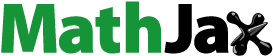
ABSTRACT
This study investigated the historical and future wave power potential in the Gulf of Guinea (GoG) with the aim of identifying high-density wave energy locations for potential exploitation. To estimate wave power density (WPD) for three time periods (past: 1979–2005, mid-century: 2026–2050, and end-century: 2081–2100), we utilized significant wave height and mean wave period obtained from eight General Circulation Models. Using an ensemble of these WAVEWATCH III simulated datasets, we calculated WPD and assessed overall and seasonal trends, projecting changes under Representative Concentration Pathway (RCP) 4.5 and 8.5 scenarios. Results revealed higher potential WPD in the western GoG, particularly near the coast, with increased values offshore. Spatially, WPD change rates varied widely (−0.021 to 0.039 kW/m per year), suggesting both positive and negative trends, though generally low. Projections indicated a potential increase from 0.5 to 1.0 kW/m by the end of the century. The estimated potential power for harvesting exceeded 14,000 MW, with offshore regions showing better wave converter performance. This study concludes that GoG's wave energy is a promising renewable resource, offering a potential solution to future power needs and contributing to regional greenhouse gas emission mitigation.
1 Introduction
The need for migration from fossil fuel dependent power generation to more renewable means has become pressing as the impacts of climate change continue to increase globally (Sawin et al. Citation2016). Relative to solar and wind, ocean waves have better predictability as a renewable energy resource in addition to higher energy density (Mwasilu and Jung Citation2019). The major cities in the Gulf of Guinea (GoG) such as Abidjan, Accra, Cotonou, Douala, Lagos and Lomé which are also where the major portion of the power is consumed are all on the coast. The location of this major load centres are close to the potential locations of the ocean wave power generators, which is an added advantage in terms of reduced cost for power distribution.
However, despite these aforementioned potentials, the exploitation of the ocean wave energy as a source of power is still under-utilised globally due to the high Levelized Cost of Energy (LCOE). The LCOE is the price at which the generated electricity must be sold for power generation to be considered profitable (Lehmann et al. Citation2017). This relatively high LCOE is expected to become economical in the future as technologies for ocean wave harvesting emerge. The LCOE has traditionally served as a widely used metric to compare the cost-effectiveness of different energy sources, including wave energy (Guillou, Lavidas, and Chapalain Citation2020). However, due to the unique challenges and characteristics associated with wave energy projects, it’s important to consider alternative metrics that offer a more comprehensive view of their economic viability.
One such alternative to LCOE is the Net Present Value (NPV) analysis, which takes into account both the initial investment costs and the future cash flows generated by a wave energy project. By factoring in the time value of money, NPV provides a more detailed assessment of the project’s financial feasibility over its entire lifespan, incorporating elements such as operational expenses, capital outlays, and revenue projections (Dalton, Alcorn, and Lewis Citation2010). The Internal Rate of Return (IRR) is another metric that holds significance in assessing wave energy projects (Simal et al. Citation2017). Calculating the discount rate at which the net present value of projected cash flows equals zero, IRR offers insight into the potential return on investment. It aids in comparing wave energy projects with other investment opportunities and provides a gauge of profitability. In the West African context, Adesanya et al. (Citation2020) found that though the initial investment is high, it is poised to bring about more economical electricity generation and transmission, environmental enhancements, and a consistent energy supply that aligns with demand in West Africa, contrasting favourably with the conventional generation methods.
For a more immediate measure, the payback period presents an alternative approach by quantifying the time required for the initial investment in a wave energy project to be recuperated through generated revenue. A shorter payback period suggests a quicker return on investment and could be viewed favourably from a financial perspective. Considering the broader context, the Levelized Avoided Cost of Energy (LACE) accounts for the value generated by producing energy from wave sources compared to not generating energy and relying on an alternative source (Coe et al. Citation2022). It evaluates potential cost savings and benefits in relation to other options. In light of the uncertainties inherent to wave energy, sensitivity analysis becomes crucial. This approach assesses how variations in key parameters, such as equipment costs, operational performance, and energy prices, impact the economic feasibility of the project. It provides a range of potential outcomes, offering a more comprehensive perspective. Furthermore, as wave energy projects can have significant environmental and social implications, metrics that encompass these factors are also valuable. Evaluating reductions in greenhouse gas emissions, local job creation, and enhanced energy security contributes to the holistic assessment of a wave energy exploitation.
In evaluating the profitability of wave energy projects in a developing region such as the GoG, it is important to adopt a combination of these alternative metrics, aligning them with the project’s context and objectives. Singular metrics might not provide a comprehensive view, and the evolving landscape of technological advancements, policy shifts, and market dynamics can significantly influence the economic viability of wave energy initiatives over time. For example, factors such as capacity of the project which indicates the actual energy output as a percentage of the maximum potential, and the reliability of the technology in demanding ocean conditions can offer insights unique to wave energy assessment. Opportunity of job creation and attraction of related investments will be other factors that are important to decide the feasibility of the wave energy exploitation project in a region such as the GoG. In addition, the possibility of installing the wave power generators alongside other generators like offshore wind farms is also expected to make the exploitation of ocean wave power economical in the future (Osinowo Citation2019). Judging from the previous information, one can conclude that the best metrics for assessing cost-effectiveness of ocean wave energy in the GoG will be a hybrid of different metrics which is beyond the scope of the present study.
A number of studies assessing the potential of wave energy resources have been carried out in various parts of the world. These include the Indian Ocean (Karunarathna et al. Citation2020), China (Liang et al. Citation2013), Persian Gulf (Kamranzad, Etemad-Shahidi, and Chegini Citation2013), Malaysia (Mirzaei, Tangang, and Juneng Citation2014), Australia (Cuttler, Hansen, and Lowe Citation2020; Hughes and Heap Citation2010). These studies observed that temporal variations in wave power can result from even little change in wind wave climate. Some of these studies have also explored the different wave energy converters (WEC) available and their efficiency in varying locations. For example, Henriques et al. (Citation2019) assessed the oscillating-water-columns (OWCs) converter type which ae considered one of the most efficient type currently in use. This is owing to their advantage in a commendable energy conversion efficiency, enabling them to effectively harness wave energy and transform it into usable power. Their structural simplicity is another compelling facet since they are designed with straightforward configurations, facilitating their assembly and deployment. This simplicity streamlines the installation process, potentially reducing both time and costs associated with setup (Cabral et al. Citation2020). Another crucial advantage of OWCs is their inherent reliability which is attributed to the strategic placement of all mechanical components above the still water level (SWL). By avoiding direct exposure to the challenging marine environment, these components are shielded from the corrosive effects and physical stresses that prevail in such settings. This design consideration significantly enhances the longevity and dependability of OWCs, minimising the need for frequent maintenance or repairs (Ciappi et al. Citation2022).
A study by Yusov et al. (Citation2021) gives details of other cost-effectiveness WECs. In addition to OWCs, the field of WECs encompasses a diverse array of technologies that are actively being explored and developed. Each of these technologies offers distinct approaches to harnessing the energy of ocean waves, and they hold promise for contributing to renewable energy generation. Point absorbers, for instance, are buoyant structures that rise and fall with the motion of waves. These devices are tethered to the seabed and employ their vertical movement to generate mechanical power through hydraulic systems, which is then transformed into electricity. This design benefits from its relative simplicity and adaptability to varying wave conditions. Attenuators present another innovative concept with elongated, floating structures oriented perpendicular to the direction of wave propagation (Yusov et al. Citation2021). They consist of interconnected segments that flex and move in response to wave motion, creating relative movement between segments that can be converted into mechanical power and subsequently transformed into electrical energy. Overtopping devices operate by utilising the potential energy inherent in waves as they wash over a barrier. This elevated water is collected in a reservoir and subsequently released through turbines as it flows back down to its original level, generating electricity in the process. This design leverages the dynamic interaction between wave energy and gravity to produce power. Terminators, on the other hand, capture wave energy by capitalising on the pressure difference between their front and back sides. Positioned perpendicular to wave direction, these devices convert the varying pressure levels into mechanical power, which is then converted into electrical energy through appropriate mechanisms. Submerged pressure differential systems take advantage of the pressure discrepancies between the surface and subsurface due to wave activity. By effectively capturing and converting these pressure differences, these systems can generate mechanical energy that is then converted into electricity, offering a unique approach to wave energy extraction. Resonant devices align with the natural frequencies of waves or oscillate at frequencies closely related to them. This resonance is harnessed to convert the motion of the device into usable electrical power through various conversion mechanisms (Moretti et al. Citation2020). Babarit et al. (Citation2012) proposed some power matrices through wave-to-wire (W2W) modelling approaches for the selection of WECs by comparing different set-up at different sites. They did this by carrying out a combination of computational and numerical modelling of physical phenomena and W2W output for each device, employing the equations of motion and hydrodynamic modelling methods. Subsequently, they used W2W models to compute power matrices for individual devices, along with determining the average yearly power uptake across five distinct representative wave locations along the European Coast. This study provided the required background for the choice of power matrices needed in the current study.
