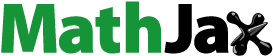
ABSTRACT
The weather conditions in one location can affect consumption patterns and the volume of electricity load demand (ELD) on power systems. This paper presents a wavelet-regression method to analyse the characteristic of ELD in Indonesia towards weather variables under tropical climate namely temperature, solar radiation exposure, humidity and wind speed using daily data from 2018 to 2020. The city of Makassar is used as a case study. The continuous wavelet transform (CWT) and wavelet transform coherence (WTC) analyses reveal that the variability of ELD, solar radiation and humidity showed high fluctuations within a period of 2 to 32 days. Strong relationships are found between the load and three weather variables (temperature, solar radiation exposure and humidity) which are represented in the colourful plots. Another result from multiple linear regression analysis for the whole period shows temperature has the highest impact on the volume of ELD, followed by solar radiation, and humidity. During the seasonal period, the impact of the seasons on the ELD is more pronounced in the rainy season compared to the dry season. The presented results are helpful for power management to meet ELD in Indonesia.
1. Introduction
Electricity is considered a vital key to socio-economic growth in one place. Therefore, power systems that can supply electricity continuously with predetermined quality standards to connect consumers from all sectors are required. Interruptions of electricity supply will have adverse economic effects on society as they can disrupt household and business activities, production processes in the industry, and access to social services, including safety and security concerns (Nduhuura, Garschagen, and Zerga Citation2021; Wu, Huang, and Wu Citation2018). These highlight the substantial role of reliable electrical energy service to existing consumers. To achieve this, gaining a detailed understanding of the load demand characteristics for a power system is crucial (Avdakovic, Ademovic, and Nuhanovic Citation2013). In general, comprehensive knowledge of load characteristics can be used by power utilities to maintain the daily operation effectiveness of an electricity network and planning process. Critical load drivers and their impact on electricity consumption during specific periods can be observed by analysing variables that influence load demand.
In many countries, the increase in electricity load over time is driven by several main sectors based on final end users including industrial, commercial and residential sectors. The electricity load has a typical pattern that can be affected by several factors, and one of them is the weather factor. The change in weather conditions can impact consumer’s behaviour in terms of the duration, setting and types of electrical devices used, which can alter the electricity demand profile in power systems (Salehizade et al. Citation2015). As stated in Emodi, Chaiechi, and Beg (Citation2018) and Valor, Meneu, and Caselles (Citation2001), electricity production in the energy sector is highly sensitive to weather fluctuations due to its close connection with parameters such as temperature, humidity and wind speed. Therefore, it is important for planners or electricity operators to have a detailed understanding of weather parameters that have the greatest impact on the load pattern. Furthermore, since electricity cannot be effectively stored at a large scale (Hammond and Waldron Citation2008; Jose, Mathew, and Krishnan Citation2016; Valor, Meneu, and Caselles Citation2001), a failure to correctly calculate the effect of load drivers can result in the depletion of resources and economic losses. This implies electricity is ideally generated at the right portion at all times as it must be used directly or instantly consumed (Valor, Meneu, and Caselles Citation2001). However, the effect of weather conditions on ELD can be different at certain places due to some factors including geographical location, urbanism situation, type of existing industries and commerce, and used building code (Gastli et al. Citation2013; Jo and Kim Citation2022). It is needed to develop a specific framework and analyse load demand based on the environment where the load is observed (Akil et al. Citation2014; Bonkaney, Sanda, and Balogum Citation2019; Gastli et al. Citation2013; Wangpattarapong et al. Citation2008). As the context may change under specific environments, conducting studies in different places will provide more knowledge about load demand.
Several studies from different countries have been published regarding electricity demand and climate conditions by constructing a model using certain methods. For example (Salehizade et al. Citation2015), the role of seasonal fluctuations of temperature on electricity consumption was analysed using the elasticity coefficient approach for the Fars region in Iran. From the study, they found that air temperature changes mainly for the maximum temperature has a strong correlation with electricity use. Bonkaney, Sanda, and Balogum (Citation2019) applied a wavelet coherence approach to reveal the relationship between daily ELD and weather variables in Niamey, Niger. They found that high seasonal interdependence between temperature and ELD in the periodicity of 256 to 512 days and 128 to 256 days, and humidity is in the periodicity of 256 to 512 days. In Avdakovic, Ademovic, and Nuhanovic (Citation2013) regression and wavelet coherence were used to analyse the typical relationship between temperature variables and load demand for three European countries. The results showed a relatively high linear relationship between temperature and load demand for tested power systems in each country (UK, Slovakia and Bosnia and Herzegovina). Also from the results, the time frames where a high correlation occurred between temperature and load can be identified. Akil and Miyauchi (Citation2013) analysed a typical relationship between commercial electricity load and weather fluctuation in Japan using seasonal regression models. They reported that cooling and heating degree days (CDD and HDD) variables have the highest effects on seasonal loads. Further, optimum temperature references for the seasonal loads were 19°C in summer and autumn, and 16°C for winter and spring. In Almuhtady et al. (Citation2019) the degree-day method was adopted to investigate electricity consumption in Jordan related to mean air temperature. The main conclusions were that the ELD sensitivity for temperature conditions increased on average, namely 11% for hot weather and 16.4% for cold weather. Also, electricity demand for cooling and heating saturated at 32.9 °C and 4.7 °C, respectively. In Sarkodie, Ahmed, and Owusu (Citation2021) the effect of climate variability on ELD in Norway was examined by using kernel-based regularised least squares technique. They found that electricity load from solar and wind was influenced by historical and behavioural patterns for residential, commercial and industrial sector ELD. Cawthorne et al. (Citation2021) investigated the impact of temperature fluctuation on electricity load in the Southern US by using regression analysis. The results showed the growth of the regional population explains most of the ELD variability on a decade time scale, while temperature can explain 44% to 67% variability of the seasonal loads. Du, Yu, and Wei (Citation2020) developed a partially linear functional coefficient technique to evaluate the effect of climatic change on electricity use for the residential sector in China. They found that climate change affected residential load more in hot weather than in cold weather. Also, climate sensitivity was influenced by income level as represented by GDP. Istiaque and Khan (Citation2018) analysed the effect of ambient temperature on electricity use in Dhaka by adopting statistical regression. It is reported the dependence of the ELD in Dhaka, Bangladesh on the ambient temperature is 75%. The decreasing of temperature by 1°C can reduce load demand around 81 MW. Kapler (Citation2021) applied the wavelet method to analyse household electricity load patterns during winter in Poland. It is reported that the use of the Morlet wavelet for household load profile study is slightly better than Meyer’s wavelet as its shape is more fitting to the shape of the load profile. In recent studies, Jo and Kim (Citation2022) applied multiscale geographically weighted regression to observe the effect of various factors including temperature variables on electricity load for buildings in Korea. They found that temperature, household characteristics, and age of construction are some critical factors for building’s electricity consumption. Next Condori, Lora, and Camargo (Citation2022) applied a heating and cooling degree days approach to reveal the correlation between climate fluctuation and ELD in Huancayo. They concluded that the increase of ELD in Huancayo, Peru, was affected partially by lower temperature.
