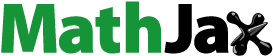
Abstract
The aim of this study was to assess the most important risk factors that influence productive life (PL) and to estimate genetic parameters for PL using a Weibull proportional hazards model in two Spanish dairy goat breeds belonging to different production systems. The dataset consisted of 70,695 and 49,176 lactation records of Florida and Payoya females, respectively, collected over the last two decades. A total of 6,227 (24.21%) Florida and 3,452 (20.42%) Payoya females had censored information. The pedigree included a total of 56,901 animals for Florida and 59,957 for Payoya. The effects included in the model for both breeds were the age at first kidding and the herd-year-season of birth of the doe as time-independent effects; and the age at kidding, the herd-year-season of kidding, the within-herd class of milk production deviation and the combination between the lactation number and the duration of lactation as time-dependent effects. Estimation of genetic parameters was made using a Weibull proportional hazards model using Survival Kit V6.0 software. The results from the Weibull animal models gave estimates of additive genetic variances of 0.99 ± 0.0003 and 0.156 ± 0.021, which corresponds to a heritability of 0.43 ± 0.025 and 0.11 ± 0.021 for Florida and Payoya, respectively. The magnitude of heritability obtained for PL suggests that a substantial response to selection may be expected for longevity, especially in the Florida breed. These results may contribute to the development of a routine genetic evaluation to improve PL in Spanish dairy goat breeds.
HIGHLIGHTS
A Weibull proportional hazards model has been used to analyse productive life in Spanish goat breeds raised under different production systems.
Productive life is influenced by many factors, with milk production the most important.
According to the heritability estimated, a significant genetic response could be obtained by direct selection for productive life, especially in the Florida breed.
Introduction
The purpose of breeding programs in Spanish dairy goats is to increase farmers’ profitability and, currently, selection is carried out for milk production and composition traits (Molina et al. Citation2018). The negative impact on other traits such as fertility and longevity have been widely commented (Pryce et al. Citation2004; Oltenacu and Algers Citation2005). For this reason, in recent years, there has been a growing interest in evaluating factors of economic importance, other than those currently used in the breeding program, such as the somatic cell score (Jimenez-Granado et al. Citation2022), as well as morphological, and more recently, reproductive traits (Ziadi, Muñoz-Mejías, Sánchez et al. Citation2021; Ziadi, Muñoz-Mejías, Sánchez Rodríguez et al. Citation2021).
Longevity is an indirect global trait used to quantify the productive lifespan of livestock, and for increasing the profitability of farms. In dairy ruminants, the definitions of longevity include: (i) true longevity (all culling reasons, including milk productivity); and (ii) functional longevity (all culling reasons, except milk productivity) (Sasaki Citation2013). The increase in the functional life of females can result in a fall in replacement costs, because of the better utilisation of the animals, which therefore increases farm incomes.
The genetic basis of longevity, or similar traits, such as stayability or survival in dairy goats, has been documented in various studies using repeatability linear and threshold models (Castañeda-Bustos et al. Citation2014; Geddes et al. Citation2018; Palhière et al. Citation2018; Scholtens et al. Citation2018). Regarding the methods for estimating genetic parameters of longevity, the approaches used include threshold, random regression and proportional hazard models (PH). PH procedures model the hazard function of failure (death or culling of a goat) at every time t, from the starting point of the productive life of the female (generally the first kidding), to the last observation time, which could be culling, or death or censoring time (Ducrocq et al. Citation1988). In addition, survival analysis using PH models may provide useful information for stayability studies. These analyses can accurately accommodate censored data, consider the time-dependent environmental impact, and manage the skewed distribution of longevity characteristics (Colosimo and Giolo Citation2006; Imbayarwo-Chikosi et al. Citation2018). In addition, linear, threshold, and random regression models generally produce a lower estimation of longevity heritability than survival analysis models on the original scale (Ducrocq Citation1997; Jamrozik and Schaeffer Citation1997; Kern et al. Citation2014).
