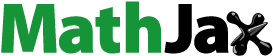
Abstract
Mastitis is one of the most significant diseases affecting dairy cattle profitability. A genome wide association study (GWAS) was performed using the selective genotyping approach to identify Quantitative Trait Loci (QTL) associated with mastitis susceptibility. According to the received antibiotic treatments in all their productive life, 52 lactating cows have been classified in resistant (no therapy and low SCC) and susceptible (more than one treatment and high SCC) to mastitis. Genotyping of animals was performed using the NEOGEN’s GGP Bovine 100K SNP chip and QTL were identified comparing the SNPs allelic frequencies between the two groups. 26 SNPs related to mastitis susceptibility were identified in 9 chromosomes. The use of treatment data, coupled with SCC from milk recording, improved cow’s classification accuracy in resistant and susceptible individuals. For this reason, the mandatory recording of treatments active from January 2022, could be a new source of information to improve the genetic selection for mastitis resistance.
Introduction
Mastitis is one of the most significant diseases affecting dairy cattle herds’ profitability and is normally treated with antibiotic therapy. As reported by Mitchell et al. (Citation1998) the most common use of antibiotic drugs in dairy cattle farming is to treat mastitis infection. In fact, clinical mastitis in lactating cows is mainly treated with intramammary administration of antibiotic while in severe cases of mastitis antibiotics are also used parenterally (Merle et al. Citation2013; Oliveira and Ruegg Citation2014; Gomes and Henriques Citation2016).
The blanket therapy method to reduce the prevalence of intramammary infections, foresee that all the animals of a herd are treated with antibiotic during the dry period. This practice led to an increase in bacteria antimicrobial resistance (Kabera et al. Citation2021). The Regulation (EU) 2019/6 on veterinary medicinal products, entered in to force in January 2022, promotes a more conscious use of antibiotic products (https://eur-lex.europa.eu/legal-content/EN/TXT/PDF/?uri=CELEX:32019R0006&from=EN). To reduce the use of antibiotics, in the recent years, the selective dry method has been implemented in dairy farms. According to this approach only cows that show mastitis symptoms during lactation are treated.
Immune response to mastitis is a complex trait under the control of multiple genes (Naserkheil et al. Citation2022). With the availability of high throughput genotyping technologies, genome-wide association studies (GWAS) have become a useful tool for fine-scale quantitative trait loci (QTL) mapping (Hayes et al. Citation2009). The discovery of genomic regions that influence quantitative (complex) traits may provide an opportunity to gather new information about gene function, and lead to a better understanding of how they interact to impact physiology and immune response to infectious diseases (Sharifi et al. Citation2020). In the recent past, many studies identified QTL affecting mastitis susceptibility in dairy cattle, using genetically correlated measures such as Somatic Cell Count (SCC) and its logarithmic transformation Somatic Cell Score (SCS) (Sahana et al. Citation2014; Welderufael et al. Citation2018; Meier et al. Citation2020).
Given the complexity of this trait, genetic selection to increase immune resistance to mastitis in dairy cattle populations has been developed for decades using, as indicator, milkt SCC (Thompson-Crispi et al. Citation2014; Moretti et al. Citation2021).
Selective DNA pooling (Darvasi and Soller Citation1994) is a recognised method used by several authors in GWAS analyses to map QTL either in case vs control studies or applied to complex traits (Strillacci et al. Citation2014; Lipkin et al. Citation2016; Peletto et al. Citation2017; Kurz et al. Citation2019). This approach is based on the theoretical demonstration that most information deriving from a GWAS depends on the marker allelic frequencies of the best and worst individuals according to the phenotype distribution in the population: with a maximum of 25% per tail, the less and more extreme is the proportion of considered individuals, the more powerful is the statistical analysis (Darvasi and Soller Citation1992). With the selective genotyping and DNA pooling approach, it’s then possible to genotype only individuals showing the most extreme values in an extended sampling, thus reducing the costs of genomic analysis and maintaining high statistical power.
A key factor in a GWAS to disclose QTL associated to mastitis resistance is to accurately classify cows in resistant and susceptible. In this study, to achieve an accurate classification of resistant and susceptible cows to mastitis, we proposed an innovative selection criterion based on multiple information on the same individual along all its productive life. The criterion here used has been based on the antibiotic treatments for mastitis across all the lactations of each cow, coupled with the available SCC data from routine milk tests registered during cows’ productive life. We performed then a GWAS using a selective genotyping of individuals and the DNA pooling design approach and statistics to identify QTL associated with mastitis susceptibility in Holstein dairy cows.
