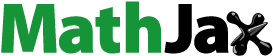
Abstract
The aim of this study was to estimate genetic parameters and investigate the genomic background of scrotal circumference and semen parameters in the Italian Simmental bulls. Scrotal circumference, number of normal spermatozoa, ejaculate volume, spermatozoa motility, and total spermatozoa were measured on 622 bulls, of which 603 had genotypes for 42,141 SNP. Variance components of scrotal circumference were estimated with an animal model that included the fixed effects of birth year, animal age, and measurement method, and the random effects of day of measurement and animal. In the model for the other traits, the scrotal circumference was added as a covariate to account for its influence on the semen parameters. A genome-wide association study was carried out using the ssGBLUP-approach. Heritabilities ranged from 0.07 ± 0.05 (spermatozoa motility) to 0.50 ± 0.14 (scrotal circumference). A total of 13 SNP passed the Bonferroni correction threshold and the number of significantly associated markers ranged from 1 (ejaculate volume and spermatozoa motility) to 5 (total spermatozoa). Genes already associated with reproduction parameters were retrieved close to the significant SNP. Results of the present study gave preliminary insights about the genetic determinism of semen quality in Italian Simmental bulls.
Low to moderate heritabilities were estimated for semen traits.
Few markers were associated with the phenotypes, suggesting their polygenic determinism.
Candidate genes already associated in literature with sperm traits were found.
Highlights
Introduction
The Italian Simmental (IS) is dual-purpose cattle breed mainly farmed in small herds located in the mountainous area of North-eastern Italy. The breeding program is handled by the Italian Simmental Breeders Association (ANAPRI). The beef aptitude is evaluated in approximately 250 young bulls every year through the performance test (Cesarani et al. Citation2020), where average daily gain, body size, muscularity, and feet and legs are evaluated. At the end of the performance test, the bulls are used for progeny testing for milk production traits through artificial insemination (the top 15% of the animals, about 20–30 bulls per year) or natural service (the top 16% to 25%); the remaining 60% are slaughtered. The composition of the dual-purpose selection index is: 44% milk production, 24% meat production, 19.5% morphology, and 12.5% fitness. Even if the IS breed is generally characterised by good fertility (e.g. quite short calving to conception interval and calving interval, low non-return rate), the genetic selection for milk production could reduce reproductive performances, because of the negative genetic correlation between these two traits (e.g. Walsh et al. Citation2011; Ma et al. Citation2019). In order to prevent or limit the reduction of fertility, the latter has been included in breeding programs worldwide (Philipsson and Lindhé Citation2003; Miglior et al. Citation2005). The genetic improvement of fertility is usually based on female phenotypes (such as conception or pregnancy rates, calving interval, and oestrus index) some of which are difficult to measure routinely at population level. Fertility problems could, however, also be due to male subfertility (Han and Peñagaricano Citation2016). Male fertility parameters are now routinely evaluated in the IS at the end of the PT. Thus, they could be quite easily incorporated into the breeding program. However, an important preliminary step is the assessment of the genetic variability and the genetic determinism of these traits. The aim of this work was to estimate the heritability of some male fertility traits in the IS breed and to investigate their genomic background using a genome-wide association study.
Materials and methods
Data
The study was carried out on a sample of 622 IS bulls intended for both artificial insemination or natural service. Available phenotypes were: scrotal circumference (SC), number of normal spermatozoa (NS), ejaculate volume (EV), spermatozoa motility (SM), total spermatozoa (TS). Since data were recorded in routine measurements at the end of the Performance Test, the Animal Care and Use Committee approval was unnecessary.
These male fertility traits were phenotypically analysed in Corte Pause et al. (Citation2022). Briefly, at the end of the performance test, the SC of each bull is measured transversely using a tape (Reliabull Scrotal Tape, Lane Manufacturing Inc., Denver, CO, USA) according to Hopkins and Spitzer (Citation1997).