Up to now, estimations of genetic parameters in dairy goat longevity with survival analysis have only been available in a single study (Ziadi et al. Citation2023), in the Spanish Florida breed raised under intensive to semi-intensive production systems.
Nevertheless, in the dairy goat sector in Spain in general, and Andalusia in particular, there are a multitude of production systems with their own characteristics, which can be grouped into three main categories: semi-extensive or mixed, semi-intensive and intensive. The Payoya breed represents a production system with a high level of extensification, while the Florida breed presents a higher level of intensification.
Thus, the aim of this study was to evaluate the possible risk factors and to estimate genetic parameters for productive life in the Florida and Payoya dairy goat breeds, as examples of Spanish breeds with different production systems, using a survival analysis based on the Weibull proportional hazards model.
Materials and methods
Data
The dataset used consisted of data on the production and pedigree available from the National Association of Florida Goat Breeders (ACRIFLOR) and the Association of Payoya Goat Breeders (ACAPA) for the Florida and Payoya breeds, respectively. The Payoya breed is raised under semi-extensive to extensive production systems, while, for the Florida breed, semi-intensive to intensive production systems are used. In the analysis, we included records on Florida and Payoya females born between 2004–2018 and 1998–2020, with at least the first kidding occurring between 2006–2020 and 2000–2021, respectively. Female longevity was measured through the productive life (PL), calculated as the total days in milk in a female’s life between the first kidding and the last known lactation end date or culling. Contemporary groups with less than ten records were removed from the analysis. Does younger than 12 or older than 24.6 months at first kidding and females with an incomplete record (age at the first kidding unknown or some uncontrolled intermediate kidding) were also pruned out. After data editing, the final dataset consisted of 70,695 and 49,176 lactation records from 25,722 and 13,608 females belonging to 83 and 41 genetically-linked herds for Florida and Payoya, respectively. Genetic links between the herds were ensured by the use of artificial insemination and the sale of bucks. The does were classified as presenting records which were uncensored (for dead animals) or right-censored (for animals living at the time of the analysis). A total of 19,495 and 13,446 does had completed their productive life, while 6,227 (24.21%) and 3,452 (20.42%) does had censored information for Florida and Payoya, respectively.
The pedigree was traced back for as many generations as available in the breed herd book, and the additive genetic relationship matrix included a total of 56,901 animals for Florida and 59,957 for Payoya.
Estimation of genetic parameters
The productive life of the does was evaluated by survival analysis, using a Weibull proportional hazards model (Ducrocq Citation1994).
The analysis was performed using the following model for both breeds:
where λ(t) is the hazard function (current probability of culling) of the doe t days after its first kidding, λ0(t) is the baseline hazard function t days after the most recent kidding; agefirsti is the time-independent effect of age i at first kidding (monthly intervals from 12 to 15; >15 to 19 and >19 to 24); birth_herd*year*seasonj is the time-independent effect of herd j combined with the year and season of birth of the doe (667 and 456 classes for Florida and Payoya, respectively); kidding_herd*year*seasonk is the time-dependent effect of herd k combined with the year and season of kidding (829 and 388 classes for Florida and Payoya, respectively); agel is the time-dependent effect of age l at kidding (monthly intervals from 15 to 19; > 19 to 24; > 24 to 36; > 36 to 48; > 48 to 60 and > 60 mo); levelprodm is the time-dependent effect of the within-herd class of milk production deviation m (4 classes) - this effect was calculated as the annual deviation of milk production for each doe with respect to the average production of its herd; and lactation number*duration of lactation is the time-dependent effect of lactation number n (1–6), combined with duration of lactation (1–4) – with the classes of lactation duration defined by cut-off points empirically as follows: 90d to 210d; > 210d to 240d; > 240d to 300d, and 300d to 400d for Florida; 90d to 200d; > 200d to 240d; > 240d to 280d, and 280d to 400d for Payoya; finally, go is the random additive genetic value of animal o in the pedigree. The statistical significance of the fixed effects in the proportional hazard model was tested using Likelihood ratio tests.