Especially for the wavelet transform (WT) method, it has gained traction across many disciplines in recent years (Bonkaney, Sanda, and Balogum Citation2019). This creates new opportunity for analysing data in various fields including in the electrical engineering area (Avdakovic et al. Citation2013; Kapler Citation2021) such as in (Bilgili et al. Citation2021; Cen, Yoo, and Lim Citation2022; González-Concepción, Gil-Fariña, and Pestano-Gabino Citation2012; Grinsted, Moore, and Jevrejeva Citation2004; Khochiani and Nademi Citation2019; Lee and Choi Citation2019; Moudjari et al. Citation2019; Saroha et al. Citation2021; Zhao et al. Citation2018). Wavelet is a wave function within a finite duration that simultaneously provides time and frequency information. The capabilities of the wavelet in exploring the co-movement correlation between two variables at different time-frequency spaces make this method attractive. It can provide broad information on how investigated variables affected each other throughout time interval observation. Basically, this method has been developed as an alternative to STFT methods that rely on fixed windows. Conversely with STFT, the wavelet window can stretch into a more extended scale to determine the low-frequency dynamics and be compressed into a smaller scale to measure the high-frequency dynamics of the signal (Aguiar-Conraria and Soares Citation2011). WT is classified into two class transformations: discrete and continuous wavelet transforms (DWT and CWT) (Grinsted, Moore, and Jevrejeva Citation2004). DWT is commonly used for image processing, denoising, and extraction purposes, while the latter is used to analyse time-series data (Grinsted, Moore, and Jevrejeva Citation2004).
As a part of our research to understand completely ELD characteristics in Indonesia, this present study proposed wavelet-regression methodology with a focus on observing in detail the typical association between ELD and weather parameters under tropical climates. Firstly, CWT analysis is performed to observe variability for each variable. Secondly, WTC is applied to analyse dynamic coherence among considered variables across various time scales. Finally, regression analysis is used to rank and quantify the influence of the variables on the ELD under the whole and seasonal periods. To the best of our knowledge, there are very limited studies on ELD in Indonesia related to weather conditions in the international literature. Specifically, no systematic studies are found so far using wavelet transform in the Indonesian context. As described above, the contributions of the research and novelty can be summarised as follows:
Developing and applying wavelet – regression methodology to reveal typical load characteristics under tropical climate.
Load characteristics may not be the same in different countries (specific environments), therefore this study can fill the research gap by providing more insight into typical Indonesian ELD to international readers.
As information from the proposed models is practically helpful for sustainable energy consumption through better power management to meet ELD in Indonesia, therefore, the presented study can contribute towards advancing research in sustainability.
Next, Section 2 presents typical electricity load and weather variables in the studied city, Section 3 provides the methodology, results are presented in Section 4, and finally the conclusions and future work are given in Section 5.
2. Input data
In this work, daily ELD data in Makassar for three consecutive years are used from 1 January 2018 to 31 December 2020. As the biggest metropolitan city in the eastern Indonesia region and identified as the city with the largest population outside Java island, Makassar city in Sulawesi island is chosen as a case study with a number of electricity consumers is around 835 thousand in the year 2018 (Statistics Indonesia - Makassar City Citation2021). The electricity energy use data from PT. PLN (Persero) in Makassar (power utility) are accumulation load for all electricity sectors. Meanwhile meteorological data were obtained from Meteorology, Climatology, and Geophysical Agency (BMKG) data centre in Makassar. The weather variables include temperature average (°C), solar radiation exposure (hour), humidity (%) and wind speed (m/sec). The considered weather variables which are assumed to have an effect on ELD are based on the literatures (Akil et al. Citation2014; Bonkaney, Sanda, and Balogum Citation2019; Cawthorne et al. Citation2021; Salehizade et al. Citation2015). The usage of three years daily data for each variable in this study is due to data availability. However, as ELD patterns are relatively repeated in one year, thus, the three years data for analysis are sufficient for characterisation as it can demonstrate clear patterns for the whole and specified time ranges.