At the ned of the PT, the top ranked bulls were examined for semen quality as outlined by the manual of the SFT (Society of Theriogenology; Koziol and Armstrong Citation2018). The volume (mL) of the ejaculation was measured using a 15 mL collection tube (code 62.554.502, Sarsted s.r.l., Italy) connected to the artificial vagina . Total motility (defined as the percentage of spermatozoa that exhibit motility of any form) and progressive motility (defined as the percentage of spermatozoa that are moving in a rapid linear fashion) were estimated visually, by an experienced veterinarian in charge of the Bull breeding soundness evaluation at the genetic centre, from 10 microscopic fields at 37 °C using a phase-contrast microscope at 200X magnification (CH 2, Olympus, Japan) equipped with a heated stage (HT 50, Minitube, Germany). For the motility evaluation, raw semen was first diluted to 1:60 in sterile 0.9% NaCl solution. Spermatozoa motility was evaluated after placing a 15 µL drop of semen on a pre-warmed glass slide, covered by a 20 × 20 mm coverslip. The spermatozoa concentration was measured using a photometer (Accucell, IMV-Tecnologies, France) by diluting 40 µl of semen in 3960 µl of 0.9% NaCl, and the total number of spermatozoa was calculated by multiplying the ejaculate volume for sperm concentration. For the morphological evaluation of spermatozoa, a smear was prepared by mixing 7 µL of raw semen with 90 µL of eosin-nigrosin solution. Morphological characteristics of spermatozoa (percentage of normal and abnormal spermatozoa) were assessed using bright-field microscopy, at 1000X magnification (Orthoplan, Leitz, Germany), by counting at least 200 sperm cells as defined by Barth and Oko (Citation1989). . Medium density SNP genotypes were available for 603 bulls. After quality control, 42,141 SNP mapped on the 29 autosomal chromosomes of the ARS-UCD 1.3 assembly were retained for the analysis. Phenotypes, pedigree, and genotypes were provided by ANAPRI.
Heritability estimation
Variance components were estimated using a pedigree-based animal model. The model used for the scrotal circumference was:
[1]
[1]
where: YoB was the fixed effect of the year of birth of the bull (9 levels); Age was the covariate of the age of the bull at the measurement day (average of 427 ± 19 days, from 384 to 499); Method was the fixed effect of the measurement method (two different types of tape); Date was the random effect of the measurement day (95 levels); animal was the random effect of the animal (3,044 total animals in the relationship matrix); e was the random residual effect.
The animal model adopted for the semen parameters was the following:
[2]
[2]
where YoB, Age, and Date are described above, and SC was the covariate of the scrotal circumference, that was included because of its strong influence on the semen parameters.
In both models, the animal effect was distributed as where A is the pedigree relationship matrix and
is the variance associated with the additive genetic effect. The random effect of the date was distributed as
where I is an identity matrix and
is the variance associated with the random effect of the measurement day. Finally, the random error term was distributed as
where I is an identity matrix and
is the variance associated with the residual error. As suggested by several studies (e.g. Lourenco et al. Citation2014; Pocrnic et al. Citation2017; Cesarani et al. Citation2021), the pedigree was traced back 3 generations from the animals with phenotypes and genotypes (total of 3,044 animals).
Variance components were estimated via a Gibbs sampling algorithm implemented in GIBBSF90+ program (Misztal et al. Citation2014). From a single chain with 100,000 samples, the first 10,000 were discarded as burn-in, and one sample every 10 was saved to compute means and standard deviations of the posterior distributions using POSTGIBBSF90 (Misztal et al. Citation2014). Heritability (h2) across date, i.e. not considering in the denominator the variance associated with the date of analysis, was computed as:
with the variances described above.
Genome-wide association study and gene mapping
The estimated variance components were used in the genome-wide association study (GWAS) carried out using single-step genomic approach (ssGBLUP; Legarra et al. Citation2009), where the A inverse is replaced by the H inverse (Aguilar et al. Citation2010), which is a relationship matrix that combines both pedigree (A) and the genomic relationships (G). Once the (G)EBV are estimated with the ssGBLUP, they can be backsolved to marker’s effects because of the equivalence between GBLUP and SNP-BLUP (Wang et al. Citation2012). Moreover, the individual SNP variances were estimated. Models [1] and [2] were used for the GWAS, with the animal effect distributed as where H is described above.
