow yield (kg/d).

Vaccenic acids exhibited very different patterns among the HLs (Figure , 3rd plot), with the extremes represented by the small linear increase in Alpine Grey herds and the strong curvilinear decrease in Holstein herds. The effect of CL (Figure , 4th plot), on the other hand, was very similar in the four breeds with relevant linear decreases in all cases. The negative association between vaccenic acid and EME traits (van Lingen et al. Citation2014) was also reported by other authors (Dijkstra et al. Citation2011; Mohammed et al. Citation2011; van Gastelen et al. Citation2018). In addition, the variation in vaccenic acid contributed to an unfavourable effect on EME at increasing HL and CLs.
The major fatty acid considered, 18:1 cis9 (oleic acid), decreased at increasing Herd and CLs (more in the former and less in the latter case) (Figure , 5th and 6th plots). Linoleic acid exhibited an almost opposite pattern to oleic acid, with a strong curvilinear increase for HL and much smaller curvilinear increases for CL (Figure , 7th and 8th plots). As both FA were found to have a negative relationship with the EME of dairy cows (van Lingen et al. Citation2014), they had opposite effects on CH4 emission: oleic acid unfavourable and linoleic acid favourable at increasing HL and CL. Several authors have found oleic acid to have a tempering effect on EME, whereas the effect of linoleic acid was less consistent and was found to be negatively (Mohammed et al. Citation2011) and positively (van Gastelen et al. Citation2018) correlated with the EME of dairy cows.
The complexity of the interactions between breed, farm intensiveness level and cow productivity, and the different weights assigned to the informative FA in the equations for estimating CH4 yield and CH4 intensity may have the potential to capture the differences in feeding regimes, metabolic burdens and the management and environmental conditions of cows and herds. At least in part, the greater differences observed for increasing HL, compared to CL, would be likely due to different feeding practices applied to different breeds and different farms within breeds in terms of forage to concentrate ratio and feed ingredients, that could modify the rumen fermentations towards different proportion of volatile fatty acids.
Effects of herd intensiveness level and cow productivity level by breed of cow on EME traits
EME traits, whether derived directly or indirectly from informative FA and milk FTIR spectra, were also characterised by different patterns in different breeds at increasing HL and CL. The effects on EME of interactions between the cow’s genotype and farming systems were observed in previous studies (Ross et al. Citation2014).
The effect of HL on CH4 yield was always curvilinear, decreasing particularly at higher HLs, and stronger in Holstein (and Simmental) than in the other breeds (Figure ). Comparing the two methods of estimating EME, Direct-IR (EME predicted from milk FTIR spectra) and Indirect-IR (EME predicted from informative FA predicted from milk FTIR spectra), we find they had similar curved shapes, similar rankings of breeds, but the HL effect had a broader range in the former case than in the latter. The effect of CL on CH4 yield was much less evident than the effect of HL, and the difference between the two methods of EME prediction was modest. It is evident that the variation in dMEO (daily milk net energy output; MJ/d) of the different herds reflected above all differences in the compositions of the diets and DMI.
Figure 5. Predicted enteric CH4 yield (LSM ± CI, CH4/DMI, g/kg dry matter intake) predicted directly from milk FTIR spectra or indirectly from predictive informative milk fatty acids by breed, herd intensiveness class level or cow production class level plotted against actual cow yield (kg/d).

At the CL, the animals belong to the same herd and were often fed the same diets (especially if total mixed rations are used). In this case, the only differences arise from the different DMI of cows of different productivity levels and from feed selection behaviour. Bear in mind that stratification of the cows was based on the average output of their entire productive life and therefore did not reflect differences in different lactation stages. In more traditional farms, an amount of concentrate was provided in the milking parlour or, in the case of tie stalls, at the manger (sometimes using automatic feeders). In any case, the same compound feed was generally provided to all animals and the average difference between individual cows over the entire lactation period was moderate.
Regarding CH4 intensity per kg of fat-protein corrected milk, the prediction methods yielded similar patterns with respect to the interaction between the HL and CL and the breed of cow (Figure ). The Alpine Greys had the highest average values and the greatest decrease in CH4 intensity at increasing Herd and CLs. In contrast, Holsteins had the lowest emissions and were the least affected by HL and CL. The Simmental and Brown Swiss herds and cows were intermediate with respect to the other breeds.
