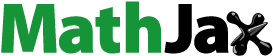
ABSTRACT
Ephemeral digital trace data can decrease the completeness, reproducibility, and reliability of social media datasets. Systematic post deletions thus potentially bias the results of computational methods used to map actors, content, and online information diffusion. Therefore, the aim of this study was to assess the extent and distribution of message deletion across different data types using data from the hybrid messenger service Telegram, which has experienced an influx of deplatformed users from mainstream social media platforms. A repeatedly scraped sample of messages from public Telegram groups and channels was used to investigate the effect of message ephemerality on the consistency of Telegram datasets. The findings revealed that message deletion introduces biases to the computational collection and analysis of Telegram data. Further, message ephemerality reduces dataset consistency, the quality of social network analyses, and the results of computational content analysis methods, such as topic modeling or dictionaries. The implications of these findings for scholars aiming to use Telegram data for computational research, possible solutions, and contributions to the methodological advancement of studying online political communication are discussed further in this article.
Digital media in general, and social media platforms in particular, have contributed to the pluralization, extension, and dispersion of the public sphere (Dahlgren, Citation2005). Political deliberation is enabled by online platforms and thus less confined by the traditional gatekeeping structures of mediating legacy media (Bruns, Citation2008). The digital trace data produced in the process facilitates large-sample computational social science studies (van Atteveldt & Peng, Citation2018) on the topics, dynamics, and outcomes of such deliberation processes.
Most social media platforms allow message deletion, which increases the fluid and ephemeral nature of the digital trace data derived from them. The reasons for message deletion are manifold, ranging from involuntary deletions caused by platform governance or state censorship to voluntary deletions motivated by political tactics, personal regret or privacy concerns. With respect to political communication, partisan posts are especially at risk of deletion (Bastos, Citation2021). The technical possibility of data ephemerality may also be perceived as platform affordance by users (Neubaum & Weeks, Citation2022).
Ephemeral social media trace data increase the possibility of incomplete, irreproducible, and unreliable datasets (Fang et al., Citation2020; Walker, Citation2017). Partial, nonrandom social media data inaccessibility can potentially bias subsequent data analyses, as Ho (Citation2020) and Morstatter et al. (Citation2013) have reported. Rogers (Citation2020) stated that the unavailability of removed posts poses a hindrance to the critical assessment of social media platforms.
Telegram is a popular platform for users with counter-hegemonic political opinions that are prone to message deletion. Its popularity as an organizational tool for online connective action (Bennett & Segerberg, Citation2012) and the formation of counterpublics in the networked public sphere (Kaiser & Rauchfleisch, Citation2019) has spurred an increase in scholarly research concerning public communication on this platform. Currently, the analysis of public communication on social media platforms relies heavily on social network and automated content analyses of text or image data. This is also the case with the growing literature on the different political actors leveraging Telegram’s mass communication features.
While both these major approaches – that is, social network analysis and automated content analysis – are applied in computational studies on Telegram-based communication and are helpful for mapping actors, content, and the diffusion of topics (Heft & Buehling, Citation2022), they might be sensitive to message deletion. However, a systematic study of message deletion practices on Telegram and their implications for research on contested political communication is, to date, unavailable in the literature. Therefore, bridging this gap was the aim of the present study. By doing so, the objective was to provide researchers with insights about data collection on Telegram and the consequences of the data collection’s timing
To evaluate the extent of possible biases that emerge from the selective deletion of specific message data types, repeatedly scraped message data from 25 public Groups and 25 public ChannelsFootnote1 for political communication were collected and analyzed after two time intervals. In addition to determining the data types deleted, this study was conducted to identify the data types that are disproportionally prone to deletion. Further, the word distribution of deleted and nondeleted text data was analyzed. Several computational content classification models were used to determine the extent to which message deletion introduces biased results in these methods. Significant effects on the outcomes of content analysis using a computational dictionary and topic modeling were found. An evaluation of hyperlink and platform-internal reference distributions was conducted to identify the potential biases in the data used in social network analyses.Footnote2
The theoretical contribution of this study is the descriptive quantification of message deletion, taking into account different data types and technical platform features. The biases that occur in computational content analyses and (cross-platform) network analyses are highlighted in this article. The practical relevance of this analysis for the investigation of public communication on Telegram arises from the technical functionalities of the Telegram API used by most researchers: When scraping public Groups or Channels, all messages are referenced by unique, consecutively numbered identifiers. Researchers analyzing a given Channel or Group can easily determine the amount of missing data in the scraped messages and the research limitations that may arise.
The following section of this paper presents a literature review on the data deletion practices of social media platforms in general as well as an overview of the functionalities and users of Telegram as a hybrid platform. Then, the research questions of this study are stated, and a detailed account of the data and methods used is provided. The results show that a disproportionally large share of text messages is deleted from Group chats, resulting in biased top-term distributions, in turn biasing the results of computational content analyses. The distributions of most-used hyperlinks are also skewed, leading to possible biases in subsequent hyperlink analyses. Telegram-internal references are only slightly affected by message ephemerality. Subsequently, a discussion of these results and the possible implications for scholars working with Telegram data are presented.
Literature review
Data deletion practices on social media platforms
Digital trace data, such as the data derived from observations of public communication on social media platforms, have allowed social scientists to conduct studies with large sample sizes (van Atteveldt & Peng, Citation2018). However, the online communication data used in these studies varied depending on the time difference between the original creation of the communication and data collection by researchers (Bachl, Citation2018; Schatto-Eckrodt, Citation2022; Walker, Citation2017). This data ephemerality in the social media context has been studied from different angles.
Previous research has uncovered the post and profile characteristics that increase the likelihood of content deletion on major social media platforms such as Twitter (Volkova & Bell, Citation2017) and YouTube (Kurdi et al., Citation2020). These deletions are partly explained by platform governance (Gorwa, Citation2019) and state censorship (Bamman et al., Citation2012; King et al., Citation2014). While these are unlikely to be the causes of message deletions on Telegram, given its minimal compliance with national lawmakers (Maréchal, Citation2018), there is a rich amount of literature on the reasons for and determinants of individual content deletion.
The literature shows that voluntary message deletion occurs when users try to preserve desirable external perceptions. Posts that users regret and subsequently delete often involve topics such as the use of intoxicants, politics, and religion or contain profane language (Wang et al., Citation2011). Almuhimedi et al. (Citation2013) showed the prevalence of these reasons for regret in a large Twitter dataset. Xu et al. (Citation2013) examined the extent to which tweets associated with bullying are deleted by either the bully or the victim. With the aim of curating their personal timelines and corresponding external perceptions, teenagers and young adults tend to engage in the deletion of their own messages or comments left by other users (Gagrčin, Citation2022; Marwick & Boyd, Citation2014).
With respect to political debates, Bastos (Citation2021) analyzed Twitter posts of users in favor of and against Brexit and found that partisan posts were more likely to be deleted. The reason might be attributed to regret over posting low-quality content, as per the authors’ interpretations, or to platform moderation. Walker (Citation2017) concluded that data ephemerality is not only a function of the time lag between data creation and data scraping but is also connected to the contentiousness of the topic discussed. The affordance of message deletion in a political debate was discussed by Neubaum and Weeks (Citation2022). The authors stated that the data ephemerality introduced by this affordance might hinder the recording of the entirety of online political deliberation as well as incentivize individuals to share opinions that they perceive as harmful for themselves if recorded forever. Message deletion has been discussed as an affordance for political actors because it is often a part of protest tactics (Daffalla et al., Citation2021; Neumayer & Stald, Citation2014) where there is a perceived risk of communications about offline actions being accessed by authorities when frisking individual mobile devices. The motive of community protection by regularly deleting far-right political messages on Telegram has also been mentioned by Scheffler et al. (Citation2021) and Urman and Katz (Citation2022).
Telegram and its affordances for political actors
Telegram, launched in 2013 by the developers of the Russian social media platform VK, is promoted as a messenger app that supports and facilitates free speech (Maréchal, Citation2018). While it provides encrypted and nonencrypted one-to-one chats and closed (invite-only) group chats, the possibility of creating public Channels and Groups sets Telegram apart from competing encrypted chat messengers, such as WhatsApp, Signal, and Threema (Frischlich et al., Citation2022). Channels support one-to-many communication, in which distinct administrator(s) can broadcast messages to all users subscribing to a Channel. Public Groups allow for many-to-many communications among all members of a chat group. Urman et al. (Citation2021) argued that top – down broadcasting in Channels and personalized content sharing in Groups can foster collective and connective action, respectively. Both Channels and Groups can be found via an internal search function and joined by any Telegram user. Posts in Channels and Groups may include text, videos, pictures, audio files, hyperlinks, and all other data types. Further, messages from Groups or Channels can be forwarded to other Telegram entities, enabling platform-internal references (Baumgartner et al., Citation2020; Nobari et al., Citation2017). Due to the limitations of the search function, these forwarded messages serve as an essential facilitator of Group and Channel discovery by users and scholars (Peeters & Willaert, Citation2022).