The survival analysis was performed using the Survival Kit V6.0 software package (Mészáros et al. Citation2013). Given the non-linear nature of the models used in the survival analysis, there is no heritability definition equivalent to that of linear models.
The formula to estimate the heritability for the animal model of Mészáros et al. (Citation2010) was modified by removing the term corresponding to the herd-year variance as follows:
where
is the additive genetic variance and P is the proportion of uncensored records.
The reliability R2 for each estimated breeding value (EBV) was estimated as:
where pev is the prediction error variance calculated as the square of the standard error for each EBV.
Results and discussion
The distribution of censored and uncensored records for productive life (PL) in the Florida and Payoya breeds is summarised in . The proportion of right-censored records was quite low (24.21% and 20.42% for Florida and Payoya, respectively), with both values being smaller than that reported in New Zealand dairy goats (32.85%; Gautam et al. Citation2017) and greater than the value observed in the Saanen breed (19.52%; Ferreira et al. Citation2020), being the definition of longevity and the models used in these two studies different from ours. PL ranged from 127 to 3445 days (4.16 to 113 months) and from 90 to 3537 days (3 to 116 months) for Florida and Payoya, respectively. Average censoring age and average failure age after first kidding were 1,096.93 − 1,445.55 days (36 – 47 months), and 1,390.95 – 1,490.04 days (45.6 − 48.8 months) for Florida and Payoya, respectively. Previous studies with different definitions of longevity in dairy goats estimated an average of 625 d for productive life defined as the total days in production until 72 months of age in US breeds (Castañeda-Bustos et al. Citation2017), 1,726 d for length of true life and 1,121.68 d for mean lifetime in milk in UK dairy goats (Geddes et al. Citation2018), 967 d for functional longevity in Saanen and 1,007 d in Alpine goats (Palhière et al. Citation2018).
Table 1. Descriptive statistics of censoring and failure time in the Florida and Payoya breeds.
Female longevity is a trait of the utmost importance, especially in dairy breeds with a high level of intensification, where females are subjected to more productive stress and their longevity is reduced, as the Florida breed. This breed is known for its high milk production, with an average milk yield of 611.4 ± 7.8 kg (ARCA Citation2021) per lactation. In fact, only 52.7% of Florida females have more than six parities, compared with 78.6% in the Payoya breed (Ziadi, Muñoz-Mejías, Sánchez et al. Citation2021). In addition, in breeds classified as being in risk of extinction, as is the case of Payoya, extending the productive life is highly advisable, because it allows females to be kept longer in the herd and produce a larger number of kiddings and lactations. In addition, extending the life of breeding females involves increasing the generation interval, and therefore reducing consanguinity per unit of time. For this reason, the inclusion of longevity traits in the selection program of these breeds could be highly relevant in order to increase the productive life of the females and the chances of the breed’s survival as a population.
Fixed effects and culling risks
The results of the Likelihood ratio tests assessing the statistical relevance of all the factors involved in defining hazard risk in the doe are presented in . All covariates in the fixed model were found to be highly significant at p < 0.00001.
Table 2. Likelihood ratio last test statement for productive life (PL) in the Florida and Payoya breeds.
The estimates of the time-independent and time-dependent fixed effects, expressed as a log-risk ratio, are shown in and for the Florida and Payoya breeds. The log-risk ratio increased linearly with age at first kidding in both breeds (). Does with a very early first kidding (12–15 months of age) showed a lower risk of culling compared to does kidding for the first time after 19 months of age. In this context, a negative relationship was also observed between late age at first parity and longevity in other studies in dairy cattle (Terawaki et al. Citation2006; Imbayarwo‐Chikosi et al. Citation2017). Late kidding is presumably caused by certain problems associated with herd management, fertility and other health problems. Consequently, the lifetime production of a doe that starts its reproduction life late will never be totally compensated, since this animal will be limited by the number of lactations and the average lactation length (Sewalem et al. Citation2005).
