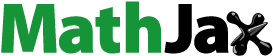
ABSTRACT
Misfolding of the prion protein is central to prion disease aetiology. Although understanding the dynamics of the native fold helps to decipher the conformational conversion mechanism, a complete depiction of distal but coupled prion protein sites common across species is lacking. To fill this gap, we used normal mode analysis and network analysis to examine a collection of prion protein structures deposited on the protein data bank. Our study identified a core of conserved residues that sustains the connectivity across the C-terminus of the prion protein. We propose how a well-characterized pharmacological chaperone may stabilize the fold. Also, we provide insight into the effect on the native fold of initial misfolding pathways identified by others using kinetics studies.
Introduction
The conformational conversion of the prion protein into the aggregation prone isoform is the major event in prion aggregate formation. The templated misfolding process involves recognition between PrPSc (that is, the misfolded form of the prion protein) and the physiological form of the prion protein, followed by prion protein conformational re-arrangement. Thus, rational anti-prion drug design could target the prion fibril, the conformational conversion event, or the natively folded PrPC. Recently resolved three-dimensional (3D) structures of prion fibrils [Citation1] exhibit a shared PIRIBS quaternary structure [Citation2–7] or a 4-rung β [Citation8] topology. In all of these, there are distinctions in the backbone geometry and network of side chain interactions. Therefore, although prion fibril structure is appealing for rational drug design, specificity is challenging. Targeting the conformational conversion event requires mechanistic understanding of the misfolded conformations of the prion protein [Citation9]. Significant advances indicate that pharmacological chaperones bind unfolded prion protein [Citation10]. However, the question remains on how the chaperone molecule would interact with the presumably conformationally rich pool of unfolded prion protein conformations. A more promising alternative in structure-based drug design focuses on the native PrPC [Citation11]. While the 3D structure of the intrinsically disordered N-terminus has yet to be resolved, the 3D structure of the C-terminus of PrPC has proven well tested in a number of mammal species using nuclear magnetic resonance (NMR) and x-ray crystallography techniques. Evidence points at the C-terminus of PrPC as a candidate for structure-based drug design: firstly, the main component of the resolved structures is the misfolded C-terminus of the prion protein [Citation1]. Secondly, residue substitutions linked to differentiated aggregation propensity are mainly found in the C-terminus of the protein [Citation12]. And thirdly, recently proposed therapeutics are known to recognize the first α-helix of the C-terminus [Citation13].
A blueprint of the structural dynamics of the C-terminus of the prion protein speeds up the identification of molecular targets to stabilize the native fold. Local conformational effects resulting from sequence differences have been investigated [Citation14,Citation15]. However, no consensus picture exists yet of the commonalities of the structural dynamics of the native fold across species. Our study aims to test the hypothesis that the structural dynamics of mammalian prion proteins converge to a connectivity pattern that explains subdomains coupling. Determining the long-range connectivity of the protein conformation depicts the structural patterns that stabilize the fold and identifies sites to be used as targets for pharmacological chaperones action. In addition, understanding the dynamics of the protein topology will shed light on how initial misfolding may impact residue connectivity and how the breaking of that connectivity may shape the misfolding pathway.
For our study, we selected NMR-derived 3D structures of the prion protein from mammal species with distinct susceptibility to prion diseases. We aim to capture structural dynamics information from the NMR conformers. We excluded protein structures derived from x-ray crystallography from our study because of the intrinsic static nature of the resolved structures. To examine the connectivity patterns of the C-terminus of PrPC, we used normal mode analysis (NMA) to model all conformers. NMA captures efficiently the large amplitude (that is, slow frequency) and collective motions within thermodynamic fluctuations of the folded protein structure [Citation16,Citation17]. For the purpose of mapping the intrinsic dynamics of the protein, our workflow has the following advantages: NMA is not computationally demanding, uses a small number of input parameters, and allows us to include in our analysis a number of experimentally determined protein structures. This approach has proven to enhance understanding of protein structure dynamics [Citation18,Citation19].
Our analysis of distal and coupled protein sites identified a core of conserved residues across species that sustain the residue interaction network: four hydrophobic residues from β-sheet 2, α-helix 2, and α-helix 3. Interestingly, α-helix 2 and α-helix 3 have residues that belong to distinct coupled subdomains. We illustrate how the structural dynamics map helps us to understand the stabilizing effect of well-characterized pharmacological chaperones and propose an interpretation of initial misfolding pathways.