The p-values for all SNP were computed based on their prediction error variance according to Aguilar et al. (Citation2019) using the POSTGSF90 software (Misztal et al. Citation2014). A marker was considered significantly associated with p < 0.05. Moreover, the Bonferroni correction was applied as the ratio between alpha and the number of independent blocks, which was computed using the –blocks flag of PLINK (v. 1.9; Purcell et al. Citation2007). Using the NCBI online database (https://www.ncbi.nlm.nih.gov/genome/gdv/) genes mapped near each significant SNP (± 250 kb; Cesarani et al. Citation2019; Manca et al. Citation2020) were retrieved.
Results and discussion
Variance components estimation
Heritability for SC was of the same magnitude as in a report (0.46 ± 0.07) of a composite cattle population from Nebraska (Russell et al. Citation2021). Siqueira et al. (Citation2012) reported h2 of 0.45 ± 0.02 and 0.63 ± 0.05 for SC in Nellore bulls, offspring of super-precocious or precocious females, respectively. Lower h2 were estimated for SC in Mexican Charolais-Charbray (0.18 ± 0.02; Martínez-Velázquez et al. Citation2020) and in Red Angus (0.32 ± 0.02; McAllister et al. Citation2011) cattle breeds. Carvalho Filho et al. (Citation2020) analysed the genetic parameter for fertility traits in Nellore bulls and they reported h2 of 0.47 ± 0.12 for SC and 0.07 ± 0.08 for progressive sperm motility, which is very close to the h2 estimated in the present work for spermatozoa motility (0.07 ± 0.05). The heritability estimated for SM (Table ) was larger than in a report in Austrian Simmental (0.04 ± 0.01; Gredler et al. Citation2007), a population closely related to the IS. In the same study the authors reported h2 of 0.18 ± 0.02 and 0.22 ± 0.02 for ejaculate volume and total number of spermatozoa, respectively. Similar h2 were reported for ejaculate volume in a multi-breed cattle population (0.20 ± 0.04) by Berry et al. (Citation2019) and in Holsten bulls (0.22 ± 0.05) by Druet et al. (Citation2009), whereas lower h2 (0.09 ± 0.08) was estimated in Hereford bulls (Kealey et al. Citation2006). Heritability of 0.09 ± 0.04 was estimated for the number of spermatozoa in Holstein bulls (Druet et al. Citation2009), which is close to the estimate of the present study (0.11 ± 0.08).
Table 1. Heritability of the investigated traits.
The h2 estimates reported in literature for the analysed traits are quite heterogeneous, mainly because the male fertility traits are characterised by a larger variability compared to the female ones, as pointed out by Berry et al. (Citation2019). Typically, heritability for semen traits as well as other reproduction traits is lower (e.g. Ma et al. Citation2019), compared to morphological ones such as SC, which usually has moderate to high heritability. Moreover, these lower heritabilities estimated for the semen traits (i.e. normal spermatozoa, total spermatozoa and their motility, and ejaculate volume) compared to the SC was expected because the former are largely influenced by the environmental factors (Carvalho Filho et al. Citation2020). The quite large impact of the environment on the semen traits was considered in the present study by fitting the date of sampling as random effect in the model. The sampling date explained from 4% (EV) to 19% (SC and NS) of the total variance.
Genome-wide association study
Figure shows the Manhattan plot of the GWAS analyses. A total of 11,630 SNP was significant without the Bonferroni correction (Table ). The Bonferroni correction was implemented in the GWAS procedure to account for multiple comparisons and to decrease the false positive rate (Johnson et al. Citation2010). When the sample size is small (as the case of the present study) and the correction for the multitude of comparisons is too conservative (as the case of the Bonferroni correction) the rate of false negative could increase (Johnson et al. Citation2010). The Bonferroni correction is considered overly conservative also because close SNP are in linkage disequilibrium and, thus, they are correlated and tend to be inherited together in blocks that are not independent. In order to prevent possible over-corrections when applying Bonferroni (i.e. increase in the false negative rate), we used the number of independent blocks (N = 866) instead of the number of SNP in the denominator of the Bonferroni formula. After applying this Bonferroni correction,13 SNP were found to be significant in the GWAS analysis (dots above the red line in the Figure ): the number of markers significantly associated with each trait ranged from 1 for EBV and SM, to 5 fort TS, respectively.