As a preliminary characteristic analysis, show electricity use, temperature, and solar radiation duration values for the studied period. From a sample of the data in January 2018 as in , the volume of ELD is not the same every day and similar conditions occurred for temperature and solar radiation exposure values. However in , variation of the electricity demand has the same tendency with temperature fluctuation values generally. Meanwhile in the electricity load only follows variations of solar radiation exposure on certain days. Next, wavelet transform and regression analysis are proposed to reveal more detailed load characteristics as further discussed in the next section.
3. Methods
The method or workflow utilised to reveal load characteristics in a tropical climate based on wavelet and regression methodologies is shown in . In this study, wavelet and regression analysis for observed data are simulated using the commonly used Biwavelet and Waveslim libraries in the R language and EViews, respectively. The Waveslim library is used to transform the wavelet using signal processing techniques, including signal decomposition. On the other hand, Biwavelet is used to generate the graphical approach of correlation between observed parameters, including the CWT and WTC analysis. The following subsections provide a detailed description of each step of the research.
3.1. Preliminary data processing
It is known that data with good statistical properties are required before implementing wavelet analysis. For this purpose, preliminary data processing can be done in the first step (Raza, Shah, and Sharif Citation2019; Sharif et al. Citation2017). One of the approaches that can be used for preliminary data processing is continuously compounded return also called log return (Wu and Shahidehpour Citation2010). In this study, the approach is applied to change the actual time series data into return series form to make the statistical properties of the observed variables (input data) in terms of the outcome more comparable. In (Wu and Shahidehpour Citation2010), the one-period continuously compounded return series is formulated in Eq. (1).
3.2. Multi-resolution analysis (MRA): CWT
In the second step, MRA analysis using the normalisation data is performed to explore signals at different frequencies within different resolutions. Signals with means, variances and covariance that change over time known as non-stationary signals are primarily found in a wide range of practical applications. One of the MRA analysis form which is capable to illustrate frequency and time resolution at the same time is CWT. Basically, CWT computes time-series convolution with a scaled and translated version of the wavelet function. The scale of the wavelet can be stretched or compressed
. The changing width of the wavelet allows this transform to compute every single spectrum component in the signal. Here, the Morlet wavelet with
is chosen as the wavelet function as it is adequate to represent the best compromise between time and frequency concentration (Bilgili et al. Citation2021). Given a time-series x(t), CWT concerning wavelet ψ can be written as in Eq. (2) (Aguiar-Conraria and Soares Citation2011).
3.3. WTC
To assess the correlation level between two variables across the various time scales, WTC is deployed in the next step. The wavelet coherence for two time series and
is generally formulated as follows.
In EquationEquation (4)(4)
(4) ,
is the coherence between two variables at specific frequency and time, s is the spectrum value, and Wx is the wavelet transform in n frequency. The
value is required in the range
where if the numbers close to
indicate a weak correlation between the two observed time series while when the coefficient value
nearly
indicating a strong correlation between the two time series.
As phase difference is applied as a reference to identify the typical relationship between variables, a phase difference of indicates that the observed time series is moved together or has a positive correlation, whereas anti-phase or negative correlation for two time series is marked with a phase difference with
or
. In the WTC spectrum, a phase relationship is illustrated by an arrow with specific directions. If
then the time series y is leading, and if
then x is leading within in phase relationship for both. On the other hand, for the anti-phase relationship if the arrow appointed in quadrant
then x is leading, and if
then y is leading where here, x represents electricity load, whereas y represents the analysed four weather variables including temperature, solar radiation, humidity, and wind speed. Adopted in Aguiar-Conraria and Soares (Citation2011), the phase difference circle is given in .
3.4. Regression model
To obtain more information about the characteristic of the load, the multiple linear regression method was applied in the last step. Multiple linear regression is used to observe a typical relationship between studied variables and it is generally formulated as in EquationEquation. (5(5)
(5) ) (Mir et al. Citation2021)
In the above equations, ELDwp, ELDrs, and ELDds are electricity load for the whole period, rainy season, and dry season period, respectively. TEM is temperature, SOL is solar radiation exposure, HUM is humidity, and WIN is wind speed. Meanwhile δ0 or γ0 or λ0 is a coefficient constant, and others are regression coefficients for related models.
Following Mirasgedis et al. (Citation2006) and Taylor and Buizza (Citation2003) to tackle autocorrelation, the autoregressive structure is involved in the error term of each model as in Eq. (9). Here, it is examined until second-order autoregressive, and then applied standard statistical tests (Akaike Information Criterion (AIC) and Schwarz Criterion (SC)) for selecting the best appropriate model among options. The AIC and SC are two statistical measures for comparative assessment among alternative time series models which are formulated by Eqs. (10) and (11), respectively (Profillidis and Botzoris Citation2018). The smaller the AIC and SC values, the better the model in terms of the fit of the model among options related to the analysed data as the tests show an estimation regarding information lost for a specific model (Pardo, Meneu, and Valor Citation2002; Profillidis and Botzoris Citation2018). Some studies that applied AIC and SC tests as a foundation for model selection can be found in (Mirasgedis et al. Citation2006; Pardo, Meneu, and Valor Citation2002).
where øp is constant, p is the order of autoregressive, and ɛt is white noise.
In EquationEquations (10)(10)
(10) and (Equation11
(11)
(11) ) above, n is the data number, l is the log of the likelihood function, and k is the estimated parameters number.