Figure 1. Time-independent effect of the age at first kidding expressed as culling log-risk ratio for productive life in survival analysis in the Florida and Payoya breeds.

Figure 2. Time-dependent effects expressed as the culling log-risk ratio for productive life survival analysis in the Florida and Payoya breeds.

It was noteworthy that, for the age at kidding, the level with the highest log-risk ratio was observed in young females (>12–15 months) in both breeds, which then decreased suddenly in the second age group at kidding and then decreased and remained stable until the last age groups (). This sudden decrease in Florida in the second age group can be explained by the fact that, in this breed, due to its higher level of intensification, a high-intensity selection process is applied in females between the first and second parities. Indeed, 24.7% of the Florida females are culled between the first and second parities, compared to only 14.8% in Payoya (Ziadi, Muñoz-Mejías, Sánchez et al. Citation2021).
The log-risk ratios showed big differences in herd-year season of doe birth, which reflected the importance of this effect on PL: these were from −5.77 to 6.50 in Florida and from −5.57 to 3.88 in Payoya (Supplementary Figure S1). There was also a wide variation in the log-risk ratios from one doe to another in herd-year-season of kidding (from −7.52 to 3.24 in Florida and from −5.24 to 4.58 in Payoya) (Supplementary Figure S2). This large discrepancy between herds could be explained by the differences in management practices, including nutrition, health, reproduction and strategy for replacing females. For instance, the range of variation of the log-risk ratio was greater in Florida than in Payoya. Despite suffering harsher environmental conditions in the case of the Payoya, its breeding area is very small (region of Andalusia), so the environmental conditions of the herds are quite similar. On the other hand, the Florida breed, although it has a more intensive system, is exploited throughout the Iberian Peninsula (Spain and Portugal) and other countries, such as France and Italy, with very different environmental conditions.
The log-risk ratio for does in the different within-herd class of milk production deviation showed a distinct pattern for productive life. Females that produce low milk yields were at a higher risk of culling (0.31 and 0.12 for Florida and Payoya, respectively), while high-producing does were at lower risk of being culled (−0.56 and −0.38 for Florida and Payoya, respectively) (). For the low level of milk production, Florida females are at a higher risk than Payoya does because of the high-intensity selection process applied in the case of intensive production systems. Milk production is one of the most important traits in dairy breeds, and has a strong influence on the decision to cull females from the herd. Many studies for longevity in dairy breeds have included milk production in the survival model because voluntary culling is based upon phenotypic production (Kern et al. Citation2016; Imbayarwo‐Chikosi et al. Citation2017), and high-producing females are less likely to be culled than low-producing females from the same herd (Vukasinovic et al. Citation2001).
The log-risk ratios of the lactation number*duration of lactation interaction in both breeds is shown in . As can be observed, the log-risk ratio increased from the first to the second lactation and was more pronounced in the Florida breed, then decreased notably and continuously until the last lactations. Also, while the risk of being culled differed throughout the lactations in both breeds, it does not present a clear tendency. In dairy cattle, the same risk factors were found to greatly influence longevity (M'hamdi et al. Citation2010; Kern et al. Citation2016; Imbayarwo‐Chikosi et al. Citation2017). In Spanish dairy goats, only one study has been conducted to assess environmental factors that influence the length of productive life in the Florida breed (Ziadi et al. Citation2023), and this produced the same results as those found in this study. This previous study was conducted to obtain preliminary results with a different measure of longevity than that of the current one and applying a simpler approach (the Cox model), which does not require specific assumptions about the shape of the survival distribution. In the actual study, we have opted to include the Payoya breed along with Florida to allow comparison of this trait of longevity between two distinct production systems: extensive for the Payoya breed and more intensive for the Florida breed.
Also, in dairy goats, studies have been carried out to identify risk factors for the culling of females (Malher et al. Citation2001; Pérez-Razo et al. Citation2004; Gautam et al. Citation2017; Ferreira et al. Citation2020), although they remain scarce in comparison with the abundant information available for dairy cattle.