Materials and methods
Ethical issue
All animal procedures performed in this study were carried out in accordance with the guidelines of the care and use of laboratory animals established by the Italian and UE laws (D. Lgs n. 2014/26, 2010/63/UE); the project operative procedures on animals were approved by the Animal Welfare Committee of the Università degli Studi di Milano (OPBA) protocol number 160_2019.
Sampling and genotyping
For this study, data from a total of 170 adult lactating Holstein cows from a single herd with at least 2 completed lactations were used. All the antibiotic treatments for mastitis done on each animal recorded by the owner, a professional veterinarian, as well as the SCC data from routine milk recording scheme were available for each cow since 2014 (the oldest cow was of 7th parity).
The use of data of only one herd allows to eliminate any environmental difference among management. Additionally, the owner is a professional veterinarian capable as such to clearly identify mastitis cases to be treated.
Animals were classified as susceptible and resistant using as primary criterion the antibiotic treatments provided to animals for mastitis: i) resistant cows didn’t receive any treatment along their productive life; ii) susceptible cows received at least one treatment.
In addition, the information from milk SCC has been considered. Milk SCC values were from the official milking recording by ARAL, the Lombardy region farmers association (data available for each cow according to the AT4 ICAR system for all lactations). All the registered data for SCC for all the lactations of each cow were investigated to identify peaks of SCC simultaneously to an antibiotic treatment. This information has been used to assign a Case Score (CS) as follows: CS = 1 for any SCC test higher than 1 million cells/mL; CS = 0.5 for any SCC value between 400,000 and 1 million cells/mL; CS = 0 for SCC values below 400,000 cells/mL. The cut-off of 400,000 cells/mL has been chosen because representative of clinical mastitis events according to (Beaudeau et al. Citation1997).
More to less susceptible cows were then ranked according to number of treatments received (from zero to 9) and, within treatments CS (from zero to 6) (see Table to visualise the CS values attributed to each cow).
Table 1. Genome Wide Association Study (GWAS) results: SNPs over the Bonferroni (bold) and False Discovery Rate (FDR) genome wide thresholds (0.05 significance value) together with candidate genes.
There were 26 cows with no treatment and CS equal zero. Consequently 26 susceptible cows were identified according to number of treatments and CS. These cows represented the 15% best and worst cows, in the normal distribution of the population’s phenotypes, respect to the trait ‘resistance to mastitis’. The individual EBVs for SCC were explored to compare consistency with additive genetic values.
The 26 resistant and 26 susceptible cows (Table ) were then sampled using a Tissue Sampling Unit to collect ear tissue. DNA was obtained using the Quick-DNA™ Miniprep Kit of Zymo Research (Zymo Research Corporation). SNP genotyping was performed on each individual with the NEOGEN’s GGP Bovine 100K. Only SNPs located on the 29 autosomes, according to the bovine genome assembly ARS-UCD1.2, were considered in this study.
Statistical analysis
According to the selective DNA pooling design, both the resistant (R) and the susceptible (S) cows were randomly divided in two subgroups (R1 and R2 and S1 and S2, respectively), each of them representing a biological replicate. For each of the 4 subgroups (R1, R2, S1 and S2), the allele frequency at each marker was obtained. The frequency of alleles in each subgroup was obtained from the individual genotyping.
The frequencies were then analysed as in the selective DNA pooling design proposed by (Darvasi and Soller Citation1994). Here, instead of pooling before the genotyping, we have the individual genotyping data to calculate the allele frequency, reducing as such the possible error in DNA pooling and allele frequency estimate from pools and maintaining the power and the properties of the experimental design and analysis.
The multiple markers test
The statistical analysis was performed using the A allele frequencies at each marker, one of the two possible alleles (A or B) in a genotype. The frequencies were obtained separately for each subgroup of the tails (R1, R2, S1, S2), using the ‘genotype statistics by marker’ function of Golden Helix’s SVS software (SNP and Variation Suite v8.9 module, Golden Helix Inc., Bozeman, MT, USA).
We performed the GWAS analysis comparing the average A allele frequency at each SNP of resistant individuals with those of the susceptible ones, using an in-house script of the R software (version 4.0.5). Monomorphic markers were filtered out because non informative. Additionally, those SNPs lying in the top 5% of the absolute value of allele difference between replicates (i.e. R1 vs R2 and S1 vs S2) were also excluded from the analysis. After this editing, out of the 89,784 SNPs on the autosomes 58,285 SNPs were available for the association analysis.
