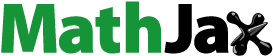
Abstract
Individual methane emissions are a potential breeding goal for selection plans aimed at improving the sustainability of ruminant farming systems. However, a large-scale recording of individual methane emissions is hampered by the high costs of the equipment and the logistics of the experiments. Equations have been developed to predict methane emissions in dairy cattle based on variables such as dry matter intake (DMI), gross energy intake, body weight and milk fatty acids (FAs) profile. No equations are currently available for dairy sheep. The aims of this study were: (i) to estimate the enteric methane yield (eMY, expressed as g/kg of DMI) and enteric methane intensity (eMI, expressed as g/kg of FPCM) of dairy sheep using equations developed for dairy cattle that consider as predictors milk FA; (ii) to evaluate the effect of stage of lactation, parity and month of lambing on eMY and eMI; (iii) to estimate the heritability of eMY and eMI. For this purpose, 964 individual milk samples from Sarda dairy ewes were analysed for milk composition and FA profile. Nine different equations were used to predict eMY and eMI. Values of eMY ranged from 19.4 g/kg to 20.4 g/kg of DMI, whereas values of eMI ranged from 15.1 g/kg to 21.0 g/kg of DMI, respectively. Stage of lactation was an important factor in the variability of eMY (p < .01). Indeed, this trait increased towards the end of lactation. Both eMY and eMI were negatively correlated with milk yield, lactose concentration and urea and positively correlated with fat concentration and NaCl, respectively. Heritability of eMI and eMY estimated using a single trait model was 0.13 ± 0.05 and 0.05 ± 0.04, respectively. The values of heritability estimated for eMY using a bivariate models ranged from 0.10 to 0.16 and they were higher than the value estimated for eMI. Results of the present study, even if based on prediction equations developed for cows, increased the knowledge about the phenotypic and genetic background of methane emissions in Sarda dairy sheep.
Highlights
Methane emissions were predicted from fatty acids using equation developed for cows.
Estimates were in agreement with the values reported in literature.
Methane emissions were lowly heritable.
Introduction
Methane represents one of the most important greenhouse gases (GHGs) contributing to the increase of global warming. Enteric fermentation of ruminants contributes for about one-third on the total anthropogenic emission of this gas (United Nations Environment Programme and Climate and Clean Air Coalition Citation2021). Considering its high radiative forcing and the relative short life in the atmosphere, the reduction of methane emission is considered central to achieve the climate stabilisation goals of the Paris Agreement, and to help limit global warming to 1.5 °C above preindustrial levels (Cain et al. Citation2021). For these reasons, ruminant livestock farming systems are subjected to strong and increasing pressure from public opinion, and the reduction of enteric methane production is one of the main targets of GHG mitigation practices for the ruminant sector (Hristov et al. Citation2013; Beauchemin et al. Citation2022).
In this scenario, several studies on enteric methane emission, the metabolism, the biochemistry, the microbiology as well as the factors that influence its production have been conducted, aimed at developing strategies for reducing its contribution to the global warming (Boadi et al. Citation2004; Beauchemin et al. Citation2008; Cottle et al. Citation2011). Among possible mitigation strategies, the two main approaches are represented by the changes in the diet (e.g. use of concentrates, oils, secondary plant metabolites and additives) and the direct manipulation of the rumen ecosystem (e.g. defaunation, immunisation, using acetogens) (Boadi et al. Citation2004; Kumar Citation2014). The selection for reducing individual methane emission could represent an interesting option, considering recent evidence related to the certain heritability of the methane emission (Pinares-Patiño et al. Citation2013) and the potential inclusion of this trait in the genomic selection programs (de Haas et al. Citation2011). However, the measure of this phenotype represents the main constraint. The quantification of methane emission through standard direct methods (e.g. respiration chambers, green feeders) is expensive and time consuming and it can be applied only on small-scale individual animal measurements (Hammond et al. Citation2016; Patra Citation2016). Indirect quantification through prediction equations based on easy to record proxies could help to estimate this phenotype on population scale. Equations have been developed based on several predictor variables, such as dry matter intake (DMI), intake and digestibility of dietary components, forage to concentrate ratio, gross energy intake, body weight (BW), information about the animal performances (i.e. milk yield, BW), and milk or meat components (Sejian et al. Citation2011; Ramin and Huhtanen Citation2013; Patra and Lalhriatpuii Citation2016). One promising proxy for the prediction of enteric methane production in dairy species is represented by the concentration of milk fatty acids (FAs) (Van Gastelen et al. Citation2018; Engelke et al. Citation2019; Requena et al. Citation2020) because they share biological pathways with enteric methane production (Chilliard et al. Citation2009; Dijkstra, Van Zijderveld, et al. Citation2011). Published prediction equations based on milk FA profile are almost all for dairy cattle and they were developed for predicting methane yield per unit of DMI (enteric methane yield (eMY); g CH4/kg DMI) and methane intensity per unit of fat/protein-corrected milk (enteric methane intensity (eMI); g CH4/kg FPCM). At date, no equation has been published to predict methane production in dairy sheep based on milk FA profile. Although sheep contribution to the global enteric CH4 represents less than 10% (FAOSTAT Citation2018), it assumes a higher relevance in the areas where sheep industry represents an important sector of agriculture (e.g. Mediterranean countries, Australia and New Zealand).
The aims of this study were to: (i) estimate the enteric MY and MI of dairy sheep using prediction equations developed for dairy cattle; (ii) study the effect of stage of lactation, parity and month of lambing on MY and MI; (iii) estimate the heritability of MY and MI; (iv) and study phenotypic and genetic correlations with milk traits.