Partial chat encryption and minimal compliance with national law due to the commitment to free speech (Maréchal, Citation2018) are affordances that drive the use of Telegram by political actors and people confronted with state repression. As Telegram’s terms of service only prohibit the promotion of violence and illegal pornography in public Channels (Telegram, Citation2022), users banned from other social media platforms have turned to Telegram as a platform with less content moderation (Bryanov et al., Citation2022; Rogers, Citation2020). This also holds for the regular users’ ability to communicate anonymously when facing governmental online censorship and communication barriers, as is the case in Iran (Akbari & Gabdulhakov, Citation2019; Kargar & McManamen, Citation2018), Russia (Akbari & Gabdulhakov, Citation2019; Ermoshina & Musiani, Citation2021), Belarus (Bykov et al., Citation2021; Wijermars & Lokot, Citation2022), and Hong Kong (Urman et al., Citation2021). In practice, Telegram reserves the right to ban Channels, Groups, or users; this has resulted in coordinated bans on Islamist Channels/Groups (Amarasingam et al., Citation2021; Conway et al., Citation2019) and targeted but nontransparent deplatforming of far-right terrorist Channels/Groups in the United States (Collier et al., Citation2021) and Germany (Frischlich et al., Citation2022; Gerster et al., Citation2021). The deletion or banning of Channels or Groups makes the content therein unavailable to users, but messages that were forwarded from those entities to other Groups and Channels remain available in the entity they have been forwarded to. Further, the deletion of a user account does not result in the unavailability of this user’s posts.
Despite tentative attempts at selective platform governance, most far-right and conspiracy theory actors can still rely on Telegram as a cornerstone for community building and communication strategies. Past research has shown that Telegram’s public chat functions are features that are used by different far-right actors in Great Britain (Bovet & Grindrod, Citation2020), the United States (Bryanov et al., Citation2022), and Germany (Gerster et al., Citation2021; Schulze et al., Citation2022; Urman & Katz, Citation2022). Adjacent and overlapping communities of conspiracy theory propagators have been found, for example, in the Netherlands (Peeters & Willaert, Citation2022), Germany (Gerster et al., Citation2021; Holzer, Citation2021; Hoseini et al., Citation2021), and English-, Spanish-, and Portuguese-speaking countries (Hoseini et al., Citation2021).
Objectives
With its increase in popularity as a tool for public communication, Telegram has sparked interest in the research community. However, as a consequence of individual message deletion, scholars looking to analyze Telegram data may have to work with incomplete datasets. Missing data result in information loss (Little & Schenker, Citation1995) and are known to bias study results if they differ systematically from available data.
Notably, Telegram has attracted an influx of far-right activists and conspiracists (Bryanov et al., Citation2022; Garry et al., Citation2021; Hoseini et al., Citation2021; Urman & Katz, Citation2022). To map the extent of their networks, as well as the prevalence and diffusion of their communications, researchers have turned to conducting computational content analyses and social network analyses (Heft & Buehling, Citation2022). However, the partisan political views expressed in such posts tend to have a higher probability of deletion (Bastos, Citation2021; Walker, Citation2017) for either personal reasons (Neubaum & Weeks, Citation2022) or tactical reasons (Daffalla et al., Citation2021; Neumayer & Stald, Citation2014). The incompleteness of social media data, whether for reasons of data ephemerality (Fang et al., Citation2020, Citation2022) or social media API restrictions (Ho, Citation2020; Morstatter et al., Citation2013), can result in biased results for subsequent analyses.
Further, Telegram allows for two methods of public communication, namely Groups and Channels. The former facilitates many-to-many communication and attributes message deletion rights to the Group’s administrator(s) and the message author. The latter enables one-to-many communication and thus grants author and moderation rights to the Channel’s administrator(s) only. Studies exploring Telegram communities have investigated one or both types of public communication. Therefore, considering the context of data collection from Groups and Channels on Telegram, the following research question was formulated for the present study:
RQ 1a: To what extent are messages deleted across Channels and Groups?
Data collection strategies on Telegram range from repeated data collection over a period of time (Urman & Katz, Citation2022) to one-time collection of historical communication data. To gain insights into the possible consequences of different data collection strategies on data completeness, the following research question was formed:
RQ 1b: To what extent does an increase in the time between original data creation and data collection affect the amount of missing messages in a dataset?
The text data derived from Telegram Channels and Groups in the context of political communication has previously been used to gain insights into the various issues discussed (Bryanov et al., Citation2022; Hoseini et al., Citation2021) and language used (Scheffler et al., Citation2021; Schulze et al., Citation2022). Further, visual content shared on fringe and mainstream social media platforms has been analyzed to quantify how racist and hate content is proliferated online (McSwiney et al., Citation2021; Zannettou et al., Citation2018) and what their characteristics are (Chen et al., Citation2022). Studies by Macklin (Citation2022) and Guhl and Davey (Citation2020) show the importance of multimedia content of (terrorist) far-right propaganda on Telegram. Analyses based on single data types as well as multimedia approaches to the analysis of Telegram data (Sosa & Sharoff, Citation2022) are prone to biased outcomes in case of a selective deletion of single data types. Accordingly, the following research questions were formed:
RQ 2a: Does the distribution of data types of deleted messages differ from that of nondeleted messages across Channels and Groups?
RQ 2b: To what extent does an increase in the time between original data creation and data collection affect the distribution of data types of deleted messages compared to that of nondeleted messages?
RQ 3a: To what extent does message deletion influence the overall word distribution in the data collected and, subsequently, the outcomes of computational content analyses across Channels and Groups?
RQ 3b: To what extent does an increase in the time between original data creation and data collection influence the overall word distribution in the data collected and, subsequently, the outcomes of computational content analyses?
The technical features of hyperlink embedding and entity forwarding enable embedding in both cross-platform information ecosystems and Telegram-internal actor and information ecosystems. Studies focusing on political communities on Telegram have used associated data to map platform-internal communities (Bovet & Grindrod, Citation2020; Garry et al., Citation2021; Gill, Citation2021; Zehring & Domahidi, Citation2023) and external information sources (Bryanov et al., Citation2022; Gerster et al., Citation2021). Further, the search function for Telegram Groups and Channels only provides minimal results, and results can only be retrieved if users know part of the channel name beforehand (Jalilvand & Neshati, Citation2020). To mimic the actual user behavior on Telegram (Peeters & Willaert, Citation2022), researchers often start with a set of Channels/Groups known ex-ante and then utilize forwarded messages for snowball sampling a more extensive set of entities (Baumgartner et al., Citation2020; Hoseini et al., Citation2021; Urman & Katz, Citation2022). Accordingly, the following research questions were formulated for the present study:
RQ 4a: To what extent does message deletion influence the overall distribution of platform-external and platform-internal references in the data collected across Channels and Groups?
RQ 4b: To what extent does an increase in the time between original data creation and data collection influence the overall distribution of platform-external and platform-internal references in the data collected?
Data and Methods
Data collection
The dataset analyzed in this study was collected from the public communication of the Querdenken movement on Telegram. Querdenken is a German movement acting as a central mobilization platform and forum against COVID-19 containment measures (Loucaides et al., Citation2021). Querdenken activists in decentralized local chapters organize street protests and online collective action (Bennett & Segerberg, Citation2012). As their primary communication channel, Telegram (Holzer, Citation2021) is used for discussions and mobilization. Due to an increase in anti-democratic, far-right, conspiratorial views in the movement’s communications (Hunger et al., Citation2021; Nachtwey et al., Citation2020), Querdenken is now under observation by the German domestic intelligence agency Bundesamt für Verfassungsschutz (Bundesamt für Verfassungsschutz, Citation2021).
The public communication data of Querdenken on Telegram was obtained through a search for Querdenken Groups and Channels via a three-stage snowball sampling process. Initial queries for Querdenken entities in Telegram’s search engine yielded a seed sample of 168 entities (Groups and Channels). After identifying all sources of forwarded messages and mentions in the messages retrieved in each snowball sampling stage, 395 entities containing the word Querdenken in their name, handle, or self-description were identified. This procedure for Group and Channel discovery on Telegram is consistent with the existing literature on computational analyses of Telegram data (Baumgartner et al., Citation2020; Peeters & Willaert, Citation2022; Urman et al., Citation2022). In this study, a set of 25 Groups and 25 Channels with the highest member/subscriber counts was identified (see Appendix A.1). Limiting this set to 50 entities ensured that the analysis was focused on the deletion behaviors of communities with large audiences, which served as a marker of relevance. Further, due to heavy posting activity at times, focusing on a small number of entities ensured a timely execution routine of the scraper employed. Then, each of the entities’ chat histories were repeatedly scraped during two time periods of 14 days each: from January 19, 2022, to February 02, 2022, and from February 07, 2022, to February 21, 2022. No major external events that could have influenced posting and deletion behaviors during these time periods were identified. Each scraping iteration had an average time interval of 24.9 minutes, and all messages sent in the five days prior to the scraping iteration for each given entity were archived. Truncating the dataset after nine days allowed for the monitored observation of each post for five days. The messages sent in the aforementioned periods were scraped again in an additional round of data collection on September 16, 2022, seven months after they were posted.
