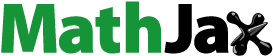
ABSTRACT
With global supply chains facing increasing complexity and uncertainty, mitigating sustainable supply chain risks (SSCRs) has become an important research topic. Industry 5.0 (I5.0) is a supplement to, and re-development of, Industry 4.0, but no research on improving supply chain resilience (SCRE) through I5.0 drivers to mitigate SSCRs currently exists. Therefore, we developed a QFD approach to investigate the relationship between SSCRs, SCRE, and I5.0 drivers. This study utilises the FDM-FDISM-ANP-TOPSIS method, considers China’s largest private logistics enterprises as the object of empirical analysis, and establishes a sustainable supply chain identification system and SCRE evaluation index system for logistics enterprises through two-stage House of Quality. The findings reveal that 20 key I5.0 significantly bolster 17 SCRE, thereby mitigating 13 SSCRs by QFD-MCDM framework. These I5.0 drivers play a pivotal role in enhancing SCRE of logistics enterprises. This study contributes to the field of supply chain risk resilience in sustainable engineering by integrating the perspectives of SSCRs, SCRE and I5.0. It underscores the necessity for managers in logistics enterprises to develop SCRE solutions that incorporate both SSCRs and I5.0 considerations. Furthermore, our findings offer valuable insights for other industries aiming to bolster their supply chain competitive advantages in an increasingly complex global landscape.
1. Introduction
In the present-day world, the escalating prominence of social issues stemming from air pollution, resource scarcity, and environmental degradation has piqued growing interest in sustainable development (Khan, Zhang, Kumar, et al. Citation2020; Syed et al. Citation2019). Scholars and researchers have witnessed a growing interest in sustainable research pertaining to supply chains (Khan, Zhang, Kumar, et al. Citation2020; X. Y. Xu et al. Citation2022). Integrating sustainability into supply chain operation management can help enterprises in realising market competitive advantages and planning key business strategies (Khan, Zhang, Kumar, et al. Citation2020; Koberg and Longoni Citation2018; H. C. Liu et al. Citation2019; Raut et al. Citation2019). Therefore, the implementation of sustainable supply chain management can bolster the competitive advantage of enterprises, improve their responsiveness to social and environmental uncertainties, and enhance both financial performance and economic benefits (Govindan and Gholizadeh Citation2021; Nikkhil, Manas, and Dibyajyoti Citation2021).
Improving supply chain resilience (SCRE) is an effective method of mitigating sustainable supply chain risks (SSCRs) (Hosseini, Ivanov, and Dolgui Citation2019; Kwak, Seo, and Mason Citation2018; J. Yang et al. Citation2021). As a dynamic capability, SCRE allows for a quick response to uncertain problems to gain market competitive advantages, thus effectively coping with supply chain risks in an uncertain environment (Ozdemir et al. Citation2022; N. Singh Citation2020; J. Um and Han Citation2021). Because frequent emergencies may damage the sustainable performance of the supply chain, effective SCRE can mitigate the detrimental effects of SSCRs (He et al. Citation2020). Several studies indicate that Industry 4.0 can improve SCRE and grant enterprises sustainable competitive advantages (Ralston and Blackhurst Citation2020). However, existing literature predominantly focuses on Industry 4.0, often overlooking the integration of Industry 5.0 (I5.0) in the study of supply chain resilience (SCRE). As a vital progression and enhancement of Industry 4.0, Industry 5.0 is instrumental in augmenting SCRE (Sindhwani et al. Citation2022). Notably, I5.0, representing the forefront of industrial research, is dedicated to catalysing industrial transformation towards sustainability, human-centricity, and resilience. This shift provides pivotal solutions for the efficient interaction within supply chains (Breque, Nul, and Petridis Citation2021). Mehdiabadi et al. (Citation2022) underscore that I5.0 prioritises the sustainability of SCRE, which is key to reducing production costs and invigorating supply chains. Consequently, to effectively navigate the risks and dynamics inherent in sustainable supply chains, it is imperative for logistics enterprises to devise SCRE strategies that incorporate both sustainable supply chain risks (SSCRs) and the principles of I5.0.
In recent years, quality function deploy-multiattribute decision-making has been successfully applied to solving the problem of green supply-chain selection (Yazdani et al. Citation2017), electronic manufacturing supply chain risks (Ralston and Blackhurst Citation2020), and supply chain traceability issues (Erol et al. Citation2022). Therefore, we developed a QFD approach to investigate the interrelationships among three groups of variables: sustainable supply chain risks (SSCRs), supply chain resilience (SCRE), and Industry 5.0 (I5.0) drivers. This study aims to establish an integrated QFD- MCDM framework. Utilizing two stages of the House of Quality (HoQ), we connect SSCRs, SCRE, and I5.0 drivers, ultimately prioritising sustainable supply chain risk resilience solutions for logistics enterprises. This study offers practical contributions by enabling logistics enterprises to implement flexible and sustainable supply chain risk resilience strategies. Additionally, stakeholders and participants involved in sustainable supply chain risk resilience are likely to find the outcomes of this research of significant interest. Therefore, the following issues will be discussed in this study:
What are the key drivers of SSCRs, SCRE, and I5.0 in the sustainable supply chain of a logistics enterprise?
How can the QFD-MCDM framework be integrated to connect three groups of variables and provide decision support for a logistics enterprise’s sustainable supply chain?
How can a logistics enterprise integrate I5.0 key drivers into SCRE and mitigate SSCRs?
The remainder of this paper is organised as follows: Section 2 presents a literature review of SSCRs, SCRE, and I5.0. Section 3 introduces the construction of a QFD-MCDM integration framework. Section 4 conducts empirical research and analysis on the logistics enterprise. Finally, Section 5 presents the results, contributions, and future research directions.
2. Literature review
2.1. Sustainable supply chain risks
The investigation of SSCRs offers valuable insights for the sustainable development of enterprises (Abdel-Basset and Mohamed Citation2019), and researchers have pursued various research directions in the context of sustainability (Amin et al. Citation2022). Lee and Vachon (Citation2016) proposed that logistics and transportation connections, natural disasters, packaging waste, and other environmental issues disrupt sustainable supply chains. To address these disruptions, Valinejad and Rahmani (Citation2018) proposed a five-dimensional approach for evaluating SSCRs: encompassing the aspects of economy, society, environment, technology, and institutions. Zulfiquar et al. (Citation2020) proposed a fuzzy SWARA-fuzzy COPRAS framework to effectively identify and rank solutions aimed at mitigating risks in sustainable remanufacturing supply chains. Additionally, Moktadir et al. (Citation2021) applied this five-dimensional approach to examine 30 potential sustainable supply chain risk factors in the Bangladesh leather industry. Notably, the study of SSCRs has gained significant attention, and an increasing number of scholars and practitioners are devoted to addressing SSCRs.