Materials and methods
Data
Individual milk samples of 964 Sarda dairy ewes farmed in 47 flocks located in the island of Sardinia (Italy) were collected from April to July, during the morning milking, by the Provincial Association of Animal Breeders, that also provided data on milk yield and milk composition. The FA profile of the milk samples was measured using gas chromatography (GC) as previously described by Correddu et al. (Citation2017) and Correddu et al. (Citation2021). In addition to the FA profile (including individual and classes of FA), the mean chain length (MCL) and the mean unsaturation (MU) were calculated according to Kaylegian et al. (Citation2009). Moreover, the calculations proposed by Kaylegian et al. (Citation2009) were partially modified to obtain new unsaturation indices, considering the cis or trans bond configuration of each FA: the mean cis-unsaturation (MCU) was obtained calculating the ratio between the total FA concentration (mmol) weighted for number of cis double bonds and total FA concentration (mmol); the mean trans-unsaturation (MTU) was obtained calculating the ratio between the total FA concentration (mmol) weighted for number of the trans double bonds and total FA concentration (mmol); the cis/trans ratio (CTT) was computed as the ratio of the last two indices.
Table 1. Equation retrieved in literature for estimating methane yield and intensity in dairy cows.
Methane prediction
In the present study, the methane production was estimated as methane yield (eMY; grams of methane emitted per kilogram of DMI, g/kg DMI) and methane intensity (eMI; grams of methane emitted per kilogram of fat and protein corrected milk, g/kg FPCM) using nine equations developed for bovine milk (Table ). The selection of the equations was based on the milk FAs included, their unit of measure (g/100 g of total FA or total lipids) and the result of the estimation (grams of methane emitted per kilogram of DMI or grams of methane emitted per kilogram of fat and protein corrected milk). A total of 18 different FA were included in the equations; among them, only one considered a single FA (CLAc9,t11, Equation (4)), whereas the others considered from three (Equation (8)) to six different FA (Equation (5)). The most represented FA were C18:1t10 and C18:1t11, retrieved in six equations (Equations (1), (2), (3), (5), (6) and (7)).
Table 2. Description of the involved dataset.
Statistical analyses
Individual methane production used for the statistical analysis was obtained by the average of six equations for eMY and three equations for eMI. Data were analysed with the following mixed linear model:
where Y is the considered phenotype (eMY or eMI); DIM is the fixed effect of the days in milk class (five levels: 1 = from 45 to 110; 2 = from 111 to 140; 3 = from 141 to 170; 4 = from 171 to 200; 5 = from 201 to 249 days); PAR is the fixed effect of parity (seven levels: from first to parity number seven); MONTH is the fixed effect of the month of lambing (four levels: 1 = October–November; 2 = December; 3 = January; 4 = February–March); a description of the number of animals within each class is given in Table ; FTD is the random effect of the flock test day contribution (62 levels) distributed as N(0,I
), where I is an identity matrix and
is the variance associated with the effect; animal is the random effect of animal, distributed as N(0,A
), where A is the pedigree-based relationship matrix with 4963 animals, tracking back the pedigree for three generations from the animals with phenotypes (Pocrnic et al. Citation2017; Cesarani et al. Citation2021) and
is the additive genetic variance; e is the random effect of the residuals, distributed as N(0,I
), where I is an identity matrix and
is the random residual term. The statistical analysis was carried out using the PROC MIXED of SAS software version 9.2 (SAS Institute Inc., Cary, NC) and significance was declared for p < .05.
Heritability (h2) and the contribution of FTD to the total variance (V:FTD) were computed as:
with the variances described above.
Moreover, genetic correlations between eMY or eMI and milk production traits (i.e. milk daily production, fat, protein, lactose, pH, casein, urea, NaCl and SCS) were estimated running a series of bivariate models. Variance components, h2, V:FTD and genetic correlations were estimated via the gibbsf90+ (Misztal et al. Citation2014) sampler, with 100,000 iterations, a burn-in period of 10,000 samples and storing every 10 samples. Posterior means for all parameters were calculated using postgibbsf90 (Misztal et al. Citation2014).
Results and discussion
The estimates of the methane emissions are reported in Table . Values of eMY were very similar among equations, with the means ranging from 19.4 g/kg of DMI for the Equation (2) to 20.4 g/kg of DMI for Equations (1) and (6), respectively. Mean values obtained for eMI ranged from 15.1 g/kg of FPCM for the Equation (7) to 21.0 g/kg of DMI for Equation (8).
Table 3. Descriptive statistic of methane yield (CH4, g/kg DMI) and methane intensity (CH4, g/kg FPCM) estimation using published equations.
For each animal, a total of six (Equations (1)–(6) for eMY) and three (Equations (7)–(9) for eMI) estimates were available. These values were then averaged to obtain a mean value for eMY and a mean value for eMI for each animal (Table ). The two average values of methane emissions were very similar, also in terms of DS, min and max. The eMY was slightly higher than eMI (19.9 and 18.7, respectively), as already reported by previous works in dairy cattle (Van Lingen et al. Citation2014; Van Gastelen et al. Citation2017). The differences between the two variables can be explained according to the considered traits used to predict the values of eMY (DMI) and eMI (FPCM). Since DMI is usually lower than FPCM, the values estimated for eMY are usually larger than those estimated for eMI.