4. Results and discussion
4.1. Preliminary data processing
The result of return series decomposition with multiple time-frequency domains is shown in . Return series in this observation refers to the time series data representation of the used variables over the period of time as presented in the Section 2. The mean value of the return series is nearly zero and the series shows more homogeneous characteristics. Based on this, the statistical property of the transformed series (after normalisation data) is better than the original series. Next, from the figure, it is seen that fluctuation is shown for the entire trend. The mean of all signals is not constant from time to time. Therefore, they can be categorised into non-stationary signals. However, among the variables, ELD has lower fluctuation than other weather variables.
4.2. WPS
Obtained WPS for all variables are depicted in . In the figures, the horizontal axis shows the entire sample period in days up to 1096 data, and the vertical axis is responsible for obtaining the frequency domain. Here, the indices were decomposed into seven scales from short to long run to get co-movement for each area in a different time and frequency resolution. The short-run scale is for capturing the signal’s high-frequency component, whereas the coarser scales are for obtaining the signal’s low-frequency component. The scale levels exhibit days dynamics from 2 to 4 days (scale 1), 4 to 8 days (scale 2), 8 to 16 (scale 3), 16 to 32 (scale 4), up to the coarser
Figure 7. CWT spectrum for each weather variable: (a) temperature, (b) solar radiation exposure, (c) humidity, and (d) wind speed.

scale of 128 to 256 days. Concerning the colour of the spectrum, it marks co-movement intensity in the observed area. The blue colours indicate no co-movement, whereas the red colours indicate a high positive co-movement in an observed area. Finally, the cone shading assists to specify which area is taken into consideration. The areas outside the cone are negligible, while the cone areas are considered statistically significant at 95% and the thick black line that covers the red spectrum represents a 5% significance level.
From the wavelet power spectrum in , they exhibit a similar pattern at a shorter scale. Short-lived noises are identified at the higher frequency level for the entire spectrum indicates abrupt changes and primarily occurs in 2 to 16 days. This means the prominent variation mainly occurred on the top of the wavelet spectrum. A close look at the observed spectrum exhibits interesting findings, amongst other weather variables mentioned, high similarities were obtained between electricity load and temperature spectrum which are marked by the significance area in the ranges 2 to 32 days.
The similarities of the high-intensity area in the ranges of 2 to 16 days which are also founded on ELD, temperature, and humidity spectrum indicate that the specific region of these variables is highly correlated. Meanwhile fewer high-intensity areas are identified within the same period on the solar radiation and wind speed spectrum. Besides the volatile information of each variable, a similar pattern of significance area that is obtained in the power spectra of observed variables confirmed if those variables are correlated in a certain way. Typical correlations amongst those variables are given in the WCT analysis.
In further analysis, shows a wide range of highly fluctuated areas throughout year 2020. The possible reason that lies behind this phenomenon is that the pandemic era of COVID-19 strikes at the beginning of the year 2020. As the COVID-19 struck, authorities have imposed specific policies to minimise activity in the public area, and as the household sector dominated the electricity usage in Makassar city, it is evident that the pandemic era was directly affecting the load variability in the year 2020. The prominent variation evidently occurred during the year 2020.
4.3. Wavelet coherence between ELD and weather parameters
4.3.1. ELD and air temperature
shows resulted wavelet coherence between ELD and temperature variable. From the figure, the high correlation between electricity load and temperature is found in a wide range of different period bands. As its arrow in the significant area is pointed in the ranges of or
, then it is classified into an in-phase relationship or positive association between the mentioned variables. In the periodicity of 64 to 128 days, a strong seasonal relationship occurred with a phase difference in quadrant
which indicates temperature leads the load demand. Comprehensively from the year 2018 to 2019, the temperature variable is affecting the variability of the load. Significance areas were also identified around the beginning of the year 2019 and 2020 in the periodicity of 2 to 64 days within electricity demand affecting the temperature. As the electricity supply in the observed area is still dominated by thermal power plants, thus abrupt changes in electricity usage that are commonly occurred at every turn of the year possibly affect the air temperature variable. Fossil fuel combustion as thermal power plant sources can be related to temporary temperature changes. As in (Du, Yu, and Wei Citation2020; Gastli et al. Citation2013; Topolewski Citation2021), the energy use from fuel consumption or traditional sources can impact climate changes such as the change of average temperature due to the increasing greenhouse gas concentration. Practically, the effect of physical quantity resulting from temperature changes influences the comfort of consumers and this condition may lead to changes in electricity consumption.
4.3.2. ELD and solar radiation exposure
WCT concerning solar radiation is given in . From the figure, a wide range of significant coherence regions is identified within an in-phase relationship, or a positive correlation between load and solar radiation is discovered. A strongly correlated area is founded in the periodicity of 4 to 32 days in the beginning year 2019. As its phase ranges in the quadrant and
, the exact conclusion of the phase relationship could not be drawn, yet it still exhibits a positive association between the two variables. A highly correlated area was also discovered in the periodicity of 2 to 64 days at the beginning of the year 2020.
4.3.3. ELD and humidity
The anti-phase relationship in the periodicity of 2 to 32 and 64 to 128 days in humidity is significant over the entire time horizon, as shown in . Here, the anti-phase relationship indicates a negative correlation between these two variables. Specifically in the periodicity of 64 to 128 days, the arrows appointed in the range with electricity load lagging the humidity and certain arrows just appointed in the range [
. However, in the periodicity 2 to 32 days, from 2019 to 2020 the arrows appointed in the quadrant
confirmed that humidity is lagging the electricity load. also depicts a low rate of correlation between ELD and humidity variables. It has been proven by small region formation on a scale 2 to 8 and a year transition from 2020 to 2021 where the arrows pointed in the quadrant
4.3.4. ELD and wind speed
shows wavelet coherence regarding wind speed. Unlike other variables, low coherence between wind speed and electricity load is identified. As in certain regions are highly localised, but the arrows are appointed in the scattered direction. Based on this, it can be concluded that despite some highly localised regions, the coherence between load and wind speed variables is still categorised as very low or weak. It is in line with the findings of a previous study (Bonkaney, Sanda, and Balogum Citation2019).