However, in the GoG, Osinowo et al (Citation2018, Citation2019) assessed ocean wave power potential based on 37-year wave hindcast. Another study by Adesanya et al. (Citation2020) explored the prospects of ocean wave as a renewable energy only a section of West Africa. Despite providing a much-needed baseline for comparison during intersecting period, these very few previous studies mostly cover a section of the GoG. In another study assessing the feasibility of exploiting wave power in Ghana by Tulashie et al. (Citation2022), the estimated total wave power of 7215 MW was found to be another significant addition to renewable energy source in Ghana. However, all these previous studies in the GoG have been focussed on the past emphasising the need for future centred assessment to give information about climate change-induced variations in ocean wave power potential. It is also worthy of note that these studies are few in number compared to other regions of the world coupled with the fact that they are mostly done on national scales making them unrepresentative of the potential exploitable renewable energy in the region. Therefore, the temporal coverage of both past and future period will provide an update to the previously available information while extending the knowledge on what should be expected in terms of potential wave energy around the GoG in the future. This is also needed to take advantage of the regional coastal management strategy previously proposed for the GoG region by Alves et al. (Citation2020). This regional exploitation of ocean resource is expected to increase the feasibility and profitability of evolving renewable energy source such as ocean wave in a region with relatively uniform hydrodynamic and economic conditions like the GoG.
Information on the hydrodynamics of a region may come from various sources, which include observations relying on visual aids by experts on Voluntary Observing Ships (VOSs) (Grigorieva, Gulev, and Gavrikov Citation2017; Swail et al. Citation2010; Vettor and Guedes Soares Citation2019), in-situ buoy measurements, video camera and unmanned aerial vehicles (UAVs) (Angnuureng et al. Citation2016, Citation2020; Arnaud et al. Citation2021), satellite altimeters (Young, Zieger, and Babanin Citation2011) as well as numerical wave models (Alves et al. Citation2014; Booij, Holthuijsen, and Battjes Citation2001; Chen et al. Citation2018; Hasselmann et al. Citation1988). These various wave climate data acquisition methods have their pros and cons. The most important advantage models provide, is the high spatiotemporal resolution at a relatively low cost. This gives numerical models an edge when analysing long-term metocean parameters, especially in areas with scanty observational data, like the GoG.
Wave power potential is dependent on the ocean wave climate of a region which is projected to experience significant spatial as well as temporal changes in the GoG before the end of the century (Dahunsi et al. Citation2022). This projected change warrants the need to assess the future wave power potential in the GoG to assess the magnitude of change likely to be experienced. This will aid the decision and policy on the feasibility of exploiting wave power as a source of electricity in the countries of the GoG. This will also be an addition to the steps taken globally to achieve carbon neutrality by 2050. Therefore, the objective of this study is to examine future magnitudes of ocean wave energy and the feasibility of its exploration for power generation in the countries of the GoG.
2 Study area
The GoG, as defined in this study, covers the coastal waters from the Cape Palmas in Liberia down to the Cape Lopez in Gabon (Dahunsi et al. Citation2022). This covers several West and Central African countries including the Bight of Benin in the northwest and Bight of Bonny in the southeast (). The GoG has the eastward flowing Guinea Current as the predominant ocean current in the region (Foli et al. Citation2022). Biologically, the GoG is a very productive region resulting in the upwelling region called the Guinea Current Large Marine Ecosystem (GCLME) (Abe and Brown Citation2020).
Figure 1. Map of the GoG showing the bathymetry of the region, bordering countries and major cities (black labels), coastal and inland water bodies (blue lines).

In terms of wave climate, the GoG is known to have predominantly swell waves with average conditions of approximately 1.36 m and 9.6 s for wave height and period respectively (Almar et al. Citation2015). In extreme conditions, these values can be up to 1.62 m and 10.86 s, respectively (Dahunsi et al. Citation2022). The type of microtidal condition in GoG has neap and spring tides ranges of 0.3 m and 1.8 m respectively (Alves et al. Citation2020). As seen in , the GoG has a relatively narrow continental shelf with the wide areas seen around Cape Three Point (Ghana) and the Niger Delta (Nigeria).
3 Data and methods
3.1 Data source and acquisition
The data used for this study was the contribution of the Commonwealth Scientific and Industrial Research Organisation (CSIRO), Australia to the second phase of the Coordinated Ocean Wave Climate Project (COWCLIP2.0) (Hemer and Trenham Citation2016). The modelling was done using a dynamical wave approach which was forced with 3-hourly 10 m above surface wind fields and monthly sea-ice fields from 8 General Circulation Models (GCMs) included in the Coupled Model Intercomparison Project (CMIP5) (Taylor, Stouffer, and Meehl Citation2012). This dataset contains WAVEWATCH III (WW3; Tolman Citation1991) wave model generated data using the ST3 (BAJ) source-term physics on a 1° × 1° spatial resolution (Hemer et al. Citation2013). The details of the model set-up including the number of GCM(s) used, atmospheric downscaling & wind-wave modelling method vis-à-vis the atmospheric corrections, calibration, source-term packages, spectral partition as well as the various contributing institutions (research centres) and nations are presented in Morim et al. (Citation2020). For consistency with other GCMs, minor changes were made to WW3 for some of the simulations for them to properly simulate the 360 and 365-day year, respectively. According to Bricheno et al. (Citation2015), this is to account for difference in various GCMs such as GFDL-CM3 using a 365-day calendar whereas HadGEM2-ES makes use of a 360-day model year. Therefore, the wave model was set-up to run uninterestingly including a warm-starts at the beginning of all years. This approach of modelling allows the use of the data for the starting conditions of one year as the ending conditions of the year before it.
Generally, the COWCLIP2.0 database contains 155 model results from various 10 modelling institutions with globally available information on wave climate (Morim et al. Citation2018). The simulations were validated against 26 years of satellite data for significant wave heights. The downscaling methods and validation results are as presented by Morim et al. (Citation2020). This dataset is very important as it provides the needed data for future large-scale coastal studies into climate change impacts risks and vulnerability assessments, especially for regions where regional databases are not available for future projections. Parameters produced in the COWCLIP2.0 include significant wave height (Hs), mean wave period (Tm) and mean wave direction (Dm).
For the current study, the monthly average of significant wave height (hs_ave) and average wave period (tm_ave) were extracted. This extraction was done for 3 different time slices, one in the past (1979–2005) and two in the future (2026–2045 and 2081–2100). These time slices are henceforth referred to as historical, mid-century and end-century respectively. The future simulations were done under two Representative Concentration Pathway (RCP) scenarios. The RCP4.5 is the lower emission scenario which captures efforts to stabilise the climate by adopting technologies and climate change mitigation and adaptation strategies whereas RCP8.5 scenario is the higher emission scenario releasing more greenhouse gases into the environment by the end of the 21st century.
3.2 Wave power density computation and statistical analysis
Firstly, the GoG data spatial coverage for this study was defined to range between longitudes 9° West −16° East and latitudes 3° South- 16° North. Since the various GCMs included in the COWCLIP2.0 dataset used different parametrisations to account for different ocean physics, a multi-model ensemble of all 8 GCMs was preferred. This ensemble was produced by aggregating data for the different wave climate parameters after which an average was computed. This is believed to give a better representation of the different modelling parametrisations. To assess the inter-seasonal variations in the WPD, the ensemble data was group into two major seasons in the GoG. The dry season (November–March) and the rainy season (April–October), sometimes referred to as winter and summer, respectively. The resulting data were used in the computation of the wave power density similar to the approach previously employed by similar studies in the region such as Osinowo et al. (Citation2018) and Tulashie et al. (Citation2022). This method relies on the energy equation that relates the wave power per unit crest to Hs and Tm (Defne, Haas, and Fritz Citation2009). The wave power density varies directly with the average wave period as well as the square of the significant wave height. This means that higher wave power should be exploitable from regions with high waves provided the variation of the average wave period is not much. The average wave power density (KW/m) was for each grid point over the GoG using equation 1:
where represents the wave power density measured in kW per m of wave crest, ρ is the ocean water density given as 1025 kg per m3, g is the acceleration due to gravity, Hs stands for the significant wave height in m and Tm is the average wave period given in s. This represents a simplified version of the existing wave energy flux, specifically the wave energy potential per unit crest, which is the amount of energy received at a designated location. Its definition is rooted in the cumulative representation of the wave energy spectrum. This version of wave computation methodology is favoured due to the challenges inherent in obtaining the dispersion of wave energy across frequencies and directions especially in a data deficient region such as the GoG where studies rely on global dataset. This complication arises as many wave datasets primarily encompass provide parameters like significant wave height and wave period. Given these circumstances, streamlined formulations were embraced to estimate the accessible wave power density. The adoption of these formulations hinged on distinct assumptions based on whether the context pertained to shallow waters, moderate water depths, or deep waters (Wan et al. Citation2015). However, prior extensive assessments of the available resource, such as the examination conducted for the GoG in this analysis, predominantly relied on the assumption related to deep waters. As highlight in the review by Guillou et al. (Citation2020), in deep water where the product of the wave number (k) and water depth (d) is far great than 1, the wave height and wave period are important parameters for wave energy computation.