Figure 6. Predicted enteric CH4 intensity (LSM ± CI, g/kg of corrected milk) predicted directly from milk FTIR spectra or indirectly from predictive informative milk fatty acids by breed, herd intensiveness class level or cow production class level plotted against actual cow yield (kg/d).

Lastly, the results for daily CH4 production (Figure ) clearly show the inability of the Direct-IR method to capture the expected increase in CH4 emissions with increasing HL and CL, and hence the need for further information in addition to the milk FTIR spectra (van Gastelen and Dijkstra Citation2016). In fact, the patterns were flat and there was overlap among the different breeds. In the case of the Indirect-IR method, there was a strong linear relation between milk yield and CH4 emission. The lines of the four breeds were substantially on the same trajectory. The median values of the different breeds were very different, in accordance with their different average milk yields. It appeared that at the same actual milk yield the CH4 emissions of Holstein herds and cows were slightly lower than those of the other breeds. This was in accordance with the lower CH4 intensity per kg corrected milk (Figure ), but also with the lower average energy content of Holstein milk. These results could be useful for better understanding the determinants and complex interactions resulting in CH4 emissions in commercial dairy herds.
How could FTIR EME predictions be used to mitigate the dairy sector’s carbon footprint?
The availability of a tool for rapidly and cheaply predicting EME traits of dairy cows at the population level has a threefold potential:
First, spectra of individual milk samples taken during milk recording can be used for the genetic improvement of dairy populations.
Second, spectra of bulk milk samples collected by dairy cooperatives from member farms for milk payment schemes can be used to determine possible premiums/penalties on the price of the milk in accordance with the environmental impact of each farm.
Third, almost all the milk produced for commercial purposes could be certified using predictions obtained from FTIR spectra of individual or bulk milk samples.
In all cases, an initial problem concerns the selection of the most useful EME trait to attain the desired target at the dairy chain level. At the commercial level, the objective would be to certify and apply penalties according to the amount of CH4 [or better, the global temperature potential to consider other greenhouse gases and the short lifetime of methane in the atmosphere (Mazzetto et al. Citation2022] emitted for every kg of milk (or dairy product) produced. The most useful trait in these cases is CH4 intensity.
Genetic improvement is a matter of debate (Knapp et al. Citation2014; Hristov et al. Citation2018; Beauchemin et al. Citation2020). The majority of publications in this area are concerned with daily CH4 production (Negussie et al. Citation2018). As genetic improvement concerns the animal, the objective should be to minimise the cow’s daily EME.
The use of FTIR spectrometry to develop prediction equations for estimating daily CH4 production from selected informative milk FA was investigated by Kandel et al. (Citation2015) using a procedure similar to the one used in this study. They estimated daily CH4 production from the detailed fatty acid profiles obtained from 602 milk samples analysed by gas chromatography, to which they applied four of the equations developed by Chilliard et al. (Citation2009) based on a study (8 cows, 4 diets, 32 balances) exploring the correlations between milk fatty acid profiles obtained by gas chromatography and daily CH4 production predicted using sulphur hexafluoride as tracer. They then regressed the predicted daily CH4 outputs on the FTIR spectra of the same milk samples to obtain four FTIR-based prediction equations. In the next step they applied these (Direct-IR) equations to a population database of 604,028 milk spectra (70,872 cows), then estimated the genetic parameters of the predicted EME traits. They concluded that ‘the study showed the feasibility of using MIR spectrometry results to predict fatty acid-derived CH4 indicator traits developed in the literature’, and that ‘the estimated genetic parameters of these traits suggested a potential phenotypic and genetic variability of the daily quantity of CH4 eructed by Holstein dairy cows’. In a later research using the same database (Kandel et al. Citation2017), they found that the phenotypic and genetic correlations between FTIR-predicted daily CH4 production and daily production of milk, fat and protein were generally modest and sometimes negative. This contradicts the frequent observation that higher daily milk yields go hand-in-hand with higher nutrient requirements and feed intake, hence greater CH4 production (von Soosten et al. Citation2020). The positive aspect of increasing daily milk yield is that CH4 production tends to increase less than proportionally, hence the CH4 intensity per kg of milk is expected to decrease. This means that the selection objective of dairy cow populations should not be to reduce daily CH4 production per animal (correlating with lower milk yields, and the rearing of more cows), but rather to reduce CH4 intensity, mainly by increasing production efficiency.