4.4. Ranking of weather parameters in influencing ELD
show the best regression results for the three composed models. All the models structured by autoregressive 2nd order are statistically validated. The F-statistic probability (Prob. of FS) value 0 confirms that at least one of the observed variables in the related models influences load demand (Wangpattarapong et al. Citation2008). Next, Durbin-Watson statistics around two indicate the composed model free from serial correlation problem. For handling heteroskedasticity, it is performed corrected standard error regression (Startz Citation2007).
Table 1. Regression results for the whole period.
Table 2. Regression results for the rainy season.
Table 3. Regression results for the dry season.
Under the whole period (), obtained adj. R2 value of the model is 0.9144. Referring Wangpattarapong et al. (Citation2008), this indicated 91.44% of the daily ELD in Makassar could be explained by the variation of TEM, SOL, HUM, and WIN. Among the variables, wind speed (WIN) has no significance at 5% level as shown by the p-value above 0.05. The comparison of variable significance shows that temperature (TEM) has the greatest impact on the volume of load demand, followed by solar radiation (SOL) and humidity variable (HUM). This is shown by the value of variable coefficient which is highest for TEM (50.8053) and the lowest for HUM (2.0144). The variables affect ELD in a positive direction as confirmed by obtained regression coefficients have positive sign. Temperature that has the highest impact on load demand is consistent with past research’s findings (Akil and Miyauchi Citation2013; Wangpattarapong et al. Citation2008).
In terms of the seasonal period, the adj. R2 values for rainy season and dry season models are 0.8964 and 0.9241, respectively. By applying a 5% of significance level, HUM and WIN variables are not significant in influencing load in the rainy season. Meanwhile, in dry season, SOL and WIN are found to have no significant impact on the load demand. In relation to the effect of seasons, considered weather parameters can explain the variation of ELD better in the dry season than in the rainy season as shown by their adj. R2 values. TEM is the main ELD driver for both seasons. However, from their regression coefficient values as in , the temperature variable during the rainy season has a higher impact than the dry season. The increase of 1°C temperature will raise daily electricity use to around 50.95 MWh in the rainy season, while in the dry season is 37.61 MWh. Typical weather variable values for both seasons are given in . From , temperature range in the rainy season is 24.30 °C to 33.20 °C, meanwhile in the dry season is 25.10 °C to 30.60 °C. Temperature fluctuation during rainy season is slightly higher than in other season. For SOL which has the same range value for both seasons, it has significance effect to load demand only in rainy season. Meanwhile, for HUM with seasonal range values as seen in , it influences load demand significantly only in the dry season. The increase of 1 hour solar radiation duration will increase the daily load in the rainy season by around 5.78 MWh. Meanwhile, the increasing 1% humidity in the dry season will raise daily electricity consumption by around 2.82 MWh. As for WIN, this variable is found insignificant in both seasons. If the average tariff in the year 2020 is IDR 1,071.36 per kWh (PT. PLN Persero Citation2021), increasing temperature by 1 °C and solar radiation duration by 1 hour in the rainy season will probably raise daily energy cost by around IDR 60.6 million. Meanwhile for the dry season, increasing temperature by 1 °C and humidity by 1% will raise daily energy cost by IDR 43.2 million. In other words, if significance variables in related seasons rise 1 unit, energy costs in the rainy season are 1.4 times higher than in the dry season.
Table 4. Typical weather parameters in the rainy season.
Table 5. Typical weather parameters in the dry season.
Weather variables fluctuations that change the volume of energy use are basically in line with (Bonkaney, Sanda, and Balogum Citation2019; Midiani, Subagia, and Widiantara Citation2017; Shaikh et al. Citation2014) which confirmed TEM, HUM, and SOL are some parameters that affect user convenience. The comfort level influences health of occupants, productivity, and working efficiency (Shaikh et al. Citation2013, Citation2014). Therefore, to keep the comfortable condition at home or at workplaces for example when temperature increase, occupants use more cooling devices (lower temperature setting for AC and or higher air speed for the fan) which leads to an increasing in daily ELD. Based on the electricity sectors, household and commercial sectors are often reported to be affected by weather variables such as in (Akil and Miyauchi Citation2013; Wangpattarapong et al. Citation2008). In relation to this, in South Sulawesi province where Makassar is located, ELD in Year 2020 comes from three main sectors namely residential (53.01%), industrial (19.64%), and commercial sector (18.23%) with total users from these three sectors is 97.7% (PT. PLN Persero Citation2021). Next in Indonesia, the standard comfort of users in a building particularly for temperature and humidity is 24 °C to 27 °C and 55% to 65%, respectively (Ministry of Energy and Mineral Resources Citation2012). Further seasonal analysis for the two variables () reveals 81.47% observations in the rainy season and 92.57% in the dry season the temperature value is above 27 °C. Meanwhile for humidity, 99.81%
observations in the rainy season and 94.56% in the dry season, the humidity value exceeds 65%. Using standard comfort as a reference, consumers in the observed city experienced hotter and more humid days in both seasons, resulting in higher electricity usage during the rainy season.