Consequently, as expected for a simplified method such as this, there are assumptions associated with applying this method. The formula for calculating wave energy flux, as previously provided, rests upon a series of assumptions and simplifications that aid in arriving at a straightforward expression (Ciappi et al. Citation2022). These assumptions and simplifications play a pivotal role in shaping the applicability and accuracy of the formula within specific contexts. One of such assumptions is that the formula assumes a scenario of monochromatic waves, where all waves possess identical frequencies and directions. This assumption simplifies calculations but diverges from the reality of ocean waves, which often comprise a mixture of waves with varying frequencies and directions. Moreover, the derivation of the formula relies on the framework of linear wave theory. This theoretical foundation presupposes that wave amplitudes are markedly smaller compared to the wavelength. While this approximation is suitable for gentle or small waves, it falters when considering larger, steeper waves. Additionally, the formula operates under the assumption of a non-dissipative environment, where factors like wave breaking, bottom friction, and other energy loss mechanisms are overlooked. This limitation confines the formula to an idealised scenario, neglecting the inherent dissipative characteristics of real ocean conditions. The formula also assumes uniformity across the wave field, assuming consistent wave parameters across a vast expanse. Yet, in natural settings, wave conditions display substantial variance due to variables such as wind patterns, coastal features, and underlying bathymetry. A significant simplification emerges from considering a single mode of wave propagation characterised by a single frequency and phase speed. However, the real-world ocean contains a multitude of wave modes and frequencies, rendering this simplified perspective incomplete. Furthermore, the formula doesn’t factor in directional spreading of wave energy. Real ocean waves come from diverse directions, leading to a dispersion of energy across various angles – an aspect that the formula overlooks. In terms of context, the formula presumes a constant water depth. This assumption doesn’t encapsulate scenarios where water depths fluctuate, a common occurrence in diverse ocean environments. Moreover, the formula dismisses wave interactions such as wave focusing, wave breaking, and energy transfer between different wave modes. These interactions can significantly impact wave behaviour but are absent from the formula’s scope.
The estimation of WPD is made with the assumption of standard shapes of the wave energy spectrum which may lead to over- or under-estimation in ocean with two wave energy maxima with a combination of long-crested swell and short-crested wind-sea waves, respectively. However, since the GoG is a swell dominated region and the deep ocean is considered in this study, it is assumed that these approximations will not have significant effect. The relatively narrow continental shelf seen in most places in the GoG () suggests relatively deep water.
In order to assess the pattern of the trends in the WPD of the GoG for the past and future, a trend analysis was done. The estimated trend was also subjected to Mann-Kendal test of trend significance similar to the approaches employed in Dahunsi et al. (Citation2022). This allows to see the rate of increase or decrease of the WPD over time and to see where the change in rates can be adjudged to be statistically significant with a 95% confidence limit. A positive trend suggests an increasing rate while a negative trend corresponds to decreasing WPD between the first and last years in the time slice being considered.
Also, to quantify the changes expected in the future, the average for the past WPD was subtracted from the averages of the different future time slices and RCP scenarios. This change estimation was done on a grid-by-grid basis i.e. the average of the WPD for each grid point for the past time slice was subtracted from the future average WPD value for the same grid-point. A positive value means the WPD for the particular future period is higher than that recorded in the past and vice versa. A one-wave analysis of variance (ANOVA) test was carried out to check if the average WPD for the past is significantly different from those of the future. This was followed by the post-hoc Tukey–Kramer tests to find where the differences occur. To also confirm the strength of the changes in wave climate on WPD, correlation was done for the different wave components used for the computation of the WPD i.e. Hs and Tm.
Potential WEC power output estimation
Applying a combination of numerical methods and power matrices previously used in Babarit et al. (Citation2012), Guillou and Chapalain (Citation2018), Veigas and Iglesias (Citation2014) and Rusu and Onea (Citation2016), the exploitable power in the GoG can be estimated for different WECs. This approach computes the wave energy absorption estimate for a specific device at a given location by multiplying the power matrix of the device with the scatter diagrams representing wave statistics at that location in terms of Hs and Tm. Similar to the methods and values used by Rusu and Onea (Citation2016), the performances of the three WECs previously described in Guillou and Chapalain (Citation2018) were assessed for the GoG region. The first WEC, Pelamis, designed for water deeper than 50 m operates as a 750 kW offshore floating machine. It comprises of interconnected semi-submerged cylinders through hinged joints, aligned with wave propagation. As waves traverse its length, the sections move, generating mechanical energy. This energy is then converted to electricity through power take-off systems within the joints. The second WEC, AquaBuoy, also designed for water deeper than 50 m is rated at 250 kW and features a buoy linked to an underwater cylinder housing an accelerator tube with a piston and hose pump. The buoy’s oscillations compress the pump, channelling pressurised water to a Pelton turbine for electricity generation. The third device, Wave Dragon, has a 5.9 MW rating and is a slack-moored, floating system utilising overtopping designed for water deeper than 30 m. It employs two reflecting wings to guide waves towards a ramp, with overtopped water collected and directed to Kaplan turbines for energy conversion.
The choice of WEC was informed by the public availability of information, as these technologies are already mature and in use in other parts of the world. In contrast, the assessment methods were adopted because the reference points in the previous studies where they have been applied also have an average wave climate similar to that of the GoG. The scatter matrix plots showed a similar pattern both in terms of annual total and seasonal changes, although the winter period is the most energetic in their case. To estimate power output (WPO) from each WEC, the power matrices of the WEC are multiplied elementwise by the scatter diagram representing the bivariate distributions of wave climate. EquationEquation (2)(2)
(2) shows this mathematically:
where, denotes the percentage of wave energy associated with the bin specified by column j and row i, while
signifies the corresponding power from the power matrix (given in Table S1-S3 in the supplementary section) of the WEC for the same bin.
Furthermore, in a bid to assess the efficiency of the different WEC systems in the GoG, three other performance indicators were estimated. One is the normalised non-dimensional electric power (WPE) which is a ratio of the electrical power produced at a point i,j ( to the maximum value (
of the electrical power over the entire GoG in the duration covered for each WEC. Another one is the capture width (CW) which is the width perpendicular to the wave direction within which the wave energy machine extracts power from the waves. The other is the capacity factor (CF) which is estimated as a ratio between the electric power (WPO) generated by each WEC relative to the maximum rated power (WRP) of each system. These are given by equations (3–5):
4 Results
4.1 Average wave climate in the GoG
The wave climate of the GoG defined in terms of the average Hs and Tm for the past time slice (1979–2005) presented in show both spatial and temporal variations. Spatially, it can be observed that the wave height shows a south-north and east -west increasing pattern with the lowest waves experienced between the Niger Delta and Cameroon (5°E-10°E and (2.5°N-5°N)). Higher waves are seen offshore which become lower as they travel towards the coast. This clearly shows the evolution of the predominant swell waves which is generated from the south-western part of the Atlantic Ocean as they travel towards the GoG. This spatial variation can be seen for all periods () though the magnitude varies. Temporally, it can be observed that higher waves are seen in the GoG during the rainy season compared to the dry season. This is evidenced in the average Hs of 1.25 m, 1.10 m and 1.36 m estimated for the annual overall, dry and rainy season respectively.
Figure 2. Spatial distribution of wave climate for the 1979–2005 time slice: (a) Hs overall (b) Hs dry season (c) Hs rainy season (d) Tm overall average (e) Tm dry season (f) Tm rainy season.

A look at show a seemingly reverse in the spatial variation compared to the Hs equivalence on the left side. For example, one can observe a north-south gradient in Tm distribution. Generally, wave periods show increase from south to north along the GoG. The spatial distribution of Tm also displays an east-west variation, influenced by coastal and bathymetric features. However, the eastern regions such as the Cameroon section of the GoG, near the coast, may experience shorter wave periods due to the interaction of local winds and coastal morphology. However, the interplay between the influence of swells travelling from offshore and coastal morphology may determine the Tm in other parts especially in the south around Gabon. Temporally, similar to Hs, the wave period variation in the GoG is influenced by seasonal changes. During the rainy season when atmospheric conditions are more active, wave periods are longer due to the arrival of swells from distant storm systems. Conversely, in the dry season, shorter wave periods associated with locally generated wind waves are seen in the GoG. The average Tm estimated in this study for the annual overall, dry and rainy season are 9.25, 9.06 and 9.38 respectively.
In order to see the difference in terms of magnitude between the past and future wave conditions, the wave climate (Hs and Tm) for the past was subtracted from the future projections. For the projected wave climate and the expected change in the GoG shown in , one can see that an increase in Hs is to be expected in the annual average for all RCP scenarios. This increase which ranges from 1.3 to 2.62 cm is highest by the end of century for the RCP 8.5 scenario. In contrast, the dry season is expected to experienced decrease in Hs ranging from 1.58 to 1.92 cm. The future scenarios also showed that the rainy season will continue to have higher waves compared to the dry season with an increase projected to range from 3.45 to 5.78 cm depending on the RCP scenario. For the future wave period, a general increase is expected round the year for all RCP scenarios. This increases which are highest during the rainy season range from 0.065 to 0.16 for the overall annual average, 0.044–0.096 s for the dry season and 0.081–0.21 s for the rainy season.
4.2 Average WPD for the past and future
The spatial distribution of the average WPD presented in is for the past time slice (1979–2005), which shows very similar spatial trend to all other time slices and RCP scenarios. The only difference observed are in the values from one part of the GoG to the other. It can be observed that the WPD has a north to south and east to west increasing pattern similar to that reported for Hs in previous section. Locations around Cote d’Ivoire-Ghana axis have relatively higher values compared to the Nigeria-Cameroon section of the gulf. When one compares the dry season () and rainy season () distribution of wave power, it is observed that higher values are recorded in the rainy season. This is also similar to finding for wave height reported in previous section.