In this work, the methane emissions of dairy sheep were estimated using published predictive equations developed for dairy cattle, because of the lack of specific equations for sheep. Thus, a comparison can be done only with direct measurements (Pinares-Patiño et al. Citation2011) or estimates obtained using equations based on other predictor variables (such as DMI, feeding level, BW, and the chemical composition and digestibility of the diet) (Ramin and Huhtanen Citation2013; Congio et al. Citation2022). The mean eMY estimated in the present study is larger than previous reports in crossbred sheep using direct measures (15.7 g/kg of DMI; Pinares-Patiño et al. Citation2013) and lower than the estimate (23.5 g/kg of DMI) reported by Muetzel and Clark (Citation2015) in New Zealand sheep. The result of the present work is very close to the values reported in the meta-analysis of Congio et al. (Citation2022) (19.7 g/kg DMI), and identical (19.9 g/kg DMI) to that of Belanche et al. (Citation2023) carried out on a very large dataset of 2135 observations obtained in several experiments conducted between 2003 and 2018 in research institutions across 13 countries. Moreover, it is very close to the constant methane yield factor (20.9 g CH4/kg DMI) used in New Zealand to compute methane emissions from sheep based on DMI (Muetzel and Clark Citation2015). Similar results were also reported by Knight et al. (Citation2008) with values ranging from 21.9 to 23.8 g CH4/kg DMI for lamb and mature ewes, respectively. In this last work, methane was measured by the sulphur hexafluoride tracer technique. Moreover, the value observed in the present work corresponds to about 9–10 kg of methane emitted per ewe per year, which is in line with the IPCC (Citation2019) and Vermorel et al. (Citation2008) estimates.
The average eMI (18.7, Table ) is not easily comparable with other works in sheep, also considering that dairy sheep are less investigated. Compared to dairy cows, the value observed in this work was slightly higher. Richardson et al. (Citation2021) investigated the genetic parameters for methane emission traits in Australian dairy cows and they reported an average phenotypic value of eMI of 18.15 ± 3.82 g/kg FPCM. According to de Haas et al. (Citation2021), the average eMI of the Dutch dairy cattle population is 15.9 g/d per kg of milk, that is computed based on a daily CH4 production of 392 g and a milk production of 9000 kg in 305 days.
The milk FA included in the prediction equations were determined by GC, which is a time and money consuming technique. A cheap, valid and fast alternative is represented by the use of milk spectra, generated by spectrophotometers, to predict FA (Soyeurt et al. Citation2011; Caredda et al. Citation2016). Correddu et al. (Citation2019) applied the partial least square regression (PLSR) to milk spectra of Sarda dairy ewes to predict 15 single and nine groups of FAs starting from the values measured in the laboratory using the GC. Among the various FA, the authors estimated the content of CLAc9,t11 from the milk spectra (R2 of 0.69 ± 0.03 and RMSEP of 0.26 ± 0.01), which is used to predict eMY in Equation (4) in Table reported by van Gastelen et al. (Citation2017). Thus, we compared the values of eMY predicted using the CLAc9,t11 measured using GC and the one estimated from the milk spectra (Correddu et al. Citation2019). The average values of eMY were 20.0 ± 3.3 (range 5.1–25.2) and 20.0 ± 2.89 (range 10.25–27.19) using the measured and estimated values of CLAc9,t11 respectively. The use of FA predicted from the spectra coupled with the equations reported in literature, even if based on cattle data, would allow the estimation of methane emissions in sheep at the population level.
The methane emission was affected only by the stage of lactation: the effect of DIM was highly significant (p < 001) for eMI and close to the significance threshold for the eMY (p = .06). Both methane phenotypes increased along the lactation (Figure ), with higher differences found in eMI between the first and the last DIM (17.9 and 19.5 g CH4/kg FPCM). The DMI is positively associated to the methane emission, and it represents one of the main drivers of its production (Mills et al. Citation2003; Belanche et al. Citation2023). For this reason, considering the DMI pattern of variation throughout a lactation, an effect of stage of lactation on methane emission is expected. Considering that in the present study, no information were available on DMI and daily methane emission, the reason for the increase of the eMY along lactation, even if not significant, can be only hypothesised. It could be related to a decrease of DMI or increase of daily methane emission along lactation or, more likely, it could be explained by changes in the proportion of forage in the diet that would be increased at late lactation. This last hypothesis agrees with previous studies in dairy cows (Garnsworthy et al. Citation2012; Bittante et al. Citation2018). In particular, Garnsworthy et al. (Citation2012) analysed methane emissions from 215 Holstein-Friesians cows in the UK and they reported an increase of daily methane emissions until the 10th week of lactation, and a constant trend thereafter. A similar result was reported for the daily methane emissions by Bell et al. (Citation2014): these authors found increasing CH4 concentration over the first 20 weeks of lactation, but they were relatively constant after that. The significant increase of eMI along lactation was expected considering that the milk yield decreased, following the typical pattern of lactation curve, and consequently the ratio between methane and milk productions, consequently, increased.
Figure 1. Effect of lactation stage (days in milk, DIM) on methane yield (a) and methane intensity (b). DMI: dry matter intake; FPCM: fat and protein corrected milk.

On the other hand, the evolution of methane emission follows the pattern of variation of milk FA included in the prediction equations. In particular, the increase during lactation mirrors the decrease of those FA that derive, directly or after rumen biohydrogenation, from the fresh forage consumption (C18:1t10 and C18:1t11 CLAc9,t11 C18:3n3) and that have negative coefficients in the equations.
Indeed, in the typical extensive Mediterranean farm system of sheep, the availability of fresh forages and their quality decrease in late lactation, which occurs in early summer (Nudda et al. Citation2014). For the same reason, on the contrary, most of the FA with positive coefficients in the prediction equations have a typical increase along lactation; this is the case of some odd and branched chain FA as isoC15:0 isoC16:0 (Nudda et al. Citation2021). This effect could be related to the increase of fibre concentration in the forage, that can stimulate the rumen fermentation. The increase of FAs of microbial origin and the methane production are two consequences of the fibre fermentation.
Parity is one of the other factors that has been reported to influence the enteric methane production. Compared to pluriparous animals, first-parity animals in general produce less methane due to the lower DMI and BW (Bittante et al. Citation2018). Indeed, smaller animals consume less feed and then emit less methane (Hristov et al. Citation2013). However, the lack of the effect of parity on both eMY and eMI observed in the present can be explained by the lower DMI and milk yield of primiparous sheep, that counterbalance the reduction of methane emission in the two considered traits. The lack of parity effect on methane emission was also reported in dairy cattle (Bittante et al. Citation2018).