Figure 1. Manhattan plot of the genome-wide association study results. The blue line indicates the significance threshold (0.05), whereas the red line indicates the Bonferroni threshold (0.05 divided by the number of blocks).

Table 2. Result of the genome-wide association study and markers found to be significant with or without the Bonferroni correction1.
Table also shows the percentage of the trait variance explained by the significant SNP before and after the Bonferroni correction, respectively. The variances ranged from 25.36% (NS) to 27.58% (EV) when considering the SNP before Bonferroni, and from 0.00% (NS) to EV (0.08%) after the correction. The latter is interesting because just one SNP (located on BTA10) can explain slightly less than 1% of the ejaculate volume; this could have interesting implications for the weighted version of the ssGBLUP (Zhang et al. Citation2016; Alvarenga et al. Citation2020).
Gene mapping
A total of 57 genes were mapped in the significant regions (Supplementary Table 1), some of which have been previously reported to be related to the sperm traits. GWAS on semen traits have been carried out in several cattle breeds (Peñagaricano et al. Citation2012; Puglisi et al. Citation2016; Sweett et al. Citation2020), as well as on other species such as sheep (Serrano et al. Citation2021), goats (Talouarn et al. Citation2020), and pigs (Diniz et al. Citation2014; Marques et al. Citation2018), in order to decipher their genomic backgrounds.
A GWAS carried out on Italian Holstein bull sperm quality (Puglisi et al. Citation2016) found a significant region on BTA18 at 16.77 Mb, which is very close to the region highlighted in the present study for SM (BTA18, 16.07–16.57 Mb). Cai et al. (Citation2017) analysed the male infertility in cattle yak and reported two genes found also in the present study: the CNTNAP2, located in the region on BTA4 significant for SC, and the CCL24, located in the region on BTA25 significant for NS. The upregulation of CCL24 in the scrotal skin could indicate a subclinical infection (González-Barrio et al. Citation2021). The ZP3 gene, located on chromosome 25 and associated with NS, has sperm-binding activity (Kanai et al. Citation2007).
Among the genes found in the significant regions, the PHKB located on BTA18 is of particular interest. The PHKB has been associated to spermatozoa motility, in pathways related to calcium homeostasis (Mahé et al. Citation2022); the relationship between calcium and several sperm functions is well known (Bernecic et al. Citation2019).
The MDH2 gene, which was found in the present study in a region associated with NS, was listed among the overexpressed genes in high-fertile bull spermatozoa (Aslam et al. Citation2018). This gene is involved in the final steps of the Krebs cycle, and its reduction may negatively impact the motility, capacitation, hyper-activation, and fertilising ability of spermatozoa. Verma et al. (Citation2014) reported the GCNT3 gene as potentially associated with sperm fertility in buffalo.
Two genes mapped in the regions on BTA5 associated with TS were previously associated with bull fertility traits: the WNT5B, reported to be associated with semen volume, number of sperm and sperm motility in a Thai multibreed dairy population (Sarakul et al. Citation2018), and the ADIPOR2, involved in the organisation of sperm structural and functional traits (Kasimanickam et al. Citation2013). In a GWAS for male fertility carried out in Holstein-Friesian bulls, the SPAST gene was reported to be associated with poor sperm motility (Hering et al. Citation2014). This gene was found in the present study among the candidate genes for TS. The abundance of GOSR1 gene, located on chromosome 19, in spermatozoal transcripts was associated with higher fertility dairy sires by Card et al. (Citation2017). The CD81 gene has implications in the intactness of the acrosome of spermatozoa (Jankovicova et al. Citation2016).
The POR gene, which is expressed exclusively in the late stages of spermatogenesis, has been associated with spermatids and spermatozoa in humans (Keber et al. Citation2013), whereas IGF-2, H19, and UPK3B genes were associated with sperm traits and maturation of gametes in mice (Yu et al. Citation2003; Kuriyama et al. Citation2017).