As a result, the dataset consisted of repeatedly scraped chat logs of two nine-day periods, with the (non)deletion status of each message updated for five days in 24.9-minute intervals. Three of the observed Channels and one Group were excluded from the sample because there were no messages posted on them. Further, two Channels and two Groups were unavailable during the later scraping iteration. In total, 29,963 individual messages were recorded from 22 Channels and 24 Groups. The deletion status of the messages in the dataset was evaluated using two different methods: First, messages that were recorded in one scraping iteration but were unavailable in a subsequent iteration were labeled deleted (caught), as their contents and metadata were known. Notably, Telegram’s API assigns unique, consecutive ID numbers to the messages within a given entity, thus identifying each message starting from the first one posted after entity creation. This peculiarity offers researchers insights into the amounts of missing data they are dealing with.
Next, messages that were deleted but not recorded could still be identified, even when they had been sent but deleted between scraping iterations. These were labeled deleted (not caught), and their contents and metadata were unknown. When assessing the time difference between the posting and deletion of messages, the data showed that most messages that were caught prior to deletion were deleted within the first 24 hours after their original appearance (see Appendix A.2). A minority of posts were deleted up to 96 hours after their first appearance. Still, the overall choice of recording the deletion status of messages for up to five days proved reasonable. This was in line with the average message deletion times of posts on Twitter, as reported by Almuhimedi et al. (Citation2013) and Pfeffer et al. (Citation2022).
Methods
Analysis of deleted data types
After determining the extent of message deletion and the data types involved, a bootstrap sampling process was conducted to assess whether the deletion of Telegram messages and their data types differed from random deletions. The total number of deleted (caught) messages and their data types were determined for this analysis. A random sample of the same size was excluded from the full dataset (including both deleted (caught) and nondeleted messages), and the number of missing data types in this sample was computed upon random deletion. This process was iterated 10,000 times for each data type to arrive at the distributions of missing data types in the datasets with random deletion. Comparing the deleted (caught) data against these distributions allowed for judgments to be made on the randomness of purposely deleted messages on Telegram and, subsequently, on how historical Group and Channel chat data might be biased. This procedure was in line with Ho’s (Citation2020) approach to investigating possible biases in data retrieved from Facebook’s API. Ideally, samples would be drawn from all messages sent, both deleted and nondeleted. Since not all deleted messages were caught in the data collection process in this study, the sample only included nondeleted and deleted (caught) messages. Therefore, it cannot be fully treated as sampling from the whole population. It was assumed that the deleted (caught) messages unavailable shortly after posting would share characteristics with deleted (not caught) messages. For this reason, the bootstrap sampling process was repeated after excluding those short-lived deleted (caught) messages as a robustness check.
Top-word analysis
To explore how deleted messages influence the results of automated text analyses of Telegram chat data, the top terms used in the retrieved datasets were analyzed. This was done by comparing the most-used terms of the full dataset to the most-used terms of the dataset without the deleted (caught) messages and, again, to 10,000 datasets with randomly deleted messages. Similar to the procedure proposed by Morstatter et al. (Citation2013) and Ho (Citation2020), Kendall’s (Citation1945) correlation coefficient τ was computed to compare the rankings of the top words in the full dataset to those of the datasets without the deleted (caught) messages. In this statistical approach, the number of concordant pairs (list items with the same relation to each other in both lists, such as item i being ranked higher than item j) was compared with the number of discordant pairs (items that have different relations to each other in both lists). Kendall’s τ was computed as follows:
Here, PC denotes the number of concordant pairs, whereas PD denotes the number of discordant pairs, and TA and TB represent the items that appeared in one list but were missing in the other. After creating a usage ranking of the 38,251 terms observed in the data, τ was calculated, starting with the list of the 10 most-used terms in both datasets. The computation was repeated while increasing the list lengths in steps of 10 until a list of the top 2000 most-used terms was formed (see Appendix A.7 for a list of the most-used terms). Kendall’s τ of the full dataset and the datasets without deleted (caught) messages were then compared to the correlation between the full dataset and 10,000 dataset samples with randomly deleted messages. Deviations in Kendall’s τ of the randomly deleted data from Kendall’s τ of the observed data with nonrandom deletions indicate a bias. The corpus used for this analysis consisted of the text content of all undeleted and deleted (caught) messages, which, following Maier et al. (Citation2018), were stripped of numeric values, special characters, stop words, and URLs. The R package ”quanteda” (Benoit et al., Citation2018) was used to remove German stop words and for stemming.
Reference analysis
Deleted Telegram messages containing references to platform-internal or platform-external sources can bias the results of subsequent reference analyses. To examine the possible biases, Kendall’s τ was also computed percentwise for the top 1–100% lists of referenced hyperlinks (N = 1,319) as well as for the forwarded Telegram-internal sources (N = 1,160) in the full sample, the nondeleted samples, and the set of 10,000 samples with random message deletion. The hyperlinks for this analysis were extracted from the message texts and aggregated at the second-level domain. Exceptions were made for hyperlinks referring to Twitter, Facebook, and YouTube. Links to these major social media platforms were followed and then – if the referred account was accessible – aggregated at the account or page level. For the analysis of actor networks and for snowball sampling, which many Telegram studies rely upon, the distribution of weak ties (Granovetter, Citation1973) that were unavailable because of message deletion were examined. Weak ties are theorized as distant, sporadic connections of nodes in a social network. These edges facilitate and enhance information flow (and, in this case, enable node detection via snowball sampling) because they are able to form bridges between several tight-knit communities (Granovetter, Citation1973; Rajkumar et al., Citation2022). For this, the number of hyperlinks and forwarded entities that were unavailable to scholars after deletion was compared to the number of disappearing weak ties in the set of 10,000 dataset samples with randomly deleted messages.
Topic modeling
As the first step for the analysis of the consequences of the detected text deletion on subsequent computational content analyses, a range of correlated topic models (Blei & Lafferty, Citation2007) was estimated using the R package “stm” (Roberts et al., Citation2019) (see Appendix A.5 for the exact model specification used). While research suggests that the quality of Latent Dirichlet Allocation (LDA) estimation results can be diminished by short document lengths (Hong & Davison, Citation2010), this analysis is used across a number of studies involving Telegram data (Bryanov et al., Citation2022; La Morgia et al., Citation2021; Sear et al., Citation2022). Maier et al. (Citation2020) proposed measuring reliability and reproducibility scores to compare topic models estimated using different corpora. If several topic models estimated from the same corpus achieve a high reliability score, this indicates that the word-topic distributions produced are robust against the model’s probabilistic nature and the noise caused by a subpar corpus. A high reproducibility score is achieved when topic models estimated with different parameter combinations or different corpora produce a similar topic structure – that is, when the word-topic distributions of two topic models resemble each other. In the present study, similar topic model parameter combinations were run for the full corpus, the nondeleted corpus after five days, and the nondeleted corpus after seven months.
To compare two topic models’ results, their word-topic matrices need to be compared to determine whether their estimated topics are similar. According to Niekler and Jähnichen (Citation2012) and Maier et al. (Citation2020), two topics from different models are matched when the cosine similarity of the words with the highest probabilities within the respective topics exceeds a certain threshold. If a topic does not exceed this threshold with any topic from the model it is compared with, it is deemed unmatched. Relying on the configurations reported by Maier et al. (Citation2020), the 20 highest-probability words for each topic in the present study were chosen, and the similarity threshold was set at 0.5.
The reproducibility score of a model describes the share of topics that are matched between topic model estimations stemming from different corpora, thus indicating how well a comparison model can reproduce the topic structure of a base model. In this study, all topic model hyperparameter combinations were run 15 times for each corpus to account for the stochasticity of the correlated topic model estimation. A pairwise comparison between the base models (full corpus) and comparison models (only nondeleted) resulted in reproducibility scores, of which the means and standard deviations are reported in the Results section.
The reliability score describes how well the topic modeling results based on different estimations with the same corpus can be mapped onto each other. Due to the nondeterministic correlated topic modeling process and depending on the corpus, a complete matching of topics from every estimation run is not necessarily attainable. A pairwise comparison of all estimation runs resulted in reliability scores for each of the hyperparameter combinations calculated in the present study.