It is also important that when planning a strategy for mitigating the environmental impact of a given sector a holistic approach should be taken, as one action aimed at reducing a source of greenhouse gas could cause an increase in other emissions or a worsening of other impact categories (Gerber et al. Citation2013; Lynch Citation2019). Moreover, even when considering EME alone, it is essential to evaluate the correlations with fertility, longevity, and other welfare and health traits of the cows, as it has been shown that variations in these traits may have a more important effect on the sector’s EME than the variation expected from the genetic improvement of production traits (Van Middelaar et al. Citation2015; Llonch et al. Citation2017; von Soosten et al. Citation2020; Beauchemin et al. Citation2020).
Conclusions
The enteric CH4 emissions of dairy cows predicted at the population level from milk FTIR spectra and informative fatty acid proportions vary according to the predicted EME trait considered, the methodology used, the breed of cow, herd intensiveness, the productivity of individual cows within herd, and their interactions. Direct-IR (milk FTIR spectra → EME) and Indirect-IR (milk FTIR spectra → informative milk FA → EME) yield similar results when used to predict CH4 yield per kg of DMI and CH4 intensity per kg of fat-protein corrected milk. However, Direct-IR was found to be unreliable for predicting daily CH4 production in g/d, which could be more accurately estimated by multiplying the recorded daily corrected milk yield by the CH4 intensity per kg milk. Different breeds were differently affected by the level of herd intensiveness and, to a lesser degree, by the individual cow’s production level. These results offer a better understanding of the determinants and complex interactions that give rise to the CH4 emissions of commercial dairy herds, and could be useful for the genetic improvement of dairy cows, environmental certification of farms, and perhaps also for determining new milk payment schemes.
Ethical approval
The study was based on a dataset obtained from the milk recording systems of Italian dairy populations and did not include any direct handling of animals to take measurements or samples, and did not require authorisation by the University Ethical Committee.
Acknowledgments
We thank the Associazione Provinciale delle Organizzazioni Zootecniche Altoatesine/Vereinigung der Südtiroler Tierzuchtverbände and the Federazione Latterie Alto Adige/Sennereiver- band Südtirol (Bolzano/Bozen, Italy) for providing the data.
Disclosure statement
The authors declare that they have no known competing financial interests or personal relationships that could have appeared to influence the work reported in this paper
Data availability statement
The results and analyses presented in this paper freely available upon request.
Additional information
Funding
References
- Beauchemin KA, Ungerfeld EM, Eckard RJ, Wang M. 2020. Review: fifty years of research on rumen methanogenesis: lessons learned and future challenges for mitigation. Animal. 14(S1):S2–S16.
- Berton M, Bittante G, Zendri F, Ramanzin M, Schiavon S, Sturaro E. 2020. Environmental impact and efficiency of use of resources of different mountain dairy farming systems. Agric Syst. 181:102806.
- Bittante G. 2022. Effect of breed of cow, farm intensiveness, and cow’s productivity on infrared predicted milk urea. J Dairy Sci. 105(6):5084–5096. doi: 10.3168/jds.2021-21105.
- Bittante G, Cecchinato A. 2020. Heritability estimates of enteric methane emissions predicted from fatty acid profiles, and their relationships with milk composition, cheese-yield and body size and condition. Ital J Anim Sci. 19:114–126.
- Bittante G, Cecchinato A, Schiavon S. 2018. Dairy system, parity, and lactation stage affect enteric methane production, yield, and intensity per kilogram of milk and cheese predicted from gas chromatography fatty acids. J Dairy Sci. 101(2):1752–1766.
- Bittante G, Cecchinato A, Tagliapietra F, Schiavon S, Toledo-Alvarado H. 2021. Effects of breed, farm intensiveness and cow productivity level on cheese-making ability predicted using infrared spectral data at the population level. J Dairy Sci. 104(11):11790–11806. doi: 10.3168/jds.2021-20499
- Bittante G, Cipolat-Gotet C. 2018. Direct and indirect predictions of enteric methane daily production, yield, and intensity per unit of milk and cheese, from fatty acids and milk Fourier-transform infrared spectra. J Dairy Sci. 101(8):7219–7235.
- Bittante G, Cipolat-Gotet C, Cecchinato A. 2020. Genetic parameters of different FTIR-enabled phenotyping tools derived from milk fatty acid profile for reducing enteric methane emissions in dairy cattle. Animals. 10(9):1617–1654.