Interestingly, some of the genes found in the present study were previously found to be associated with female fertility in cattle. Killeen et al. (Citation2016) compared the endometrial gene expression in high and low fertility heifers, and they reported three genes found also in this study: TMIGD1, associated with TS, and GTF2A2 and GCNT3 associated with EV, respectively (Supplementary Table 1). The YWHAG gene, found in the present study associated with NS, is involved in the spontaneous abortions in cattle (Oliver et al. Citation2019) and it has also implications with intramammary infections (Chen et al. Citation2015). Finally, Guarini et al. (Citation2019) analysed the reproductive disorders in Canadian Holstein, and they reported three genes potentially related to cystic ovaries in cattle that were associated with NS in the present study: SRRM3, DTX2 and UPK3B genes.
Conclusions
Results of the present work highlighted the possibility of including male fertility parameters in the breeding program of the Italian Simmental cattle breed. The traits seem to be polygenic, with a small proportion of the genetic variance explained by many markers. A few markers were associated with the male fertility traits, and genes in these significant regions are of interest for future studies to further understand the biological pathways that affect male fertility traits.
Supplemental Material
Download MS Excel (14 KB)Disclosure statement
No potential conflict of interest was reported by the author(s).
Data availability statement
Restrictions apply for the datasets used for this study since they belong to the Italian Simmental Breeders Association (ANAPRI), and they were used under agreement for this research.
Correction Statement
This article has been corrected with minor changes. These changes do not impact the academic content of the article.
References
- Aguilar I, Legarra A, Cardoso F, Masuda Y, Lourenco D, Misztal I. 2019. Frequentist p-values for large-scale-single step genome-wide association, with an application to birth weight in American Angus cattle. Genet Sel Evol. 51(1):28.
- Aguilar I, Misztal I, Johnson DL, Legarra A, Tsuruta S, Lawlor TJ. 2010. Hot topic: a unified approach to utilize phenotypic, full pedigree, and genomic information for genetic evaluation of Holstein final score. J Dairy Sci. 93(2):743–752.
- Alvarenga AB, Veroneze R, Oliveira HR, Marques DB, Lopes PS, Silva FF, Brito LF. 2020. Comparing alternative single-step GBLUP approaches and training population designs for genomic evaluation of crossbred animals. Front Genet. 11:263.
- Aslam MM, Sharma VK, Pandey S, Kumaresan A, Srinivasan A, Datta TK, Mohanty TK, Yadav S. 2018. Identification of biomarker candidates for fertility in spermatozoa of crossbred bulls through comparative proteomics. Theriogenology. 119:43–51.
- Barth AD, Oko RJ. 1989. Abnormal morphology of bovine speramtozoa. 1st ed. Ames, IA: Iowa State University Press. p. 8–12.
- Bernecic NC, Gadella BM, Leahy T, de Graaf SP. 2019. Novel methods to detect capacitation-related changes in spermatozoa. Theriogenology. 137:56–66.
- Berry DP, Eivers B, Dunne G, McParland S. 2019. Genetics of bull semen characteristics in a multi-breed cattle population. Theriogenology. 123:202–208.
- Cai X, Yu S, Mipam T, Yang F, Zhao W, Liu W, Cao SZ, Shen L, Zhao F, Sun L, et al. 2017. Comparative analysis of testis transcriptomes associated with male infertility in cattleyak. Theriogenology. 88:28–42.
- Card CJ, Krieger KE, Kaproth M, Sartini BL. 2017. Oligo-dT selected spermatozoal transcript profiles differ among higher and lower fertility dairy sires. Anim Reprod Sci. 177:105–123.
- Carvalho Filho I, Marques DBD, de Campos CF, Guimarães JD, Guimarães SEF, Lopes PS, Fonseca e Silva F, Veroneze R. 2020. Genetic parameters for fertility traits in Nellore bulls. Reprod Domest Anim. 55(1):38–43.