Results
Structural dynamics is conserved across species
To investigate structural dynamics similarity, we analysed the profile of the root mean square inner product (rmsip) between any two conformers. The calculation included all non-trivial modes. In other words, the first six modes with an eigenvalue of zero corresponding to overall translations and rotations were discarded (see ; scale is zoomed in the range 0.65 to 1). By convention, a rmsip value greater than 0.6 indicates similarity of the deformation modes [Citation20,Citation21]. The pairwise inter-species structural dynamics similarity ranges from 68% to 95%. The pattern indicates that the large and collective modes of deformation of the native topology across all species are conserved, regardless of the protein sequence similarity (see Supplemental material, Figure S1). Although a pattern might suggest similarity for two groups of prion protein structures (group 1: bankvole, mouse, and wallaby; group 2: bovine, cat, dog, elk, hamster, human, and pig), we are cautious in not overinterpreting our data. The analysis we performed on the following sections does not reflect the apparent pattern suggested for the grouping (see SI Figure S1c). We interpret that rmsip differences reflect thermodynamic fluctuations but not structural dynamics distinctiveness.
α2-α3 loop has the largest relative conformational flexibility in the globularly folded C-terminus of the prion protein
To examine the conformational flexibility of the protein structure, we analysed the Cα atomic position root mean square fluctuation (rmsf) profile. We calculated the rmsf from the normal mode analysis of each NMR conformer. shows the largest backbone conformational flexibility (greatest relative rmsf) in the α2-α3 loop. The peak of the profile shows Lys 194, Gly195, and Glu196 as the residues with the largest flexibility in all structures. The fragment with the second largest set of rmsf values corresponds to the β2-α2 loop. And, the third largest rmsf values correspond to the second half of the β1- α1 loop. In contrast, the α1-α2 loop is the most rigid loop based on the relative rmsf values.
Figure 2. The top bar indicates secondary structure elements of the C-terminus of PrPC: three α-helices and two short β-sheets. Figure 2a shows the root mean square fluctuation of each residue. Figure 2b shows the betweenness centrality of each residue. Figure 2c shows the eigenvector centrality of each residue. In all cases, residue numbering is according to human PrPC.

The rmsf profile of α-helix 1 indicates a slightly greater level of flexibility than the other two helices. That is, the fragments with the least backbone flexibility correspond to α-helix 2 and α-helix 3. Note that unique residue substitutions in the prion protein of species with low susceptibility to prion diseases (Asp159 and His177 in dog PrPC, Ser167 and Lys173 in horse PrPC, Ile203 in pig PrPC, and Ala225 in rabbit PrPC) do not show a salient rmsf value. The resolution of Cα-atom normal mode analysis cannot differentiate local flexibility of the protein backbone due to residue substitutions; the observed flexibility pattern lies within expected fluctuations of the native state. The profile of protein backbone flexibility we found agrees with previous work that investigated conformational flexibility using principal component analysis [Citation14] and NMR studies [Citation22].
The boundary between the two largest dynamic subdomains crosses through the middle of α-helix 2 and α-helix 3
To identify dynamic subdomains in the protein structure, we used the GeoStaS algorithm [Citation23] as implemented in Bio3D [Citation24]. The algorithm identifies protein regions that move as rigid bodies and captures both translational and rotational motions. Our analysis identified two subdomains (see ). The subdomains were calculated from the Cartesian coordinates of each conformer in the NMR ensemble, and then averaged over the ensemble. All protein structures show a very similar pattern: One subdomain includes β-sheet 1, β-sheet 2, β2-α2 loop, the first half of α-helix 2, and the second half of α-helix 3. The other subdomain has β1-α1 loop, α-helix 1, α1-β2 loop, the second half of α-helix 2, α2-α3 loop, and first half of α-helix 3. That is, the boundary plane between the two subdomains crosses through the middle of helices 2 and 3.
Figure 3. Figure 3a shows the two main subdomains identified with the GeoStaS algorithm; each subdomain is displayed with different colour. Figure 3b shows the main correlated couplings (lines in pink colour). Figure 3c shows the main anticorrelated couplings (lines in green colour). The ribbon illustration corresponds to human PrPC.