A single-marker test for the marker-trait association was used, and the p-value for each marker was calculated as follows:
(1)
(1)
where Dtest is the difference of the A allele frequencies means among tails, and Dnull is the difference of the A allele frequencies means within tails.
The association analysis results were then visualised through a Manhattan plot made with the R package ‘qqman’ (Turner Citation2014). Both the Bonferroni and the FDR genome wide correction were used to set the 5% significance thresholds.
Gene annotation and functional analyses
The multi-species SNPchiMp v.3 database (Nicolazzi et al. Citation2015) was used to convert the Illumina SNP name of significant markers in the SNP rsID code (Reference SNP cluster ID).
The Variant Effect Predictor (VEP) tool of Ensembl database (https://www.ensembl.org/Tools/VEP) allowed to annotate the significant SNPs through the rsID codes according to the Bos taurus genome assembly ARS-UCD1.2 (Annotation Release: 106). The gene annotation analysis was performed using the Database for Annotation, Visualisation and Integrated Discovery – DAVID (https://david.ncifcrf.gov/home.jsp) with default parameters.
Results and discussions
One of the main weakness in QTL analysis for mastitis is the availability of accurate phenotype to cluster animals in susceptible and resistant. Genetic improvement has been performed based on SCC from milk recording tests. Nevertheless, the sole use of SCC data from milk recording to identify mastitis cases is suboptimal: a mastitis case can in fact happen within two subsequent milk recording tests occurring every 4 weeks in the AT4 system.
In this study, to classify in an accurate manner susceptible and resistant animals to mastitis, we used veterinary treatments provided to each cow along their complete productive life. To minimise false positive (and negatives) we considered only cows with at least 2 completed lactations. The resistant in particular (Table ) were cows with at least 3 lactations without any treatment. To make the classification more robust we coupled the treatment with the SCC. The expectation was towards high SCC in correspondence of treatment cases. This was always the case in the 26 individuals selected in this study as susceptible, except one. This individual received 2 treatments in 3 lactations: the date of treatments was in between two subsequent milk recording tests for SCC.
All resistant individuals had CS equal zero and the average EBV for SCC was 106.5, with all EBVs greater than 100, value representing the population's genetic basis. The susceptible had an average EBV for SCC of 99.2, but several of them had positive value (i.e. greater than 100). A possible interpretation is that the genetic evaluation is very effective in identifying resistant to mastitis individuals, while the susceptible ones may be misidentified due to the AT4 method. For this reason, the classification usually done in susceptible vs resistant animals based on EBV may be suboptimal in GWAS aimed to map QTL.
In Figure the scheme of the sampling and of the experimental design is summarised according to the criteria here used to cluster individuals in susceptible and resistant, i.e. case to control study.
Figure 1. Experimental design scheme: Susceptible (S1 and S2) and Resistant (R1 and R2) cows in a Selective genotyping and pooling approach.

GWAS results
The Manhattan plot in Figure shows the -log10(p) of the GWAS analysis with the Bonferroni (red line) and the FDR (blue line) genome wide thresholds, both set at 0.05 significance value. The 3 SNPs over the red threshold are located in chromosomes 16 and 28.
Figure 2. Graphical representation (Manhattan plot) of Genome Wide Association Study (GWAS) results. Horizontal lines represent the Bonferroni (red line) and the False Discovery Rate (FDR) (blue line) genome wide thresholds, both set at 0.05 significance value.

We considered as QTL the region of about 400,000 base pair located upstream and downstream (±200,000 bp) each significant SNP (Table S2). In Table , we reported the significant SNP and the genes falling within these regions. Twenty-six SNPs (annotated on 9 chromosomes) resulted located above the 5% FDR genome wide threshold (in bold, the ones over the 5% Bonferroni threshold). Among these SNPs, 16 were intragenic, all located in intronic positions. Only 10 SNPs lied between genes (intergenic). Gene enrichment results for the genes listed in Table (±200,000 bp) are reported in Table S3.
As shown in Figure and in concordance with available literature, 21 of the genes listed in Table were already associated with immune response and mastitis resistance (n = 12), with adipogenesis and feed efficiency traits (n = 5) and with productive and reproductive traits (n = 4).