Phenotypic correlations between methane emissions and milk traits
Phenotypic correlations between the two methane phenotypes and milk traits are reported in Figure . Methane yield was negatively correlated with milk yield (–0.13), lactose concentration (–0.27), pH (–0.06) and urea (–0.22), whereas it was positively correlated with fat (0.38), protein (0.08) and casein (0.08), and NaCl (0.16). Methane intensity was negatively correlated with milk yield (–0.25), lactose (–0.22) and urea (–0.06), whereas it was positively correlated with fat (0.25) and NaCl (0.18).
Figure 2. Phenotypic correlations estimated between methane emission (yield and intensity) and other milk traits. Bold values indicate significant correlation (p < .05).

Although weak, the negative correlation between eMY and milk yield could be explained by the individual feed efficiency of animals. This result suggests that animals with low methane yield have high milk yield. This is consistent with the negative relationship of eMY with DMI, probably because the increase in passage rate and the decrease in retention time in the rumen lead to a decrease of rumen fermentation, which decreases the production of methane per unit of feed (Niu et al. Citation2018). The correlation between eMI and milk yield (–0.25) was stronger than that found between eMY and milk yield (–0.13), probably because the equations for eMI estimation accounts for the FPCM. Our results are in agreement with Manzanilla-Pech et al. (Citation2021) who found a strong negative correlation (–0.59) between milk yield and eMI. The negative correlation between the two traits supposes a dilution effect of methane emissions: the larger the milk yield, the lower the eMI.
The negative correlation between methane (both yield and intensity) and lactose concentration reflected the relationship between the methane emissions and the milk yield. In fact, lactose is strongly correlated to milk volume (Fuertes et al. Citation1998; Haile-Mariam and Pryce Citation2017) due to its high osmolarity of this disaccharide.
The positive correlations found between fat concentration and both eMY and eMI were in line with previous studies (Bougouin et al. Citation2019; Saborío-Montero et al. Citation2022). This result could be explained by the strong relationship between the synthesis of milk fat and the acetate, which is the principal carbon source for lipid synthesis. Moreover, the acetate production in the rumen is related to the production of hydrogen that represents the substrate for the methanogen bacteria (Moraes et al. Citation2014) and, thus, for the methane production.
A negative correlation was found between urea and methane emission, both in terms of yield and intensity, with the first exhibiting the largest value (–0.22). The biological relationship between milk urea and enteric methane can be found in the dietary protein. A strong relationship exists between the level of dietary protein and the milk concentration of urea (Cannas et al. Citation1998); moreover, the excess of protein in the diet (that leads to an increase of urea in the milk) could reduce the rumen pH and increase the propionic acid and, consequently, could decrease the methane production (Dijkstra, Oenema, et al. Citation2011).
The methane emissions displayed interesting phenotypic correlations with the unsaturation and chain length indices (Figure ). Methane yield had low correlation with MCL (0.12) and relatively high negative correlation with MU (–0.50); on the contrary, eMI had high positive correlation with MCL (0.52) and low correlation with the MU index. The highest value of correlation was found between the eMY and the MTU index (–0.93).
Heritability and genetic correlation of methane emissions
The h2 estimated for eMY and eMI using a single trait model were rather low: 0.13 ± 0.05 and 0.05 ± 0.04, respectively. The FTD component explained a large part of the total variance: 0.64 ± 0.05 for eMY and 0.65 ± 0.05 for eMI. The heritability estimated for eMY in the present study is in agreement with the value (0.13 ± 0.03) reported for crossbred sheep between 5 and 10 months of age (Pinares-Patiño et al. Citation2013), whereas it is much lower than that reported by Pinares-Patiño et al. (Citation2011), who estimated heritability of 0.30 ± 0.26 for eMY in 10 months old ewes. Lassen and Løvendahl (Citation2013) estimated heritability of methane emissions in dairy cattle, and they observed a h2 of 0.21 ± 0.06 for eMI (CH4, g/L milk produced). Bittante and Cecchinato (Citation2020) reported values of h2 ranging from 0.06 to 0.09 and V:FTD contributions (0.71 for eMY and 0.57 for eMI) in agreement with the values here estimated. A meta-analysis employing pooled data from sheep and cattle revealed heritability values for eMI and eMY of 0.23 ± 0.03 and 0.19 ± 0.02, respectively (Brito et al. Citation2018).
In the present work, bivariate models were run to estimate heritability and genetic correlation between eMY, eMI and other milk traits (Table ). The values of h2 estimated for eMY using the two-traits models were low and they ranged from 0.10 (when analysed with MU and MCU) to 0.16 (with MTU). The value of V:FTD was very high in all bivariate models involving eMY: these values were nearly constant, and they ranged from 0.59 ± 0.05 to 0.64 ± 0.05. The highest value of FTD for eMY was estimated when the trait was analysed with MTU. The genetic correlations between eMY and the other traits ranged from −0.97 ± 0.03 (MTU) to 0.77 ± 0.14 (with CTT) and they showed large standard errors, sometimes even larger than the estimates (Table ). The latter could be due to the particular structure of some of the involved traits and the small sample size. The negative genetic correlation between MDP and eMY (–0.40 ± 0.32) is intriguing: the increase of MDP would lead to a reduction of eMY, which is desirable.
Table 4. Heritability, variance explained by the flock test date and genetic correlations estimated for methane yield and intensity using the bivariate models.