The development of an integrated framework to address SSCRs is crucial for achieving sustainable supply chains (M. Xu et al. Citation2019). Enterprises with a risk identification and assessment framework can effectively prevent and respond to various risks. Therefore, we aim to build a comprehensive framework to achieve a sustainable supply chain. Accordingly, we initially discuss the classification of risk types as classified by various scholars in the recent five years. Liu et al. (Citation2018) divided risk into environment, quality, and operation dimensions. Ganguly and Kumar (Citation2019) divided them into supply, information, financial, and manufacturing risks. Babu et al. (Citation2021) considered nine categories: environmental, information technology, market, supply, delay, transportation, demand, delay, financial, and process risks. Tavakoli Haji Abadi and Avakh Darestani (Citation2021) considered eight types: economic, environmental, organisational, sustainable supply, sustainable production, sustainable distribution, sustainable recovery, and IT-related risks. Alshehri et al. (Citation2022) categorised SSCRs into seven categories: economic, manufacturing and operation, environmental, management policy, quality, market, and social risks.
From the aforementioned literature, 30 SSCRs factors in Appendix A were selected and classified into internal, cooperation, and external risks. Key SSCRs will be evaluated in Section 4 after fuzzy Delphi method (FDM) screening.
2.2. Supply chain resilience
SCRE is crucial for supply chain risk management and refers to the supply chain’s ability to restore its original state or transition to a more favourable state after disruptions (Glickmann and White Citation2006; Ponomarov and Holcomb Citation2009; Sheffi and Rice Citation2005). According to Hosseini et al. (Citation2019), SCRE is defined as an enterprise’s capacity to promptly respond to supply chain disruptions in order to meet customers’ evolving demands. Accordingly, enterprises can quickly respond to disturbances and maintain high perception through SCRE to realise market competitive advantages (Bui et al. Citation2020; Sá et al. Citation2020; Stone and Rahimifard Citation2018). Um and Kim (Citation2019) proposed the significance of SCRE in mitigating supply chain risks, enabling enterprises to sustain their competitiveness, enhance customer service benefits, and foster sustainable development.
Accordingly, over the past five years, researchers have examined various SCRE indicators. Stone et al. (Citation2018) proposed five core SCRE indicators: flexibility, redundancy, risk awareness culture, early warning, and safety. Singh et al. (Citation2019) categorised SCRE into multiple dimensions, including collaboration, sustainability, agility, redundancy, flexibility, visibility, information sharing, robustness, sensitivity, supply chain risk management culture, security, speed, adaptability, market position, risk control, public-private partnership, and supply chain network design. Cerchioneet al. (Citation2020) examined seven SCRE indicators: flexibility, redundancy, visibility, disaster preparedness, market capability, and financial capability. Bayramova et al. (Citation2021) divided SCRE into various components, including redundancy, collaboration, agility, flexibility, human resource management, risk aversion, and culture. Chrisandina et al. (Citation2022) proposed five crucial factors to enhance SCRE: rapid detection, response and recovery, end-to-end visibility and data-driven control, downsizing, collaboration among stakeholders in the supply chain, and effective demand planning. The aforementioned suggests that scholars regard SCRE as having multidimensional capabilities.
From the above literature review, 34 SCRE indicators in Appendix A are selected for this study, and the key SCRE indicators will be evaluated after FDM screening in Section 4.
2.3. Industry 5.0
According to Ostergaard (Citation2016), I5.0 enables workers to have a clearer understanding of their roles within the factory and promotes collaboration between humans and machines. In contrast to Industry 4.0, which emphasises device interconnection, I5.0 focuses on human – machine collaboration at different plant levels (Johansson Citation2017). Doyle-Kent and Kopacek (Citation2020) proposed that I5.0 is the lifeline of the manufacturing industry and an important source of industrial development mode transformation. Demir et al. (Citation2019) contend that I5.0 can promote worker benefits, investment and consumers, and maximise corporate profits. Motienko (Citation2020) proposed that I5.0 can combine human operational thinking and critical thinking to assign unified and repetitive tasks to robots, thereby improving production efficiency and product quality. Hence, numerous technology visionaries expect that I5.0 will humanise manufacturing (Tange et al. Citation2020).
I5.0 has been a hot topic among researchers since 2017 (Demir, Döven, and Sezen Citation2019; Nahavandi Citation2019; Paschek, Mocan, and Draghici Citation2019). I5.0 complements the existing Industry 4.0 paradigm by catering to workers’ well-being, focusing on sustainability, and being a resilient facilitator of prosperity (Breque, Nul, and Petridis Citation2021; Ghobakhloo et al. Citation2021). Nahavandi (Citation2019) believe that I5.0 can revolutionise the manufacturing system, penetrate into the supply chain and production shop processes, improve production and operation efficiency, and shorten production time and cycle time. Motienko (Citation2020) assessed the potential and advantages of I5.0 in supply chain management and development, and smart healthcare. Frederico (Citation2021) studied the relationship between I5.0 and the supply chain, and proposed that I5.0 could enhance the strategic effect of supply chain through large-scale personalisation of products and services in pursuit of a more intelligent and sustainable supply chain. Zhang et al. (Citation2022) found that the integration of I5.0 into supply chain management helps to enhance resilience. Ghobakhloo et al. (Citation2022) investigated the relationship between I5.0 and sustainable manufacturing, developing a strategic roadmap to elucidate how Industry 5.0 can enhance sustainable manufacturing practices. Nayeri et al. (Citation2023) introduced the concept of Responsive Supply Chain 5.0 and developed an innovative method to integrate the principles of I5.0 into supply chain management, aiming to enhance business responsiveness. Masoomi et al. (Citation2023) posited that Industry 5.0-based solution methodologies have the potential to address sustainable development challenges within the renewable energy supply chain, particularly in solar energy. Ghobakhloo et al. (Citation2023) developed the strategy roadmap for enabling Industry 5.0 transformation and explained how Industry 5.0 transformation should be managed to facilitate sustainable development. Furthermore, Dwivedi et al. (Citation2023) and Sami et al. (Citation2023) have conducted studies on the interactions between Industry 5.0 and circular supply chains, providing insights that inform supply chain management practices.
Based on the aforementioned literature review, this study summarises 123 I5.0 drivers in Appendix A, which are classified into people-oriented, sustainable, resilient, and technological categories. Key I5.0 drivers after FDM screening are evaluated in Section 4.