Figure 4. Spatial distribution of average WPD for the 1979–2005 time slice: (a) overall average (b) dry season (c) rainy season.

For the past time slice, the general overview of the average WPD values in the GoG ranges from 0.25 kW/m in the coastal part during the dry season to as high as 13.59 kW/m offshore during the rainy season. Dry season records the lowest WPD while rainy season has the highest WPD, which corresponds to the low and high wave energy seasons respectively in the GoG. This same trend is recorded for all the various time slices and RCP scenarios though with varying magnitudes ().
Figure 5. Overall and seasonal averages of zonal WPD for different time slices and RCP scenarios: (a) past (b) mid-century (c) end-century.

For the future time slices, though one can see a pattern of higher values of WPD for the end-century RCP 8.5 scenario, the pattern is not uniform. For example, the RCP 4.5 scenario has relatively higher values than the RCP 8.5 scenario during the mid-century which is not the same for the end-century. RCP 4.5 suggests that wave energy will be stronger during the mid-century compared to the end-century whereas the reverse is the case for the RCP 8.5 scenarios. The overlaps seen in the lines for the various RCP scenarios () for the zonal averages suggest that the difference between the magnitudes is not large.
A comparison of the WPD for both past and future time slices with the corresponding wave climate results presented in section 4.1 emphasised the influence of wave height especially in determining the WPD of a region. For example, spatially, it can be seen in that higher wave energy is also projected for offshore (longitude of −9) which corresponds to the westernmost part of the GoG compared to the eastern section (longitude of 10). The low wave power trough seen around longitude 8–9 corresponds to the Niger Delta-Cameroon axis which is known for the least wave energy as can be verified in . This same spatial distribution is seen for all the time slices and seasons. In addition, the temporal variations from dry season to rainy season also agrees with higher WPD for the rainy season with highest wave in the GoG. Likewise, the higher wave height projected for the future in previous section is also evidenced in for all RCP scenarios. It can, therefore, be inferred that the spatiotemporal variation of the WPD in the GoG is strongly dependent on both wave height and period.
4.2 Trends in WPD for the past and future
The linear trend analysis done for each grid point in the GoG to check the rate of change of WPD within a particular time slice was averaged meridionally to give the zonal mean shown in . For the past time slice (), it is seen that the averaged overall trend is positive, meaning the WPD in the GoG experienced increase between 1979 and 2005. This increase was higher in the dry season (). However, the rainy season showed negative trend value during this period (). In , it can be seen that the values of the overall averages of the trend (cyan line) are all above zero i.e. positive trend values. Similar to the overall trend average, the values plotted on the magenta line (dry season) are also all above zero depicting positive trend for all places between 9°W to 10°E in the GoG. However, for the rainy season, the values shown by the corresponding plot (blue line) show all values are below the zero tick on the vertical axis interpreted as generally negative trend in this season. It should be noted that the trends being described are the rates of changes of WPD (KW/m per year) rather than the trend of the lines themselves. In terms of magnitude, higher positive trends are seen in the western part of the GoG towards Cote d’Ivoire-Ghana axis compared to the eastern side from the overall and dry season trend values. In the rainy season, the negative trends are higher in the western axis.
Figure 6. Zonal variations in overall and seasonal averages of trend in WPD for different time slices and RCP scenarios: (a) past (b) mid-century (c) end-century. The black asterisks on the overall and rainy lines in (a) represent significant trend.

Table 1. Summary of the average values of WPD for different time slices.
Table 2. Trends in mean overall and seasonal WPD for different time slices in kW/m per year.
A closer observation of the variations of the trend values in for all seasons showed that the transition from west to east at 0° marks continuous decrease in the values (heading towards zero) for both positive and negative trends. This is more obvious in the lines for dry and rainy season plots (magenta and blue lines). Since the magnitudes in are meridional averages, the sharp decline seen in the easternmost part coincides with the Niger Delta-Cameroon part of the GoG which have been previously shown to have low values of WPD in . It can also be seen that the magnitude of decrease reported in the rainy season is relatively lower (−4.9–17.4 × 10−3 kW/m per year) compared to the values 22.3–70.3 × 10−3 kW/m per year seen in the dry season (). The Mann–Kendall test conducted, shown by the black asterisk where statistically significant trend exists, confirmed that trend in dry season is statistically significant while the decreasing trend reported for the rainy season cannot be said to be significant at 95% confidence interval.
For the mid-century time slice, the highest positive trend is seen in the rainy season for both RCP 4.5 and 8.5 though RCP 8.5 shows values which are an order of magnitude lower than the values reported for the past time slice. In other words, most trend values in the mid-century are in the order of 10−4 compare to the 10−3 seen in the past. Additionally, this is in contrast to what was seen for 1979–2005, where dry season trends are higher. Also, the end century rainy season values are generally positive as can be seen in and . As for the dry season, the reverse is the case with majority grid points showing negative trend values resulting in an overall negative mean trend. The seemingly west to east decrease in the trend as well as the previously reported lowest trend value region in the Niger delta-Cameroon axis was also captured by the future projections. The obvious absence of the asterisk signifying the locations where statistically significant trend was found by the Mann–Kendal test suggest that the trend in the future time slice cannot be adjudged to be significant at 95% confidence level. However, these values are higher for the end century time slice compared to the mid-century though less than the rates seen in the past. This suggests a reduction in the rates of change in WPD by mid-century which will pick up towards the end of the 21st century again.
The trend analysis, similar to the spatiotemporal variations of the average WPD, demonstrates a comparable distribution pattern to the wave climate. It is important to note that the applied linear regression method takes into account distinct periods: 1979–2005, 2026–2045, and 2081–2100. This approach ensures an independent assessment of trends for the historical, mid-century, and end-century eras respectively. As a result, the outcomes remain representative of the observed patterns and rate of change, whether it’s an increase or decrease, within each time frame. Regarding future time spans, the analysis indicates a projected weakening of wave heights during the dry season, leading to reduced WPD. Conversely, the anticipated strengthening of wave conditions during rainy seasons corresponds to an increase in WPD for both mid- and end-century periods ().
4.3 Projected future change in WPD
As shown in , the average projected change in overall WPD between the past and the future ranges between 0.2 and 0.5 kW/m with the largest change seen in the RCP 8.5 climate change scenario. This same pattern is seen in the rainy season though with higher magnitudes ranging from 0.5 to 1.0 kW/m. During the dry season, the WPD is projected to reduce from the past to the future in all future RCP scenarios.
Figure 7. Averages projected changes in WPD by mid- and end-century for overall, dry season and rainy season.

The ANOVA equality of means test conducted and depicted in the boxplots in with tags ‘_a’ for overall average, ‘_s’ for rainy and ‘_w’ for dry seasons, respectively. showed that the historical time slice has a WPD that can be judged to be statistically significant from the future RCP projections in most cases. This is easily explainable from the absence of overlap between the past and the future especially during the rainy season. Though the projection based on RCP 8.5 for the end-century time slice seems to be different from the other future scenarios, the others can be seen to have overlaps in most cases.
Figure 8. Boxplots showing differences in averages WPD for all time slices and seasons (black=past time slice, blue=future RCP 4.5 and red=future RCP 8.5).

Performance assessment of different WECs in the GoG
In order to see the expected electricity output distribution, the wave climate occurrences are presented as a binned joint distribution of Hs and Tm divided into cells of 0.5 m x 0.5 s respectively in the case of Wave Dragon. However, the binning of the other two WECs were structured into 0.5 m × 0.5 s to conform with the power matrix. The colour shading of each cell represents the total power output from that bin. For a basin-wide and temporally representative overview, every point where data exists in the GoG was taken into account for all the years. An overview of the contribution from different bins was assessed using the Wave Dragon () and Aqua buoy () systems for the past time slice. The overall result obtained for the period 1979–2005 showed that about 70% of the wave power generation in the GoG comes from wave heights of 1 m and 9 s. This corresponds to an average of 3363 and 57.9 MW per year if the total shown in are divided by 27 for Wave Dragon and Aqua buoy, respectively. This same distribution is seen for the dry season with the attributed percentage of 80% though less power was generated during this period with an average of 2935.4 and 40.2 MW per year. The rainy season showed a slight difference in percentage of contribution with a more even distribution of the power output from different bins.
Figure 9. Bivariate distributions of the total wave power output with the corresponding Hs and Tm the period 1979–2005 for wave Dragon (a) overall (b) dry (c) rainy and Aqua buoy (d) overall (e) dry (f) rainy.

The annual and seasonal total power output projected for the 3 WECs summarised in showed that the magnitude varies widely based on deployed WECs. Wave Dragon showed highest expected output up to 178 GW during the rainy season for all the locations in the GoG in the last 20 years of the 21st century. This is an average of 14.2 MW per time for every grid cell if divided by the number of points (625) included in the study.
Table 3. Projected wave power output (MW) from 3 WECs for different RCP scenarios.
In order to see the performance of the WECs from one point to the other in the GoG, the power output was estimated for every grid point for the different WECs. This output was used to estimate the normalised non-dimensional electric power from one location to another to identify hotspots of power generation. This was used to show region where the WEC is performing better in generating power relative to other locations. The results showed higher values close to 1 offshore suggesting the best locations for most profitable exploitation of wave energy.