As reported in Table , the h2 estimated for eMI in the bivariate models were lower than those obtained for eMY. Values of h2 were close to zero (as showed by the standard errors larger than the estimates for some models) and ranged from 0.02 ± 0.03 (when analysed with SCS) to 0.08 ± 0.04 (MCU). The genetic correlations between eMI and the other milk traits showed a wide range of values, ranging from −0.69 ± 0.39 (with SCS) to 0.82 ± 0.20 (with MCU). The two investigated measures of methane emissions, eMY and eMI, showed a moderate and positive genetic correlation (0.46 ± 0.38), suggesting that the inclusion of a trait in the breeding scheme would act in the same direction also on the other trait. The genetic correlations reported in literature between methane emissions and milk traits are discordant. For example, Kandel et al. (Citation2017) analysed the relationship between log-transformed predicted eMI and milk, fat, and protein yields in the first two lactations of Holstein dairy cattle. Those authors reported average negative genetic correlations between the lactation yields and the methane: −0.78, −0.23 and −0.64 for milk, fat and protein yields, respectively. These values are very different from our estimates between eMI and the same milk production traits (–0.04 ± 0.59, 0.04 ± 0.53 and −0.17 ± 0.55), that however could not be considered different from zero because of the very large standard errors. Genetic correlations of −0.46 ± 0.32 and 0.11 ± 0.40 were estimated in Holstein cows between eMI (grams of methane production and energy corrected milk) and protein and fat yields, respectively (Lassen et al. Citation2016). Positive and larger estimates of genetic correlations between eMY and milk yields were reported in Polish Holstein-Friesian cows by Ghiasi et al. (Citation2022). In that study, genetic correlations were 0.82, 0.82, 0.34 and 0.98 between eMY and milk, protein, fat and lactose yields, respectively. Moreover, those authors reported very low standard errors (less than 0.03) for all the genetic correlations. Bittante and Cecchinato (Citation2020) analysed the relationship between methane emissions and milk production traits in Brown Swiss cows and they reported negative correlations between eMY and fat (–0.64) and between eMY and protein percentage (–0.53). Those authors estimated positive correlations between eMI and fat (0.15) and close to zero between eMI and protein (0.03). According to Ghiasi et al. (Citation2022), high genetic correlation between lactation milk yield and the predicted methane production is expected because high milk productions need high DMIs, resulting in high methane production.
Conclusions
Results of the present study suggest the possibility of predicting individual methane emissions in dairy sheep on the basis of milk FA profile. Even if the considered equations have been developed using cattle data, predictions for both methane intensity and yield were very close to values reported in literature for sheep, using direct and indirect measures. The possibility of using as predictors FAs estimated by the milk MIR spectra would allow to have individual methane emission phenotypes available also at population scale. Moreover, the phenotypic and genetic background of methane emissions in Sarda breed dairy ewes were investigated. The not negligible value of heritability and the positive genetic correlations of methane yield with milk FAs of nutritional interest suggest considering the inclusion of this phenotype as breeding goal in the genetic program of the breed. Considering the importance of the Sarda, which represents about 65% of the total Italian dairy sheep stock and contributes to more than 80% of the sheep milk produced in Italy, the possibility of selection for a reduced methane emission would represent an important strategy of mitigation of the environmental impact of the Italian dairy sheep industry.
Ethical approval
Animal Care and Ethical approvals were not needed as data were obtained from pre-existing databases.
Acknowledgements
The authors acknowledge the Provincial Breeders Associations (AIPA) of Cagliari, Nuoro, Sassari and Oristano (Italy); the laboratory of Sardinian Breeders Association (ARA, Oristano, Italy) for providing milk composition data; and the Italian Associations of Animal Breeders (AIA). This study was financed by the European Union – NextGenerationEU (PNRR M4C2, CN00000022 AGRITECH), by the Regional Government of Sardinia (Grant no. CRP 61608 “Il latte Ovino della Sardegna”) and by the Italian Ministry of Agriculture (Grant PRECISLAT).
Disclosure statement
No potential conflicts of interest were reported by the author(s).
Data availability statement
The data supporting this study may be available from the corresponding author upon reasonable request.
Additional information
Funding
References
- Beauchemin KA, Kreuzer M, O'Mara F, McAllister TA. 2008. Nutritional management for enteric methane abatement: a review. Aust J Exp Agric. 48(2):21–27. doi: 10.1071/EA07199.
- Beauchemin KA, Ungerfeld EM, Abdalla AL, Alvarez C, Arndt C, Becquet P, Benchaar C, Berndt A, Mauricio RM, McAllister TA, et al. 2022. Invited review: current enteric methane mitigation options. J Dairy Sci. 105(12):9297–9326. doi: 10.3168/jds.2022-22091.
- Belanche A, Hristov AN, van Lingen HJ, Denman SE, Kebreab E, Schwarm A, Kreuzer M, Niu M, Eugène M, Niderkorn V, et al. 2023. Prediction of enteric methane emissions by sheep using an intercontinental database. J Clean Prod. 384:135523. doi: 10.1016/j.jclepro.2022.135523.
- Bell MJ, Potterton SL, Craigon J, Saunders N, Wilcox RH, Hunter M, Goodman JR, Garnsworthy PC. 2014. Variation in enteric methane emissions among cows on commercial dairy farms. Animal. 8(9):1540–1546. doi: 10.1017/S1751731114001530.
- Bittante G, Cecchinato A. 2020. Heritability estimates of enteric methane emissions predicted from fatty acid profiles, and their relationships with milk composition, cheese-yield and body size and condition. Ital J Anim Sci. 19(1):114–126. doi: 10.1080/1828051X.2019.1698979.
- Bittante G, Cecchinato A, Schiavon S. 2018. Dairy system, parity, and lactation stage affect enteric methane production, yield, and intensity per kilogram of milk and cheese predicted from gas chromatography fatty acids. J Dairy Sci. 101(2):1752–1766. doi: 10.3168/jds.2017-13472.