2.4. SSCRs, SCRE and industry 5.0
At present, global supply chain risk and resilience has been extensively studied. However, SSCRs and SCRE have been little researched (Chen et al. Citation2022). Moreover, only four studies on the aforementioned have been identified. Sadeghi and Boyer Hasani (Citation2019) developed a multi-objective optimisation model to combine the advantages of supply chain resilience and maximally reduce SSCRs and vulnerability. Lotfi et al. (Citation2021) designed a closed-loop supply chain network for sustainable resilience and studied the interaction between a sustainable supply chain and resilience, considering conditional risk factors. Bhatti and Nawaz (Citation2020) conducted a survey on a sustainable tourism supply chain in Maldives and concluded that risk and resilience management of the tourism supply chain are positively correlated with sustainable supply chain performance. Hsu et al. (Citation2021) conducted an empirical study on the elevator manufacturing industry, demonstrating that key SCRE can be identified and mitigated. Therefore, while studies related to SSCRs and SCRE are crucial, there has been no research conducted from the perspective of logistics.
Studies on the use of I5.0 to improve SCRE are lacking; however, many researchers have found that industry 4.0 can improve SCRE. I5.0, as the supplement and redevelopment of the Industry 4.0 paradigm, is of great research significance for improving SCRE through I5.0. In this study, we discuss the development trend of SCRE and I5.0 by considering the related research on Industry 4.0 and SCRE. Ralston et al. (Citation2020) believe that Industry 4.0 improves supply chain resilience and can facilitate supply chain competitive advantages and improve the business performance of enterprises. Spieske et al. (Citation2021) proposed an Industry 4.0-SCRE framework, emphasising its pivotal role in enhancing SCRE. Mubarik et al. (Citation2021) by utilising supply chain mapping, found that industry 4.0 could improve supply chain resilience, thereby improving enterprise operating efficiency. Tortorella et al. (Citation2022) conducted a review on the contribution of Industry 4.0 to SCRE. The results revealed that Industry 4.0 has a positive impact on SCRE, emphasising its importance in promoting supply chain risk identification. Therefore, the above studies have shown that Industry 4.0 is critical to promote SCRE, and the application of I5.0 to improving SCRE is worthy of further research.
The aforementioned studies reveal that SCRE plays a crucial role in the study of SSCRs, and several researchers have demonstrated the feasibility and effectiveness of utilising SCRE to address SSCRs (Lina et al. Citation2021, Hsu et al. Citation2022). In addition, although a small number of I5.0 studies considered the supply chain, no empirical studies have been conducted on both SSCRs and SCRE in the context of I5.0. Through a literature search in databases, Mehdiabadi et al. (Citation2022) identified a significant research gap in I5.0 concerning supply chain topics, particularly the lack of consideration for sustainable supply chain risk. However, to the best of our knowledge, there have been no studies exploring the interrelationship among SSCRs, SCRE, and I5.0. Therefore, this study constructs a two-stage HoQ to link SSCRs, SCRE, and I5.0; determines the key I5.0 drivers to improve SCRE and mitigate SSCRs; and provides a reference for logistics service providers to make sustainable supply-chain decisions.
3. Methodology
A QFD model, drawing on the frameworks established by Bottani (Citation2009) and Lam and Bai (Citation2016), is employed to elucidate the interconnections among SSCRs, SCRE, and I5.0 drivers. The primary advantage of this model lies in its ability to construct relationships between these three variable groups. Specifically, this study incorporates two HoQ within the QFD framework to connect SSCRs with SCRE, and SCRE with I5.0. The first HoQ phase focuses on linking SSCRs and SCRE, primarily to identify which SCRE indicators effectively respond to SSCRs. The second HoQ phase establishes a connection between SCRE and I5.0, aiming to determine which I5.0 drivers significantly enhance SCRE. Furthermore, the SCRE weights calculated in the first HoQ phase serve as a basis for developing the second phase HoQ, influencing the importance weights in this subsequent stage. presents a detailed structure of these two HoQ.
This study employs FDM, fuzzy interpretative structural modelling (FISM), analysis network procedure (ANP), and TOPSIS method are used to screen out SSCR factors and SCRE indicators (see Appendix B for the specific operation steps). The results reveal the different risk factors and resilience indicators that are adapted to different enterprises. The combination method is used for the following reasons: 1. This study adopts a questionnaire survey, and FDM is currently the mainstream analysis method applied to questionnaire surveys: it can avoid the influence of extreme data. 2. FISM can avoid subjectivity in the experts’ scoring to a certain extent, clearly reflecting the role strength of various factors, and is realistic. 3. Applying ANP and TOPSIS can transform the subjective and qualitative evaluation into a quantitative expression. Therefore, the combination of qualitative and quantitative evaluations can overcome the personal preferences of decision-makers, improve the effectiveness of decision-making, and ensure that the evaluation results are practicable. Therefore, the above methods are adopted in this study to improve data objectivity. In particular, ANP-TOPSIS ensures the data are consistent and not over-processed. The research methodology in this study is shown in . The symbols numbered from (1) to (11) in the text correspond to the relevant steps in .
3.1. HoQ1: linking SSCRs and SCRE
First, the initial SSCR factors were identified, and industry experts then assessed the relationship between, and causes of, these factors to design the first round of the FDM questionnaire. Second, the obtained FDM questionnaire values were calculated, and a reasonable threshold was set for evaluation. SSCRs① and SCRE② were selected. Furthermore, the selected SSCRs were evaluated using the FISM-ANP analysis method to obtain the interaction matrix ⑥, and the weight value ⑦ was calculated. The SCRE correlation matrix ② and the SSCRs and SCRE correlation matrix ⑤ are then calculated. Finally, the key SCRE ranking ⑧was obtained based on the interaction between the two variables using the TOPSIS method.
3.2. HoQ2: linking SCRE and I5.0
After determining the initial I5.0 drivers, FDM is used to screen out I5.0 drivers ③. Second, the toughness matrix ④, I5.0, and the toughness correlation matrix are calculated. Finally, the weighting of I5.0 drivers and their priorities are obtained via the TOPSIS method.