The capture widths for the different WECs showed that the Wave Dragon has values which are two orders of magnitude higher than both of Aqua buoy and Pelamis (). This is not surprising as similar result is shown by the power output which is a result of the power matrix and power ratings of each system. The Wave Dragon also showed higher values for the capacity factor () with values ranging between 2 and 8 compared toothers 1–4.
5. Discussion
The wave conditions in the Gulf of Guinea, as outlined in the results section, corroborate previous reports on the region’s maritime environment. During the dry season, the sea experiences relatively calm conditions, a phenomenon attributed to the strengthening of trade winds from the northeast. This seasonal pattern aligns with established climatic patterns in the area. Conversely, the rainy season is marked by the prevalence of the Intertropical Convergence Zone (ITCZ), which can trigger increased storm activity and, consequently, higher wave heights. This connection between the ITCZ and wave height variations has been documented in previous research (Almar et al. Citation2015; Bird Citation2008). It is noteworthy that the average wave height of 1.36 metres and wave period of 9.6 seconds, as reported by Almar et al. (Citation2015), falls within the annual range of wave heights (0.34–1.60 m) and wave periods (5.86–10.23 s) identified in this study. This consistency in findings underscores the reliability and alignment of the results with existing knowledge of wave conditions in the Gulf of Guinea.
The relatively low wave heights observed in the Niger Delta-Cameroon region of the Gulf of Guinea can be attributed to a combination of factors, including the influence of geographical features and wind patterns. These factors work together to create a subdued wave climate in this particular area. Firstly, the presence of offshore islands like Sao Tome and Principe plays a role in sheltering the region from the full force of incoming waves. These islands act as barriers, causing waves to break and lose energy as they approach the coastline. This dampening effect contributes to the overall reduction in wave height. Secondly, the wide continental shelf in this section of the GoG also plays a significant role. A wide continental shelf allows waves to propagate over a larger area, which can result in energy dissipation. As waves travel across this extensive shelf, they tend to lose some of their energy, leading to lower wave heights along the coast.
Additionally, the orientation of the coastline relative to the prevailing wave and wind directions is a crucial factor. Coastal regions that align more closely with the prevailing winds, such as the northwesternmost part of the GoG, are more exposed to wind-driven wave generation. This exposure can lead to higher wave heights in these areas compared to regions with a more sheltered orientation (Dahunsi et al. Citation2022). In other words, the subdued wave heights observed in the Niger Delta-Cameroon section of the GoG are the result of a complex interplay of geographical features, continental shelf characteristics, and prevailing wind patterns. These factors collectively contribute to the unique wave climate experienced in this part of the Gulf.
The relative north-south decreasing pattern in Tm observed in the GoG can be attributed to the influence of distant swell systems originating from both the North Atlantic and the South Atlantic oceans. These swell systems carry waves with varying periods as they propagate across the open ocean (Forristall et al. Citation2013). This influence is particularly pronounced during the dry season when the North Atlantic swell system dominates the wave climate. The northwesternmost part of the GoG is more exposed to the effects of the North Atlantic swell, which pushes waves with longer periods closer to the easternmost coast of the Gulf. This leads to a noticeable north-south gradient in wave periods, with longer wave periods in the north and shorter periods in the south.
The east-west variation in wave period within the GoG is primarily shaped by the presence of coastal features such as headlands, bays, and inlets. These coastal features can cause wave refraction, diffraction, and reflection, resulting in variations in wave period along the coast. However, it’s important to note that the relationship between coastal features and wave period is not always straightforward, as the influence of other factors, like the South Atlantic swell, can sometimes overshadow the impacts of coastal morphology. Therefore, the complex interplay of distant swell systems, prevailing wind patterns, and coastal features contributes to the spatial and temporal variations in wave period observed in the GoG. These factors collectively shape the unique wave climate of the region.
The analysis conducted in this study consistently reveals a trend of increasing Hs in the GoG across all RCP scenarios. This observed trend aligns with broader expectations of climate change impacts, where warmer ocean temperatures and altered atmospheric conditions are anticipated to result in changes in wind patterns and ocean dynamics, consequently influencing the generation and propagation of ocean waves (De Leo, Besio, and Mentaschi Citation2021; Hemer et al. Citation2013).
The projected increase in Hs by the end of the century, especially under the RCP 8.5 scenario with higher greenhouse gas emissions, is in line with findings from previous global studies. This scenario indicates the most significant increase in wave heights, reflecting the potential intensification of extreme weather events and storms associated with climate change. Notably, the higher increase in wave heights during the rainy season further underscores the influence of enhanced atmospheric activity, such as storms, on wave conditions in the future. Additionally, the observation of a projected increase in Tm suggests that not only will wave heights increase, but the waves will also exhibit longer intervals between crests (Dahunsi et al. Citation2022). This implies a potential shift in the distribution of wave energy within the GoG. These findings collectively highlight the dynamic and interconnected nature of climate change impacts on ocean waves, emphasising the need for continued research and monitoring in understanding and adapting to these changes.
The magnitude of the average WPD in the GoG follows a distribution pattern consistent with findings from previous model dataset-based studies, particularly regarding the average and extreme wave climates, with a focus on Hs including Almar et al. (Citation2015 and Osinowo et al. (Citation2018). This observed pattern indicates a trend of increasing WPD from north to south and from east to west within the GoG, mirroring the spatial distribution reported for Hs in the preceding section. The north-south and east-west increase in WPD can be attributed to the complex interplay of various factors, including swell systems, local wind regimes, and bathymetry. This distribution pattern aligns with the regional wave climate overview presented by Dahunsi et al. (Citation2022), which is not surprising given the direct proportionality between WPD and Hs, as evident in the equation used for WPD computation. A correlation test conducted to assess the strength of this relationship yielded a high correlation coefficient of 0.98, indicating a strong association between WPD and Hs. Consequently, changes in wave height are expected to correspond to similar changes in WPD, a relationship supported by a similar study along the southeastern coast of the United States conducted by Defne et al. (Citation2009). Furthermore, the observation of consistent overlaps in WPD values for different scenarios, as previously reported for the wave climate of the GoG by Dahunsi et al. (Citation2022), prompted the conduct of statistical tests to assess significant differences in this previous study. Ultimately, their findings concluded that these differences were not statistically significant, further reinforcing the interconnected nature of wave height and WPD within the GoG and the robustness of the observed patterns.
Furthermore, the transition from lower WPD values during the dry season to higher values in the rainy season mirrors the seasonal variability observed in wave energy, akin to the patterns in the wave climate. During the rainy season, more vigorous weather conditions prevail, characterised by elevated wind speeds and extended fetch (the distance over which the wind influences wave formation). These conditions result in greater energy transfer to the waves, leading to the observed increase in WPD. This alignment underscores the strong connection between wave energy and atmospheric conditions, highlighting the pivotal role of seasonal weather patterns in influencing wave power dynamics. It is worth noting that this consistent trend of seasonal variations in WPD persists across various time slices and RCP scenarios, albeit with varying magnitudes. This consistency underscores the robustness of the findings and demonstrates the predictability of wave power dynamics under different climate scenarios.
The negative trend in WPD identified for the mid-century period aligns with findings reported by other studies in different regions. Ribeiro et al. (Citation2020), for instance, observed a similar reduction in wave power in their assessment of wave energy converters in the North Atlantic. Likewise, Karunarathna et al. (Citation2020) documented a 12–20% decrease in wave power along the coasts of Sri Lanka by mid-century, attributing it to changes in the tropical monsoon system driven by global climate change. In the GoG, Almar et al. (Citation2015) associated alterations in the region’s wave climate, particularly the dominance of swell waves, with the impacts of climate change on the extratropical Southern Annular Mode (SAM). This mode influences storm conditions in the southern Atlantic extra-tropical storm track, which contributes to the swell waves experienced in the GoG. Furthermore, global studies by Lobeto et al. (Citation2021) and Meucci et al. (Citation2020) have also reported a reduction in wave power during the mid-century, followed by an increasing trend towards the end of the century for the tropical Atlantic Ocean, where the GoG is located. Given the high correlation between Hs and WPD, it is reasonable to expect similar impacts of climate change on both parameters in the GoG.
The estimated values for the Gulf of Guinea (GoG) region during the past time slice align with findings from similar studies in the area. For instance, Tulashie et al (Citation2022) reported WPD values ranging from 7.5 to 10.5 kW/m for the Ghanaian coast, which closely resembles the values exceeding 7.5 kW/m observed along the western coast of the GoG. As one moves eastward towards the Niger Delta, Osinowo (Citation2019) affirmed the presence of very low WPD, generally less than 4 kW/m, in this part of the GoG. These studies also corroborated the seasonal variability in wave conditions and WPD, with higher Hs during the rainy season leading to increased WPD magnitude. According to Wei Zheng and Pan (Citation2014), wave energy is considered available when the WPD exceeds 2 kW/m and rich when it surpasses 20 kW/m. Based on this criterion, it can be confidently stated that the GoG boasts abundantly available wave energy resources throughout the year, even during the low-energy dry season.