Dynamic couplings identify distal protein segments that undergo anticorrelated motion
We calculated the Cα-atom position cross-correlation matrix to examine the dynamic couplings in the prion protein topology. shows the dynamic cross-correlation matrix averaged over all the NMR conformers of the C-terminus human PrPC. The colour shade indicates the extent of the correlated motion: strongest correlated motion (i.e. the matrix coefficient has a value of + 1 when fluctuations of the pair of residues exhibit the same period and the same phase) is shown in a dark cyan colour. Strongest anticorrelated motion (i.e. the matrix coefficient has a value of −1 when fluctuations of the pair of residues exhibit the same period and are 180° out of phase) is shown in a dark pink colour. and show a summary of the prominent peaks observed in the matrices (see Supplemental material, ). All structures show correlated motion between the first half of α-helix 2 (residues Val176 through Val184) and the mid-region of α-helix 3 (residue Glu207 through Ile215) (see ). A second peak of correlated motion corresponds to residues adjacent and in β-sheet 1 (residues Tyr128 through Ser135) and adjacent and in β-sheet 2 (residues Pro158 through Arg164). Two additional protein segments show correlated motion: one peak corresponds to β-sheet 2 (residues Val161 through Tyr163) and the first half of α-helix 2 (Asp178 through residue Ile184). The other peak corresponds to β-sheet 2 (residues Val161 through Tyr163) and the mid-region of β-helix 3 (Val209 through Thr216). To a lesser extent, residues in β-sheet 1 and β1-α1 loop are correlated to residues in the mid-region of α-helix 3 (Met213 through Gln217). And, residues in β-sheet 1 are correlated to residues in α-helix 2 (Ile182 and Thr183).
Figure 4. Dynamic cross correlation matrix averaged over the NMR ensemble of human PrPC. Figure 4a illustrates residues with strong correlated motion. Figure 4b illustrates residues with strong anticorrelated motion. Figure 4c and Figure 4d use the same molecular representation to highlight dashed-line ovals in-phase deformations (figure 4c) and out-of-phase deformations (figure 4d). In all cases arrows point at the matrix region that illustrates the coupling between the sets of residues.

Table 1. List of peaks that represent strongest couplings observed in the dynamical cross correlation matrix of each NMR ensemble. Two protein sites were considered to be short distance if at least one pair of Cα-atoms from each site is within 8Å.
Peaks of anticorrelated motion are well defined although the strength of the coupling is weaker than correlated motion peaks. An anticorrelated motion peak shows for α-helix 1 (residues Ser143 through Glu152) and α-helix 3 (residues Met206 through Thr216) (see ). A second anticorrelated motion peak shows between α-helix 2 (residue Asn174 through Thr183) and α-helix 3 (residue Glu221 through Gln227). A third peak shows between residues adjacent to and in β-sheet 2 (residues Asn159 through Tyr163) and α-helix 3 (residue Ser222 through Gln227). A fourth anticorrelated motion peak shows between residues in β-sheet 1 (residue Tyr128 through Leu130) and the α2-α3 loop (residues Thr191 through Glu196). A fifth peak connects two regions of α-helix 3: residue Val209 through Thr216 and residue Ser222 through Gln227). Few more, although less populated, peaks correspond to connectivity between β-sheet 1 and C-terminus of α-helix 3, α-helix 1 and α-helix 2, β-sheet 2 and α2-α3 loop, β2-α2 loop and helices 2 and 3, and α-helix 2 and α2-α3 loop (see ).
The patterns identified in the dynamic cross-correlation matrices are also illustrated in the vector field representation of the slowest modes of deformation of the C-terminus PrPC (see for human PrPC). Each arrow indicates the magnitude and direction of the deformation of each residue. shows green arrows in a mostly parallel orientation illustrating correlated motions between β-sheets and the mid region of α-helix 2 and α-helix 3. shows pairs of arrows pointing at an angle greater than 90°Corresponding to anticorrelated motions that involve mainly α-helix 1 and α2-α3 loop.
A cluster of four hydrophobic residues bridges interactions in the residue interaction network
To examine the residue connectivity, we built an undirected network based on the atomic position cross-correlation matrix (see Methods section). From the network, we calculated the betweenness centrality (see ) and the eigenvector centrality (see ) [Citation25,Citation26]. A residue with high betweenness centrality acts as a bridge that lies on many short paths connecting two residues. A residue with high eigenvector centrality is connected to other highly connected residues. To select key residues based on the centralities, we calculated the z-score. Any residue with a z-score greater than two (that is, a residue whose centrality value is at least two standard deviations greater than the mean) was determined to be crucial for the intrinsic dynamics of the protein (see ) structure [Citation27]
Table 2. Residues that exhibit largest betweenness and eigenvector centrality z-score. A z-score greater than 2 means that the value is more than two standards deviations away from the mean. A locality factor = 5 Å filters out long-range effects by dampening the strength of the dynamic coupling between distant residues.
In all species, Val161 (located in β-sheet 2), Val180 (located in α-helix 2), and Val210 (located in α-helix 3) show high betweenness centrality when all long-range correlations are included (locality factor of λ = 100 Å). In other words, the three valine residues bridge the greatest number of residue interactions.