- Boadi D, Benchaar C, Chiquette J, Massé D. 2004. Mitigation strategies to reduce enteric methane emissions from dairy cows: update review. Can J Anim Sci. 84(3):319–335. doi: 10.4141/A03-109.
- Bougouin A, Appuhamy JRN, Ferlay A, Kebreab E, Martin C, Moate PJ, Benchaar C, Lund P, Eugène M. 2019. Individual milk fatty acids are potential predictors of enteric methane emissions from dairy cows fed a wide range of diets: approach by meta-analysis. J Dairy Sci. 102(11):10616–10631. doi: 10.3168/jds.2018-15940.
- Brito LF, Schenkel FS, Oliveira HR, Cánovas A, Miglior F. 2018. Meta-analysis of heritability estimates for methane emission indicator traits in cattle and sheep. Proceedings of the World Congress on Genetics Applied to Livestock Production, Volume Challenges – Environmental; Feb; Auckland, New Zealand.
- Cain M, Jenkins S, Allen MR, Lynch J, Frame DJ, Macey AH, Peters GP. 2021. Methane and the Paris Agreement temperature goals. Philos Trans R Soc A. 380(2215):20200456.
- Cannas A, Pes A, Mancuso R, Vodret B, Nudda A. 1998. Effect of dietary energy and protein concentration on the concentration of milk urea nitrogen in dairy ewes. J Dairy Sci. 81(2):499–508. doi: 10.3168/jds.S0022-0302(98)75602-4.
- Caredda M, Addis M, Ibba I, Leardi R, Scintu MF, Piredda G, Sanna G. 2016. Prediction of fatty acid content in sheep milk by mid-infrared spectrometry with a selection of wavelengths by genetic algorithms. LWT Food Sci Technol. 65:503–510. doi: 10.1016/j.lwt.2015.08.048.
- Cesarani A, Masuda Y, Tsuruta S, Nicolazzi EL, VanRaden PM, Lourenco D, Misztal I. 2021. Genomic predictions for yield traits in US Holsteins with unknown parent groups. J Dairy Sci. 104(5):5843–5853. doi: 10.3168/jds.2020-19789.
- Chilliard Y, Martin C, Rouel J, Doreau M. 2009. Milk fatty acids in dairy cows fed whole crude linseed, extruded linseed, or linseed oil, and their relationship with methane output. J Dairy Sci. 92(10):5199–5211. doi: 10.3168/jds.2009-2375.
- Congio GF, Bannink A, Mayorga OL, Rodrigues JP, Bougouin A, Kebreab E, Carvalho PCF, Abdalla AL, Monteiro ALG, Ku-Vera JC, et al. 2022. Prediction of enteric methane production and yield in sheep using a Latin America and Caribbean database. Livest Sci. 264:105036. doi: 10.1016/j.livsci.2022.105036.
- Correddu F, Cellesi M, Serdino J, Manca MG, Contu M, Dimauro C, Ibba I, Macciotta NPP. 2019. Genetic parameters of milk fatty acid profile in sheep: comparison between gas chromatographic measurements and Fourier-transform IR spectroscopy predictions. Animal. 13(3):469–476. doi: 10.1017/S1751731118001659.
- Correddu F, Murgia MA, Mangia NP, Lunesu MF, Cesarani A, Deiana P, Giuseppe P, Nudda A. 2021. Effect of altitude of flock location, season of milk production and ripening time on the fatty acid profile of Pecorino Sardo cheese. Int Dairy J. 113:104895. doi: 10.1016/j.idairyj.2020.104895.
- Correddu F, Serdino J, Manca MG, Cosenza G, Pauciullo A, Ramunno L, Macciotta NPP. 2017. Use of multivariate factor analysis to characterize the fatty acid profile of buffalo milk. J Food Compost Anal. 60:25–31. doi: 10.1016/j.jfca.2017.03.008.
- Cottle DJ, Nolan JV, Wiedemann SG. 2011. Ruminant enteric methane mitigation: a review. Anim Prod Sci. 51(6):491–514. doi: 10.1071/AN10163.
- de Haas Y, Veerkamp RF, de Jong G, Aldridge MN. 2021. Selective breeding as a mitigation tool for methane emissions from dairy cattle. Animal. 15(Suppl. 1):100294. doi: 10.1016/j.animal.2021.100294.
- de Haas Y, Windig JJ, Calus MPL, Dijkstra J, De Haan M, Bannink A, Veerkamp RF. 2011. Genetic parameters for predicted methane production and potential for reducing enteric emissions through genomic selection. J Dairy Sci. 94(12):6122–6134. doi: 10.3168/jds.2011-4439.
- Dijkstra J, Oenema O, Bannink A. 2011. Dietary strategies to reducing N excretion from cattle: implications for methane emissions. Curr Res Environ Sustain. 3(5):414–422. doi: 10.1016/j.cosust.2011.07.008.
- Dijkstra J, Van Zijderveld SM, Apajalahti JA, Bannink A, Gerrits WJJ, Newbold JR, Perdok HB, Berends H. 2011. Relationships between methane production and milk fatty acid profiles in dairy cattle. Anim Feed Sci Technol. 166–167:590–595. doi: 10.1016/j.anifeedsci.2011.04.042.
- Engelke SW, Daş G, Derno M, Tuchscherer A, Wimmers K, Rychlik M, Kienberger H, Berg W, Kuhla B, Metges CC. 2019. Methane prediction based on individual or groups of milk fatty acids for dairy cows fed rations with or without linseed. J Dairy Sci. 102(2):1788–1802. doi: 10.3168/jds.2018-14911.
- FAOSTAT. 2018. FAO statistical data base. Rome, Italy: FAOSTAT.