- Bougouin A, Appuhamy J, Ferlay A, Kebreab E, Martin C, Moate PJ, Benchaar C, Lund P, Eugène M. 2019. Individual milk fatty acids are potential predictors of enteric methane emissions from dairy cows fed a wide range of diets: approach by meta-analysis. J Dairy Sci. 102(11):10616–10631.
- Chilliard Y, Martin C, Rouel J, Doreau M. 2009. Milk fatty acids in dairy cows fed whole crude linseed, extruded linseed, or linseed oil, and their relationship with methane output. J Dairy Sci. 92(10):5199–5211.
- de Haas Y, Pszczola M, Soyeurt H, Wall E, Lassen J. 2017. Invited review: phenotypes to genetically reduce greenhouse gas emissions in dairying. J Dairy Sci. 100(2):855–870.
- Difford GF, Løvendahl P, Veerkamp RF, Bovenhuis H, Visker MHPW, Lassen J, de Haas Y. 2020. Can greenhouse gases in breath be used to genetically improve feed efficiency of dairy cows? J Dairy Sci. 103(3):2442–2459.
- Dijkstra J, van Zijderveld SM, Apajalahti JA, Bannink A, Gerrits WJJ, Newbold JR, Perdok HB, Berends H. 2011. Relationships between methane production and milk fatty acid profiles in dairy cattle. Anim Feed Sci Technol. 166-167:590–595.
- Eskildsen CE, Rasmussen MA, Engelsen SB, Larsen LB, Poulsen NA, Skov T. 2014. Quantification of individual fatty acids in bovine milk by infrared spectroscopy and chemometrics: understanding predictions of highly collinear reference variables. J Dairy Sci. 97(12):7940–7951.
- Ferragina A, de los Campos G, Vazquez AI, Cecchinato A, Bittante G. 2015. Bayesian regression models outperform partial least squares methods for predicting milk components and technological properties using infrared spectral data. J Dairy Sci. 98:8133–8151.
- Ferrand-Calmels M, Palhière I, Brochard M, Leray O, Astruc JM, Aurel MR, Barbey S, Bouvier F, Brunschwig P, Caillat H, et al. 2014. Prediction of fatty acid profiles in cow, ewe, and goat milk by mid-infrared spectrometry. J Dairy Sci. 97:17–35.
- Gerber PJ, Hristov AN, Henderson B, Makkar H, Oh J, Lee C, Meinen R, Montes F, Ott T, Firkins J, et al. 2013. Technical options for the mitigation of direct methane and nitrous oxide emissions from livestock: a review. Animal. 7(Suppl 2):220–234.
- Gottardo P, Penasa M, Righi F, Lopez-Villalobos N, Cassandro M, De Marchi M. 2017. Fatty acid composition of milk from holstein-friesian, brown swiss, simmental and alpine grey cows predicted by mid-infrared spectroscopy. Ital J Anim Sci. 16(3):380–389.
- Heck JML, van Valenberg HJF, Dijkstra J, van Hooijdonk ACM. 2009. Seasonal variation in the Dutch bovine raw milk composition. J Dairy Sci. 92:4745–4755.
- Hristov AN, Kebreab E, Niu M, Oh J, Bannink A, Bayat AR, Boland TM, Brito AF, Casper DP, Crompton LA, et al. 2018. Symposium review: uncertainties in enteric methane inventories, measurement techniques, and prediction models. J Dairy Sci. 101:6655–6674.
- ISO. 2013. ISO 9622:2013 Milk and liquid milk products - guidelines for the application of mid-infrared spectrometry 14. Available from: https://www.iso.org/standard/56874.html#:∼:text=ISO%209622%7CIDF%20141%3A2013,absorption%20of%20mid%2Dinfrared%20radiation.
- ISO. 2020. Milk and milk products - guidelines for the application of near infrared spectrometry, updated guideline. ISO 21543:2020. Available from: https://www.iso.org/standard/77606.html
- Kandel PB, Gengler N, Soyeurt H. 2015. Assessing variability of literature based methane indicator traits in a large dairy cow population. Biotechnol Agron Soc Environ. 19:11–19.
- Kandel PB, Vanrobays ML, Vanlierde A, Dehareng F, Froidmont E, Gengler N, Soyeurt H. 2017. Genetic parameters of mid-infrared methane predictions and their relationships with milk production traits in Holstein cattle. J Dairy Sci. 100:5578–5591.
- Knapp JR, Laur GL, Vadas PA, Weiss WP, Tricarico JM. 2014. Invited review: enteric methane in dairy cattle production: quantifying the opportunities and impact of reducing emissions. J Dairy Sci. 97:3231–3261.