According to Terawaki et al. (Citation2006), culling during the early stage of lactation corresponds to extreme cases, such as those animals with a very low milk production or severe functional problems. In contrast, the risk of culling is greater at the end of lactation, when production is lower, when it is known whether the female is pregnant or not and when her body condition score is better (Ducrocq Citation1999).
The Weibull parameter ρ, which describes the shape of the baseline hazard function, was 4.64 for Florida and 2.49 for Payoya, corroborating the fact that Florida females are at a much higher and more immediate risk of being culled than Payoya does, due to their intensive production systems.
Heritability, EBVs and reliability
The estimates of parameters from the Weibull survival analysis for PL and the derived parameters (heritability, EBV averages, reliability, and risk averages) are presented in . The marginal posterior mean estimates of the additive genetic variance in this study were 0.99 ± 0.0003 and 0.156 ± 0.021, which corresponds to a heritability of 0.43 ± 0.025 and 0.11 ± 0.021 for Florida and Payoya, respectively. This finding suggests that there is a genetic variability for longevity of goat does and reasonable genetic improvement in productive life can be achieved through selection in Florida and Payoya breeds. The lower h2 observed for Payoya could be due to the fact that this breed is exploited under a more extensive production system than the Florida breed and lactations suffer more management variations. The heritability of different definitions of longevity estimated in earlier studies in dairy goats has produced values of around 10% (Castañeda-Bustos et al. Citation2017; Palhière et al. Citation2018). However, these estimates were made by linear models.
Table 3. Estimates of parameters from Weibull analysis for productive life (PL) in the Florida and Payoya breeds.
The genetic analysis of longevity in dairy goats using survival analysis is available in only one study for another definition of longevity (length of productive life) in the Florida breed, with a value of 0.58 (Ziadi et al. Citation2023). In this same breed, with a Cox model, not including the effects related to milk production, an h2 of 0.17 was obtained (Ziadi et al. Citation2022). This result highlighted the importance of milk production in the productive life of dairy females, and ignoring this effect in the survival model leads to an underestimation of the heritability of this trait.
Additionally, estimates of reliability by survival analysis depend on the proportion of censored and uncensored records available, and increase as the ratio of censored records decreases (Yazdi et al. Citation2002). In this work, 24,21% and 19.16% of the data employed was censored for Florida and Payoya, respectively. These values are much lower than the maximum limit established by Vukasinovic et al. (Citation1997), who recommended not more than 40% of censored records in order to obtain accurate estimates.
Finally, the phenotypic and genetic (expressed in average standardised female EBVs) trends over time for productive life are shown in for both breeds. The phenotypic trajectory presented a decrease in both breeds. In the same way, trends in breeding values showed a decline of −0.64 and −0.85 in genetic standard deviations for the period 2004–2018 (−0.05 and −0.06 per year) in Florida and Payoya, respectively. Positive EBVs corresponded to a lower risk of doe culling, whilst negative EBVs implied a higher risk of culling. This means that young does had a lower probability of staying in the herd longer than their mothers and, as a consequence, a lower probability of completing an increased number of kiddings and lactations. Similarly, (Jenko et al. Citation2013; Imbayarwo-Chikosi et al. Citation2018) observed a similar unfavourable trend in Slovenian Brown cattle and South African cattle, respectively. According to these authors, this negative genetic trend could have been due to the decline in functional traits (e.g. fertility and udder health traits) resulting from intense selection for milk production. Indeed, an increased incidence of somatic cell count (SCC) was observed in the Florida breed (Jimenez-Granado et al. Citation2022), the reason for this increase in SCC being the intense selection of females for high milk production, leading to a greater, constant interaction between the udder and the milking equipment, thus increasing the likelihood of mammary gland infections.