Genes involved in mastitis and in immune response
Among the genes associated with immune response and with mastitis, the PTK2B – Protein Tyrosine Kinase 2 Beta – gene plays a role in the regulation of humoral immune response and is required for macrophage polarisation and migration towards sites of inflammationFootnote1. This gene resulted associated with clinical mastitis in Holstein Chinese cattle via GWAS and through a Post-transcriptional Analysis (Yang et al. Citation2019). The same authors found that the PTK2B gene was down-regulated in peripheral blood leukocytes of cows affected by clinical mastitis as well as in vitro lipopolysaccharides (E. coli) stimulated bovine mammary epithelial cells.
Other genes were found differentially expressed in case-control studies focused on mastitis resistance: the EPHX2 gene – Epoxide Hydrolase 2 – (Li T et al. Citation2019), the PLXNA2 gene – Plexin A2 – (Asselstine Citation2021), the DGL2 gene – Discs Large MAGUK Scaffold Protein 2 – (Wang et al. Citation2020), and the LYST gene – Lysosomal Trafficking Regulator – (Naserkheil et al. Citation2022). This last gene encodes for a protein involved in the transport of molecules into and from lysosomes and from lysosome-related organelles: loss of its function lead to the development of abnormally large lysosomes, and when the immune cells are involved, these altered lysosomes could interfere with the immune response to pathogens (Westphal et al. Citation2017).
Naderi et al. (Citation2018) applying a random forest statistical approach, identified the GAS1 – Growth Arrest Specific 1 – as candidate gene involved in clinical mastitis resistance; the role of GAS1 in morphology and physiology mechanisms proper of the udder has been suggested by (Jaggi et al. Citation1996). Finally, the PTPRZ1 – Protein Tyrosine Phosphatase Receptor Type Z1 – is one of the genes mapped closely to genomic regions found to be statistically associated with somatic cell content in dairy cows (Chen et al. Citation2015).
The remaining genes reported in Figure are all involved in immune response: the LGALS8 (Galectin-8) gene encodes for a membrane receptor with the ability to activate intracellular bacterial killing and it would seem to have an important role in neutrophils migration into target tissues (Lopreiato et al. Citation2020). The AGK (Acylglycerol Kinase) gene is indispensable for the metabolic reprogramming of CD8+ T cell and for their function in immune responses (Hu et al. Citation2019); the TRIM35 (Tripartite motif TRIM35) gene has an important role on innate immunity against viral infections (Sun et al. Citation2020). Finally, also for PRKCQ Protein kinase C theta (PKC-θ) and PPP2R2A (Protein Phosphatase 2 Regulatory Subunit Balpha) genes an immunity role has been reported, as indicated in human studies (Anel et al. Citation2012; Prince et al. Citation2020; Li Z et al. Citation2021).
Genes involved in adipogenesis and feed efficiency traits
Food represents the energy input that the body uses for physiological or to cope with pathological processes, and for this reason feed efficiency is considered one of the most important characteristics in animal husbandry (Friggens et al. Citation2013). Over the years, several authors investigated a possible link between a prompt immune response and high feed efficiency in different species (Vigors et al. Citation2016; Zerjal et al. Citation2021). Among the genes identified in this study as possible candidate ones for mastitis resistance, two have been previously associated with feed efficiency traits: ETS1 – ETS Proto-Oncogene 1, Transcription Factor – (De Lima et al. Citation2020) and IPO11 – Importin 11. This latter is part of a group of genes harbouring SNPs that are part of a patented marker panel to select animals for feed efficiency.Footnote2 IPO11 is also a nearby gene associated with SCS (Somatic Cell Score) in Holstein cattle (Welderufael et al. Citation2018).
Moreover, the PCP4 gene (Purkinje cell protein 4) has been identified as one of the major genes regulating feed efficiency in beef cattle (Machugh et al. Citation2019) and Amaral et al. (Citation2019) found that the DSCAM – DS cell adhesion molecule – regulates the Residual Feed Intake in pigs. The homolog of this gene (DSCAM) in insects and crustaceans have an immunity function regulating their immune response to pathogens (Ng and Kurtz Citation2020); even though the results of this study make us speculate that this gene could have an immunity role in the bovine species, there are no evidence of this function in the vertebrates.