Computational content analysis
To further determine the aggregate content-wise differences between the deleted and nondeleted text messages, several computational content analysis measures developed for similar corpora and contexts were used. Interpreting the results of these methods was helpful both for the identification of message contents and for determining how the results of such measures might be biased if the deleted (caught) messages are not taken into account during the analysis.
First, a dictionary for the computational analysis of German right-wing populist conspiracy discourse (RPC-Lex), developed and validated by Puschmann et al. (Citation2022), was used to analyze the linguistic features and common tropes of right-wing populism and conspiracy theories by counting how often a social media post mentions the keywords contained in the dictionary. A post was assigned to one of the RPC-Lex categories if both the majority of counted words were from this category and at least three words from the category were found (for a short description of the categories, see Appendix A.10).
Second, the toxicity and severe toxicity levels of nondeleted and deleted posts were assessed via Perspective API (Jigsaw, Citation2022). This API enables antisocial text detection, and while its exact construction is not publicly documented due to its commercial use, Perspective has been used in academic studies in the context of right-wing conspiracy discourse (Hoseini et al., Citation2021; Šipka et al., Citation2022). The analysis in the present study focused on the API’s categories of toxic content, which is “a rude, disrespectful, or unreasonable comment that is likely to make people leave a discussion” (Jigsaw, Citation2022) and severely toxic content, which is “a very hateful, aggressive, disrespectful comment or otherwise very likely to make a user leave a discussion or give up on sharing their perspective” (Jigsaw, Citation2022).
Third, the Distributed Dictionary Representations (DDR) method (Garten et al., Citation2018), a combination of computational dictionaries and word-embedding vectors, was employed to assess the level of populism in deleted and nondeleted messages. The DDR method generates a continuous measure of similarity between a concept and a document by calculating the cosine similarity between the mean word-embedding vector of a concept dictionary and the mean word vector representation of each document in a corpus (Garten et al., Citation2018). This method was implemented using the R package “dictvectoR” (Thiele, Citation2022). The fasttext word-embeddings model used in Thiele’s (Citation2023) study, which was trained on a corpus of 3.5 M Facebook user comments in German language, as well as the dictionary developed and validated for capturing populism using the DDR method in the same study were applied (see Appendix A.12 for the full dictionary).
Results
Deleted data types
Answering the first research question concerning the prevalence of deleted data types in the observed Telegram Groups and Channels required an account of messages that had not been deleted and deleted messages that were caught before deletion.
There were fewer deleted messages in Channels than in Groups, while the share of messages that were deleted before they could be caught in the data collection process was higher in the Channels ().
Figure 1. Scraped messages by deletion status. Messages in dataset by day sent (a) and entity type (b).

The results of differentiating between the types of sharable data can be found in Appendix A.3. Messages themselves were differentiated as organic or forwarded: forwarded messages contain information from other Telegram entities and are used for information distribution, whereas organic messages have information stemming from the message author (although the content might be copied and pasted). The share of forwarded nondeleted messages was higher than the share of organic ones in Channels. Further, the number of forwarded messages that were deleted (caught) were disproportionally higher than the number of organic messages in Channels. There were slightly more nondeleted organic messages in Groups than forwarded ones, but the share of deleted organic messages was drastically higher than the share of deleted forwarded messages. The message counts also showed that messages containing only text dominated the sample of nondeleted messages in Groups, with a share of about 58%, which was nevertheless exceeded by the percentage of deleted (caught) text messages (88% of all deletions). Another conspicuous feature of the sample was the low proportion of deleted (caught) videos and pictures in Group messages compared to their share in nondeleted messages, while both proportions were about equal in Channels.
A high variation of deletion practices was observed across entities (). While the share of nondeleted messages in Groups ranged from 0 to 100%, its mean was found to be considerably lower and its standard deviation higher than those of nondeleted messages in Channels. The percentage and variation of caught and uncaught deleted messages were higher across Groups than Channels. In contrast, the standard deviations of both deleted message types exceeded their means in Channels.
Table 1. Entity-level summary statistics of the recorded messages’ deletion status.
shows the results of the random deletion tests outlined in the Data and Methods section. Each grid cell displays a distribution histogram of the randomly deleted data types.
Figure 2. Histograms of random deletion tests. The histograms show the distribution of the data types of the randomly deleted messages. The red area delineates the confidence interval of two standard deviations from the mean. The blue line marks the number of deleted messages in the sample.

The shaded areas in the plots delineate the interval of two standard deviations from the mean for the randomly deleted samples, indicating that observations of deleted data types in the full sample outside this area were significantly different from random deletions. The first columns of Channel data show that after the early scraping interval, organic messages were not deleted more often in the observed sample than they would if deletions happened at random. When scraped seven months later, the data revealed that a disproportionate amount of forwarded messages was deleted, indicating a bias. Columns three and four show the bias observed in the deleted Group messages: organic messages were deleted significantly more often than random deletion suggests. The same result is shown in the row illustrating the results of text data. The amount of text data deleted in Groups was significantly higher during the study period than random. In contrast, significantly lower numbers of all the other data types analyzed – pictures, text & pictures, videos, audio, and other (containing different kinds of applications)—were deleted in the observed Group sample than in the Group samples with random deletion. With respect to Channels, comparing the sheer quantities of deleted data types to the quantities data in the random deletion sample yielded no significant results, with the notable exception of video data: A significantly higher amount of deleted videos was found in the messages scraped after seven months than in the random deletion samples.
As described in the previous section, a considerable number of messages in Groups and Channels were not caught because they were sent and deleted in between scraping intervals, and thus could not be retrieved. Therefore, robustness checks were run to ensure that the results were not biased by any structural differences between messages that were deleted shortly after sending and messages that were visible for an extended period of time before deletion. Running the same analyses, after excluding all messages that were sent and deleted but caught within one scraping interval and within two scraping intervals, yielded similar results to the analyses above (see Appendix A.4). Consequently, there was no sign of a significant bias in the analyzed sample data stemming from the messages not caught during the collection process.
Most-used words and most-referenced sources
To investigate whether the deleted text messages affected word distribution, Kendall’s τ rank correlation between the most-used words in the different samples was determined and analyzed. The results of this analysis are depicted in . In the sample containing the words posted in Groups, a significantly lower τ was found for the nondeleted data retrieved than for the 10,000 random deletion samples. The correlation between the full sample and the nondeleted sample was lower when fewer top words were considered, implying that the comparative variation in word ranks was the highest among the most-used terms. The correlation graphs converged as the number of top words increased, and τ exceeded 0.75 after 1000 top words were considered (early sample). The nondeleted messages scraped after seven months showed a similar correlation with the full sample for the highest-ranked top words, but the correlation converged slower with the subsequent extension of top words compared. While fewer text deletions were observed in Channels, the τ of the nondeleted messages was lower than that computed for the random deletion samples, with an expectedly lower correlation with the sample scraped later. These results indicated a bias in the word distribution of the dataset after excluding deleted messages, holding true for Groups and Channels alike.
Figure 3. Kendall’s τ of most-used words in the sample. The shaded areas display the confidence interval of two standard deviations for the random deletion samples.

As studies on Telegram communities often rely on snowball sampling and social network analyses, this investigation was also been conducted using information about Channels and Groups from which messages were forwarded. Kendall’s τ was analyzed for the ordered lists of the most-forwarded entities in the sample. The results (see ) showed that in the Group subset of the data, τ was significantly higher in the nondeleted sample than in the random deletion samples for the early scraping period. The messages available seven months after they were posted showed contrary results: τ in this sample was significantly lower than the randomly deleted messages for all percentiles of the list of top-referenced entities. Further, the number of forwarded entities missing from the sample entirely due to message deletion were analyzed. For all Groups, the number of missing entities after intentional deletion did not differ significantly from that of missing entities after the random deletion of messages in the early scraping period. In contrast, in the sample scraped later, the number of missing entities was significantly higher than it would have been if messages were deleted at random. The most-forwarded Telegram entities in the Channel subsample were found to be significantly less correlated with the most-forwarded entities from the full sample for the top 5% of entities. After including more than the top 5% of the most-forwarded entities, no significant difference in τ was seen between the nondeleted and random deletion samples when messages were scraped early. When the messages were scraped later, forwarded messages in Channels showed the same limitations as forwarded messages in Groups: the ordered list of the most forwarded entities had a significantly lower correlation with the list in the full dataset than it would if messages were deleted at random. Again, in the Channel sample scraped later, the number of missing entities was significantly higher than it would be if messages were deleted at random. Based on this, it was determined that a significant bias in forward analyses is unlikely when Telegram messages are scraped shortly after their original posting. If messages are scraped after seven months, methods based on forwarded information might produce biased results.