To filter out long-range effects, we set a locality factor λ = 5 Å that dampens the strength of the dynamic coupling between distant residues [Citation28]. Based on a high z-score of betweenness, Tyr157 (in all but one species), Val161, Thr183, and Val210 (in all but one species) bridge the greatest number of residue communication pathways. Remarkably, Val161, Val180, Val210 show a high z-score of betweenness in the regime of a long locality factor (λ = 100 Å), and a high z-score of eigenvector centrality in the regime of short locality factor (λ = 5 Å). The three valine residues, Tyr157 and Thr183 display low rmsf values and are partially buried inside the protein. The valine residues are tightly connected to the two Cysteine residues that form a disulphide bridge between α-helix 2 and α-helix 3, and Thr183 packs against Val180 and Val210 (see ). Tyrosine 157 locates in the α1-β2 loop and is partially sandwiched between α-helix 1 and α-helix 3. Hence, the high betweenness centrality for Tyr157 indicates bridging interactions between the two helices.
Figure 5. Distal but coupled residues that form the structure core of the protein structure. Molecular representation shown on the globular C-terminus of human PrPC.

To further inspect the connectivity between residues exhibiting high centrality values, we analysed the edge-betweenness communities (based on each NMR ensemble’s average atomic position cross-correlation matrix). All residues that belong to a community are highly intra-connected and loosely connected to residues in other communities. In all species, Val161, Val180, Val210, and Thr183 belong to the same one or two communities. These observations and the pattern of centralities indicate that the four hydrophobic residues form a core cluster key to maintaining the overall protein architecture together.
Pharmacological chaperones play the role of a leash connecting protein segments that exhibit anticorrelated motion
Pharmacological chaperones prevent protein misfolding by stabilizing the native fold. As a proof-of-concept, we examined how binding of a well-characterized pharmacological chaperone could stabilize the C-terminus of PrPC. To this aim, we performed molecular docking calculations similar to those reported by Petrosyan et al [Citation10]. In our calculations, we docked the pentosan polysulfate (PPS) molecule to each NMR conformer of the hamster prion protein. Our analysis indicates that hydrogen bond formation between the oxygen atoms in PPS and highly conserved charged or polar side chains on the surface of PrPC is a driving force for docking. We found three poses for PPS binding (see ), two of which (Pose 1 and Pose 2) were previously found [Citation10] (see ). Interestingly, the three preferential poses we found show binding sites in either α-helix 2 or α-helix 3 to a loop. In Pose 1, PPS forms hydrogen bonds with the C-terminus of α-helix 2 (Thr191 or Lys194) and the C-terminus of the α1-β2 loop. Remarkably, the set of residues that precede the α2-α3 loop are proposed to be an initiation site for misfolding [Citation29,Citation30]. In Pose 2, PPS forms hydrogen bonds with the mid-region of α-helix 2 (Asn181 or Lys185) and the loop prior to β-sheet 1. It remains to be examined in future studies how the glycosylation state of Asn181 may shift the availability of docking sites. In Pose 3, PPS forms hydrogen bonds with α-helix 3 and the β1-α1 loop. In all poses, the two protein sites exhibit soft dynamic coupling with each other. The two sites in Pose 1 and Pose 3 exhibit anticorrelated motion while those in Pose 2 exhibit correlated motion. We propose that PPS acts as a leash that connects two weakly anticorrelatedly coupled sites. The added connectivity pathway may stabilize the globular C-terminus without imposing a major structural strain.
Figure 6. Binding poses of PPS on hamster PrPC and dynamic cross correlation matrix averaged over the NMR ensemble of hamster PrPC. Arrows point at the matrix position that illustrates the coupling between the two protein sites to which PPS binds.

Table 3. Binding poses of the pharmacological chaperone PPS on the C-terminus of PrPC. All binding poses correspond to PPS binding to two sites on the protein. The residues that form hydrogen bonds with PPS are highly conserved across the species analysed.
A question that remains open is how the N-terminus of PrPC may modulate the availability of binding sites on the C-terminus. For example, due to metal ion binding, the disordered N-terminus comes in close contact with His140 or His177 (residue numbering according to human PrPC) [Citation31]. Histidine 140 is nearby residues in the β1-α1 loop involved in Pose 3, and His177 is nearby residues in α-helix 2 involved in Pose 2. The resulting steric hindrance could bias the docking of the PPS molecule in each pose.
Discussion
Our analysis indicates that the structural dynamics of the protein structure is robust against point mutations in non-human mammals. The large and collective motions of the protein three-dimensional structure are insensitive to subtle and local conformational effects that residue substitutions could induce. This follows from the high similarity between the normal modes of all conformers (rmsip values greater than~0.7). In addition, none of the naturally occurring residue substitutions exhibit a salient network centrality value (no z-score is greater than 2). The residue interaction network tolerates the residue substitutions by having evolved such that the mutated residues are not critical in the connectivity pattern of the native protein fold [Citation32].