The spatial distribution of wave power potential in the Gulf of Guinea, as previously illustrated in , emphasises the need for an offshore-focused approach in harnessing this abundant renewable energy resource. Such a strategy would enhance the economic feasibility of exploitation and mitigate the impacts of seasonal variations in wave power within the region. Additionally, it would expand the available sites for the installation of wave energy converters. The Gulf of Guinea encompasses a vast area, approximately 2,350,000 square kilometres, including small islands, offshore oil rigs, and marine protected areas, as documented by Osinowo et al. (Citation2018). To provide a conservative estimate, let’s assume a parallel orientation of wave energy converters along half of the coastlines of the countries surrounding the Gulf of Guinea. This would result in an average of 1500 kilometres of potential sites for energy harvesting. Based on this average coverage, the estimated potential for wave power generation during the low-energy dry season is approximately 8190 MW, calculated using the minimum WPD value of 5.46 kW/m obtained for the dry season (as presented in ). However, with the utilisation of the appropriate type of wave energy converter, this potential could soar to as high as 14,940 MW, taking into account the projected WPD value of 9.96 kW/m for the rainy season by the end of the century.
However, in order to instal WECs for effective exploitation of wave energy in the GoG, several key performance indicators (KPIs) that play pivotal roles in the feasibility and potential benefits of the project should be considered. These KPIs are needed to determine the post-installation economic metrics such as LCOE, NPV, IRR etc previously highlighted in the introductory section. Judging from the previously presented wave characteristics of the GoG including the dry and rainy seasons variabilities and the emphasis on relatively highly available WPD offshore, important KPIs include power output which helps to determine the total energy output generated by the WEC over the course of a year taking into account the diverse wave conditions experienced during both dry and rainy seasons. Another critical KPI is Energy Conversion Efficiency, which quantifies how effectively the WEC harnesses the kinetic energy of waves and converts it into usable electricity. This efficiency is a fundamental indicator of the WEC’s technological prowess and its ability to make the most of the available wave resources. The Capacity Factor further refines the assessment by considering the proportion of actual energy output to the maximum energy generation potential under ideal conditions. Reflecting both fluctuations in wave energy and operational downtime for maintenance or other reasons, the Capacity Factor speaks to the practicality of WEC deployment. In tandem with these indicators, understanding the Power Capture Width becomes essential. This parameter delineates the spectrum of wave frequencies that the WEC can efficiently capture. Given the variation in wave characteristics between seasons, this adaptability becomes crucial in ensuring consistent energy production.
The results for the three WECs considered in this study indicate that the Wave Dragon stands out with higher power output and a larger capture width, which is advantageous for a vast region like the GoG. These findings align with similar cases reported for other regions, reinforcing the effectiveness of the Wave Dragon design. For instance, studies conducted on European islands, as assessed by Rusu and Onea (Citation2016), and on the island of Fuerteventura, as investigated by Veigas and Iglesias (Citation2014), have yielded comparable results. These findings demonstrate the suitability of the Wave Dragon WEC for harnessing wave energy efficiently in various geographical contexts, making it a promising choice for the Gulf of Guinea region. The consistency of these results across different regions highlights the robust performance and adaptability of the Wave Dragon WEC, further emphasising its potential as a viable solution for renewable energy generation in coastal areas with varying wave conditions.
As previously discussed, and corroborated by both the bivariate distribution of wave power in the GoG and the normalised power output depicted in , it becomes evident that the offshore regions characterised by wave heights around 1 m and periods of 9 s represent the most reliable and efficient locations for the installation of any of the selected WECs. This spatial distribution of optimal wave energy exploitation remains consistent for all three WECs considered in this study, despite variations in wave output magnitude. Seasonal variations also exhibit a similar pattern, with increased efficiency closer to the coast during the rainy season. However, most locations demonstrate an efficiency rate exceeding 50%, with the exception of the Cameroon axis, known for its lower wave energy levels throughout the year. The efficiency values observed in this study fall within the range of 0.86 to 0.96, a range previously reported by Rusu and Onea (Citation2016). These findings emphasise the reliability and consistent performance of the selected WECs in effectively harnessing wave energy across various geographical areas within the GoG, making them promising options for renewable energy generation in this region.
In addition to the significant benefits of power generation and other socio-economic advantages that can be derived from the installation of WEC systems in the GoG, there is also the potential to address the pressing issue of coastal erosion faced by all the countries within the GoG region. This additional benefit has been highlighted in the work of Veigas and Iglesias (Citation2014), who conducted modelling studies to assess the impact of WECs on the nearshore wave climate. Their findings revealed that the presence of WECs led to a reduction in wave height in the regions where these systems were installed. This reduction in wave height subsequently resulted in a decrease in the energy reaching the nearshore areas, leading to lower rates of coastal erosion. This discovery underscores the potential for WEC installations not only to provide clean and sustainable energy but also to contribute positively to the mitigation of coastal erosion, offering a holistic solution to some of the environmental challenges faced by the GoG countries.
6. Conclusion
In conclusion, this comprehensive study has provided valuable insights into the past and potential future wave power conditions within the GoG. The spatial distribution of average WPD has revealed consistent trends in WPD patterns across various time frames and climate scenarios. The study has shed light on the seasonal variations and the intricate relationship between WPD, Hs, and atmospheric conditions, highlighting the complex interplay of oceanic and atmospheric factors that influence wave energy potential in the region. These findings offer critical information for understanding the feasibility of harnessing wave energy in the Gulf of Guinea and for formulating sustainable energy strategies tailored to the region’s unique characteristics. The projections presented here will serve as fundamental data for future initiatives involving the installation of wave energy converters, helping to identify the most economically and environmentally viable locations for these energy harvesting systems.
Notably, this study underscores that ocean wave energy holds significant promise as a source of electric power generation to meet the increasing energy demands of the countries within the Gulf of Guinea. The assessment has clearly indicated that offshore locations are the most promising for wave energy converter installations, as wave power potential diminishes significantly closer to the coastline, making nearshore exploitation economically unviable. Additionally, given the ecological significance of the Guinea Current Large Marine Ecosystem in the region, it becomes imperative to minimise the environmental impact of energy installations in coastal upwelling areas.
Furthermore, among the wave energy converter systems considered, the Wave Dragon has demonstrated superior performance across all key indicators, making it a compelling candidate for deployment in the Gulf of Guinea to maximise the utilisation of the region’s abundant wave energy resources. This research paves the way for informed decision-making and sustainable energy development in the Gulf of Guinea, contributing to both energy security and environmental conservation efforts.
Furthermore, this study has laid a crucial foundation for exploring additional potential benefits beyond electricity generation, particularly in the realm of coastal erosion mitigation. Coastal erosion represents one of the most pressing challenges faced by many countries situated along the GoG. The capacity of WECs to mitigate coastal erosion, as previously investigated by Veigas and Iglesias (Citation2014), offers a multifaceted approach that can deliver significant economic advantages to GoG nations. Traditional methods of coastal protection, such as seawalls and groynes, often come at the cost of altering natural beach landscapes, thereby diminishing their appeal as tourist destinations. In contrast, leveraging WECs to dampen wave energy while preserving the integrity of beaches holds substantial promise. This approach not only contributes to electricity generation but also safeguards the income-generating potential of these coastal regions. Importantly, it offers a sustainable solution that remains effective in the face of anticipated increases in wave intensity driven by climate change. To delve deeper into these prospects, future studies are expected to employ dynamic modelling approaches, encompassing wave simulation and behaviour analysis under various WEC setup scenarios. By adopting such comprehensive methodologies, we can further unlock the synergistic potential of wave energy utilisation for both sustainable energy generation and coastal protection in the Gulf of Guinea.
Author contributions
A.M.D.: conceptualisation; data curation; methodology; validation; visualisation; writing – original draft; writing – review and editing. B.A.K.: methodology; validation; writing – reviewing and editing.
Supplemental Material
Download MS Word (14.2 KB)Disclosure statement
No potential conflict of interest was reported by the author(s).
Data availability statement
The Coordinated Ocean Wave Climate Project phase 2.0 (COWCLIP2.0) wave climate database used in this study is publicly available at https://cowclip.org/data-access/ (Accessed: 20 July 2022).
Supplementary material
Supplemental data for this article can be accessed online at https://doi.org/10.1080/19397038.2023.2269204
Additional information
Funding
Notes on contributors
Adeola M. Dahunsi
Adeola Michael Dahunsi is a physical oceanographer with a special interest in coastal hydrodynamics especially its evolution under climate change. His research interest covers the study of both living and non-living resources in the coastal and marine environment. This interest is inspired by the desire to contribute to the data-driven sustainable management of these resources in the Gulf of Guinea (GoG) region. Therefore, he continues to work on research increasing data availability in the data-scarce region of the GoG.