4. Empirical study
This study takes the largest private logistics enterprise in China as the case object to validate the proposed framework. The company is a prominent leader in China’s logistics industry and a well-known integrated logistics service provider in China, covering a series of supply chain-related services, including procurement, production, sales, transportation, and after sales. Presently, the supply chain business of the case enterprise is divided into three parts: logistics, finance, and commerce, among which logistics is the main business. The case enterprise is chosen as the research object because of (1) typicality. As a rapidly developing and large-scale logistics enterprise in China, it can well represent the development of China’s logistics industry and supply chain. (2) Sustainability. The case enterprise takes sustainability as a basic goal of its development, embodies the idea of sustainability in the operation of the supply chain, and strives to enhance and improve the operation level of the supply chain. (3) The case company faces severe competition in the external market. In recent years, some deficits in performance have also been observed, and the resilience of the supply chain is being challenged. However, as the enterprise supply chain system becomes large and complex and the supply chain risks become increasingly severe, enterprise managers find it difficult to analyze the supply chain risk factors. Thus, they require appropriate I5.0 drivers to improve supply chain resilience. Furthermore, managers often face challenges in prioritizing SSCRs, SCRE, and I5.0 components. To address this, our study engaged eight experienced supply chain experts from the case enterprise for in-depth, structured interviews. These experts, selected for their extensive knowledge and proficiency in relevant areas, provided invaluable insights for the analysis of SSCRs. Based on their collective input, we formed an overarching judgment. The data gathered and the subsequent analysis were then applied to develop two-stage HoQs, thereby validating the proposed framework. An overview of the experts interviewed is presented in .
Table 1. Survey of interviewees.
4.1. First HoQ linking SSCRs and SCRE
4.1.1. Stage I: confirmation of important SSCRs and SCRE using FDM
The FDM questionnaire was designed and distributed based on the initial 30 SSCRs and 34 SCRE, which were calculated using EquationEquation (1)(1)
(1) in Appendix B. Following expert discussions, the SSCRs and SCRE thresholds were set as 5.0 and 5.48, respectively, leading to the elimination of factors and indicators below the threshold. The initial 13 factors are identified as the key SSCRs, and the corresponding calculation results are presented in . Similarly, the first 17 factors are regarded as the key SCRE, as shown in .
Table 2. Confirmation of important SSCRs using FDM.
Table 3. Important SCRE selected by FDM.
Following the screening process, the original 30 SSCRs were reduced to 13 via FDM, while the original 34 SCRE were screened to 17. presents the three most significant factors: communication risk, management policy and marketing strategy, and technical risk. The top-three factors in are good customer service, efficiency, and predictive ability. These key SSCRs and SCRE are analysed in sections ① and ② of the first HoQ, as shown in .
4.1.2. Stage II: verification of the interaction between key SCRE
To explore the interaction relationship between key SCRE by the screening results of FDM, the 17 SCRE matrices were further analysed. The expert group evaluated the interaction matrix to determine the interaction between each SCRE. As the upper triangle of the autocorrelation matrix of SCRE is equal to the lower triangle, the arithmetical average of the eight questionnaires recovered is calculated as its autocorrelation matrix. This matrix is part ④ of the first HoQ, and its autocorrelation matrix is presented in .
Table 4. Autocorrelation matrix of key SCRE indexes.
4.1.3. Stage 3: construct SSCRs and SCRE association matrix
SSCRs and SCRE have a mutual influence relationship, but the influence relationship between the pairwise and degree of influence differs from the autocorrelation toughness matrix: a pairwise influence relationship exists. Therefore, the arithmetic mean of SSCRs and SCRE is calculated to form the correlation matrix of SSCRs and SCRE, which is the fifth part of the first HoQ. The matrix is shown in .
Table 5. Construct SSCRs and SCRE association matrix.
4.1.4. Stage 4: construct SSCRs matrix and ranking
FISM can be used to explore the relationship between any two elements. The fuzzy association strength matrix of the SSCRs was constructed using EquationEquation (2)(2)
(2) in Appendix B, as listed in .
Table 6. Construct SSCRs fuzzy association strength matrix.
When the threshold value of the fuzzy adjacency matrix of the SSCRs key factors is set to 2.3, EquationEquation (3)(3)
(3) in Appendix B is used to transform into an adjacency matrix, where 1 indicates that the factors in the left column are directly related to the uplink factors, and 0 indicates that they are unrelated. The transformation results of the adjacency matrix of the key risk factors for a sustainable supply chain are listed in .
Table 7. Construct adjacency matrix of key SSCRs.
In the adjacency matrix, 1 indicates a strong relationship between the two elements, and 0 indicates a weak relationship. An important relationship exists between the management policy and marketing strategy (A2) and communication risk (A1), demand fluctuation (A5), enterprise competitiveness (A6), and local events (A13). Technology risk (A3) is significant for IT infrastructure, communications and information efficiency (A11). The risk (A4) introduced by suppliers is significantly related to the competitive threat (A6), financial risk (A9), and transportation interruption risk (A10) of enterprises. Demand fluctuation (A5) is significantly related to communication risk (A1), supplier risk (A4), and enterprise competitive threat (A6). Enterprise competitive threat (A6) has a significant relationship with demand fluctuation (A5), financial management ability of partners or owners (A8), and financial risk (A9). Financial risk (A9) has an important relationship with financial management ability (A8). IT infrastructure, communications, and information efficiency (A11) poses significant risks to technical risk (A3) and financial risk (A9). Local events (A13) has a significant impact on demand fluctuations (A5).
From the above calculation of the fuzzy interpretation structure model, the structure analysed by FISM is used as the basis of ANP analysis. In this study, a 0–9 scoring method is adopted. The higher the score is, the greater the impact of one element on another element will be. The relationship between various elements can be realised through expert scoring. The data of Expert 1 is taken as an example, and the weights are calculated using EquationEquation (5)(5)
(5) in Appendix B. The pairwise comparison results of each risk are shown in .
Table 8. Pairwise comparison judgment matrix of original key SSCRs by expert 1.
The SSCR weights can be calculated using EquationEquations (5)(5)
(5) and (Equation6
(6)
(6) ) in Appendix B: the calculation results are listed in .
Table 9. Weight of each factor of expert 1 under the condition of A2.
Table 10. Weight of each factor of expert 1 under the condition of A3.
Table 11. Weight of each factor of expert 1 under the condition of A4.
Table 12. Weight of each factor of expert 1 under the condition of A5.
Table 13. Weight of each factor of expert 1 under the condition of A6.
Table 14. Weight of each factor of expert 1 under the condition of A9.
Table 15. Weight of each factor of expert 1 under the condition of A11.
Table 16. Weight of each factor of expert 1 under the condition of A13.
The data from Expert 1 passed the consistency test. The ANP analysis results evaluated by eight experts are summarised using the above methods, and the average value is the weight of the ANP analysis. The results are presented in .
Table 17. Importance vector calculation results of key SSCRs.
Table 18. Importance vector calculation results of eight experts under A2.
Table 19. Importance vector calculation results of eight experts under A3.
Table 20. Importance vector calculation results of eight experts under A4.
Table 21. Importance vector calculation results of eight experts under A5.
Table 22. Importance vector calculation results of eight experts under A6.