Mapping the intrinsic structural dynamics of the C-terminus of the prion protein identifies a structural core that governs the conformational dynamics of the native state. To this end, we first identified two large subdomains that move as coherent units. To examine the connectivity within and across such subdomains, we used the dynamic couplings cross-correlation matrix approach. Correlated dynamic couplings are found primarily connecting distal protein segments within Subdomain 1. Anticorrelated dynamic couplings are found either within Subdomain 2 or across subdomains. To help us rationalize the connectivity in the protein structure, we used network theory analysis. From this, we calculated network centralities. Residues that show high network centrality are hydrophobic (Val161, Val180, Val210), regardless of the locality factor. More residues emerge with high network centrality if long-range fluctuations are filtered out. These residues are aromatic (Tyr157), hydroxylic (Thr183), or are involved in the disulphide bridge that connects α-helix 2 and α-helix 3 (Cys179 and Cys214). All these highly conserved residues are located in Subdomain 1 (except Tyr157), close to the boundary between subdomains, exhibit correlated motion, show low conformational flexibility, and are buried in the protein’s interior. Our observations suggest that residues with high network centrality form a structural core in the C-terminus of the prion protein, consistent with the helical core detected using NMR studies [Citation22]. The structural core ensures cohesion and connectivity across the network of residue interactions.
Blueprint of residue connectivity helps to conceptualize initial misfolding
Previous kinetics-based studies propose that the α2-α3 loop is an initiation site for spontaneous misfolding of the prion protein [Citation30]. As a result of perturbing the α2-α3 loop, Sengupta et al. observed three distinct steps: compaction of α-helix 2 and α-helix 3, disruption of β-sheets, and unfolding of α-helix 1. Our map of the structural dynamics of the native conformation aids in rationalizing their observations. Our analysis indicates that the α2-α3 loop exhibits the largest local mobility in the protein structure, locates (together with α-helix 1) in Subdomain 2, and shows anticorrelated motion with respect to the structural core and α-helix 1.
Based on the structural dynamics pattern we mapped, we propose that conditions perturbing the structure of the α2-α3 loop beyond the range of conformational flexibility could initiate two misfolding events. Our proposal, however, cannot provide evidence on the relative probability of occurrence and time sequence of events.
Event 1: Straining of the cohesive and highly connected structural core, due to the strong anticorrelated dynamic coupling with the α2-α3 loop. The straining may lead to two effects: (1) compaction (or distension) of the mid-region of the α2 and α3 helices, and (2) distension (or compaction) of the β-sheets.
Event 2: Loosening of α-helix 1. The conformational flexibility of α-helix 1 allows it to withstand a range of conformational perturbations. And, the helix belongs to the same large subdomain as the α2-α3 loop. Therefore, a large perturbation in the loop, which is anticorrelatedly coupled to the helix, may unfasten the helix. Zhuo et al. [Citation33] observed a similar event using accelerated molecular dynamics simulations to model prion protein misfolding in acidic conditions. We speculate that, conversely, fastening α-helix 1 may prevent the opening of the globular fold [Citation13].
We argue that the binding poses we found could offset conformational rearrangements prone to misfolding in PrPC. Event 1 could be counterbalanced by either pose while Event 2 could be counterbalanced by Pose 1 or Pose 3.
Limitations
Our modelling protocol captures large amplitude and slow frequency collective motions. Still, it is not designed to capture the subtle effects of residue substitutions on the local network of side chain connectivity or backbone conformational variability of long unstructured regions. Our protocol cannot investigate either how key residue substitutions, linked to low susceptibility to prion diseases, may alter the equilibrium between folded and partially unfolded conformations or the kinetics of misfolding pathways. For example, the modelling technique cannot recover the proposed rank of conformational flexibility in the β2-α2 loop of PrPC in mammal species [Citation34,Citation35]. A question that remains open for future studies is to examine the effect of residue substitutions in the prion protein on the fine details that shift the relative populations of Event 1 and Event 2.
Conclusions
Our study provides a consensus perspective on critical structural features of the C-terminus of the prion protein PrPC, and provide insight into the hypothesis we stated. First, we show the robustness of the protein topology against naturally occurring residue substitutions in non-human mammals. Second, we identify distal but coupled residues forming a structural core that bridges intramolecular interactions. Third, we characterize protein subdomains based on distal connectivity of the residue interaction network. Fourth, we illustrate how the structural dynamics map helps elucidate the stabilizing role of pharmacological chaperones and how to interpret recent results based on kinetics studies of initial misfolding.