Figure 4. Analyses of deleted platform-internal references. a) Kendall’s τ of most-referenced entities in the sample: The shaded areas display the confidence interval of two standard deviations from the mean for the random deletion samples. b-c) Histograms of random deletion tests: The histograms show the distribution of referenced entities missing from the data after the random deletion of messages. The red area delineates the confidence interval of two standard deviations from the mean. The blue line marks the number of entities missing from the full dataset after excluding the deleted (caught) messages.

A similar analysis was carried out for hyperlinks – that is, references linking to platform-external sources. The results () showed that in the Group data the τ of the most-referenced hyperlinks in the nondeleted sample was significantly lower than the τ of the random deletion sample for up to the top 10% (60% if scraped later) of the most-referenced hyperlinks. This was caused by a changed order of the top-hyperlink ranking, attributing a greater importance to the more frequently deleted hyperlinks and vice versa. Thus, data on the most-referenced hyperlinks shared within Groups is likely to be biased after intentional message deletion. This inference does not apply to messages retrieved from Channels, as the overall deletion count of text messages was found to be lower. An investigation of the hyperlinks completely missing from the nondeleted sample of Groups showed no significant difference from the count of missing hyperlinks in the random deletion samples. Therefore, the bias introduced by message deletion appears to be found mainly in the most-used hyperlinks, leaving the lesser-used hyperlinks unbiased. The number of hyperlinks missing in the nondeleted sample of Channels was not significantly different from the number of hyperlinks in the random deletion samples in both the early and later scraping periods.
Figure 5. Analyses of deleted platform-external references. a) Kendall’s τ of most-referenced second-level domains in the sample: The shaded areas display the confidence interval of two standard deviations from the mean for the random deletion samples. b-c) Histograms of random deletion tests: The histograms show the distribution of referenced second-level domains missing from the data after the random deletion of messages. The red area delineates the confidence interval of two standard deviations from the mean. The blue line marks the number of domains missing from the full dataset after excluding the deleted (caught) messages.

Computational content analysis results
Comparing the different topic quality measures for the correlated topic model estimations showed that the topic models based on the full corpus of nondeleted and deleted (caught) messages outperformed the models based on early or late nondeleted messages. Employing the topic replicability and reliability measure introduced by Niekler and Jähnichen (Citation2012) and Maier et al. (Citation2020) revealed that word-topic distributions estimated from the reduced corpora reproduced the word-topic distributions of the full corpus to a lesser extent than multiple topic modeling runs on the full corpus itself (). This means, for instance, that in the case of topic model estimations containing ten topics, on average only eight topics estimated from the not deleted (early) corpus were similar to the topics estimated from the full corpus. While Maier et al. (Citation2020) showed that corpus pruning and sampling do not necessarily lead to a reduction in topic replicability, the present study’s result implies that message deletion in this sample indeed led to biases in the topic modeling results. This notion was further supported when the reliability scores of multiple topic modeling runs with the same corpus and parameter combinations were inspected: for the reduced corpora, the internal reliability of the topic models was at the same level as that of the full corpus topic model. Further, the mean word-topic exclusivity and mean semantic coherence (Roberts et al., Citation2019) were compared across model specifications (see Appendix A.6). The results, again, showed that the topics estimated from the full corpus had higher semantic coherence and word-topic exclusivity than the topics computed from nondeleted messages alone.
Figure 6. a) Replicability scores for correlated topic models estimated across different corpora and topic number specifications with full corpus topic estimations as reference; the bar showing the full corpus replicability denotes the reliability score as a reference. Bars denote mean replicability scores and standard deviations. b) Reliability scores for correlated topic models estimated from the same corpora and topic number specifications. Asterisks denote the level of significance resulting from a Mann – Whitney test for differences in sample distributions: ***p < 0.001; **p < 0.01; *p < 0.05.

In the analyses employed to determine content-wise differences between deleted and nondeleted messages, specifically the toxicity analysis using Perspective API (Jigsaw, Citation2022) and the populism analysis employing the DDR approach (Garten et al., Citation2018), no meaningful differences between the message types were detected (see Appendix A.11 and A.13). This result contradicts earlier findings on the deletion of contentious messages, which are likely specific to Telegram as a platform and right-wing conspiracy theorist groups as a counterpublic. Consequently, such computational analysis methods are not likely to be biased as a result of message deletion.
The computational dictionary analysis for the categorization of German online right-wing populist conspiracy theory discourse led to more nuanced results (). Across Channels and Groups alike, the share of anti-immigration messages was found to be higher among deleted messages than among nondeleted messages. Anti-elitist content was more prevalent in nondeleted messages. Channels differed from Groups in having a higher prevalence of nationalist and conspiracist content in deleted messages than in nondeleted ones. Thus, applying this computational dictionary method only to the nondeleted messages would bias the results and any conclusions derived from it. The changing relations between the sample of available messages after five days (early) and after seven months (later) were indicative of dynamic content deletion behavior over time; for example, anti-immigration content in Groups seemed to have been deleted early on, as the overall share of such content was less pronounced later.
Discussion and Conclusion
This paper investigated the biases introduced by message deletion in Telegram chat data. Apart from being a private instant messenger service, Telegram is an attractive communication tool for political actors due to technical features such as Channels for broadcasting and Groups for public discussion (Conway et al., Citation2019; Rogers, Citation2020; Schulze, Citation2020; Wijermars & Lokot, Citation2022). Research has shown that messages on social media are deleted for various reasons, ranging from embarrassment (Wang et al., Citation2011) to government censorship (Fu et al., Citation2013). Messages containing contested political messages are also more likely to be deleted (Bastos, Citation2021; Walker, Citation2017), either because of content quality or the strategic dimension of message deletion (Daffalla et al., Citation2021; Neumayer & Stald, Citation2014). To identify the biases that may arise in public Telegram message data due to deletion practices, as well as the consequences for further computational social science methods, a dataset was collected containing Telegram messages that were deleted later on.
To answer RQ 1a, first, the overall prevalence of deleted messages in the sampled data was determined, differentiating between Channels and Groups. Fewer messages were deleted in Channels than in Groups. This could be because in Channels, only the administrators can write and delete messages. In contrast, every member and administrator of a Group has the right to write and delete messages, which results in a higher number of retrieved messages in Groups as well as a higher share of deleted messages. It could not be inferred from the study data whether the message deletions in Groups stemmed from individual deletion choices, the administrators deleted posts because they violated the Group’s code of conduct, or some messages were automatically deleted messages by chatbots. Many messages were deleted within 30 minutes after posting, and not all of them were caught during the data collection process. Still, a longer period of time between the original post and data collection process increased the number of missing posts in the dataset, especially among the messages scraped from Groups, which answers RQ 1b.
Comparing the distribution of deleted messages across data and entity types (RQ 2a) painted a clear picture of which data types were deleted selectively. Group messages containing texts were found to be deleted significantly more than random deletion would suggest. No negative bias in visual, audio or other data coverage was found. While this implies that these data types had not been deleted disproportionally in general, it remains possible that specific contents encoded in those data types were deleted tactically. As shown in the following analyses, computational content analyses based on text data might be biased as a result. Because of the resulting imbalances in the data types retrieved, multimedia analyses are also at risk of over-representing visual data. With respect to RQ 2b, the disproportionate absence of data types after message deletion stayed stable over time, except for forwarded messages and video data sent to Channels; these were, to a large extent, unavailable after seven months.
Measuring the word distribution of the collected corpora (RQ 3a) revealed post deletion biases for Channels and Groups, with a larger top-word distribution change in the latter. With the larger time interval between message creation and data collection, the difference in distribution became more severe (RQ 3b). It was found that text message deletion impairs subsequent topic modeling and leads to biases in computational content analysis results. While these biases do not result in an outright discouragement of using such methods, they imply that researchers need to be aware of the possibly reduced quality of their models, rendering it worthwhile to consider the amount of deleted content in their dataset when interpreting their results. This answers RQ 3b and is applicable to both data collection intervals.
To answer RQs 4a and 4b, Telegram-internal forwarded messages were examined to determine whether message deletion introduces biases to the data concerning the most-referenced Telegram entities. In the case of Channels, this could not be rejected. The possible biases to network analyses induced by message deletion were found likely to increase with an increase in the time span between the original posting of a message and its collection. This bias is also likely to increase over time in Groups. As snowball sampling is the dominant procedure of Telegram entity detection in the literature and weak ties provide important functions in social networks, the number of references removed from the sample due to message ephemerality was examined. It was found that the number of references leaving the sample because of message deletions differs from that of random deletions, which is likely to bias social network analyses or snowball sampling if the messages are not scraped shortly after their original posting. Also, snowball sampling procedures employing relevance criteria, to determine which Telegram entities to include in the sample, are potentially biased by the changing order of most-referenced entities. Procedures favoring the inclusion of Telegram entities that have the most references (Urman et al., Citation2021) are likely to produce varying outcomes depending on the time of data collection.