Bennet Atsu Kwame Foli
Bennet Atsu Kwame Foli, an accomplished Oceanographer and Earth Observation Analyst, boasts over a decade of professional expertise in Marine and Fisheries Sciences, with a focus on remote sensing and geospatial data analysis. Holding advanced degrees, including a Ph.D. in Marine Science, Bennet excels in ocean wave analysis, modelling, and early warning system development. His dedication to the field is evident in his contribution to international projects and mentorship programs, highlighting his strong publication record and commitment to training and capacity development. During his tenure as an Oceanographer at the University of Ghana, Bennet played a vital role in enhancing coastal vulnerability and ocean state early-warning services. He actively engages stakeholders, showcasing strong communication skills and a proactive approach to problem-solving. Bennet's expertise was pivotal in securing funding for the GMES & Africa phase-2 project, highlighting his grant proposal writing and project execution abilities. Bennet's technical skills include GIS and satellite data application, MATLAB programming, and the use of Unmanned Aerial Vehicles (UAVs) for environmental monitoring. Beyond his professional pursuits, he finds joy in photography and DIY electronics. Overall, Bennet stands as a dedicated and innovative professional in the field of Oceanography and Earth observation, contributing significantly to environmental research and sustainability.
References
- Abe, J., and B. E. Brown. 2020. “Towards a Guinea Current Large Marine Ecosystem Commission.” Environmental Development 36:100590. https://doi.org/10.1016/j.envdev.2020.100590.
- Adesanya, A., S. Misra, R. Maskeliunas, and R. Damasevicius. 2020. “Prospects of Ocean-Based Renewable Energy for West Africa’s Sustainable Energy Future.” Smart & Sustainable Built Environment 10 (1): 37–50. https://doi.org/10.1108/SASBE-05-2019-0066.
- Almar, R., E. Kestenare, J. Reyns, J. Jouanno, E. J. Anthony, R. Laibi, M. Hemer, Y. Du Penhoat, and R. Ranasinghe. 2015. “Response of the Bight of Benin (Gulf of Guinea, West Africa) Coastline to Anthropogenic and Natural Forcing, Part1: Wave Climate Variability and Impacts on the Longshore Sediment Transport.” Continental Shelf Research 110:48–59. https://doi.org/10.1016/j.csr.2015.09.020.
- Alves, B., D. B. Angnuureng, P. Morand, and R. Almar. 2020. “A Review on Coastal Erosion and Flooding Risks and Best Management Practices in West Africa: What Has Been Done and Should Be Done.” Journal of Coastal Conservation 24 (3): 38. https://doi.org/10.1007/s11852-020-00755-7.
- Alves, J.-H. G. M., A. Chawla, H. L. Tolman, D. Schwab, G. Lang, and G. Mann. 2014. “The Operational Implementation of a Great Lakes Wave Forecasting System at NOAA/NCEP.” Weather and Forecasting 29 (6): 1473–1497. https://doi.org/10.1175/WAF-D-12-00049.1.
- Angnuureng, D. B., R. Almar, K. Appeaning Addo, B. Castelle, N. Senechal, S. W. Laryea, and G. Wiafe. 2016. “Video Oberservation of Waves and Shoreline Change on the Microtidal James Town Beach in Ghana.” Journal of Coastal Research 75 (sp1): 1022–1026. https://doi.org/10.2112/SI75-205.1.
- Angnuureng, D. B., P.-N. Jayson-Quashigah, R. Almar, T. C. Stieglitz, E. J. Anthony, D. W. Aheto, and K. A. Addo. 2020. “Application of Shore-Based Video and Unmanned Aerial Vehicles (Drones): Complementary Tools for Beach Studies.” Remote Sensing 12 (3): 394. https://doi.org/10.3390/rs12030394.
- Arnaud, K. K., F. Bonou, Z. Sohou, D. B. Angnuureng, and R. Almar. 2021. “Evaluation of Hydromorphological Conditions of Grand Popo Beach Using Two Unique Video Cameras.” Interpretation 9 (4): SH57–SH66. https://doi.org/10.1190/INT-2021-0023.1.
- Babarit, A., J. Hals, M. J. Muliawan, A. Kurniawan, T. Moan, and J. Krokstad. 2012. “Numerical Benchmarking Study of a Selection of Wave Energy Converters.” Renewable Energy 41:44–63. https://doi.org/10.1016/j.renene.2011.10.002.
- Bird, E. C. F. 2008. Coastal Geomorphology: An Introduction. New York, USA: John Wiley & Sons.
- Booij, N., L. Holthuijsen, and J. Battjes. 2001. “Ocean to Near-Shore Wave Modelling with SWAN.” Coastal Dynamics’ 1:335–344. https://doi.org/10.1061/40566(260)34.
- Bricheno, L., H. Cannaby, T. Howard, K. McInnes, M. Palmer, A. K. M. Saiful Islam, and A. Haque. 2015. “Extreme Sea Level Projections.” Environmental Science Processes & Impacts 17 (7): 1311–1322. https://doi.org/10.1039/c4em00683f.
- Cabral, T., D. Clemente, P. Rosa-Santos, F. Taveira-Pinto, T. Morais, F. Belga, and H. Cestaro. 2020. “Performance Assessment of a Hybrid Wave Energy Converter Integrated into a Harbor Breakwater.” Energies 13 (1): 236. https://doi.org/10.3390/en13010236.
- Chen, T., Q. Zhang, Y. Wu, C. Ji, J. Yang, and G. Liu. 2018. “Development of a Wave-Current Model Through Coupling of FVCOM and SWAN.” Ocean Engineering 164:443–454. https://doi.org/10.1016/j.oceaneng.2018.06.062.
- Ciappi, L., I. Simonetti, A. Bianchini, L. Cappietti, and G. Manfrida. 2022. “Application of Integrated Wave-To-Wire Modelling for the Preliminary Design of Oscillating Water Column Systems for Installations in Moderate Wave Climates.” Renewable Energy 194:232–248. https://doi.org/10.1016/j.renene.2022.05.015.
- Coe, R. G., G. Lavidas, G. Bacelli, P. H. Kobos, and V. S. Neary. 2022. “Minimizing Cost in a 100% Renewable Electricity Grid: A Case Study of Wave Energy in California.” International Conference on Offshore Mechanics and Arctic Engineering Hamburg, Germany 85932: V008T09A073.
- Cuttler, M. V. W., J. E. Hansen, and R. J. Lowe. 2020. “Seasonal and Interannual Variability of the Wave Climate at a Wave Energy Hotspot off the Southwestern Coast of Australia.” Renewable Energy 146:2337–2350. https://doi.org/10.1016/j.renene.2019.08.058.
- Dahunsi, A. M., F. F. Bonou, O. A. Dada, and E. Baloïtcha. 2022. “Spatio-Temporal Trend of Past and Future Extreme Wave Climates in the Gulf of Guinea Driven by Climate Change.” Journal of Marine Science and Engineering 10 (11): 1581. https://doi.org/10.3390/jmse10111581.
- Dalton, G. J., R. Alcorn, and T. Lewis. 2010. “Case Study Feasibility Analysis of the Pelamis Wave Energy Convertor in Ireland, Portugal and North America.” Renewable Energy 35 (2): 443–455. https://doi.org/10.1016/j.renene.2009.07.003.
- Defne, Z., K. A. Haas, and H. M. Fritz. 2009. “Wave Power Potential Along the Atlantic Coast of the Southeastern USA.” Renewable Energy 34 (10): 2197–2205. https://doi.org/10.1016/j.renene.2009.02.019.
- De Leo, F., G. Besio, and L. Mentaschi. 2021. “Trends and Variability of Ocean Waves Under RCP8.5 Emission Scenario in the Mediterranean Sea.” Ocean Dynamics 71 (1): 97–117. https://doi.org/10.1007/s10236-020-01419-8.
- Foli, B. A. K., K. Appeaning Addo, J. K. Ansong, and G. Wiafe. 2022. “Ocean State Projections: A Review of the West African Marine Environment.” Journal of Coastal Conservation 26 (6): 1–14. https://doi.org/10.1007/s11852-022-00908-w.
- Forristall, G. Z., K. Ewans, M. Olagnon, and M. Prevosto. 2013. “The West Africa Swell Project (WASP).” Proceedings of the International Conference on Offshore Mechanics and Arctic Engineering - OMAE 2 B (June). https://doi.org/10.1115/OMAE2013-11264.
- Grigorieva, V. G., S. K. Gulev, and A. V. Gavrikov. 2017. “Global Historical Archive of Wind Waves Based on Voluntary Observing Ship Data.” Oceanology 57 (2): 229–231. https://doi.org/10.1134/S0001437017020060.
- Guillou, N., and G. Chapalain. 2018. “Annual and Seasonal Variabilities in the Performances of Wave Energy Converters.” Energy 165:812–823. https://doi.org/10.1016/j.energy.2018.10.001.
- Guillou, N., G. Lavidas, and G. Chapalain. 2020. “Wave Energy Resource Assessment for Exploitation—A Review.” Journal of Marine Science and Engineering 8 (9): 705. https://doi.org/10.3390/jmse8090705.
- Hasselmann, S., K. Hasselmann, E. Bauer, P. Janssen, G. J. Komen, L. Bertolli, P. Lionello, A. Guillaume, V. J. Cardone, and J. A. Greenwood. 1988. “The WAM Model—A Third Generation Ocean Wave Prediction Model.” The WAM Model—A Third Generation Ocean Wave Prediction Model Journal of physical oceanography 18 (12): 1775–1810. https://doi.org/10.1175/1520-0485(1988)018<1775:TWMTGO>2.0.CO;2.
- Hemer, M. A., Y. Fan, N. Mori, A. Semedo, and X. L. Wang. 2013. “Projected Changes in Wave Climate from a Multi-Model Ensemble.” Nature Climate Change 3 (5): 471–476. https://doi.org/10.1038/nclimate1791.