Methods
Input structures
Atomic Cartesian coordinates of the C-terminus prion protein structures (C-terminus PrPC) were obtained from the Protein Data Bank [Citation36]. We selected structures based on three criteria: (1) structure resolved using solution nuclear magnetic resonance (NMR), (2) sequence included only the globularly folded C-terminus, and (3) sequence corresponded to the wildtype form. A total of 13 NMR ensembles were selected, one per mammal species. The species included display differential susceptibility to prion diseases (pdb id in parenthesis): bankvole (2k56), bovine (1dx1), cat (1×yj), dog (1×yk), elk (1×yw), hamster (1β10), horse (2ku4), human (1qlz), mouse (2l39), pig (1×yq), rabbit (2fj3), sheep (1y2s), and wallaby (2kfl). Only residues common to all structures (residue 125 to 227; human PrPC residue numbering) were included in our analysis. All conformers in each NMR ensemble were included in all analysis.
Structural dynamics
To examine the conformational variance of Cα atomic positions, the root mean square fluctuation (rmsf) was calculated for each conformer in the NMR ensemble. To investigate the slow and collective motions encoded in the protein conformation, each conformer in each NMR ensemble was analysed using elastic network model (ENM) Cα normal mode calculations [Citation16,Citation17]. To capture the full dynamics of the protein topology, all non-trivial normal modes were included in our analysis except when stated otherwise [Citation37]. The root mean square inner product (rmsip) between all normal modes of any pair of conformers was calculated.
To identify the correlation between distant protein residues, the cross-correlations matrix of atomic displacements in each NMR conformer was calculated over all normal modes. The cross-correlation value between two residues i and j, Cij, ranges from −1 (anticorrelation, fluctuations out of phase) to +1 (correlation, fluctuations in phase). The closer the absolute value |Cij| to 1, the stronger the dynamic coupling.
To investigate the network of residue interactions, we used a dynamic network analysis method [Citation38]. To build the protein network, each residue was mapped to a node. Two nodes (node i and node j) are connected by one edge. Each connecting edge is assigned a weight according to the value from the cross-correlation matrix (-log |Cij|). From this, we calculated network centralities [Citation26–28]: The betweenness centrality measures the number of times a residue bridges the shortest path between two other residues. The eigenvector centrality measures how well a residue is connected to other well-connected residues. To examine the effect of long-range couplings, the calculations of the centralities were performed for a locality factor λ of 100 Å (includes long-range couplings) and 5 Å (dampens down long-range couplings). The Girvan-Newman method [Citation39] was used to partition the network into communities. The connections between residues that belong to the same community are denser than connections to other residues.
To find large and rigid subdomains in the protein structures, we used the GeoStaS algorithm [Citation23], with the k-means clustering. In the calculation, we included the first ten non-trivial modes and set the search for two subdomains to avoid overfitting. The geometry-based method identifies protein fragments that move coherently based on correlations in translational and rotational motions.
Analysis was performed with Bio3D version 2.0 [Citation24] as implemented in the R software environment [Citation40].
Docking
Blind docking calculations were performed with Autodock 4.2 [Citation41]. The input prion protein structure was hamster PrPC (pdb ID: 1b10; 25 conformers). The input pentosan polysulfate (PPS) structure was taken from [Citation42], consistent with previous work [Citation10]. The grid was built using Autogrid4 with a size of 126 × 126 × 126 points and a spacing of 0.375 Å. The Lamarckian genetic algorithm (GA) was selected with a population size of 300. The number of energy evaluations was 25 000 000 and the number of generations was 27 000. A total of 50 docking runs were performed for each NMR conformer. From the combined pool of 1,250 binding poses, we selected those with a score more negative than −2 kcal/mol to analyse hydrogen bond formation.
Molecular graphics were generated with VMD version 1.9.x [Citation43] and pymol [Citation44].
Supplemental Material
Download MS Word (8.3 MB)Acknowledgments
The authors acknowledge valuable discussions with Rafayel Petrosyan. Resources were provided in part by the MERCURY consortium (http://mercuryconsortium.org/) under NSF grants CHE-1229354, CHE-1662030, and CHE-2018427.
Disclosure statement
No potential conflict of interest was reported by the author(s).
Supplemental data
Supplemental data for this article can be accessed online at https://doi.org/10.1080/19336896.2023.2186674
Additional information
Funding
References
- Telling GC. The shape of things to come: structural insights into how prion proteins encipher heritable information. Nat Commun. 2022;13(1):4003. DOI:10.1038/s41467-022-31460-8.