5. Conclusions
This study applies a wavelet-regression approach to reveal the characteristic of Indonesian electricity load demand (ELD) related to temperature, solar radiation exposure, humidity and wind speed using three years of daily data from 2018 to 2020. Based on the continuous wavelet transform and wavelet transform coherence analyses, it can be concluded that variability of ELD, humidity and solar radiation highly fluctuates in the ranges of 2 to 32 days. The power spectrum especially for ELD showed highly fluctuated areas throughout the year 2020 as COVID-19 struck at the beginning of 2020. Next, a strong relationship is acquired between ELD and temperature, solar radiation exposure, and humidity. For temperature is in the periodicity of 2 to 64 days and 64 to 128 days, for solar radiation is in the periodicity of 4 to 32 days and 2 to 64 days, meanwhile for humidity is the periodicity of 2 to 32 days and 64 to 128 days. Unlike other variables, low coherency was found for wind speed and load demand. Further analysis using regression approach showed temperature has the highest impact on the volume of load demand and followed by solar radiation, and humidity under the whole period. In terms of the seasonal period, the effect of seasons on the ELD is higher in the rainy season than in the dry season. When seasonal load drivers raise 1 unit simultaneously (temperature and solar radiation in the rainy season, and temperature and humidity in the dry season), then energy costs in the rainy season are 1.4 times higher than in the dry season. As this study provides primary variables governing the changeability in electricity use, it is hoped that the presented results can contribute to power management such as a basis to control daily power system operation and to improve forecasting and plan to meet ELD. This research type is in line with Papageorgiou et al., (Citation2020) which highlighted the importance of finding ways to better manage the operation of power systems including electricity generation and energy use in modern life. With some extensions, future research will attempt to develop a wavelet methodology to analyse consumer load profiles during and after the post-COVID-19 pandemic in Indonesia.
Acknowledgments
The authors acknowledge the power utility and BMKG center data in Makassar in providing data for this study. We thank the people at DGEE Research Group in UNHAS for their support in completing this research. Thank also to the anonymous reviewers for their suggestions to enhance the quality of this paper.
Disclosure statement
No potential conflict of interest was reported by the authors.
Data availability statement
Supporting data for this research are provided upon request to corresponding author.
Additional information
Notes on contributors
Yusri Syam Akil
Yusri Syam Akil is a senior lecturer at the Department of Electrical Engineering, Hasanuddin University, Indonesia. His research interests include energy management, power demand analysis and forecasting, and power system optimization.
Dewi M. Fortuna
Dewi M. Fortuna is a student at the Department of Electrical Engineering, Hasanuddin University, Indonesia. Her research interests include power demand analysis and data science.
Hasniaty A.
Hasniaty A is a lecturer at the Department of Electrical Engineering, Hasanuddin University, Indonesia. Her research interests include power system analysis, power quality and energy management.
References
- Aguiar-Conraria, L., and M. J. Soares (July 12, 2011). The Continuous Wavelet Transform: A Primer. Accessed May 28, 2022. http://www3.eeg.uminho.pt/economia/nipe/docs/2011/NIPE_WP_16_2011.pdf.
- Akil, Y. S., and H. Miyauchi. 2013. “Seasonal Regression Models for Electricity Consumption Characteristics Analysis.” Engineering 5 (1): 108–114. https://doi.org/10.4236/eng.2013.51b020.
- Akil, Y. S., Syafaruddin, T. Waris, and A. A. H. Lateko (2014). “The Influence of Meteorological Parameters Under Tropical Conditon on Electricity Demand Characteristic: Indonesia Case Study.” Proceedings of the 1stInternational Conference on Information Technology, Computer and Electrical Engineering 383–387. IEEE.
- Almuhtady, A., A. Alshwawra, M. Alfaouri, W. Al-Kouz, and I. Al-Hinti. 2019. “Investigation of the Trends of Electricity Demands in Jordan and Its Susceptibility to the Ambient Air Temperature Towards Sustainable Electricity Generation.” Energy, Sustainability and Society 9 (39): 1–18. https://doi.org/10.1186/s13705-019-0224-1.
- Avdakovic, S., A. Ademovic, and A. Nuhanovic. 2013. “Correlation Between Air Temperature and Electricity Demand by Linear Regression and Wavelet Coherence Approach: UK, Slovakia and Bosnia and Herzegovina Case Study.” Archieves of Electrical Engineering 62 (4): 521–532. https://doi.org/10.2478/aee-2013-0042.
- Avdakovic, S., A. Nuhanovic, M. Kusljugic, E. Becirovic, and E. Turkovic. 2013. “Wavelet Multiscale Analysis of a Power System Load Variance.” Turkish Journal of Electrical Engineering and Computer Sciences 2:1035‒1043. https://doi.org/10.3906/elk-1109-47.
- Bilgili, F., D. B. Lorente, S. Kuskaya, F. Ünlü, P. Gençoğlu, and P. Rosha. 2021. “The Role of Hydropower Energy in the Level of CO2 Emissions: An Application of Continuous Wavelet Transform.” Renewable Energy 178:283–294. https://doi.org/10.1016/j.renene.2021.06.015.
- Bonkaney, A. L., I. S. Sanda, and A. A. Balogum. 2019. “Wavelet Analysis of Daily Energy Demand and Weather Variables.” Journal of Energy 2019:1–7. https://doi.org/10.1155/2019/4974107.
- Cawthorne, D., A. R. D. Queiroz, H. Eshraghi, A. Sankarasubramanian, and J. F. DeCarolis. 2021. “The Role of Temperature Variability on Seasonal Electricity Demand in the Southern US.” Frontier Sustainable Cities 3:1–11. https://doi.org/10.3389/frsc.2021.644789.