The EBF2 (B-cell factor 2) gene promotes differentiation of brown adipocytes and controls its adaptive cold response (Angueira et al. Citation2020). Several studies have shown that adipocytes have immunological functions capable of employing and activating immune cells: adipocyte in fact, would appear to be an antigen-presenting cell (APC) expressing MHC class I and II molecules (Song and Deng Citation2020).
Genes involved in productive and reproductive traits
Over the years, selection for increased production in specialised dairy breeds, such as the Holstein one, determined a correlated genetic response causing a reduction in reproductive efficiency and in the ability to cope with diseases and infections, such as mastitis.
In this research two candidate genes for mastitis resistance (RSU1 - Ras Suppressor Protein 1; B3GALNT2 - β-1,3-N-acetylgalactosaminyltransferase 2) were previously reported as associated with milk yield (Farhadian et al. Citation2018; Poulsen et al. Citation2019). The CACNB2 (Calcium Voltage-Gated Channel Auxiliary Subunit Beta 2) gene here found associated with mastitis resistance was previously found associated with reproductive traits (Sammad et al. Citation2022) as well as the WEE2 (oocyte meiosis inhibiting kinase, also known as WEE1B) gene that has been associated with oocyte development in donkeys (Zhang et al. Citation2022) and fertilisation failure in pigs and humans (Shimaoka et al. Citation2009; Hanna et al. Citation2020).
The existence of a clear genetic correlation between mastitis with production and fertility may explain why the genes here found as candidate ones for mastitis resistance were previously reported for other traits. In fact, according to Martin et al. (Citation2019), the genetic correlation values between age at first insemination and clinical mastitis or considering SCS indirect measure are −0.04 and −0.24, respectively. The same authors estimated a more negative correlation value between first service to conception and clinical mastitis (-0.41). Also, between SCS and milk yield the genetic correlation values found in literature result negative (Haile-Mariam et al. Citation2001; Samoré et al. Citation2011)
Conclusions
In this study we used the data of antibiotic treatments for mastitis, recorded by the herd veterinarian on 170 Holstein cows for 7 years. These data were coupled with longitudinal recording of SCC allowing to rank individuals from the most susceptible (largest number of treatments along lactations and SCC peaks (recorded during the milk recording tests) to the most resistant (no treatments in at least 3 lactations and no SCC peaks). The cows used in the GWAS analysis are then assumed to represent the extreme 15% more susceptible and more resistant of the underlying distribution of mastitis resistance.
Some of the genomic regions containing QTL identified in the present study were confirmed in previous studies that considered different phenotypes to map genetic basis of mastitis resistance: among them clinical mastitis, SCC and SCS. This suggests that the method used to classify the individuals with the medical treatment records, could improve the identification of QTL regions related to immune resistance to mastitis.
The new regulations on veterinary treatments make mandatory the recording of antibiotic prescription for each individuals treatment. The integration of this new database with routine milk recording data for SCC may represent then a new possibility to improve the genetic selection for mastitis resistance.
Author contributions
MGS and AB: Conceived and designed the study; CP: participated in data interpretation and revised the manuscript; RM: performed the experiments, MGS and FB: data analysis; TM = collected all data and sampled animals; MGS and AB = wrote and revised the manuscript. All authors reviewed and approved the final version of the manuscript.
Supplemental Material
Download MS Word (17.9 KB)Supplemental Material
Download MS Excel (23.4 KB)Supplemental Material
Download MS Word (22.1 KB)Disclosure statement
No potential conflict of interest was reported by the author(s).
Data availability statement
The data are still under embargo as part of a project still active.
Additional information
Funding
Notes
References
- Amaral AJ, Bressan MC, Almeida J, Bettencourt C, Moreira O, Sá J, Gama-Carvalho M, Bessa R, Gama LT. 2019. Combining genome-wide association analyses and gene interaction networks to reveal new genes associated with carcass traits, meat quality and fatty acid profiles in pigs. Livest Sci. 220:180–189.
- Anel A, Aguiló JI, Catalán E, Garaude J, Rathore MG, Pardo J, Villalba M. 2012. Protein kinase C-θ (PKC-θ) in natural killer cell function and anti-tumor immunity. Front Immunol. 3:187.
- Angueira AR, Shapira SN, Ishibashi J, Sampat S, Sostre-Colón J, Emmett MJ, Titchenell PM, Lazar MA, Lim H-W, Seale P. 2020. Early B cell factor activity controls developmental and adaptive thermogenic gene programming in adipocytes. Cell Rep. 30(9):2869–2878.e4.