The same analysis was conducted on the hyperlinks extracted from the dataset. The results showed that the order of the most-referenced hyperlinks in the subset of Group messages was significantly biased. However, it converged to the random deletion samples’ τ after considering more hyperlinks. This observation, too, points to potentially biased outcomes of cross-platform analyses relying purely on hyperlink counts. This observation was not applicable to the Channel subsample. The reason for this can be attributed to the data type of hyperlink messages, which are considered text messages and more likely to be deleted in Groups than in Channels. This further hints at a variation in the deletion behavior of Channel administrators and Group members that cannot be fully explained without information on the initiator of a deletion. Unless Groups are continuously scraped to counter message ephemerality, a consequence for scholars who wish to explore hyperlink counts in Telegram Groups is to not overemphasize the exact order of the most-shared hyperlinks. Since the detection of weak cross-platform ties is not systematically distorted by message deletions, a bias is not to be expected when analyzing the least-shared hyperlinks.
The differing findings for Channels and Groups across all analyses in this study suggest that researchers dealing with Telegram data should pay attention to the technical and affordance differences between the two forms of public communication because they are likely to have differing biases and limitations. The diverging degrees of ephemerality across entity types is possibly due to a combination of more spontaneous writing in group chat environments, followed by regretful or strategic deletion and moderation by Group administrators, who delete messages which violate their code of conduct. Still, biases introduced by message deletion are partly evident in Channels, too. Only the administrators can write and delete, and this environment possibly encourages more thoughtful posts. Further, biases may occur because in a setting of networked movement formation, Telegram Channels facilitate top – down information distribution and movement and identity-building functions, for which tactical posting and deletion decisions differ from the ones of individual users in chat groups. Therefore, deleted messages in Channels point to either tactical reasons for deletion or personal ones. Thorough analyses of the motivations and causes of message deletion in counter-hegemonic political and right-wing conspiratorial chat groups need to be conducted.
A limitation of this study is the Telegram entities from which the data was collected. While Telegram’s affordances appear attractive for the far-right and conspiracy theorists in the Global North, context factors, especially German ones, cannot be ruled out. In addition, strategic purges of whole social media post histories, potentially biasing scraped Telegram data in different ways, have been reported in the literature (Neumayer & Stald, Citation2014; Ringel & Davidson, Citation2022), although this was not observed in the present study’s dataset. While all the observed Groups and Channels are part of the same movement, deletion practices vary decisively between entities (see ). Therefore, the generalizability of this study’s analyses is likely.
Following the suggestions of Bachl (Citation2018), the need for a data-collection plan by researchers, including timely and regular collection intervals, was highlighted in this study. Generally, researchers studying a phenomenon using Telegram data have no choice but to rely on the historical chat data available in Channels and Groups, with little to no possibility of employing a data collection plan that includes regular, timely scraping. In this situation, the present study enables scholars working with Telegram data to reflect on the possible limitations of their research because the amount of missing data can easily be inferred from the consecutively numbered message IDs in their sample. Further, a collaborative practice of privacy-sensitive data sharing among scholars using Telegram data can support the imputation of missing data and increase the overall reliability and replicability of Telegram studies.
Acknowledgments
The author expresses gratitude to the associate editor, Dr. Marko Bachl, and the three anonymous reviewers for the thorough and constructive review process.
Disclosure statement
No potential conflict of interest was reported by the author.
Additional information
Funding
Notes on contributors
Kilian Buehling
Kilian Buehling is a doctoral researcher in the research group Digitalization and the Transnational Public Sphere at the Weizenbaum Institute for the Networked Society, Berlin, and the Institute for Media and Communication Studies, Freie Universität Berlin. His previous work contains research in information science, quantitative innovation economics and scientometrics.
Notes
1 Public chat groups on Telegram are referred to as Groups and public channels are called Channels from hereon.
2 The online appendix, pre-processed message metadata and replication code for this study are available via OSF: https://osf.io/b7x3p/.
References
- Akbari, A., & Gabdulhakov, R. (2019). Platform surveillance and resistance in Iran and Russia: The case of Telegram. Surveillance & Society, 17(1/2), 223–231. https://doi.org/10.24908/ss.v17i1/2.12928
- Almuhimedi, H., Wilson, S., Liu, B., Sadeh, N., & Acquisti, A. (2013). Tweets are forever: A large-scale quantitative analysis of deleted tweets. Proceedings of the 2013 Conference on Computer Supported Cooperative Work, 897–908. https://doi.org/10.1145/2441776.2441878
- Amarasingam, A., Maher, S., & Winter, C. (2021). How Telegram disruption impacts Jihadist platform migration. Centre for Research and Evidence on Security Threats.
- Bachl, M. (2018). An evaluation of retrospective Facebook content collection. In Dynamische Prozesse der öffentlichen Kommunikation: Methodische Herausforderungen (pp. 57–72). Halem. Preprint. https://doi.org/10.31219/osf.io/6txge
- Bamman, D., O’connor, B., & Smith, N. (2012). Censorship and deletion practices in Chinese social media. First Monday, https://doi.org/10.5210/fm.v17i3.3943
- Bastos, M. (2021). This account doesn’t exist: Tweet decay and the politics of deletion in the brexit debate. The American Behavioral Scientist, 65(5), 757–773. https://doi.org/10.1177/0002764221989772
- Baumgartner, J., Zannettou, S., Squire, M., & Blackburn, J. (2020). The pushshift Telegram dataset. Proceedings of the International AAAI Conference on Web and Social Media, Atlanta, GA, USA, 14, 840–847. https://doi.org/10.1609/icwsm.v14i1.7348
- Bennett, W. L., & Segerberg, A. (2012). The logic of connective action. Information, Communication & Society, 15(5), 739–768. https://doi.org/10.1080/1369118X.2012.670661
- Benoit, K., Watanabe, K., Wang, H., Nulty, P., Obeng, A., Müller, S., & Matsuo, A. (2018). quanteda: An R package for the quantitative analysis of textual data. Journal of Open Source Software, 3(30), 30. https://doi.org/10.21105/joss.00774
- Blei, D. M., & Lafferty, J. D. (2007). A correlated topic model of Science. The Annals of Applied Statistics, 1(1), 1. https://doi.org/10.1214/07-AOAS114
- Bovet, A., & Grindrod, P. (2020). The activity of the far right on Telegram v2.11. https://doi.org/10.13140/RG.2.2.16700.05764
- Bruns, A. (2008). Life beyond the public sphere: Towards a networked model for political deliberation. Information Polity, 13(1–2), 71–85. https://doi.org/10.3233/IP-2008-0141
- Bryanov, K., Vasina, D., Pankova, Y., & Pakholkov, V. (2022). The other side of deplatforming: Right-wing Telegram in the wake of trump’s Twitter ouster. In D. A. Alexandrov, A. V. Boukhanovsky, A. V. Chugunov, Y. Kabanov, O. Koltsova, I. Musabirov, & S. Pashakhin (Eds.), Digital transformation and global society (pp. 417–428). Springer International Publishing. https://doi.org/10.1007/978-3-030-93715-7_30
- Bundesamt für Verfassungsschutz. (2021). Neuer Phänomenbereich “Verfassungsschutzrelevante Delegitimierung des Staates” (Accessed: April 04 2022). https://web.archive.org/web/20220318174618/https://www.verfassungsschutz.de/SharedDocs/kurzmeldungen/DE/2021/2021-04-29-querdenker.html
- Bykov, I. A., Medvedeva, M. V., & Hradziushka, A. A. (2021). Anonymous communication strategy in Telegram: Toward comparative analysis of Russia and Belarus. Communication Strategies in Digital Society Seminar (ComSds), 14–17. https://doi.org/10.1109/ComSDS52473.2021.9422858
- Chen, K., Kim, S. J., Gao, Q., & Raschka, S. (2022). Visual framing of science conspiracy videos. Computational Communication Research, 4(1), 1. https://doi.org/10.5117/CCR2022.1.003.CHEN
- Collier, K., Schecter, A., & Kaplan, E. (2021, January 14). Telegram, a recent haven for the far right, purges extremist content. NBC News, https://web.archive.org/web/20220326070323/
- Conway, M., Khawaja, M., Lakhani, S., Reffin, J., Robertson, A., & Weir, D. (2019). Disrupting daesh: Measuring takedown of online terrorist material and its impacts. Studies in Conflict & Terrorism, 42(1–2), 141–160. https://doi.org/10.1080/1057610X.2018.1513984
- Daffalla, A., Simko, L., Kohno, T., & Bardas, A. G. (2021). Defensive technology use by political activists during the Sudanese revolution. 2021 IEEE Symposium on Security and Privacy (SP), 372–390. https://doi.org/10.1109/SP40001.2021.00055
- Dahlgren, P. (2005). The internet, public spheres, and political communication: Dispersion and deliberation. Political Communication, 22(2), 147–162. https://doi.org/10.1080/10584600590933160
- Ermoshina, K., & Musiani, F. (2021). The Telegram ban: How censorship “made in Russia” faces a global internet. First Monday, 26(5). https://doi.org/10.5210/fm.v26i5.11704
- Fang, Z., Dudek, J., & Costas, R. (2020). The stability of Twitter metrics: A study on unavailable Twitter mentions of scientific publications. Journal of the Association for Information Science and Technology, 71(12), 1455–1469. https://doi.org/10.1002/asi.24344
- Fang, Z., Dudek, J., & Costas, R. (2022). Facing the volatility of tweets in altmetric research. Journal of the Association for Information Science and Technology, 73(8), 1192–1195. n/a(n/a). https://doi.org/10.1002/asi.24624
- Frischlich, L., Schatto-Eckrodt, T., & Völker, J. (2022). Withdrawal to the shadows: Dark social media as opportunity structures for extremism. CoRE NRW Forschungspapier, 3, 39.