- Cesarani A, Hidalgo J, Garcia A, Degano L, Vicario D, Masuda Y, Misztal I, Lourenco D. 2020. Beef trait genetic parameters based on old and recent data and its implications for genomic predictions in Italian Simmental cattle. J Anim Sci. 98(8):skaa242.
- Cesarani A, Masuda Y, Tsuruta S, Nicolazzi EL, VanRaden PM, Lourenco D, Misztal I. 2021. Genomic predictions for yield traits in US Holsteins with unknown parent groups. J Dairy Sci. 104(5):5843–5853.
- Cesarani A, Sechi T, Gaspa G, Usai MG, Sorbolini S, Macciotta NPP, Carta A. 2019. Investigation of genetic diversity and selection signatures between Sarda and Sardinian Ancestral black, two related sheep breeds with evident morphological differences. Small Rumin Res. 177:68–75.
- Chen X, Cheng Z, Zhang S, Werling D, Wathes DC. 2015. Combining genome wide association studies and differential gene expression data analyses identifies candidate genes affecting mastitis caused by two different pathogens in the dairy cow. OJAS. 05(04):358–393.
- Corte Pause F, Crociati M, Urli S, Monaci M, Degano L, Stradaioli G. 2022. Environmental factors affecting the reproductive efficiency of Italian simmental young bulls. Animals. 12(18):2476.
- Diniz DB, Lopes MS, Broekhuijse MLWJ, Lopes PS, Harlizius B, Guimarães SEF, Duijvesteijn N, Knol EF, Silva FF. 2014. A genome-wide association study reveals a novel candidate gene for sperm motility in pigs. Anim Reprod Sci. 151(3-4):201–207.
- Druet T, Fritz S, Sellem E, Basso B, Gerard O, Salas‐Cortes L, Humblot P, Druart X, Eggen A. 2009. Estimation of genetic parameters and genome scan for 15 semen characteristics traits of Holstein bulls. J Anim Breed Genet. 126(4):269–277.
- González-Barrio D, Diezma-Díaz C, Gutiérrez-Expósito D, Tabanera E, Jiménez-Meléndez A, Pizarro M, González-Huecas M, Ferre I, Ortega-Mora LM, Álvarez-García G. 2021. Identification of molecular biomarkers associated with disease progression in the testis of bulls infected with Besnoitia besnoiti. Vet Res. 52(1):106.
- Gredler B, Fuerst C, Fuerst‐Waltl B, Schwarzenbacher H, Sölkner J. 2007. Genetic parameters for semen production traits in Austrian dual‐purpose Simmental bulls. Reprod Domest Anim. 42(3):326–328.
- Guarini AR, Lourenco DAL, Brito LF, Sargolzaei M, Baes CF, Miglior F, Misztal I, Schenkel FS. 2019. Genetics and genomics of reproductive disorders in Canadian Holstein cattle. J Dairy Sci. 102(2):1341–1353.
- Han Y, Peñagaricano F. 2016. Unraveling the genomic architecture of bull fertility in Holstein cattle. BMC Genet. 17(1):143.
- Hering DM, Olenski K, Kaminski S. 2014. Genome-wide association study for poor sperm motility in Holstein-Friesian bulls. Anim Reprod Sci. 146(3-4):89–97.
- Hopkins FM, Spitzer JC. 1997. The new Society for Theriogenology Breeding Soundness Evaluation System. Vet Clin North Am Food Anim Pract. 13(2):283–293.
- Jankovicova J, Frolikova M, Sebkova N, Simon M, Cupperova P, Lipcseyova D, Michalkova K, Horovska L, Sedlacek R, Stopka P, et al. 2016. Characterization of tetraspanin protein CD81 in mouse spermatozoa and bovine gametes. Reproduction. 152(6):785–793.
- Johnson RC, Nelson GW, Troyer JL, Lautenberger JA, Kessing BD, Winkler CA, O'Brien SJ. 2010. Accounting for multiple comparisons in a genome-wide association study (GWAS). BMC Genomics. 11(1):1–6.
- Kanai S, Yonezawa N, Ishii Y, Tanokura M, Nakano M. 2007. Recombinant bovine zona pellucida glycoproteins ZP3 and ZP4 coexpressed in Sf9 cells form a sperm‐binding active hetero‐complex. Febs J. 274(20):5390–5405.