- Cen, S., J. H. Yoo, and C. G. Lim. 2022. “Electricity Pattern Analysis by Clustering Domestic Load Profiles Using Discrete Wavelet Transform.” Energies 15:1–18. https://doi.org/10.3390/en15041350.
- Condori, J., G. A. Lora, and S. D. Camargo (2022). “The Impact of Climate Change on Electricity Demand in the City of Huancayo.” Proceedings of the 6thInternational Conference on Energy and Environmental Science, 1008: 1–6. https://doi.org/10.1088/1755-1315/1008/1/012001.
- Du, K., Y. Yu, and C. Wei. 2020. “Climatic Impact on China’s Residential Electricity Consumption: Does the Income Level Matter.” China Economic Review 63:1–12. https://doi.org/10.1016/j.chieco.2020.101520.
- Emodi, N. V., T. Chaiechi, and A. R. A. Beg. 2018. “The Impact of Climate Change on Electricity Demand in Australia.” Energy & Environment 29 (7): 1–35. https://doi.org/10.1177/0958305x18776538.
- Gastli, A., Y. Charabi, R. A. Alammari, and A. M. Al-Ali (2013). “Correlation Between Climate Data and Maximum Electricity Demand in Qatar.” Proceedings of the IEEE GCC Conference and Exhibition, Doha, Qatar, 565–570. IEEE.
- González-Concepción, C., M. C. Gil-Fariña, and C. Pestano-Gabino. 2012. “Using Wavelets to Understand the Relationship Between Mortgages and Gross Domestic Product in Spain.” Journal of Applied Mathematics 2012:1–17. https://doi.org/10.1155/2012/917247.
- Grinsted, A., J. C. Moore, and S. Jevrejeva. 2004. “Application of the Cross Wavelet Transform and Wavelet Coherence to Geophysical Time Series.” Nonlinear Processes in Geophysics 11 (5/6): 561–566. https://doi.org/10.5194/npg-11-561-2004.
- Hammond, G. P., and R. Waldron (2008). “Risk Assessment of UK Electricity Supply in a Rapidly Evolving Energy Sector.” Proceedings of the IMechE Part A: J. Power and Energy 222, 623–642. https://doi.org/10.1243/09576509JPE543.
- Istiaque, A., and S. I. Khan. 2018. “Impact of Ambient Temperature on Electricity Demand of Dhaka City of Bangladesh.” Energy and Power Engineering 10 (07): 319–331. https://doi.org/10.4236/epe.2018.107020.
- Jo, H., and H. Kim. 2022. “Analyzing Electricity Consumption Factors of Buildings in Seoul, Korea Using Multiscale Geographically Weighted Regression.” Buildings 12 (678): 1–19. https://doi.org/10.3390/buildings12050678.
- Jose, D., M. Mathew, and A. Krishnan (2016). “Weather Dependency of Electricity Demand: A Case Study in Warm Humid Tropical Climate.” Proceedings of the 3rdInternational Conference on Electrical Energy Systems, Chennai, India, 102–105. IEEE.
- Kapler, P. 2021. “An Application of Continuous Wavelet Transform and Wavelet Coherence for Residential Power Consumer Load Profiles Analysis.” Bulletin of the Polish Academy of Sciences: Technical 69 (1): 1–8. https://doi.org/10.24425/bpasts.2020.136216.
- Khochiani, R., and Y. Nademi. 2019. “Energy Consumption, CO2 Emissions, and Economic Growth in the United States, China, and India: A Wavelet Coherence Approach.” Energy & Environment 31 (5): 886–902. https://doi.org/10.1177/0958305x19881750.
- Lee, H. K., and Y. S. Choi. 2019. “Application of Continuous Wavelet Transform and Convolutional Neural Network in Decoding Motor Imagery Brain-Computer Interface.” Entropy 21 (12): 1–11. https://doi.org/10.3390/e21121199.
- Midiani, L. P. I., I. W. A. Subagia, and I. B. G. Widiantara (2017). “Characterized Temperature and Humidity for Classroom Comfort Zone in Bali.” Proceedings of the International Joint Conference on Science and Technology, Bali, Indonesia, 248–251.
- Ministry of Energy and Mineral Resources. (2012). Republic of Indonesia Government Regulation No. 13 Year 2012 About Savings in Electricity Consumption. Accessed February 4, 2023. https://migas.esdm.go.id/uploads/regulasi/profil_peraturan_304.pdf.
- Mirasgedis, S., Y. Sarafidis, E. Georgopoulou, D. P. Lalas, M. Moschovits, F. Karagiannis, and D. Papakonstantinou. 2006. “Models for Mid-Term Electricity Demand Forecasting Incorporating Weather Influences.” Energy 31 (2–3): 208–227. https://doi.org/10.1016/j.energy.2005.02.016.
- Mir, A. A., K. Ullah, Z. A. Khan, F. Bashir, T. U. R. Khan, and A. Altamimi. 2021. “Short Term Load Forecasting for Electric Power Utilities: A Generalized Regression Approach Using Polynomials and Cross-Terms.” Engineering Procedia 12 (21): 1–4. https://doi.org/10.3390/engproc2021012021.
- Moudjari, A., I. Kezzouli, H. Talbi, and A. Draa. 2019. “A Wavelet-Based Approach for Business Protocol Discovery of Web Services from Log Files.” Bulletin of the Polish Academy of Sciences: Technical 67 (3): 535–546. https://doi.org/10.24425/bpasts.2019.129652.