Table 23. Importance vector calculation results of eight experts under A9.
Table 24. Importance vector calculation results of eight experts under A11.
Table 25. Importance vector calculation results of eight experts under A13.
The key factors affecting sustainable supply chain risks, A2, A3, A4, A5, A6, A9, A11 and A13, are determined through FISM analysis in the previous section, and the average value of the importance vector of each factor is obtained, which is connected with the adjacency matrix in to form a supermatrix with key risks. This matrix is part ⑥ of the first HoQ, and its autocorrelation matrix is presented in .
Table 26. Key SSCRs autocorrelation matrix.
By multiplying the ANP weight by the key SSCRs autocorrelation matrix, the integrated weight of SSCRs can be obtained and sorted according to the integrated weight. The SSCRs factor weight and ranking result is listed in and can be used as part ⑦ of the first HoQ.
Table 27. Weights ranking results of key SSCRs.
reveals that the top-five key SSCRs factors in terms of weight rank are financial management ability (A8), changes in tax collection and barriers (A9), transportation interruption risk (A10), technology risk (A3), and communication risk (A1).
4.1.5. Stage 5: TOPSIS ranking between SSCRs and SCRE
The key SSCRs autocorrelation matrix, SSCRs and SCRE autocorrelation matrix, and SCRE autocorrelation matrix in, and 6, respectively, were multiplied to obtain a comprehensive initial matrix for TOPSIS analysis of SSCRs and SCRE, as presented in .
Table 28. TOPSIS initial matrix of supply chain resilience index analysis.
Communication (A1), technical risks (A3), the risks of suppliers (A4), demand fluctuations (A5), enterprise competitive threat (A6), lack of high-quality talent (A7), the tax change or barriers (A9), transportation interruption risk (A10), environmental pollution (A12), and local event type (A13) belong to the cost index. Specifically, a small value of this type of indicator is desirable. Therefore, the reciprocal method is adopted to transform it into a beneficial indicator to ensure the whole matrix exhibits the same trend, as presented in .
Table 29. Syntrended results of SSCRs and SCRE.
The dimensionality of the matrix is processed using EquationEquation (10)(10)
(10) in Appendix B, and the normalised initial matrix is obtained, as listed in .
Table 30. Key SCRE formalization initial matrix in TOPSIS.
The matrices of the positive and negative ideal solutions were obtained by processing EquationEquations (12)(12)
(12) , (Equation13
(13)
(13) ), (Equation14
(14)
(14) ), (Equation15
(15)
(15) ), and (Equation16
(16)
(16) ) in Appendix B, and the positive and negative ideal solutions, Euclidean distance, and relative proximity and their ranking were then obtained according to Equations (18), (19), (Equation20
(22)
(22) ), (21), and (22), as presented in .
Table 31. Construct SCRE positive and negative ideal solutions, Euclidean distance, relative proximity and ranking.
According to the relative proximity ranking of SCRE analysed by TOPSIS, the top-five indicators are confidence (B14), risk awareness (B11), market positioning (B6), human resource management (B5), and flexibility (B13), which are indices closest to the optimal solution. This section is part ⑧ of the first HoQ, as shown in .
In the first HoQ, FDM, FISM, ANP, and TOPSIS were combined to obtain the key SSCRs and SCRE rankings, and the results are shown in .
4.2. Second HoQ linking SCRE and I5.0 drivers
4.2.1. Stage I: selection of key I5.0 drivers using FDM
Enterprises face gradually increasing uncertainty due to fierce market competition. Logistics enterprises have realised the need to create a supply chain for the logistics service system to quickly respond to uncertainty and deal with risk factors. In this study, SSCRs, SCRE, and I5.0 are considered to broaden the perspective of supply chain management for logistics enterprises.
In this study, FDM and TOPSIS were used to bridge key SCRE and I5.0 drivers. From a literature review and expert analysis, 123 initial drivers were selected. From these, the FDM questionnaire was designed: experts were asked to evaluate the maximum and minimum values of each driver on a scale between 0 and 10. After the questionnaire was recovered, the extreme values (more than two times the standard deviation) were removed, and the values were calculated.
Considering the expert discussion and actual case enterprises, the number of I5.0 drivers was reduced from 123 to 20 through screening and subdivided into four dimensions: people first, sustainability, resilience, and technology. The calculations presented in will be included in section ③ of the second HoQ, along with , and .
Table 32. Key I5.0 drivers selected by FDM.
4.2.2. Stage II: the autocorrelation matrix of I5.0 drivers was constructed
The autocorrelation questionnaire on I5.0 drivers was designed using the 20 I5.0 key driving factors selected via FDM, and the average value of each index was calculated to construct the autocorrelation matrix. The value of is equal to, that is, the upper triangle and the lower triangle of the matrix are the same, and the matrix construction results are listed in . This comprises part ⑨ of the second HoQ.
Table 33. Autocorrelation matrix of key I5.0 drivers.
4.2.3. Stage III: construction the association matrix between SCRE and I5.0 drivers
An influential relationship exists between SCRE and I5.0 drivers. Key I5.0 drivers can effectively improve the SCRE. Therefore, the association matrix between SCRE and I5.0 drivers is herein constructed, which is the primary part of the second HoQ. The matrix is listed in .
Table 34. Correlation matrix of SCRE and I5.0 drivers.
4.2.4. Stage Ⅳ: TOPSIS ranking of SCRE and I5.0 key drivers
The initial matrix for TOPSIS analysis was obtained by multiplying the I5.0 key driver autocorrelation matrix and key SCRE index with the I5.0 key driver correlation matrix and key SCRE autocorrelation matrix, as presented in .
Table 35. TOPSIS initial matrix of key I5.0 driver analysis.
Because all the factors exhibit a positive trend, co-trending is unnecessary. The initial matrix was standardised using EquationEquation (10)(10)
(10) in Appendix B, and the positive and negative ideal solutions, Euclidean distance, and relative proximity were obtained using EquationEquations (17)
(17)
(17) , (18), and (19), respectively. The calculation results are presented in .
Table 36. Euclidean distance and relative proximity.
The top-five indicators according to the I5.0 relative closeness ranking of TOPSIS analysis are responsible consumption and production (C7), organisational justice, trust and innovation (C4), energy conservation and emission reduction (C10), customising products and services to customers (C9), and cost and expense management (C20).