- Kasimanickam VR, Kasimanickam RK, Kastelic JP, Stevenson JS. 2013. Associations of adiponectin and fertility estimates in Holstein bulls. Theriogenology. 79(5):766–777.
- Kealey CG, MacNeil MD, Tess MW, Geary TW, Bellows RA. 2006. Genetic parameter estimates for scrotal circumference and semen characteristics of Line 1 Hereford bulls. J Anim Sci. 84(2):283–290.
- Killeen AP, Diskin MG, Morris DG, Kenny DA, Waters SM. 2016. Endometrial gene expression in high-and low-fertility heifers in the late luteal phase of the estrous cycle and a comparison with midluteal gene expression. Physiol Genomics. 48(4):306–319.
- Koziol JH, Armstrong CL. 2018. Manual for Breeding Soundness Examination of Bulls. 2nd ed. Society of Theriogenol-ogy: mathews, Alabama, USA, 33-37, p. 84–113.
- Kuriyama S, Tamiya Y, Tanaka M. 2017. Spatiotemporal expression of UPK3B and its promoter activity during embryogenesis and spermatogenesis. Histochem Cell Biol. 147(1):17–26.
- Legarra A, Aguilar I, Misztal I. 2009. A relationship matrix including full pedigree and genomic information. J Dairy Sci. 92(9):4656–4663.
- Lourenco DAL, Misztal I, Tsuruta S, Aguilar I, Lawlor TJ, Forni S, Weller JI. 2014. Are evaluations on young genotyped animals benefiting from the past generations? J Dairy Sci. 97(6):3930–3942.
- Ma L, Cole JB, Da Y, VanRaden PM. 2019. Symposium review: genetics, genome-wide association study, and genetic improvement of dairy fertility traits. J Dairy Sci. 102(4):3735–3743.
- Mahé C, Lavigne R, Com E, Pineau C, Locatelli Y, Zlotkowska AM, Alminana C, Tsikis G, Mermillod P, Schoen J, et al. 2022. Spatiotemporal profiling of the bovine oviduct fluid proteome around the time of ovulation. Sci Rep. 12(1):4135.
- Manca E, Cesarani A, Gaspa G, Sorbolini S, Macciotta NPP, Dimauro C. 2020. Use of the multivariate discriminant analysis for genome-wide association studies in cattle. Animals. 10(8):1300.
- Marques DB, Bastiaansen JW, Broekhuijse ML, Lopes MS, Knol EF, Harlizius B, Guimarães SEF, Silva FF, Lopes PS. 2018. Weighted single-step GWAS and gene network analysis reveal new candidate genes for semen traits in pigs. Genet Sel Evol. 50(1):1–14.
- Martínez-Velázquez G, Ríos-Utrera A, Román-Ponce SI, Baeza-Rodríguez JJ, Arechavaleta-Velasco ME, Montaño-Bermúdez M, Vega-Murillo VE. 2020. Genetic correlations between scrotal circumference, heifer fertility and stayability in Charolais–Charbray cattle. Livest Sci. 232:103914.
- McAllister CM, Speidel SE, Crews DH, Jr, Enns RM. 2011. Genetic parameters for intramuscular fat percentage, marbling score, scrotal circumference, and heifer pregnancy in Red Angus cattle. J Anim Sci. 89(7):2068–2072.
- Miglior F, Muir BL, Van Doormaal BJ. 2005. Selection indices in Holstein cattle of various countries. J Dairy Sci. 88(3):1255–1263.
- Misztal I, Tsuruta S, Lourenco D, Aguilar I, Legarra A, Vitezica Z. 2014. Manual for BLUPF90 family of programs. University of Georgia, Athens, GA.
- Oliver KF, Wahl AM, Dick M, Toenges JA, Kiser JN, Galliou JM, Moraes JGN, Burns GW, Dalton J, Spencer TE, et al. 2019. Genomic Analysis of Spontaneous Abortion in Holstein Heifers and Primiparous Cows. Genes. 10(12):954.