- Wang L-Q, Zhao K, Yuan H-Y, et al. Cryo-EM structure of an amyloid fibril formed by full-length human prion protein. Nat Struct Mol Biol. 2020;27(6):598–602. DOI:10.1038/s41594-020-0441-5
- Glynn C, Sawaya MR, Ge P, et al. Cryo-EM structure of a human prion fibril with a hydrophobic, protease-resistant core. Nat Struct Mol Biol. 2020;27(5):417–423. DOI:10.1038/s41594-020-0403-y
- Kraus A, Hoyt F, Schwartz CL, et al. High-resolution structure and strain comparison of infectious mammalian prions. Mol Cell. 2021;81(21):4540–4551.e6. DOI:10.1016/j.molcel.2021.08.011
- Hoyt F, Standke HG, Artikis E, et al. Cryo-EM structure of anchorless RML prion reveals variations in shared motifs between distinct strains. Nat Commun. 2022;13(1):4005. DOI:10.1038/s41467-022-30458-6
- Manka SW, Zhang W, Wenborn A, et al. 2.7 Å cryo-EM structure of ex vivo RML prion fibrils. Nat Commun. 2022;13(1):4004. DOI:10.1038/s41467-022-30457-7
- Chen E-L, Kao H-W, Lee C-H, et al. 2.2 Å Cryo-EM tetra-protofilament structure of the hamster Prion 108–144 fibril reveals an ordered water channel in the center. J Am Chem Soc. 2022;144(30):13888–13894. DOI:10.1021/jacs.2c05479.
- Spagnolli G, Rigoli M, Orioli S, et al. Full atomistic model of prion structure and conversion. PLOS Pathog. 2019;15(7):e1007864. DOI:10.1371/journal.ppat.1007864
- Srivastava KR, Lapidus LJ. Prion protein dynamics before aggregation. Proc Natl Acad Sci. 2017;114(14):3572–3577.
- Petrosyan R, Patra S, Rezajooei N, et al. Unfolded and intermediate states of PrP play a key role in the mechanism of action of an antiprion chaperone. Proc Natl Acad Sci U S A. 2021;118(9):e2010213118. DOI:10.1073/pnas.2010213118
- Rigoli M, Spagnolli G, Faccioli P, et al. Ok Google, how could I design therapeutics against prion diseases? Curr Opin Pharmacol. 2019;44:39–45.
- Myers R, Cembran A, Fernandez-Funez P. Insight from animals resistant to prion diseases: deciphering the genotype – morphotype – phenotype code for the Prion Protein. Front Cell Neurosci. 2020;14. DOI:10.3389/fncel.2020.00254
- Mead S, Khalili-Shirazi A, Potter C, et al. Prion protein monoclonal antibody (PRN100) therapy for Creutzfeldt–Jakob disease: evaluation of a first-in-human treatment programme. Lancet Neurol. 2022;21(4):342–354. DOI:10.1016/S1474-4422(22)00082-5
- Gendoo DMA, Harrison PM, Legname G. The landscape of the prion protein’s structural response to mutation revealed by principal component analysis of multiple NMR ensembles. PLoS Comput Biol. 2012;8(8):e1002646.
- Soto P, Claflin IA, Bursott AL, et al. Cellular prion protein gene polymorphisms linked to differential scrapie susceptibility correlate with distinct residue connectivity between secondary structure elements. J Biomol Struct Dyn. 2021;39(1):129–139. DOI:10.1080/07391102.2019.1708794
- Brooks B, Karplus M. Harmonic dynamics of proteins: normal modes and fluctuations in bovine pancreatic trypsin inhibitor. Proc Natl Acad Sci. 1983;80(21):6571–6575.
- Hinsen K, Petrescu A-J, Dellerue S, et al. Harmonicity in slow protein dynamics. Chem Phys. 2000;261(1):25–37. DOI:10.1016/S0301-0104(00)00222-6
- Yao X-Q, Skjærven L, Grant BJ. Rapid characterization of allosteric networks with ensemble normal mode analysis. J Phys Chem B. 2016;120(33):8276–8288.
- Zhang S, Gong W, Han Z, et al. Insight into shared properties and differential dynamics and specificity of secretory phospholipase A2 family members. J Phys Chem B. 2021;125(13):3353–3363. DOI:10.1021/acs.jpcb.1c01315
- David CC, Jacobs DJ. Characterizing Protein motions from structure. J Mol Graph Model. 2011;31:41–56.
- Amadei A, Ceruso MA, Di Nola A. On the convergence of the conformational coordinates basis set obtained by the essential dynamics analysis of proteins’ molecular dynamics simulations. Proteins. 1999;36(4):419–424.