- Asselstine V. 2021. Functional regulatory elements influencing mastitis in Holstein dairy cows. https://atrium.lib.uoguelph.ca/xmlui/handle/10214/26638
- Beaudeau F, Seegers H, Fourichon C, Hortet P. 1997. Association between milk somatic cell counts up to 400000 cells/ml and clinical mastitis in dairy cows. In: 4 Rencontres autour des recherches sur les ruminants, Paris (France), 4-5 Dec 1997. Paris (France): Institut de l’Elevage.
- Chen X, Cheng Z, Zhang S, Werling D, Wathes DC. 2015. Combining genome wide association studies and differential gene expression data analyses identifies candidate genes affecting mastitis caused by two different pathogens in the dairy cow. OJAS. 05(04):358–393.
- Darvasi A, Soller M. 1992. Selective genotyping for determination of linkage between a marker locus and a quantitative trait locus. Theor Appl Genet. 85(2-3):353–359.
- Darvasi A, Soller M. 1994. Selective DNA pooling for determination of linkage between a molecular marker and a quantitative trait locus. Genetics. 138(4):1365–1373.
- de Lima AO, Koltes JE, Diniz WJS, de Oliveira PSN, Cesar ASM, Tizioto PC, Afonso J, de Souza MM, Petrini J, Rocha MIP, et al. 2020. Potential biomarkers for feed efficiency-related traits in nelore cattle identified by co-expression network and integrative genomics analyses. Front Genet. 11:189.
- Farhadian M, Rafat SA, Hasanpur K, Ebrahimi M, Ebrahimie E. 2018. Cross-species meta-analysis of transcriptomic data in combination with supervised machine learning models identifies the common gene signature of lactation process. Front Genet. 9:235.
- Friggens NC, Brun-Lafleur L, Faverdin P, Sauvant D, Martin O. 2013. Advances in predicting nutrient partitioning in the dairy cow: recognizing the central role of genotype and its expression through time. animal. 7(s1):89–101.
- Gomes F, Henriques M. 2016. Control of bovine mastitis: old and recent therapeutic approaches. Curr Microbiol. 72(4):377–382.
- Haile-Mariam M, Bowman PJ, Goddard ME. 2001. Genetic and environmental correlations between test-day somatic cell count and milk yield traits. Livest Prod Sci. 73(1):1–13.
- Hanna CB, Mudaliar D, John K, Allen CL, Sun L, Hawkinson JE, Schönbrunn E, Georg GI, Jensen JT. 2020. Development of WEE2 kinase inhibitors as novel non-hormonal female contraceptives that target meiosis †. Biol Reprod. 1032020(2):368–377.
- Hayes BJ, Bowman PJ, Chamberlain AJ, Goddard ME. 2009. Invited review: genomic selection in dairy cattle: progress and challenges. J Dairy Sci. 92(2):433–443.
- Hu Z, Qu G, Yu X, Jiang H, Teng X-L, Ding L, Hu Q, Guo X, Zhou Y, Wang F, et al. 2019. Acylglycerol kinase maintains metabolic state and immune responses of CD8+ T cells. Cell Metab. 30(2):290–302.e5.
- Jaggi R, Marti A, Guo KE, Feng Z, Friis RR. 1996. Regulation of a physiological apoptosis: mouse mammary involution. J Dairy Sci. 79(6):1074–1084.
- Kabera F, Roy J-P, Afifi M, Godden S, Stryhn H, Sanchez J, Dufour S. 2021. Comparing blanket vs. selective dry cow treatment approaches for elimination and prevention of intramammary infections during the dry period: a systematic review and meta-analysis. Front Vet Sci. 8:688450.
- Kurz JP, Yang Z, Weiss RB, Wilson DJ, Rood KA, Liu GE, Wang Z. 2019. A genome-wide association study for mastitis resistance in phenotypically well-characterized Holstein dairy cattle using a selective genotyping approach. Immunogenetics. 71(1):35–47.
- Li T, Gao J, Zhao X, Ma Y. 2019. Digital gene expression analyses of mammary glands from meat ewes naturally infected with clinical mastitis. R Soc Open Sci. 6(7):181604.
- Li Z, Li Y, Wang X, Yang Q. 2021. PPP2R2B downregulation is associated with immune evasion and predicts poor clinical outcomes in triple-negative breast cancer. Cancer Cell Int. 21(1):13.