- Peñagaricano F, Weigel KA, Khatib H. 2012. Genome‐wide association study identifies candidate markers for bull fertility in Holstein dairy cattle. Anim Genet. 43:65–71.
- Philipsson J, Lindhé B. 2003. Experiences of including reproduction and health traits in Scandinavian dairy cattle breeding programmes. Livest. Prod. Sci. 83(2-3):99–112.
- Pocrnic I, Lourenco DAL, Bradford HL, Chen CY, Misztal I. 2017. Impact of pedigree depth on convergence of single-step genomic BLUP in a purebred swine population. J Anim Sci. 95(8):3391–3395.
- Puglisi R, Gaspa G, Balduzzi D, Severgnini A, Vanni R, Macciotta NPP, Galli A. 2016. Genomewide analysis of bull sperm quality and fertility traits. Reprod Domest Anim. 51(5):840–843.
- Purcell S, Neale B, Todd-Brown K, Thomas L, Ferreira MAR, Bender D, Maller J, Sklar P, de Bakker PIW, Daly MJ, et al. 2007. PLINK: a toolset for whole-genome association and population-based linkage analysis. Am J Hum Genet. 81:559–575.
- Keber R, Rozman D, Horvat S. 2013. Sterols in spermatogenesis and sperm maturation. J Lipid Res. 54(1):20–33.
- Russell CA, Pollak EJ, Spangler ML. 2021. Genetic parameter estimates for bull prolificacy and its relationship with scrotal circumference in a commercial beef cattle population. Transl Anim Sci. 5(3):txab128.
- Sarakul M, Elzo MA, Koonawootrittriron S, Suwanasopee T, Jattawa D, Laodim T. 2018. Characterization of biological pathways associated with semen traits in the Thai multibreed dairy population. Anim Reprod Sci. 197:324–334.
- Serrano M, Ramón M, Calvo JH, Jiménez MÁ, Freire F, Vázquez JM, Arranz JJ. 2021. Genome-wide association studies for sperm traits in Assaf sheep breed. Animal. 15(2):100065.
- Siqueira J, Oba E, Pinho R, Quintino H, Eler J, Miranda Neto T, Guimarães S, Guimarães J. 2012. Heritability Estimate and Genetic Correlations of Reproductive Features in Nellore Bulls, Offspring of Super Precocious, Precocious and Normal Cows Under Extensive Farming Conditions. Reprod Domest Anim. 47(2):313–318.
- Sweett H, Fonseca PAS, Suárez-Vega A, Livernois A, Miglior F, Cánovas A. 2020. Genome-wide association study to identify genomic regions and positional candidate genes associated with male fertility in beef cattle. Sci Rep. 10(1):1–14.
- Talouarn E, Bardou P, Palhière I, Oget C, Clément V, Tosser-Klopp G, Rupp R, Robert-Granié C, The VarGoats Consortium 2020. Genome wide association analysis on semen volume and milk yield using different strategies of imputation to whole genome sequence in French dairy goats. BMC Genet. 21(1):1–13.
- Verma A, Rajput S, De S, Kumar R, Chakravarty AK, Datta TK. 2014. Genome-wide profiling of sperm DNA methylation in relation to buffalo (Bubalus bubalis) bull fertility. Theriogenology. 82(5):750–759.e1.
- Walsh SW, Williams EJ, Evans ACO. 2011. A review of the causes of poor fertility in high milk producing dairy cows. Anim Reprod Sci. 123(3-4):127–138.
- Wang H, Misztal I, Aguilar I, Legarra A, Muir WM. 2012. Genome-wide association mapping including phenotypes from relatives without genotypes. Genet Res (Camb). 94(2):73–83.
- Yu Z, Guo R, Ge Y, Ma J, Guan J, Li S, Sun X, Xue S, Han D. 2003. Gene expression profiles in different stages of mouse spermatogenic cells during spermatogenesis. Biol. Reprod. 69:37–47.
- Zhang X, Lourenco D, Aguilar I, Legarra A, Misztal I. 2016. Weighting strategies for single-step genomic BLUP: an iterative approach for accurate calculation of GEBV and GWAS. Front Genet. 7:151.