- Lassen J, Løvendahl P, Madsen J. 2012. Accuracy of noninvasive breath methane measurements using Fourier transform infrared methods on individual cows. J Dairy Sci. 95:890–898.
- Llonch P, Haskell MJ, Dewhurst RJ, Turner SP. 2017. Current available strategies to mitigate greenhouse gas emissions in livestock systems: an animal welfare perspective. Animal. 11(2):274–284.
- Lynch J. 2019. Availability of disaggregated greenhouse gas emissions from beef cattle production: a systematic review. Environ Impact Assess Rev. 76:69–78.
- Maurice-Van Eijndhoven MHT, Bovenhuis H, Soyeurt H, Calus MPL. 2013. Differences in milk fat composition predicted by mid-infrared spectrometry among dairy cattle breeds in the Netherlands. J Dairy Sci. 96(4):2570–2582.
- Maurice-Van Eijndhoven MHT, Hiemstra SJ, Calus MPL. 2011. Short communication: milk fat composition of 4 cattle breeds in the Netherlands. J Dairy Sci. 94(2):1021–1025.
- Mazzetto AM, Falconer S, Ledgard S. 2022. Mapping the carbon footprint of milk production from cattle: a systematic review. J Dairy Sci. 105:22117.
- Mohammed R, McGinn SM, Beauchemin KA. 2011. Prediction of enteric methane output from milk fatty acid concentrations and rumen fermentation parameters in dairy cows fed sunflower, flax, or canola seeds. J Dairy Sci. 94(12):6057–6068.
- Moumen A, Azizi G, Ben Chekroun K, Baghour M. 2016. The effects of livestock methane emission on the global warming: a review. IJGW. 9(2):229–253.
- Negussie E, de Haas Y, Dehareng F, Dewhurst RJ, Dijkstra J, Gengler N, Morgavi DP, Soyeurt H, van Gastelen S, Yan T, et al. 2017. Invited review: large-scale indirect measurements for enteric methane emissions in dairy cattle: a review of proxies and their potential for use in management and breeding decisions. J Dairy Sci. 100(4):2433–2453.
- Negussie E, Gengler N, Pszczola M. 2018. Combining heterogeneous across-country data for prediction of enteric methane from proxies in dairy cattle Combining heterogeneous across-country data for prediction of enteric methane from proxies in dairy cattle. Proc World Congr Genet Appl to Livest Prod. 2:760. https://library.wur.nl/WebQuery/wurpubs/543527.
- Niermöller A, Holroyd S. 2019. Application of near infrared spectrometry for the analysis of milk and milk products. Bullettin IDF. 497(2019):1–19.
- NRC. 2001. Nutrient requirements of dairy cattle. Seventh revised ed. Washington (DC): National Academy Press.
- Pegolo S, Cecchinato A, Casellas J, Conte G, Mele M, Schiavon S, Bittante G. 2016. Genetic and environmental relationships of detailed milk fatty acids profile determined by gas chromatography in Brown Swiss cows. J Dairy Sci. 99:1315–1330.
- Pérez P, de los Campos G. 2014. Genome-wide regression and prediction with BLGR statistical package. Genetics. 198(2):483–495.
- Poulsen NA, Gustavsson F, Glantz M, Paulsson M, Larsen LB, Larsen MK. 2012. The influence of feed and herd on fatty acid composition in 3 dairy breeds (Danish Holstein, Danish Jersey, and Swedish Red). J Dairy Sci. 95(11):6362–6371. doi: 10.3168/jds.2012-5820
- Rico DE, Chouinard PY, Hassanat F, Benchaar C, Gervais R. 2016. Prediction of enteric methane emissions from Holstein dairy cows fed various forage sources. Animal. 10(2):203–211.
- Ross SA, Chagunda MGG, Topp CFE, Ennos R. 2014. Effect of cattle genotype and feeding regime on greenhouse gas emissions intensity in high producing dairy cows. Livest Sci. 170:158–171.
- Rovere G, de los Campos G, Lock AL, Worden L, Vazquez AI, Lee K, Tempelman RJ. 2021. Prediction of fatty acid composition using milk spectral data and its associations with various mid-infrared spectral regions in Michigan Holsteins. J Dairy Sci. 104(10):11242–11258.
- Schiavon S, Sturaro E, Tagliapietra F, Ramanzin M, Bittante G. 2019. Nitrogen and phosphorus excretion on mountain farms of different dairy systems. Agric Syst. 168:36–47.