4.3. Results and discussion
Generally, enterprise resources are limited, making it challenging to enhance SCRE in all aspects simultaneously. To effectively cope with SSCRs, enterprise managers should prioritise SCRE and gradually invest in maximising production and operation benefits because the Pareto effect can be utilised using a few key factors when resource utilisation is effective. Therefore, we applied the Pareto effect to the QFD-MCDM framework in this study. Moreover, we discuss the importance ranking of these three variables with the results of the two HoQ as a reference to improve SCRE and mitigate the SSCRs of a logistics enterprise using key I5.0 drivers, as shown in . If all key SCRE can be improved, resistance to SSCRs can be greatly improved.
4.3.1. HoQ1: connecting SSCRs and SCRE to get the most critical ranking
In this study, SSCRs and SCRE were connected by the first HoQ. As listed in , the top-five key SSCRs are enterprise’s financial management capability (A8), changes and barriers in tax collection (A9), transportation disruption risk (A10), technology risk (A3), and communication risk (A1). lists the top-five key SCRE indicators as confidence (B14), risk awareness (B11), market positioning (B6), human resource management (B5), and flexibility (B13). The above key SSCRs and SCRE should be the focus for enterprise managers.
As shown in , the key SCRE priorities calculated using TOPSIS can significantly mitigate SSCRs. The TOPSIS ranking results show that confidence (B14) ranks first in SCRE, indicating that confidence is the basis of cooperation in the face of SSCRs. A good confidence relationship can enhance stability upstream and downstream of the supply chain and increase resistance to fierce market competition. If the financial management of the enterprise is poor, for example, a shortage of the capital chain will cause abnormal changes in supply chain orders, it will lead to a crisis of confidence. Christopher et al.(Citation2004) proposed that enhancing confidence can reduce supply chain risks. Therefore, confidence (B14) is helpful for enterprises seeking to mitigate SSCRs, which can be achieved by establishing a sound credit risk management and early warning mechanism.
The second-ranking SCRE is risk awareness (B11), which indicates that enterprises should improve their awareness of risk response as the risks related to the supply chain become increasingly prominent. Two examples can be used to illustrate this. In 2011, due to the tsunami and earthquake in Japan, suppliers of parts to Toyota had an insufficient estimate of natural risks, resulting in the risk of transportation disruption (A10), which resulted in missed delivery schedules (Hosseini, Ivanov, and Dolgui Citation2019). Another example is the outbreak of COVID-19 in early 2020, which caused unprecedented supply chain disruptions, halted the production and flow of materials, affected the demand and supply side of logistics infrastructure, directly affected personnel and operational needs, and even threatened corporate finances. Logistics service providers must perform operational changes to survive (Wackett Citation2020). Therefore, establishing risk awareness (B11) and a complete-risk early warning system is crucial, as is presetting different possible risks in advance and formulating corresponding measures. Therefore, increasing risk awareness can mitigate SSCRs, especially for the top-five key SSCRs.
In third place in SCRE is market positioning (B6). Evaluating the enterprise’s financial management ability (A8); improving the service quality to win the trust of customers; strategically adopting a differentiation competitive strategy to break trade barriers; improving the competitiveness of the different business modules; and avoiding transportation disruptions (A10), technical risks (A3), SSCRs (A1), communication, risk among others is necessary for establishing an accurate market position and improving the quality of enterprise service. For example, DHL, the world’s leading postal and logistics group, precisely positioned the market through logistics innovation of emerging green technologies, green production, reducing carbon emissions and energy consumption, and increasing the use of renewable energy to maintain a competitive advantage in the market (Cosimato et al. Citation2015). Tesla replaced gasoline and diesel with lithium batteries, developed low-carbon vehicles to meet consumers’ demand preferences for low-carbon products, obtained government tax breaks, and avoided traditional automotive industry barriers. In 2020, their total sales volume reached 500,000 units, indicating that market positioning is crucial to the development of enterprises (Ma et al. Citation2021). Therefore, market positioning (B6) is related to the competitive advantage of enterprises in the market and has a positive role in mitigating SSCRs.
Human resource management (B5) ranked fourth in SCRE. Effective human resource management can avoid risks, enhance risk awareness, and improves the financial management ability of enterprises (A8). It includes paying attention to the satisfaction of employees’ self-development needs, conducting professional skills training, improving the adaptability and application ability of digital technology, and promoting all-round employee development. Humayun (Citation2021) believed that HRM can effectively improve the sustainable development needs of supply chain members. Evidently, human resource management plays an indispensable role in the supply chain. Therefore, improving human resource management (B5) can mitigate the occurrence of SSCRs.
Flexibility (B13) ranks fifth among the SCRE factors. Flexibility plays an important mediating role between strategic partnership and business performance, and can effectively avoid risks such as communication risk (A1) and transportation disruption risk (A10). Yang et al. (Citation2021) demonstrated that flexibility can promote information transmission among suppliers, manufacturers, and retailers; improve the supply chain transparency; and enhance the market risk response ability of enterprises. Therefore, to maintain a flexible supply chain, the relationship with suppliers must be coordinated and a stable supply chain structure established. Second, the flexible use of human resources, funds, and equipment helps to improve the overall financial management ability of enterprises (A8). Finally, building an information resource cooperation platform is necessary to establish a good partnership with suppliers to reduce communication risks (A1). Therefore, flexibility (B13) is critical to mitigating SSCRs.
Therefore, in situations with limited resources, enterprises should focus on the above five most-critical SCRE to mitigate SSCRs and maximise production and operation efficiency. Taking into account the long-term development of enterprises, the other key SCRE identified in this study can also be used as an important reference for enterprises to mitigate SSCRs and gradually applied and promoted.
4.3.2. HoQ2: connecting SCRE and I5.0 to obtain the most critical I5.0 ranking
Among the driving I5.0 factors, 20 important factors are selected from the four dimensions in , indicating that all the important factors deployed from SCRE in this study can cover the entirety of I5.0.
The human dimension comprises the following: attaching importance to human needs (C1); focusing on collaboration between artificial intelligence and cognition (C2); motivating workers (C3); organisational justice, trust, and innovation (C4); and paying attention to different values and needs of society (C5).
The sustainable dimension comprises the following: advanced digital technologies to reduce product waste and cost (C6), responsible consumption and production (C7), industrialisation, innovation in infrastructure (C8), customising products and services to customers (C9), energy saving and emission reduction (C10).
The ductile dimension comprises the following: adaptability (coping with external changes) (C11), improve production flexibility and increase control redundancy (C12), improve the level of autonomy and control (C13), new operation management model (C14), and improve supply chain recovery and mitigation mechanism (C15).