- Fu, K., Chan, C., & Chau, M. (2013). Assessing censorship on microblogs in China: Discriminatory keyword analysis and the real-name registration policy. IEEE Internet Computing, 17(3), 42–50. https://doi.org/10.1109/MIC.2013.28
- Gagrčin, E. (2022). Your social ties, your personal public sphere, your responsibility: How users construe a sense of personal responsibility for intervention against uncivil comments on Facebook. New Media & Society, 146144482211174. https://doi.org/10.1177/14614448221117499
- Garry, A., Walther, S., Rukaya, R., & Mohammed, A. (2021). Qanon conspiracy theory: Examining its evolution and mechanisms of radicalization. Journal for Deradicalization, 26(1).
- Garten, J., Hoover, J., Johnson, K. M., Boghrati, R., Iskiwitch, C., & Dehghani, M. (2018). Dictionaries and distributions: Combining expert knowledge and large scale textual data content analysis: Distributed dictionary representation. Behavior Research Methods, 50(1), 344–361. https://doi.org/10.3758/s13428-017-0875-9
- Gerster, L., Kuchta, R., Hammer, D., & Schwieter, C. (2021). Stützpfeiler Telegram. Wie Rechtsextreme und Verschwörungsideolog:innen auf Telegram ihre Infrastruktur ausbauen. https://www.isdglobal.org/isd-publications/stutzpfeiler-telegram-wie-rechtsextreme-und-verschworungsideologinnen-auf-telegram-ihre-infrastruktur-ausbauen/
- Gill, G. (2021). Fascist cross-pollination of Australian conspiracist Telegram channels. First Monday, 26(12). https://doi.org/10.5210/fm.v26i12.11830
- Gorwa, R. (2019). What is platform governance? Information, Communication & Society, 22(6), 854–871. https://doi.org/10.1080/1369118X.2019.1573914
- Granovetter, M. S. (1973). The strength of weak ties. The American Journal of Sociology, 78(6), 1360–1380. https://doi.org/10.1086/225469
- Guhl, J., & Davey, J. (2020). A safe space to hate: White supremacist mobilisation on Telegram. Institute for Strategic Dialogue, 26. https://www.isdglobal.org/isd-publications/a-safe-space-to-hate-white-supremacist-mobilisation-on-telegram/
- Heft, A., & Buehling, K. (2022). Measuring the diffusion of conspiracy theories in digital information ecologies. Convergence: The International Journal of Research into New Media Technologies, 28(4), 940–961. https://doi.org/10.1177/13548565221091809
- Ho, J.C.-T. (2020). How biased is the sample? reverse engineering the ranking algorithm of Facebook’s Graph application programming interface. Big Data & Society, 7(1), 2053951720905874. https://doi.org/10.1177/2053951720905874
- Holzer, B. (2021). Zwischen Protest und Parodie: Strukturen der “Querdenken”-Kommunikation auf Telegram (und anderswo). Die Misstrauensgemeinschaft der ”Querdenker”. Die Corona-Proteste aus kultur- und sozialwissenschaftlicher Perspektive (pp. 125–157). Frankfurt am Main: Campus Verlag.
- Hong, L., & Davison, B. D. (2010). Empirical study of topic modeling in Twitter. Proceedings of the First Workshop on Social Media Analytics - SOMA’ 10, 80–88. https://doi.org/10.1145/1964858.1964870
- Hoseini, M., Melo, P., Benevenuto, F., Feldmann, A., & Zannettou, S. (2021). On the globalization of the qanon conspiracy theory through Telegram. arXiv preprint arXiv:2105.13020.
- Hunger, S., Völker, T., & Gonzatti, D. S. (2021). Der Verlust der Vielfalt: Die Corona-Proteste in Deutschland werden durch eine radikale Minderheit geprägt. WZB-Mitteilungen, 172, 30–32.
- Jalilvand, A., & Neshati, M. (2020). Channel retrieval: Finding relevant broadcasters on Telegram. Social Network Analysis and Mining, 10(1), 23. https://doi.org/10.1007/s13278-020-0629-z
- Jigsaw. (2022). Perspective API - Attributes and Languages. https://developers.perspectiveapi.com/s/about-the-api-attributes-and-languages
- Kaiser, J., & Rauchfleisch, A. (2019). Integrating concepts of counterpublics into generalised public sphere frameworks: Contemporary transformations in radical forms. Javnost - the Public, 26(3), 3. https://doi.org/10.1080/13183222.2018.1558676
- Kargar, S., & Mcmanamen, k. (2018). censorship and collateral damage: Analyzing the Telegram ban in Iran (SSRN Scholarly Paper ID3244046). Social Science Research Network. https://doi.org/10.2139/ssrn.3244046
- Kendall, M. G. (1945). The treatment of ties in ranking problems. Biometrika, 33(3), 239–251. https://doi.org/10.1093/biomet/33.3.239
- King, G., Pan, J., & Roberts, M. E. (2014). Reverse-engineering censorship in China: Randomized experimentation and participant observation. Science, 345(6199), 1251722. https://doi.org/10.1126/science.1251722
- Kurdi, M., Albadi, N., & Mishra, S. (2020). “Video unavailable”: Analysis and prediction of deleted and moderated youtube videos. 2020 IEEE/ACM International Conference on Advances in Social Networks Analysis and Mining (ASONAM), 166–173. https://doi.org/10.1109/ASONAM49781.2020.9381310
- La Morgia, M., Mei, A., Mongardini, A. M., & Wu, J. (2021). uncovering the dark side of telegram: Fakes, clones, scams, and conspiracy movements. (arXiv:211113530) arXiv, https://doi.org/10.48550/arXiv.2111.13530
- Little, R. J. A., & Schenker, N. (1995). Missing Data. In G. Arminger, C. C. Clogg, & M. E. Sobel (Eds.), Handbook of statistical modeling for the social and behavioral sciences (pp. 39–75). Springer US. https://doi.org/10.1007/978-1-4899-1292-3_2
- Loucaides, D., Perrone, A., & Holnburger, J. (2021, March 31). How Germany became ground zero for the COVID infodemic. OpenDemocracy. https://www.opendemocracy.net/en/germany-ground-zero-covid-infodemic-russia-far-right/
- Macklin, G. (2022). The extreme right, climate change and terrorism. Terrorism and Political Violence, 34(5), 979–996. https://doi.org/10.1080/09546553.2022.2069928
- Maier, D., Niekler, A., Wiedemann, G., & Stoltenberg, D. (2020). How document sampling and vocabulary pruning affect the results of topic models. Computational Communication Research, 2(2), 2. https://doi.org/10.5117/CCR2020.2.001.MAIE
- Maier, D., Waldherr, A., Miltner, P., Wiedemann, G., Niekler, A., Keinert, A., Pfetsch, B., Heyer, G., Reber, U., Häussler, T., Schmid-Petri, H., & Adam, S. (2018). Applying LDA topic modeling in communication research: Toward a valid and reliable methodology. Communication Methods and Measures, 12(2–3), 93–118. https://doi.org/10.1080/19312458.2018.1430754
- Maréchal, N. (2018). From Russia with crypto: A political history of Telegram. 8th {USENIX} Workshop on Free and Open Communications on the Internet ({FOCI} 18), Baltimore, MD, USA.