Conclusions
According to our results, functional longevity in Florida and Payoya dairy goat breeds is influenced by the age at first kidding, herd-year-season of birth of the doe, age at kidding, herd-year-season of kidding, milk production level and lactation number with duration of lactation interaction. Improving these sources of voluntary culling helps to approximate functional longevity, defined as the aptitude to delay involuntary culling. The estimate of heritability of productive life was high for the Florida breed and low for Payoya, but indicated that at least some improvement can be achieved through direct selection for this trait. Furthermore, the declining average breeding values over time suggest that longevity trait should be included in the genetic evaluation of Spanish goat breeds as a way of increasing dairy farm profitability.
Ethical approval
This study did not require manipulation or any change in the usual handling of the animals, since we have worked directly with the routine records provided by the breeders’ associations of the Florida and Payoya breeds.
Supplemental Material
Download MS Word (529.6 KB)Acknowledgments
The authors thank the ACRIFLOR (Asociación Nacional de Criadores de Ganado Caprino de Raza Florida – National Association of Florida Goat Breeders) and the ACAPA (Asociación de Criadores de Raza Caprina Payoya – Association of Payoya Goat Breeders) for kindly providing the data used in this study.
Disclosure statement
No potential conflict of interest was reported by the author(s).
Data availability statement
The data that support the findings of this study are available on request from the corresponding author with the permission of ACRIFLOR (National Association of Florida Goat Breeders) and ACAPA (Association of Payoya Goat Breeders).
References
- ARCA. 2021. Sistema Nacional De Información De Razas. Raza Caprina Florida, Ministerio De Agricultura, Alimentación Y Medio Ambiente. Gobierno De España. https://www.mapa.gob.es/es/ganaderia/temas/zootecnia/razas-ganaderas/razas/catalogo-razas/caprino/florida/default.aspx
- Castañeda-Bustos V, Montaldo H, Valencia-Posadas M, Shepard L, Pérez-Elizalde S, Hernández-Mendo O, Torres-Hernández G. 2017. Linear and nonlinear genetic relationships between type traits and productive life in US dairy goats. J Dairy Sci. 100(2):1232–1245. doi:10.3168/jds.2016-11313.
- Castañeda-Bustos VJ, Montaldo HH, Torres-Hernández G, Pérez-Elizalde S, Valencia-Posadas M, Hernández-Mendo O, Shepard L. 2014. Estimation of genetic parameters for productive life, reproduction, and milk-production traits in US dairy goats. J Dairy Sci. 97(4):2462–2473. doi:10.3168/jds.2013-7503.
- Colosimo EA, Giolo SR. 2006. Análise de sobrevivência aplicada. São Paulo: Editora Edgard Blucher.
- Ducrocq V. 1994. Statistical analysis of length of productive life for dairy cows of the Normande breed. J Dairy Sci. 77(3):855–866. doi:10.3168/jds.S0022-0302(94)77020-X.
- Ducrocq V. 1997. Survival analysis, a statistical tool for longevity data. In: 48th annual meeting of the European association for animal production. 14. p; Vienna, Austria.
- Ducrocq V. 1999. Topics that may deserve further attention in survival analysis applied to dairy cattle breeding: some suggestions. Interbull Bulletin. 21:181–181.
- Ducrocq V, Quaas R, Pollak E, Casella G. 1988. Length of productive life of dairy cows. 2. Variance component estimation and sire evaluation. J Dairy Sci. 71(11):3071–3079. doi:10.3168/jds.S0022-0302(88)79907-5.
- Ferreira TA, Martins PG, Gouveia GC, Abreu LR, Gouveia AM, Facó O, Colosimo EA, Furusho-Garcia IF, Pereira IG. 2020. Proportional hazard models associated with the survival of dairy goats reared in a tropical environment. Small Ruminant Research. 184:106063. doi:10.1016/j.smallrumres.2020.106063.
- Gautam M, Stevenson MA, Lopez-Villalobos N, McLean V. 2017. Risk factors for culling, sales and deaths in New Zealand dairy goat herds, 2000–2009. Front Vet Sci. 4:191. doi:10.3389/fvets.2017.00191.