The technology dimension comprises the following: renewable energy storage and trusted autonomous technology (C16), financial resources, financial resources (C17), market competitive pressure (C18), fully integrate enterprise resource planning (C19), and cost and expense management (C20)
In the second HoQ, SCRE acts as an intermediate bridge connecting the SSCRs and I5.0 drivers. reveals that the top-five key I5.0 drivers are responsible consumption and production (C7), organisational justice, trust and innovation (C4), energy saving and emission reduction (C10), customising products and services to customers (C9), and cost and expense management (C20). Being the five most critical I5.0 drivers of the case enterprise, we analyse them in order of rank below.
Responsible consumption and production (C7) enables the case company to align with sustainable and intelligent practices, achieve precise market positioning (B6), establish a perfect privacy protection system to protect the information security of upstream and downstream users of the supply chain as the goal, and improve the confidence (B14) between enterprises. According to Juniati et al. (Citation2020) reviewed and analysed 44 sustainability reports and 453 annual reports of Indonesian listed enterprises from 2014 to 2016 and pointed out that enterprises tend to support responsible consumption and production to realise sustainable development goals (Juniati, Paulina, and Carol Citation2020). Within the entire supply chain, it is essential to consider not only economic benefits, such as reinforcing product quality and safety oversight to improve enterprise risk awareness (B11) and enhance enterprise flexibility (B13), but also consider human resource management (B5) to form a good working environment and meet the development needs of employees.
Organizational justice, trust, and innovation (C4) can promote confidence (B14) and enhance risk awareness (B11) upstream and downstream of the enterprise, which is conducive to establishing a good organisational atmosphere, improving the job satisfaction and happiness of employees, and making human resource management (B5) more harmonious. Cultivating employees’ innovation ability to grasp the market positioning (B6) can facilitate the sustainable development of the enterprise and make the enterprise flexible. For example, Akram et al. (Citation2020) by investigating the innovative work behaviours of 345 employees in China’s telecom industry, found that organisational justice and trust can promote positive work behaviours in employees, inculcate their willingness to express their ideas and implement them in the organisation, and create a good innovation atmosphere.
Energy conservation and emissions reduction (C10) is an effective path towards sustainable development. Green packing, green transportation, green marketing, and green office imply grasping the risk awareness (B11) to ensure production is in line with the market positioning (B6) product sustainable supply chain, making the enterprise more flexible. For example, DHL, a global leader in logistics, focuses on the Paris Agreement and the United Nations Sustainable Development Goals, optimises energy efficiency through sustainable fuels, reduces dependence on fossil fuels and pollutants, and promotes its sustainable development with sustainable carbon management practices to enhance its competitive edge (Deweerdt, Dargusch, and Hill Citation2022). Therefore, energy saving and emission reduction (C10) promotes the benign development of the supply chain from the perspective of enterprise strategy and benefits enterprise supply chain management.
Customise products and services to customers (C9), by accurately targeting market positioning (B6), provides customers with customised products and services, ensuring risk awareness (B11). This facilitates developing value-added services and providing integrated supply chain solutions to improve customer viscosity and increase enterprise flexibility (Bl3). For example, Maddikunta et al. (Citation2021) pointed out that mass production of customised and personalised products can improve output and customer satisfaction, thereby generating value-added services and helping supply chain management to reduce costs. Therefore, customising products and services to customers (C9) can ensure a diversified development of the supply chain from the perspective of the enterprise.
Cost and expense Management (C20) indicates that enterprises should pay attention to supply chain collaboration and fine management of costs in each link to prevent capital chain shortage to improve the enterprise risk awareness (B11) and enhance upstream and downstream supply chain confidence (B14). Um and Kim (Citation2019) demonstrated that supply chain collaboration can offer transaction cost advantages and improve the performance of enterprises. Furthermore, cost and expense management (C20) plays an important role in promoting the rapid development of enterprises with the improvement of logistics infrastructure and technological progress, thereby promoting the scale effect. Enterprises can transform from labour intensive to technology intensive, thereby reducing costs and increasing efficiency. Finally, cost and expense management (C20) enables enterprises to focus on their own market positioning (B6) to improve core competitiveness, better human resource management (B5) to improve the overall efficiency of the supply chain, and promote enterprise flexibility (B13).
Enterprises should focus on the five most critical I5.0 drivers above to mitigate SSCRs while improving supply chain resilience. Meanwhile, other important I5.0 drivers can be progressively applied to case enterprise decision support, with adequate resources, to enhance SCRE and mitigate SSCRs for supply chain sustainability.
Enterprises in different industries can also utilise the proposed framework to reduce risk. However, in constructing a risk list, it should be divided according to the risks of different industries. Therefore, this framework should be applied according to the industry characteristics, making full use of the target company’s supply chain experts to analyse and identify the risks of sustainable supply chain. Moreover, the corresponding resilience improvement measures should be conducted in a gradual cycle according to the planning, execution, inspection, and implementation processes, and its performance should be regularly reviewed.
5. Conclusions and recommendations
In the current landscape, logistics enterprises are confronted with increasingly complex risks and uncertainties. These enterprises recognise that disruptions in the supply chain can have widespread impacts on the global supply chain system. Therefore, it is imperative for these enterprises to swiftly mitigate uncertain risks within the supply chain. In response, we developed a QFD approach to explore the interrelationships among SSCRs, SCRE, and I5.0 drivers. This research expands the scope of sustainable supply chain risk resilience management by incorporating considerations of SSCRs, SCRE, and I5.0 drivers. It is crucial for managers to devise SCRE strategies that account for both SSCRs and I5.0, particularly from the perspective of logistics enterprises. Validation conducted on China’s largest private logistics enterprise reveals the following main findings:
The top-five SSCRs are the enterprise’s financial management ability, changes and barriers in tax collection, transportation disruption risk, technology risk, and communication risk.
The top-five SCRE are confidence, risk awareness, market positioning, human resource management, and flexibility.
The top-five I5.0 drivers are responsible consumption and production, organisational justice, trust and innovation, energy saving and emission reduction, customising products and services to customers, and cost and expense management.
The primary contributions of this study are outlined as follows:
Firstly, this research represents a pioneering effort to integrate I5.0 into the sustainable supply chain risk resilience framework of a logistics enterprise. We propose a QFD integrated with MCDM, utilising a combination of FDM, FISM, ANP, and TOPSIS. This approach is designed to elucidate the relationships among key SSCRs, SCRE, and I5.0 drivers, providing decision support to enhance SCRE in logistics enterprises.
Secondly, this paper offers a reference point for future research, presenting a feasible framework for logistics enterprises seeking to apply I5.0 drivers to improve SCRE and mitigate SSCRs.
Finally, the research framework and data analysis presented here can aid logistics enterprises in effectively adjusting their supply chain strategies, operations, and management directions, particularly when leveraging enterprise resources efficiently.