- Fuertes JA, Gonzalo C, Carriedo JA, San Primitivo F. 1998. Parameters of test day milk yield and milk components for dairy ewes. J Dairy Sci. 81(5):1300–1307. doi: 10.3168/jds.S0022-0302(98)75692-9.
- Garnsworthy PC, Craigon J, Hernandez-Medrano JH, Saunders N. 2012. Variation among individual dairy cows in methane measurements made on farm during milking. J Dairy Sci. 95(6):3181–3189. doi: 10.3168/jds.2011-4606.
- Ghiasi H, Sitkowska B, Piwczyński D, Kolenda M. 2022. Genetic parameters for methane emissions using indirect prediction of methane and its association with milk and milk composition traits. Animals. 12(16):2073. doi: 10.3390/ani12162073.
- Haile-Mariam M, Pryce JE. 2017. Genetic parameters for lactose and its correlation with other milk production traits and fitness traits in pasture-based production systems. J Dairy Sci. 100(5):3754–3766. doi: 10.3168/jds.2016-11952.
- Hammond KJ, Crompton LA, Bannink A, Dijkstra J, Yáñez-Ruiz DR, O’Kiely P, Kebreab E, Eugène MA, Yu Z, Shingfield KJ, et al. 2016. Review of current in vivo measurement techniques for quantifying enteric methane emission from ruminants. Anim Feed Sci Technol. 219:13–30. doi: 10.1016/j.anifeedsci.2016.05.018.
- Hristov AN, Oh J, Firkins JL, Dijkstra J, Kebreab E, Waghorn G, Makkar HPS, Adesogan AT, Yang W, Lee C, et al. 2013. Special topics—mitigation of methane and nitrous oxide emissions from animal operations: I. A review of enteric methane mitigation options. J Anim Sci. 91(11):5045–5069. doi: 10.2527/jas.2013-6583.
- IPCC. 2019. In: Calvo Buendia E, Tanabe K, Kranjc A, Baasansuren J, Fukuda M, Ngarize S, Osako A, Pyrozhenko Y, Shermanau P, Federici S, editors. Refinement to the 2006 IPCC Guidelines for National Greenhouse Gas Inventories, Prepared by the National Greenhouse Gas Inventories Programme, Volume 4: Agriculture, Forestry and Other Land Use (AFOLU), Chapter 10: Emission from Livestock and Manure Management. Switzerland: IPCC.
- Kandel PB, Vanrobays ML, Vanlierde A, Dehareng F, Froidmont E, Gengler N, Soyeurt H. 2017. Genetic parameters of mid-infrared methane predictions and their relationships with milk production traits in Holstein cattle. J Dairy Sci. 100(7):5578–5591. doi: 10.3168/jds.2016-11954.
- Kaylegian KE, Dwyer DA, Lynch JM, Bauman DE, Fleming JR, Barbano DM. 2009. Impact of fatty acid composition on the accuracy of mid-infrared fat analysis of farm milks. J Dairy Sci. 92(6):2502–2513. doi: 10.3168/jds.2008-1911.
- Knight TW, Molano G, Clark H, Cavanagh A. 2008. Methane emissions from weaned lambs measured at 13, 17, 25 and 35 weeks of age compared with mature ewes consuming a fresh forage diet. Aust J Exp Agric. 48(2):240–243. doi: 10.1071/EA07258.
- Kumar S, Choudhury PK, Carro MD, Griffith GW, Dagar SS, Puniya M, Calabro S, Ravella SR, Dhewa T, Upadhyay RC, et al. 2014. New aspects and strategies for methane mitigation from ruminants. Appl Microbiol Biotechnol. 98(1):31–44. doi: 10.1007/s00253-013-5365-0.
- Lassen J, Løvendahl P. 2013. Heritability for enteric methane emission from Danish Holstein cows using a non-invasive FTIR method. Adv Anim Biosci. 4:280.
- Lassen J, Poulsen NA, Larsen MK, Buitenhuis AJ. 2016. Genetic and genomic relationship between methane production measured in breath and fatty acid content in milk samples from Danish Holsteins. Anim Prod Sci. 56(3):298–303. doi: 10.1071/AN15489.
- Manzanilla-Pech CIV, Gordo DM, Difford GF, Pryce JE, Schenkel F, Wegmann S, Miglior F, Chud TC, Moate PJ, Williams SRO, et al. 2021. Breeding for reduced methane emission and feed-efficient Holstein cows: an international response. J Dairy Sci. 104(8):8983–9001. doi: 10.3168/jds.2020-19889.
- Mills JAN, Kebreab E, Yates CM, Crompton LA, Cammell SB, Dhanoa MS, Agnew RE, France J. 2003. Alternative approaches to predicting methane emissions from dairy cows. J Anim Sci. 81(12):3141–3150. doi: 10.2527/2003.81123141x.
- Misztal I, Tsuruta S, Lourenco DAL, Aguilar I, Legarra A, Vitezica Z. 2014. Manual for BLUPF90 family of programs. University of Georgia, Athens, USA.
- Moraes LE, Strathe AB, Fadel JG, Casper DP, Kebreab E. 2014. Prediction of enteric methane emissions from cattle. Glob Chang Biol. 20(7):2140–2148. doi: 10.1111/gcb.12471.
- Muetzel S, Clark H. 2015. Methane emissions from sheep fed fresh pasture. N Z J Agric Res. 58(4):472–489. doi: 10.1080/00288233.2015.1090460.
- Niu M, Kebreab E, Hristov AN, Oh J, Arndt C, Bannink A, Bayat AR, Brito AF, Boland T, Casper D, et al. 2018. Prediction of enteric methane production, yield, and intensity in dairy cattle using an intercontinental database. Glob Chang Biol. 24(8):3368–3389. doi: 10.1111/gcb.14094.