- Viles JH, Donne D, Kroon G, et al. Local structural plasticity of the Prion Protein. Analysis of NMR Relaxation Dynamics Biochemistry. 2001;40(9):2743–2753. DOI:10.1021/bi002898a
- Romanowska J, Nowiński KS, Trylska J. Determining geometrically stable domains in molecular conformation sets. J Chem Theory Comput. 2012;8(8):2588–2599.
- Grant BJ, Skjærven L, Yao X-Q. The bio3D packages for structural bioinformatics. Protein Sci. 2021;30(1):20–30.
- Oldham S, Fulcher B, Parkes L, et al. Consistency and differences between centrality measures across distinct classes of networks. PLoS ONE. 2019;14(7):e0220061. DOI:10.1371/journal.pone.0220061
- Foutch D, Pham B, Shen T. Protein conformational switch discerned via network centrality properties. Comput Struct Biotechnol J. 2021;19:3599–3608.
- Brysbaert G, Lensink MF. Centrality measures in residue interaction networks to highlight amino acids in protein–Protein binding. Front Bioinforma. 2021;1:0.
- Negre CFA, Morzan UN, Hendrickson HP, et al. Eigenvector centrality for characterization of protein allosteric pathways. Proc Natl Acad Sci. 2018;115(52):E12201–08. DOI:10.1073/pnas.1810452115
- Dima RI, Thirumalai D. Exploring the propensities of helices in PrP(C) to form beta sheet using NMR structures and sequence alignments. Biophys J. 2002;83(3):1268–1280.
- Sengupta I, Udgaonkar J. Monitoring site-specific conformational changes in real-time reveals a misfolding mechanism of the prion protein. Elife. 2019;8:e44698.
- Schilling KM, Tao L, Wu B, et al. Both N-Terminal and C-Terminal histidine residues of the prion protein are essential for copper coordination and neuroprotective self-regulation. J Mol Biol. 2020;432(16):4408–4425. DOI:10.1016/j.jmb.2020.05.020
- Arodź T, Płonka PM. Effects of point mutations on protein structure are nonexponentially distributed. Proteins Struct Funct Bioinforma. 2012;80(7):1780–1790.
- Zhou S, Shi D, Liu X, et al. PH-Induced misfolding mechanism of prion protein: insights from microsecond-accelerated molecular dynamics simulations. ACS Chem Neurosci. 2019;10(6):2718–2729. DOI:10.1021/acschemneuro.8b00582
- Christen B, Hornemann S, Damberger FF, et al. Prion protein NMR structure from tammar wallaby (Macropus eugenii) shows that the beta2-alpha2 loop is modulated by long-range sequence effects. J Mol Biol. 2009;389(5):833–845. DOI:10.1016/j.jmb.2009.04.040
- Sigurdson CJ, Nilsson KPR, Hornemann S, et al. A molecular switch controls interspecies prion disease transmission in mice. J Clin Invest. 2010;120(7):2590–2599. DOI:10.1172/JCI42051
- Rose PW, Prlić A, Altunkaya A, et al. The RCSB protein data bank: integrative view of protein, gene and 3D structural information. Nucleic Acids Res. 2017;45(D1):D271–81. DOI:10.1093/nar/gkw1000
- Wynsberghe AWV, Cui Q. Interpreting correlated motions using normal mode analysis. Structure. 2006;14(11):1647–1653.
- Sethi A, Eargle J, Black AA, et al. Dynamical networks in tRna: protein complexes. Proc Natl Acad Sci. 2009;106(16):6620–6625. DOI:10.1073/pnas.0810961106
- Girvan M, Newman MEJ. Community structure in social and biological networks. Proc Natl Acad Sci U S A. 2002;99(12):7821–7826.
- R Core Team. R: a language and environment for statistical computing. Vienna, Austria: R Foundation for Statistical Computing; 2020. Available from: https://www.R-project.org/
- Morris GM, Huey R, Lindstrom W, et al. AutoDock4 and AutoDockTools4: automated docking with selective receptor flexibility. J Comput Chem. 2009;30(16):2785–2791. DOI:10.1002/jcc.21256
- National Center for Biotechnology Information. PubChem compound summary for CID 37720, pentosan polysulfate. [cited 2022 Aug 3]. Available from: https://pubchem.ncbi.nlm.nih.gov/compound/37720
- Humphrey W, Dalke A, Schulten K. VMD: visual molecular dynamics. J Mol Graph. 1996;14(1): 33–38, 27–28. DOI:10.1016/0263-7855(96)00018-5
- The PyMOL Molecular Graphics System, Version 2.0; Schrödinger, LLC. https://pymol.org/2/support.html