- Marwick, A. E., & Boyd, D. (2014). Networked privacy: How teenagers negotiate context in social media. New Media & Society, 16(7), 1051–1067. https://doi.org/10.1177/1461444814543995
- McSwiney, J., Vaughan, M., Heft, A., & Hoffmann, M. (2021). Sharing the hate? memes and transnationality in the far right’s digital visual culture. Information, Communication & Society, 24(16), 2502–2521. https://doi.org/10.1080/1369118X.2021.1961006
- Morstatter, F., Pfeffer, J., Liu, H., & Carley, K. (2013). Is the sample good enough? comparing data from Twitter’s streaming API with Twitter’s firehose. Proceedings of the International AAAI Conference on Web and Social Media, Cambridge, MA, USA, 7(1), 400–408. https://doi.org/10.1609/icwsm.v7i1.14401.
- Nachtwey, O., Schäfer, R., & Frei, N. (2020). Politische Soziologie der Corona-Proteste Preprint. SocArXiv. https://doi.org/10.31235/osf.io/zyp3f
- Neubaum, G., & Weeks, B. (2022). Computer-mediated political expression: A conceptual framework of technological affordances and individual tradeoffs. Journal of Information Technology & Politics, 0(0). https://doi.org/10.1080/19331681.2022.2028694
- Neumayer, C., & Stald, G. (2014). The mobile phone in street protest: Texting, tweeting, tracking, and tracing. Mobile Media & Communication, 2(2), 117–133. https://doi.org/10.1177/2050157913513255
- Niekler, A., & Jähnichen, P. (2012). Matching results of latent Dirichlet allocation for text. Proceedings of the 11th International Conference on Cognitive Modeling, Berlin, Germany. N. Rußwinkel, U. Drewitz & H. van Rijn (eds.), 317–322. Berlin: Universitaetsverlag der TU Berlin.
- Nobari, A. D., Reshadatmand, N., & Neshati, M. (2017). Analysis of Telegram, an instant messaging service. Proceedings of the 2017 ACM on Conference on Information and Knowledge Management, 2035–2038. https://doi.org/10.1145/3132847.3133132
- Peeters, S., & Willaert, T. (2022). Telegram and digital methods: Mapping networked conspiracy theories through platform affordances. M/C Journal, 25(1), 1. https://doi.org/10.5204/mcj.2878
- Pfeffer, J., Mooseder, A., Hammer, L., Stritzel, O., & Garcia, D. (2022). This sample seems to be good enough! assessing coverage and temporal reliability of Twitter’s academic API. ArXiv: 2204 02290 [Cs], http://arxiv.org/abs/2204.02290
- Puschmann, C., Karakurt, H., Amlinger, C., Gess, N., & Nachtwey, O. (2022). RPC-lex: A dictionary to measure German right-wing populist conspiracy discourse online. Convergence: The International Journal of Research into New Media Technologies, 28(4), 1144–1171. https://doi.org/10.1177/13548565221109440
- Rajkumar, K., Saint-Jacques, G., Bojinov, I., Brynjolfsson, E., & Aral, S. (2022). A causal test of the strength of weak ties. Science, 377(6612), 1304–1310. https://doi.org/10.1126/science.abl4476
- Ringel, S., & Davidson, R. (2022). Proactive ephemerality: How journalists use automated and manual tweet deletion to minimize risk and its consequences for social media as a public archive. New Media & Society, 24(5), 1216–1233. https://doi.org/10.1177/1461444820972389
- Roberts, M. E., Stewart, B. M., & Tingley, D. (2019). Stm: An R package for structural topic models. Journal of Statistical Software, 91(1), 1. https://doi.org/10.18637/jss.v091.i02
- Rogers, R. (2020). Deplatforming: Following extreme internet celebrities to Telegram and alternative social media. European Journal of Communication, 35(3), 213–229. https://doi.org/10.1177/0267323120922066
- Schatto-Eckrodt, T. (2022). Hidden biases – the effects of unavailable content on Twitter on sampling quality. In J. Jünger, U. Gochermann, C. Peter & M. Bachl (eds.), Grenzen, Probleme und Lösungen bei der Stichprobenziehung (pp. 178–195). Herbert von Halem Verlag.
- Scheffler, T., Solopova, V., & Popa-Wyatt, M. (2021). The Telegram chronicles of online harm. Journal of Open Humanities Data, 7(0), 0. https://doi.org/10.5334/johd.31
- Schulze, H. (2020). Zur Bedeutung von Dark Social & Deplatforming. Eine quantitative Exploration der deutschsprachigen Rechtsaußenszene auf Telegram. [Dark social & deplatforming. A quantitative exploration of the German-speaking far-right scene on Telegram.]. Zeitschrift Für Semiotik, 42(3–4), 61–86.
- Schulze, H., Hohner, J., Greipl, S., Girgnhuber, M., Desta, I. & Rieger, D. (2022). Far-right conspiracy groups on fringe platforms: a longitudinal analysis of radicalization dynamics on Telegram. Convergence: The International Journal of Research into New Media Technologies, 28(4), 1103–1126. https://doi.org/10.1177/13548565221104977
- Sear, R., Restrepo, N. J., Lupu, Y., & Johnson, N. F. (2022). Dynamic topic modeling reveals variations in online hate narratives. In K. Arai (Ed.), Intelligent Computing (pp. 564–578). Springer International Publishing. https://doi.org/10.1007/978-3-031-10464-0_38
- Šipka, A., Hannák, A., & Urman, A. (2022). Comparing the language of qanon-related content on parler, gab, and Twitter. 14th ACM Web Science Conference 2022, 411–421. https://doi.org/10.1145/3501247.3531550
- Sosa, J., & Sharoff, S. (2022). Multimodal pipeline for collection of misinformation data from Telegram. (arXiv: 220412690) arXiv, https://doi.org/10.48550/arXiv.2204.12690
- Telegram. (2022, April 2). Terms of Service. https://web.archive.org/web/20220402050604/https://telegram.org/tos
- Thiele, D. (2022). dictvectoR (version v0.0.1). [Computer software]. Zenodo. https://doi.org/10.5281/zenodo.7079600
- Thiele, D. (2023). Contagious comments? How the pandemic has affected populist user comments in seven Western-European countries (in press). Computational Communication Research.
- Urman, A., Ho, J. C., & Katz, S. (2021). Analyzing protest mobilization on Telegram: The case of 2019 anti-extradition bill movement in Hong Kong. Plos One, 16(10), e0256675. https://doi.org/10.1371/journal.pone.0256675
- Urman, A., & Katz, S. (2022). What they do in the shadows: Examining the far-right networks on Telegram. Information, Communication & Society, 25(7), 904–923. https://doi.org/10.1080/1369118X.2020.1803946
- Urman, A., & Katz, S. (2022). Online publicity and outcomes of individual politically salient criminal cases in an authoritarian regime: Evidence from Russia. Political Research Exchange, 4(1), 2095920. https://doi.org/10.1080/2474736X.2022.2095920
- van Atteveldt, W., & Peng, T.-Q. (2018). When communication meets computation: opportunities, challenges, and pitfalls in computational communication science. Communication Methods and Measures, 12(2–3), 81–92. https://doi.org/10.1080/19312458.2018.1458084
- Volkova, S., & Bell, E. (2017). Identifying effective signals to predict deleted and suspended accounts on Twitter across languages. Proceedings of the International AAAI Conference on Web and Social Media, Montreal, Canada, 11(1), 290–298.
- Walker, S. (2017). The Complexity of Collecting Digital and Social Media Data in Ephemeral Contexts [ Thesis]. https://digital.lib.washington.edu:443/researchworks/handle/1773/40612
- Wang, Y., Norcie, G., Komanduri, S., Acquisti, A., Leon, P. G., & Cranor, L. F. (2011). “I regretted the minute I pressed share”: A qualitative study of regrets on Facebook. Proceedings of the Seventh Symposium on Usable Privacy and Security, 1–16. https://doi.org/10.1145/2078827.2078841
- Wijermars, M., & Lokot, T. (2022). Is Telegram a “harbinger of freedom”? the performance, practices, and perception of platforms as political actors in authoritarian states. Post-Soviet Affairs, 38(1–2), 1–21. https://doi.org/10.1080/1060586X.2022.2030645
- Xu, J.-M., Burchfiel, B., Zhu, X., & Bellmore, A. (2013). An examination of regret in bullying Tweets. Proceedings of the 2013 Conference of the North American Chapter of the Association for Computational Linguistics: Human Language Technologies, 697–702. https://aclanthology.org/N13-1082
- Zannettou, S., Caulfield, T., Blackburn, J., De Cristofaro, E., Sirivianos, M., Stringhini, G., & Suarez-Tangil, G. (2018). On the origins of memes by means of fringe Web communities. Proceedings of the Internet Measurement Conference 2018, 188–202. https://doi.org/10.1145/3278532.3278550
- Zehring, M. & Domahidi, E. (2023). German Corona Protest Mobilizers on Telegram and Their Relations to the Far Right: A Network and Topic Analysis. Social Media + Society, 9(1). https://doi.org/10.1177/20563051231155106