- Hemer, M. A., and C. E. Trenham. 2016. “Evaluation of a CMIP5 Derived Dynamical Global Wind Wave Climate Model Ensemble.” Ocean Modelling 103:190–203. https://doi.org/10.1016/j.ocemod.2015.10.009.
- Henriques, J. C. C., J. C. C. Portillo, W. Sheng, L. M. C. Gato, and A. F. D. O. Falcão. 2019. “Dynamics and Control of Air Turbines in Oscillating-Water-Column Wave Energy Converters: Analyses and Case Study.” Renewable and Sustainable Energy Reviews 112:571–589. https://doi.org/10.1016/j.rser.2019.05.010.
- Hughes, M. G., and A. D. Heap. 2010. “National-Scale Wave Energy Resource Assessment for Australia.” Renewable Energy 35 (8): 1783–1791. https://doi.org/10.1016/j.renene.2009.11.001.
- Kamranzad, B., A. Etemad-Shahidi, and V. Chegini. 2013. “Assessment of Wave Energy Variation in the Persian Gulf.” Ocean Engineering 70:72–80. https://doi.org/10.1016/j.oceaneng.2013.05.027.
- Karunarathna, H., P. Maduwantha, B. Kamranzad, H. Rathnasooriya, and K. De Silva. 2020. “Impacts of Global Climate Change on the Future Ocean Wave Power Potential: A Case Study from the Indian Ocean.” Energies 13 (11): 3028. https://doi.org/10.3390/en13113028.
- Lehmann, M., F. Karimpour, C. A. Goudey, P. T. Jacobson, and M.-R. Alam. 2017. “Ocean Wave Energy in the United States: Current Status and Future Perspectives.” Renewable and Sustainable Energy Reviews 74:1300–1313. https://doi.org/10.1016/j.rser.2016.11.101.
- Liang, B., F. Fan, Z. Yin, H. Shi, and D. Lee. 2013. “Numerical Modelling of the Nearshore Wave Energy Resources of Shandong Peninsula, China.” Renewable Energy 57:330–338. https://doi.org/10.1016/j.renene.2013.01.052.
- Lobeto, H., M. Menendez, and I. J. Losada. 2021. “Future Behavior of Wind Wave Extremes Due to Climate Change.” Scientific Reports 11 (1): 1–12. https://doi.org/10.1038/s41598-021-86524-4.
- Meucci, A., I. R. Young, M. Hemer, E. Kirezci, and R. Ranasinghe. 2020. “Projected 21st Century Changes in Extreme Wind-Wave Events.” Science Advances 6 (24): 1–10. https://doi.org/10.1126/sciadv.aaz7295.
- Mirzaei, A., F. Tangang, and L. Juneng. 2014. “Wave Energy Potential Along the East Coast of Peninsular Malaysia.” Energy 68:722–734. https://doi.org/10.1016/j.energy.2014.02.005.
- Moretti, G., M. S. Herran, D. Forehand, M. Alves, H. Jeffrey, R. Vertechy, and M. Fontana. 2020. “Advances in the Development of Dielectric Elastomer Generators for Wave Energy Conversion.” Renewable and Sustainable Energy Reviews 117:109430. https://doi.org/10.1016/j.rser.2019.109430.
- Morim, J., M. Hemer, N. Cartwright, D. Strauss, and F. Andutta. 2018. “On the Concordance of 21st Century Wind-Wave Climate Projections.” Global and Planetary Change 167:160–171. https://doi.org/10.1016/j.gloplacha.2018.05.005.
- Morim, J., C. Trenham, M. Hemer, X. L. Wang, N. Mori, M. Casas-Prat, A. Semedo, T. Shimura, B. Timmermans, and P. Camus. 2020. “A Global Ensemble of Ocean Wave Climate Projections from CMIP5-Driven Models.” Scientific Data 7 (1): 1–10. https://doi.org/10.1038/s41597-020-0446-2.
- Mwasilu, F., and J. W. Jung. 2019. “Potential for Power Generation from Ocean Wave Renewable Energy Source: A Comprehensive Review on State-Of-The-Art Technology and Future Prospects.” IET Renewable Power Generation 13 (3): 363–375. https://doi.org/10.1049/iet-rpg.2018.5456.
- Osinowo, A. A. 2019. “Combined Exploitation of Onshore Wind-Wave Energy in the Niger Delta Coasts Based on a 37-Year Hindcast Information.” Energy Management Research Journal 2 (1): 19–38.
- Osinowo, A. A., I. A. Balogun, and E. O. Eresanya. 2018. “Assessment of Wave Energy Resource in the Mid-Atlantic Based on 37-Year Numerical Hindcast Data.” Modeling Earth Systems and Environment 4 (3): 935–959. https://doi.org/10.1007/s40808-018-0484-3.
- Osinowo, A. A., E. C. Okogbue, E. O. Eresanya, and O. S. Akande. 2018. “Extreme Significant Wave Height Climate in the Gulf of Guinea.” African Journal of Marine Science 40 (4): 407–421. https://doi.org/10.2989/1814232X.2018.1542343.
- Ribeiro, A. S., M. deCastro, L. Rusu, M. Bernardino, J. M. Dias, and M. Gomez-Gesteira. 2020. “Evaluating the Future Efficiency of Wave Energy Converters Along the NW Coast of the Iberian Peninsula.” Energies 13 (14): 3563. https://doi.org/10.3390/en13143563.
- Rusu, E., and F. Onea. 2016. “Estimation of the Wave Energy Conversion Efficiency in the Atlantic Ocean Close to the European Islands.” Renewable Energy 85:687–703. https://doi.org/10.1016/j.renene.2015.07.042.
- Sawin, J. L., F. Sverrisson, K. Seyboth, R. Adib, H. E. Murdock, C. Lins, I. Edwards, M. Hullin, L. H. Nguyen, and S. S. Prillianto. 2016. Renewables 2017 Global Status Report.
- Simal, P. D., S. T. Ortega, B. Bas, N. Elginoz, R. G. Garcia, F. Del Jesus, E. Giannakis, A. Giannouli, P. Koundouri, and A. Moussoulides. 2017. “Socio-Economic Assessment of a Selected Multi-Use Offshore Site in the Atlantic.” The Ocean of Tomorrow: Investment Assessment of Multi-Use Offshore Platforms: Methodology and Applications 1 (56) :69–84. https://doi.org/10.1007/978-3-319-55772-4_5
- Swail, V., R. Jensen, B. Lee, J. Turton, J. Thomas, S. Gulev, M. Yelland, P. Etala, D. Meldrum, and W. Birkemeier. 2010. “Wave Measurements, Needs and Developments for the Next Decade.” Proceedings of The OceanObs 9 (2): 10. https://doi.org/10.5270/OceanObs09.cwp.87.
- Taylor, K. E., R. J. Stouffer, and G. A. Meehl. 2012. “An Overview of CMIP5 and the Experiment Design.” Bulletin of the American Meteorological Society 93 (4): 485–498. https://doi.org/10.1175/BAMS-D-11-00094.1.
- Tolman, H. L. 1991. “A Third-Generation Model for Wind Waves on Slowly Varying, Unsteady, and Inhomogeneous Depths and Currents.” Journal of Physical Oceanography 21 (6): 782–797. https://doi.org/10.1175/1520-0485(1991)021<0782:ATGMFW>2.0.CO;2.
- Tulashie, S. K., R. Odai, A. M. Dahunsi, S. Atisey, and J. Amenakpor. 2022. “Feasibility Study of Wave Power in Ghana.” International Journal of Sustainable Engineering 15 (1): 299–311. https://doi.org/10.1080/19397038.2022.2145384.
- Veigas, M., and G. Iglesias. 2014. “Potentials of a Hybrid Offshore Farm for the Island of Fuerteventura.” Energy Conversion and Management 86:300–308. https://doi.org/10.1016/j.enconman.2014.05.032.
- Vettor, R., and C. Guedes Soares. 2019. “Comparison of VOS and ERA-Interim Wave Data.” Proceedings of the International Conference on Offshore Mechanics and Arctic Engineering - OMAE, New York, NY: USA 3, V003T02A045. https://doi.org/10.1115/OMAE2019-95287
- Wan, Y., J. Zhang, J. Meng, and J. Wang. 2015. “A Wave Energy Resource Assessment in the China’s Seas Based on Multi-Satellite Merged Radar Altimeter Data.” Acta Oceanologica Sinica 34 (3): 115–124. https://doi.org/10.1007/s13131-015-0627-6.
- Wei Zheng, C., and J. Pan. 2014. “Assessment of the Global Ocean Wind Energy Resource.” Renewable and Sustainable Energy Reviews 33:382–391. https://doi.org/10.1016/j.rser.2014.01.065.
- Young, I. R., S. Zieger, and A. V. Babanin. 2011. “Global Trends in Wind Speed and Wave Height.” Science 332 (6028): 451–455. https://doi.org/10.1126/science.1197219.
- Yusov, M., J. Thwaites, A. Kurniawan, J. Orszaghova, and H. Wolgamot. 2021. “New Cost-Effectiveness Metric for Wave Energy Converters-Extensive Database and Comparison.” Proceedings of the 14th European Wave and Tidal Energy Conference (EWTEC) Plymouth, UK.