- Geddes L, Desire S, Mucha S, Coffey M, Mrode RA, Conington J. 2018. Genetic parameters for longevity traits in UK dairy goats. Proceedings of the 11th World Congress on Genetics Applied to Livestock Production; Auckland, New Zealand. p. 547.
- Imbayarwo-Chikosi VE, Ducrocq V, Banga CB, Halimani TE, Van Wyk JB, Maiwashe A, Dzama K. 2018. Impact of conformation traits on functional longevity in South African Holstein cattle. Anim Prod Sci. 58(3):481–488. doi:10.1071/AN16387.
- Imbayarwo‐Chikosi V, Ducrocq V, Banga C, Halimani T, Van Wyk J, Maiwashe A, Dzama K. 2017. Estimation of genetic parameters for functional longevity in the South African Holstein cattle using a piecewise Weibull proportional hazards model. J Anim Breed Genet. 134(5):364–372. doi:10.1111/jbg.12264.
- Jamrozik J, Schaeffer LR. 1997. Estimates of genetic parameters for a test day model with random regressions for yield traits of first lactation holsteins. J Dairy Sci. 80(4):762–770. doi:10.3168/jds.S0022-0302(97)75996-4.
- Jenko J, Ducrocq V, Kovač M. 2013. Comparison of piecewise Weibull baseline survival models for estimation of true and functional longevity in Brown cattle raised in small herds. Animal. 7(10):1583–1591. doi:10.1017/S1751731113001055.
- Jimenez-Granado R, Molina A, Ziadi C, Sanchez M, Muñoz-Mejías E, Demyda-Peyrás S, Menendez-Buxadera A. 2022. Genetic parameters of somatic cell score in florida goats using single and multiple traits models. Animals. 12(8):1009. doi:10.3390/ani12081009.
- Kern EL, Cobuci JA, Costa CN, Ducrocq V. 2016. Survival analysis of productive life in Brazilian holstein using a piecewise Weibull proportional hazard model. Livestock Science. 185:89–96. doi:10.1016/j.livsci.2016.01.019.
- Kern EL, Cobuci JA, Costa CN, McManus CM, Campos GS, Almeida TP, Campos RV. 2014. Genetic association between herd survival and linear type traits in Holstein cows under tropical conditions. Ital J Anim Sci. 13(3):3419. doi:10.4081/ijas.2014.3419.
- M'hamdi N, Aloulou R, Bouallegue M, Brar SK, Hamouda MB. 2010. Study on functional longevity of Tunisian Holstein dairy cattle using a Weibull proportional hazard model. Livestock Sci. 132(1-3):173–176. doi:10.1016/j.livsci.2010.05.011.
- Malher X, Seegers H, Beaudeau F. 2001. Culling and mortality in large dairy goat herds managed under intensive conditions in western France. Livestock Produc Sci. 71(1):75–86. doi:10.1016/S0301-6226(01)00242-1.
- Mészáros G, Pálos J, Ducrocq V, Sölkner J. 2010. Heritability of longevity in Large White and Landrace sows using continuous time and grouped data models. Genet Sel Evol. 42(1):13. doi:10.1186/1297-9686-42-13.
- Mészáros G, Sölkner J, Ducrocq V. 2013. The Survival Kit: software to analyze survival data including possibly correlated random effects. Comput Methods Programs Biomed. 110(3):503–510. doi:10.1016/j.cmpb.2013.01.010.
- Molina A, Muñoz E, Díaz C, Menéndez-Buxadera A, Ramón M, Sánchez M, Carabaño MJ, Serradilla JM. 2018. Goat genomic selection: impact of the integration of genomic information in the genetic evaluations of the Spanish Florida goats. Small Ruminant Res. 163:72–75. doi:10.1016/j.smallrumres.2017.12.010.
- Oltenacu PA, Algers B. 2005. Selection for increased production and the welfare of dairy cows: are new breeding goals needed? AMBIO J Hum Environ. 34(4):311–315. doi:10.1579/0044-7447-34.4.311.