- Lipkin E, Strillacci MG, Eitam H, Yishay M, Schiavini F, Soller M, Bagnato A, Shabtay A. 2016. The use of Kosher phenotyping for mapping QTL affecting susceptibility to Bovine respiratory disease. PLoS ONE. 11(4):e0153423.
- Lopreiato V, Minuti A, Morittu VM, Britti D, Piccioli-Cappelli F, Loor JJ, Trevisi E. 2020. Inflammation, migration, and cell-cell interaction-related gene network expression in leukocytes is enhanced in Simmental compared with Holstein dairy cows after calving. J Dairy Sci. 103(2):1908–1913.
- Machugh DE, Giuffra E, Alexandre PA, Naval-Sanchez M, Porto-Neto LR, Bento J, Ferraz S, Reverter A, Fukumasu H. 2019. Systems biology reveals NR2F6 and TGFB1 as key regulators of feed efficiency in beef cattle [Internet].
- Martin P, Baes C, Houlahan K, Richardson CM, Jamrozik J, Miglior F. 2019. Genetic correlations among selected traits in Canadian Holsteins. Can J Anim Sci. 99(4):693–704.
- Meier S, Arends D, Korkuć P, Neumann GB, Brockmann GA. 2020. A genome-wide association study for clinical mastitis in the dual-purpose German Black Pied cattle breed. J Dairy Sci. 103(11):10289–10298.
- Merle R, Mollenhauer Y, Hajek P, Robanus M, Hegger-Gravenhorst C, Honscha W, Käsbohrer A, Kreienbrock L. 2013. Monitoring of antibiotic consumption in cattle on agricultural farms. Berl Munch Tierarztl Wochenschr. 126(7–8):318–325.
- Mitchell JM, Griffiths MW, McEwen SA, McNab WB, Yee AJ. 1998. Antimicrobial drug residues in milk and meat: causes, concerns, prevalence, regulations, tests, and test performance. J Food Prot. 61(6):742–756.
- Moretti R, Soglia D, Chessa S, Sartore S, Finocchiaro R, Rasero R, Sacchi P. 2021. Identification of SNPs associated with somatic cell score in candidate genes in Italian Holstein Friesian bulls. Animals. 11(2):366.
- Naderi S, Bohlouli M, Yin T, König S. 2018. Genomic breeding values, SNP effects and gene identification for disease traits in cow training sets. Anim Genet. 49(3):178–192.
- Naserkheil M, Ghafouri F, Zakizadeh S, Pirany N, Manzari Z, Ghorbani S, Banabazi MH, Bakhtiarizadeh MR, Huq MA, Park MN, et al. 2022. Multi-omics integration and network analysis reveal potential hub genes and genetic mechanisms regulating bovine mastitis. Curr Issues Mol Biol. 44(1):309–328.
- Ng TH, Kurtz J. 2020. Dscam in immunity: a question of diversity in insects and crustaceans. Dev Comp Immunol. 105:103539.
- Nicolazzi EL, Caprera A, Nazzicari N, Cozzi P, Strozzi F, Lawley C, Pirani A, Soans C, Brew F, Jorjani H, et al. 2015. SNPchiMp v.3: integrating and standardizing single nucleotide polymorphism data for livestock species. BMC Genomics [Internet]. 16(1):283.,.
- Oliveira L, Ruegg PL. 2014. Treatments of clinical mastitis occurring in cows on 51 large dairy herds in Wisconsin. J Dairy Sci. 97(9):5426–5436.
- Peletto S, Strillacci MG, Capucchio MT, Biasibetti E, Modesto P, Acutis PL, Bagnato A. 2017. Genetic basis of lipomatous myopathy in Piedmontese beef cattle. Livest Sci. 206:9–16.
- Poulsen NA, Robinson RC, Barile D, Larsen LB, Buitenhuis B. 2019. A genome-wide association study reveals specific transferases as candidate loci for bovine milk oligosaccharides synthesis. BMC Genomics. 20(1):404.
- Prince C, Mitchell RE, Richardson TG. 2020. An integrative multi-omics analysis of 16 autoimmune diseases and cancer outcomes highlights immune-cell regulatory mechanisms and shared genetic architecture. medRxiv 2020.11.27.20235663. doi:.
- Sahana G, Guldbrandtsen B, Thomsen B, Holm LE, Panitz F, Brøndum RF, Bendixen C, Lund MS. 2014. Genome-wide association study using high-density single nucleotide polymorphism arrays and whole-genome sequences for clinical mastitis traits in dairy cattle. J Dairy Sci. 97(11):7258–7275.