- Shetty N, Difford G, Lassen J, Løvendahl P, Buitenhuis AJ. 2017. Predicting methane emissions of lactating Danish Holstein cows using Fourier transform mid-infrared spectroscopy of milk. J Dairy Sci. 100(11):9052–9060.
- Soyeurt H, Dardenne P, Gillon A, Croquet C, Vanderick S, Mayeres P, Bertozzi C, Gengler N. 2006. Variation in FA contents of milk and milk fat within and across breeds. J Dairy Sci. 89(12):4858–4865.
- Stocco G, Cipolat-Gotet C, Gasparotto V, Cecchinato A, Bittante G. 2018. Breed of cow and herd productivity affect milk nutrient recovery in curd, and cheese yield, efficiency and daily production. Animal. 12(2):434–444.
- Toledo-Alvarado H, Cecchinato A, Bittante G. 2017. Fertility traits of Holstein, Brown Swiss, Simmental, and Alpine Grey cows are differently affected by herd productivity and milk yield of individual cows. J Dairy Sci. 100(10):8220–8231.
- Toledo-Alvarado H, Pérez-Cabal MA, Tempelman RJ, Cecchinato A, Bittante G, de los Campos G, Vazquez AI. 2021. Association between days open and milk spectral data in dairy cows. J Dairy Sci. 104(3):3665–3675.
- Toledo-Alvarado H, Vazquez AI, de los Campos G, Tempelman RJ, Bittante G, Cecchinato A. 2018a. Diagnosing pregnancy status using infrared spectra and milk composition in dairy cows. J Dairy Sci. 101(3):2496–2505.
- Toledo-Alvarado H, Vazquez AI, de los Campos G, Tempelman RJ, Gabai G, Cecchinato A, Bittante G. 2018b. Changes in milk characteristics and fatty acid profile during the estrous cycle in dairy cows. J Dairy Sci. 101(10):9135–9153.
- van Engelen S, Bovenhuis H, Dijkstra J, van Arendonk JAM, Visker MHPW. 2015. Short communication: genetic study of methane production predicted from milk fat composition in dairy cows. J Dairy Sci. 98(11):8223–8226.
- van Gastelen S, Dijkstra J. 2016. Prediction of methane emission from lactating dairy cows using milk fatty acids and mid-infrared spectroscopy. J Sci Food Agric. 96(12):3963–3968.
- van Gastelen S, Mollenhorst H, Antunes-Fernandes EC, Hettinga KA, van Burgsteden GG, Dijkstra J, Rademaker JLW. 2018. Predicting enteric methane emission of dairy cows with milk Fourier-transform infrared spectra and gas chromatography–based milk fatty acid profiles. J Dairy Sci. 101(6):5582–5598.
- van Lingen HJ, Crompton LA, Hendriks WH, Reynolds CK, Dijkstra J. 2014. Meta-analysis of relationships between enteric methane yield and milk fatty acid profile in dairy cattle. J Dairy Sci. 97(11):7115–7132.
- van Middelaar CE, Berentsen PBM, Dijkstra J, Van Arendonk JAM, De Boer IJM. 2015. Effect of feed-related farm characteristics on relative values of genetic traits in dairy cows to reduce greenhouse gas emissions along the chain. J Dairy Sci. 98(7):4889–4903.
- Vanbergue E, Delaby L, Peyraud JL, Colette S, Gallard Y, Hurtaud C. 2017. Effects of breed, feeding system, and lactation stage on milk fat characteristics and spontaneous lipolysis in dairy cows. J Dairy Sci. 100(6):4623–4636.
- Vanlierde A, Vanrobays ML, Gengler N, Dardenne P, Froidmont E, Soyeurt H, McParland S, Lewis E, Deighton MH, Mathot M, et al. 2016. Milk mid-infrared spectra enable prediction of lactation-stage-dependent methane emissions of dairy cattle within routine population-scale milk recording schemes. Anim Prod Sci. 56(3):258–264.
- von Soosten D, Meyer U, Flachowsky G, Dänicke S. 2020. Dairy cow health and greenhouse gas emission intensity. Dairy. 1(1):3.
- Wang Q, Bovenhuis H. 2019. Validation strategy can result in an overoptimistic view of the ability of milk infrared spectra to predict methane emission of dairy cattle. J Dairy Sci. 102(7):6288–6295.