- Nduhuura, P., M. Garschagen, and A. Zerga. 2021. “Impacts of Electricity Outages in Urban Households in Developing Countries: A Case of Accra, Ghana.” Energy 14 (12): 1–26. https://doi.org/10.3390/en14123676.
- Papageorgiou, G., A. Efstathiades, M. Poullou, and A. N. Ness. 2020. “Managing Household Electricity Consumption: A Correlational, Regression Analysis.” International Journal of Sustainable Energy 39 (5): 1–11. https://doi.org/10.1080/14786451.2020.1718675.
- Pardo, A., V. Meneu, and E. Valor. 2002. “Temperature and Seasonality Influences on Spanish Electricity Load.” Energy Economics 24 (1): 55–70. https://doi.org/10.1016/S0140-9883(01)00082-2.
- Profillidis, V. A., and G. N. Botzoris. 2018. Modeling of Transport Demand: Analyzing, Calculating, and Forecasting Transport Demand. Elsevier. https://doi.org/10.1016/B978-0-12-811513-8.00003-0.
- PT. PLN (Persero). (2021). PLN Statistics 2020. Accessed July 28, 2022. https://web.pln.co.id/statics/uploads/2021/07/Statistik-PLN-2020.pdf.
- Raza, S. A., N. Shah, and A. Sharif. 2019. “Time Frequency Relationship Between Energy Consumption, Economic Growth and Environmental Degradation in the United States: Evidence from Transportation Sector.” Energy 173:706–720. https://doi.org/10.1016/j.energy.2019.01.077.
- Salehizade, A. A., M. Rahmanian, M. Farajzadeh, and A. Ayoubi. 2015. “Analysis of Temperature Changes on Electricity Consumption in Fars Province.” Mediterranean Journal of Social Science 6 (3): 610–617. https://doi.org/10.5901/mjss.2015.v6n3s2p610.
- Sarkodie, S. A., M. Y. Ahmed, and P. A. Owusu. 2021. “Ambient Air Pollution and Meteorological Factors Escalate Electricity Consumption.” Science of the Total Environment 795:1–13. https://doi.org/10.1016/j.scitotenv.2021.148841.
- Saroha, S., M. Zurek-Mortka, J. R. Szymanski, V. Shekher, and P. Singla. 2021. “Forecasting of Market Clearing Volume Using Wavelet Packet-Based Neural Networks with Tracking Signals.” Energies 14 (19): 1–21. https://doi.org/10.3390/en14196065.
- Shaikh, P. H., N. B. M. Nor, P. Nallagownden, and I. Elamvazuthi. 2013. “Building Energy Management Through a Distributed Fuzzy Inference System.” International Journal of Engineering & Technology 5 (4): 3236–3242.
- Shaikh, P. H., N. B. M. Nor, P. Nallagownden, I. Elamvazuthi, and T. Ibrahim. 2014. “A Review on Optimized Control Systems for Building Energy and Comfort Management of Smart Sustainable Buildings.” Renewable and Sustainable Energy Reviews 34:409–429. https://doi.org/10.1016/j.rser.2014.03.027.
- Sharif, A., R. Jammazi, S. A. Raza, and S. J. H. Shahzad. 2017. “Electricity and Growth Nexus Dynamics in Singapore: Fresh Insights Based on Wavelet Approach.” Energy Policy 110:686–692. https://doi.org/10.1016/j.enpol.2017.07.029.
- Startz, R. 2007. EViews Illustrated for Version 6. Quantitative Micro Software.
- Statistics Indonesia - Makassar City. (2021). Makassar City in Figures 2021. Accessed July 19, 2022. https://makassarkota.bps.go.id/publication/2021/02/26/be312e3f776bcfd005978bda/kota-makassar-dalam-angka-2021.html/.
- Taylor, J., and R. Buizza. 2003. “Using Weather Ensemble Predictions in Electricity Demand Forecasting.” International Journal of Forecasting 19 (1): 57–70. https://doi.org/10.1016/S0169-2070(01)00123-6.
- Topolewski, Ł. 2021. “Relationship Between Energy Consumption and Economic Growth in European Countries: Evidence from Dynamic Panel Data Analysis.” Energies 14 (12): 1–12. https://doi.org/10.3390/en14123565.
- Valor, E., V. Meneu, and V. Caselles. 2001. “Daily Air Temperature and Electricity Load in Spain.” Journal of Applied Meteorology 40:1413–1421. https://doi.org/10.1175/1520-0450(2001)040<1413:DATAEL>2.0.CO;2.
- Wangpattarapong, K., S. Maneewan, N. Ketjoy, and W. Rakwichian. 2008. “The Impacts of Climatic and Economic Factors on Residential Electricity Consumption of Bangkok Metropolis.” Energy and Buildings 40 (8): 1419–1425. https://doi.org/10.1016/j.enbuild.2008.01.006.
- Wu, K. Y., Y. H. Huang, and J. H. Wu. 2018. “Impact of Electricity Shortages During Energy Transitions in Taiwan.” Energy 151:622–632. https://doi.org/10.1016/j.energy.2018.03.049.
- Wu, L., and M. Shahidehpour. 2010. “A Hybrid Model for Day-Ahead Price Forecasting.” IEEE Transactions on Power Systems 25 (3): 1519–1530. https://doi.org/10.1109/TPWRS.2009.2039948.
- Zhao, Y., R. C. Laguna, Y. Zhao, J. J. Liu, X. He, J. Yianni, and P. G. Sarrigiannis. 2018. “A Wavelet-Based Correlation Analysis Framework to Study Cerebromuscular Activity in Essential Tremor.” Complexity 2018:1–15. https://doi.org/10.1155/2018/7269494.