- Palhière I, Oget-Ebrad C, Rupp R. 2018. Functional longevity is heritable and controlled by a major gene in French dairy goats. Proceedings of the 11th World Congress on Genetics Applied to Livestock Production; Auckland, New Zealand. p. 11–165.
- Pérez-Razo M, Sánchez F, Torres-Hernández G, Becerril-Pérez C, Gallegos-Sánchez J, González-Cosío F, Meza-Herrera C. 2004. Risk factors associated with dairy goats stayability. Livestock Production Science. 89(2-3):139–146. doi:10.1016/j.livprodsci.2004.02.008.
- Pryce J, Royal M, Garnsworthy P, Mao I. 2004. Fertility in the high-producing dairy cow. Livestock Production Science. 86(1-3):125–135. doi:10.1016/S0301-6226(03)00145-3.
- Sasaki O. 2013. Estimation of genetic parameters for longevity traits in dairy cattle: A review with focus on the characteristics of analytical models. Anim Sci J. 84(6):449–460. doi:10.1111/asj.12066.
- Scholtens M, Lopez-Villalobos N, Garrick D, Blair H. 2018. Heritability of longevity in New Zealand dairy goats. NZJ Anim Sci Prod. 78:11–15.
- Sewalem A, Kistemaker G, Ducrocq V, Van Doormaal B. 2005. Genetic analysis of herd life in Canadian dairy cattle on a lactation basis using a Weibull proportional hazards model. J Dairy Sci. 88(1):368–375. doi:10.3168/jds.S0022-0302(05)72696-5.
- Terawaki Y, Katsumi T, Ducrocq V. 2006. Development of a survival model with piecewise Weibull baselines for the analysis of length of productive life of Holstein cows in Japan. J Dairy Sci. 89(10):4058–4065. doi:10.3168/jds.S0022-0302(06)72449-3.
- Vukasinovic N, Moll J, Casanova L. 2001. Implementation of a routine genetic evaluation for longevity based on survival analysis techniques in dairy cattle populations in Switzerland. J Dairy Sci. 84(9):2073–2080. doi:10.3168/jds.S0022-0302(01)74652-8.
- Vukasinovic N, Moll J, Künzi N. 1997. Analysis of productive life in Swiss Brown cattle. J Dairy Sci. 80(10):2572–2579. doi:10.3168/jds.S0022-0302(97)76213-1.
- Yazdi M, Visscher P, Ducrocq V, Thompson R. 2002. Heritability, reliability of genetic evaluations and response to selection in proportional hazard models. J Dairy Sci. 85(6):1563–1577. doi:10.3168/jds.S0022-0302(02)74226-4.
- Ziadi C, Muñoz-Mejías E, Sánchez M, López MD, González-Casquet O, Molina A. 2021. Selection criteria for improving fertility in spanish goat breeds: estimation of genetic parameters and designing selection indices for optimal genetic responses. Animals. 11(2):409. doi:10.3390/ani11020409.
- Ziadi C, Muñoz-Mejías E, Sánchez Rodríguez M, López MD, González-Casquet O, Molina Alcalá A. 2021. Genetic analysis of litter size and number of kids born alive across parities in Spanish goat breeds using a random regression model. Ital J Anim Sci. 20(1):94–101. doi:10.1080/1828051X.2020.1869601.
- Ziadi C, Sánchez JP, Sánchez M, Morales R, Molina A. 2023. Survival analysis of productive life in Florida dairy goats using a Cox proportional hazards model. J Animal Breeding Genetics. 140(4):431–439. n/a(n/a)() doi:10.1111/jbg.12769.
- Ziadi C, Sánchez JP, Morales R, Muñoz-Mejías E, Sánchez M, López MD, Molina A. 2022. Genetic analysis of productive life in the Florida goat breed using a Cox proportional hazards model. In: EAAP – 73rd Annual Meeting, Porto, Portugal.