- Nudda A, Battacone G, Boaventura Neto O, Cannas A, Francesconi AHD, Atzori AS, Pulina G. 2014. Feeding strategies to design the fatty acid profile of sheep milk and cheese. Rev Bras Zootec. 43(8):445–456. doi: 10.1590/S1516-35982014000800008.
- Nudda A, Correddu F, Cesarani A, Pulina G, Battacone G. 2021. Functional odd-and branched-chain fatty acid in sheep and goat milk and cheeses. Dairy. 2(1):79–89. doi: 10.3390/dairy2010008.
- Patra AK. 2016. Recent advances in measurement and dietary mitigation of enteric methane emissions in ruminants. Front Vet Sci. 3:39. doi: 10.3389/fvets.2016.00039.
- Patra AK, Lalhriatpuii M. 2016. Development of statistical models for prediction of enteric methane emission from goats using nutrient composition and intake variables. Agric Ecosyst Environ. 215:89–99. doi: 10.1016/j.agee.2015.09.018.
- Pinares-Patiño CS, Hickey SM, Young EA, Dodds KG, MacLean S, Molano G, Sandoval E, Kjestrup H, Harland R, Hunt C, et al. 2013. Heritability estimates of methane emissions from sheep. Animal. 7(Suppl. 2):316–321. doi: 10.1017/S1751731113000864.
- Pinares-Patiño CS, McEwan JC, Dodds KG, Cárdenas EA, Hegarty RS, Koolaard JP, Clark H. 2011. Repeatability of methane emissions from sheep. Anim Feed Sci Technol. 166–167:210–218. doi: 10.1016/j.anifeedsci.2011.04.068.
- Pocrnic I, Lourenco DAL, Bradford HL, Chen CY, Misztal I. 2017. Impact of pedigree depth on convergence of single-step genomic BLUP in a purebred swine population. J Anim Sci. 95(8):3391–3395.
- Ramin M, Huhtanen P. 2013. Development of equations for predicting methane emissions from ruminants. J Dairy Sci. 96(4):2476–2493. doi: 10.3168/jds.2012-6095.
- Requena F, Peña F, Agüera E, Marín AM. 2020. A meta-analytic approach to predict methane emissions from dairy goats using milk fatty acid profile. Sustainability. 12(12):4834. doi: 10.3390/su12124834.
- Richardson CM, Nguyen TTT, Abdelsayed M, Moate PJ, Williams SRO, Chud TCS, Schenkel FS, Goddard ME, van den Berg I, Cocks BG, et al. 2021. Genetic parameters for methane emission traits in Australian dairy cows. J Dairy Sci. 104(1):539–549. doi: 10.3168/jds.2020-18565.
- Saborío-Montero A, Gutiérrez-Rivas M, Goiri I, Atxaerandio R, García-Rodriguez A, López-Paredes J, Jiménez-Montero JA, González-Recio O. 2022. Rumen eukaryotes are the main phenotypic risk factors for larger methane emissions in dairy cattle. Livest Sci. 263:105023. doi: 10.1016/j.livsci.2022.105023.
- Sejian V, Lal R, Lakritz J, Ezeji T. 2011. Measurement and prediction of enteric methane emission. Int J Biometeorol. 55(1):1–16. doi: 10.1007/s00484-010-0356-7.
- Soyeurt H, Dehareng F, Gengler N, McParland S, Wall E, Berry DP, Coffey M, Dardenne P. 2011. Mid-infrared prediction of bovine milk fatty acids across multiple breeds, production systems, and countries. J Dairy Sci. 94(4):1657–1667. doi: 10.3168/jds.2010-3408.
- United Nations Environment Programme and Climate and Clean Air Coalition. 2021. Global methane assessment: benefits and costs of mitigating methane emissions. Nairobi: United Nations Environment Programme; [accessed 2022 Mar 14]. https://wedocs.unep.org/bitstream/handle/20.500.11822/35917/GMA:ES.pdf.
- Van Engelen S, Bovenhuis H, Dijkstra J, Van Arendonk JAM, Visker MHPW. 2015. Genetic study of methane production predicted from milk fat composition in dairy cows. J Dairy Sci. 98(11):8223–8226. doi: 10.3168/jds.2014-8989.
- Van Gastelen S, Antunes-Fernandes EC, Hettinga KA, Dijkstra J. 2017. Relationships between methane emission of Holstein Friesian dairy cows and fatty acids, volatile metabolites and non-volatile metabolites in milk. Animal. 11(9):1539–1548. doi: 10.1017/S1751731117000295.
- Van Gastelen S, Antunes-Fernandes EC, Hettinga KA, Dijkstra J. 2018. The relationship between milk metabolome and methane emission of Holstein Friesian dairy cows: metabolic interpretation and prediction potential. J Dairy Sci. 101(3):2110–2126. doi: 10.3168/jds.2017-13334.
- Van Gastelen S, Mollenhorst H, Antunes-Fernandes EC, Hettinga KA, van Burgsteden GG, Dijkstra J, Rademaker JLW. 2018. Predicting enteric methane emission of dairy cows with milk Fourier-transform infrared spectra and gas chromatography-based milk fatty acid profiles. J Dairy Sci. 101(6):5582–5598. doi: 10.3168/jds.2017-13052.
- Van Lingen HJ, Crompton LA, Hendriks WH, Reynolds CK, Dijkstra J. 2014. Meta-analysis of relationships between enteric methane yield and milk fatty acid profile in dairy cattle. J Dairy Sci. 97(11):7115–7132. doi: 10.3168/jds.2014-8268.
- Vermorel M, Jouany JP, Eugène M, Sauvant D, Noblet J, Dourmad JY. 2008. Evaluation quantitative des émissions de méthane entérique par les animaux d’élevage en 2007 en France. INRA Prod Anim. 21:403–418.