- Sammad A, Zhang H, Shi R, Dong Y, Luo H, Chen Z, Liu L, Guo G, Liu A, Wang Y. 2022. A post-GWAS functional analysis confirming effects of three BTA13 genes CACNB2, SLC39A12, and ZEB1 on dairy cattle reproduction. Front Genet. 13:882951.
- Samoré AB, Román-Ponce SI, Vacirca F, Frigo E, Canavesi F, Bagnato A, Maltecca C. 2011. Bimodality and the genetics of milk flow traits in the Italian Holstein-Friesian breed. J Dairy Sci. 94(8):4081–4089.
- Sharifi S, Pakdel A, Jahanbakhsh J, Aryan Y, Mahdavi A, Ebrahimie E. 2020. Molecular mechanisms of resistance to bovine mastitis. Livest Sci. 239:104068.
- Shimaoka T, Nishimura T, Kano K, Naito K. 2009. Cell cycle critical effect of pigWee1B on the regulation of meiotic resumption in porcine immature oocytes. Cell Cycle. 8(15):2375–2384.
- Song J, Deng T. 2020. The adipocyte and adaptive immunity. Front Immunol. 11:593058.
- Strillacci MG, Frigo E, Schiavini F, Samoré AB, Canavesi F, Vevey M, Cozzi MC, Soller M, Lipkin E, Bagnato A. 2014. Genome-wide association study for somatic cell score in Valdostana Red Pied cattle breed using pooled DNA. BMC Genet. 15(1):106–110.
- Sun N, Jiang L, Ye M, Wang Y, Wang G, Wan X, Zhao Y, Wen X, Liang L, Ma S, et al. 2020. TRIM35 mediates protection against influenza infection by activating TRAF3 and degrading viral PB2. Protein Cell. 11(12):894–914.
- Thompson-Crispi K, Atalla H, Miglior F, Mallard BA. 2014. Bovine mastitis: frontiers in immunogenetics. Front Immunol. 5:493.
- Turner SD. 2014. qqman: an R package for visualizing GWAS results using QQ and Manhattan plots. Biorxiv. 005165. doi:
- Vigors S, O'Doherty JV, Kelly AK, O'Shea CJ, Sweeney T. 2016. The effect of divergence in feed efficiency on the intestinal microbiota and the intestinal immune response in both unchallenged and lipopolysaccharide challenged ileal and colonic explants. PLoS One. 11(2):e0148145.
- Wang D, Liu L, Augustino S, Duan T, Hall TJ, MacHugh DE, Dou J, Zhang Y, Wang Y, Yu Y. 2020. Identification of novel molecular markers of mastitis caused by Staphylococcus aureus using gene expression profiling in two consecutive generations of Chinese Holstein dairy cattle. J Anim Sci Biotechnol. 11(1):1–17.
- Welderufael BG, Løvendahl P, De Koning D-J, Janss LLG, Fikse WF. 2018. Genome-wide association study for susceptibility to and recoverability from mastitis in Danish Holstein cows. Front Genet. 9:141.
- Westphal A, Cheng W, Yu J, Grassl G, Krautkrämer M, Holst O, Föger N, Lee K-H. 2017. Lysosomal trafficking regulator Lyst links membrane trafficking to toll-like receptor–mediated inflammatory responses. Journal of Experimental Medicine. 214(1):227–244.
- Yang F, Chen F, Li L, Yan L, Badri T, Lv C, Yu D, Zhang M, Jang X, Li J. 2019. Three novel players: PTK2B, SYK, and TNFRSF21 were identified to be involved in the regulation of bovine mastitis susceptibility via GWAS and post-transcriptional analysis. Front Immunol. 10:1579.
- Zerjal T, Härtle S, Gourichon D, Guillory V, Bruneau N, Laloë D, Pinard-van der Laan M-H, Trapp S, Bed’hom B, Quéré P. 2021. Assessment of trade-offs between feed efficiency, growth-related traits, and immune activity in experimental lines of layer chickens. Genet Sel Evol. 53(1):44.
- Zhang F-L, Zhang S-E, Sun Y-J, Wang J-J, Shen W. 2022. Comparative transcriptomics uncover the uniqueness of oocyte development in the donkey. Front Genet. 13:839207.