5.1. Theoretical and practical implications
The significance of this study lies in its pioneering exploration of I5.0 within the realm of SSCRs and SCRE. This novel investigation sheds light on the integration of I5.0 into the mitigation of SSCRs and enhancement of SCRE in logistics enterprises. Our findings reveal that the top five SSCRs pose significant challenges to the implementation of sustainable supply chains in these enterprises. Conversely, the five most crucial SCRE and I5.0 drivers have been identified as effective in addressing these challenges, lending practical managerial significance to our research. We recommend that managers prioritise the evaluation and management of these top five factors in SSCRs, SCRE, and I5.0. Additionally, it is advisable for them to tailor these considerations to the specific contexts of their respective enterprises.
This study contributes to the literature on sustainable supply chain management by empirically examining the interplay between SSCRs, SCRE, and I5.0 in logistics enterprises. The insights provided offer theoretical foundations for managers to leverage I5.0 drivers in mitigating sustainable supply chain risks and bolstering supply chain resilience.
Looking ahead, it is imperative for future research to integrate I5.0 with supply chain considerations across various industries. This integration will enable the identification of industry-specific risk factors and the development of tailored I5.0-driven solutions for sustainable supply chain risk resilience.
5.2. Limitations and future implications
While this research provides valuable insights, it is not without limitations, which present opportunities for future studies.
Firstly, the empirical analysis was conducted solely on China’s largest private logistics enterprise, thus not encompassing enterprises from other countries. This geographical limitation suggests that future research could benefit from a broader, more diverse set of enterprises across different countries to enhance the generalizability of the findings.
Secondly, there is an opportunity to extend the research to include medium and small-sized logistics enterprises. Investigating a wider range of company sizes could yield a more comprehensive understanding of the relationship between SSCRs, SCRE, and I5.0 drivers.
Lastly, future studies should consider including suppliers and customers of logistics enterprises as respondents. This would allow for a more holistic analysis of the interplay between SSCRs, SCRE, and I5.0, providing a more rounded perspective.
Additionally, the focus on a single logistics enterprise within China means the study’s implications are primarily relevant to specific national contexts and related industries. This limitation highlights the necessity for caution when generalising the study’s findings to different countries and industrial settings, due to unique country-specific characteristics and behaviours.
Authors contributions
C.-H.H.: Conceptualization, Methodology, Writing-review and editing
J.Z.W: Methodology, Software, Funding acquisition, Formal analysis, Writing-original draft
T.Y.Z: Data curation, Visualization, Supervision
J.Y.C: Visualization, Project administration, Resources
All authors reviewed the results and approved the final version of the manuscript.
Acknowledgments
The authors of this paper would like to acknowledge greatly to all the authors who have published valuable research articles and books that have been used for this review paper.
Disclosure statement
No potential conflict of interest was reported by the author(s).
Data availability statement
Data sharing is not applicable to this article.
Additional information
Funding
Notes on contributors
Chih-Hung Hsu
Chih-Hung Hsu received his bachelor’s degree in Industrial Management from Taiwan University of Science and Technology in 1996, master’s degree in Industrial Management from Taiwan University of Science and Technology in 1999, doctor’s degree in industrial Engineering and Engineering Management from Tsinghua University in Hsinchu, Taiwan in 2005, and master’s degree in International logistics from the Department of Shipping Management, Ocean University of Taiwan in 2011. His current research involves multi-attribute decision making and data mining in resilient, agile, green and sustainable supply chains.
Jin-Zheng Wu
Jin-Zheng Wu received the B.Sc. degree in Transportation from the Fujian University of Technology, Fujian, China, in 2020. He is currently pursuing the M.Sc. degree in Mechanical & Industrial Engineering from the Fujian University of Technology, Fujian, China. His main areas of interest are multi-attribute decision making, supply chains and Industry5.0.
Ting-Yi Zhang
Ting-Yi Zhang served as a associate professor of Institute of Industrial Engineering, College of Management, FuJian University of Technology. His main areas of interest are supply chain management, production system scheduling and optimization.
Jia-Yi Chen
Jia-Yi Chen received the B.Sc. degree in Logistics management from the Fujian University of Technology, Fujian, China, in 2022.
References
- Abdel-Basset, M., and R. Mohamed. 2019. “A Novel Plithogenic TOPSIS- CRITIC Model for Sustainable Supply Chain Risk Management.” Journal of Cleaner Production 247:119586. https://doi.org/10.1016/j.jclepro.2019.119586.
- Akram, T., S. Lei, M. J. Haider, and S. T. Hussain. 2020. “The Impact of Organizational Justice on Employee Innovative Work Behavior: Mediating Role of Knowledge Sharing.” Journal of Innovation & Knowledge 5 (2): 117–129. https://doi.org/10.1016/j.jik.2019.10.001.
- Alshehri, S. M. A., X. J. Wang, S. A. A. Shah, and Y. A. Solangi. 2022. “Analysis of Core Risk Factors and Potential Policy Options for Sustainable Supply Chain: An MCDM Analysis of Saudi Arabia’s Manufacturing Industry.” Environmental Science and Pollution Research 29 (17): 25360–25390. https://doi.org/10.1007/s11356-021-17558-4.
- Amin, F. U., Q. L. Dong, K. Grzybowska, Z. Ahmed, and B. R. Yan. 2022. “A Novel Fuzzy-Based VIKOR-CRITIC Soft Computing Method for Evaluation of Sustainable Supply Chain Risk Management.” Sustainability 14 (5): 2827. https://doi.org/10.3390/su14052827.
- Babu, H., P. Bhardwaj, and A. K. Agrawal. 2021. “Modelling the Supply Chain Risk Variables Using ISM: A Case Study on Indian Manufacturing SMEs.” Journal of Modelling in Management 16 (1): 215–239. https://doi.org/10.1108/JM2-06-2019-0126.
- Bayramova, A., D. J. Edwards, and C. Roberts. 2021. “The Role of Blockchain Technology in Augmenting Supply Chain Resilience to Cybercrime.” Buildings 11 (7): 283. https://doi.org/10.3390/buildings11070283.
- Bhatti, M. A., and M. A. Nawaz. 2020. “The Impacts of Tourism Risk Management, it Adoption, Agility and Resilience on the Sustainable Tourism Supply Chain Performance of Maldives’ Tourism Industry.” IRASD Journal of Management 2 (2): 100–108. https://doi.org/10.52131/jom.2020.0202.0020.
- Bottani, E. 2009. “A fuzzy QFD approach to achieve agility.” International Journal of Production Economics 119 (2): 380–391. https://doi.org/10.1016/j.ijpe.2009.02.013.
- Breque, M., L. D. Nul, and A. Petridis. 2021. Industry 5.0: Towards a Sustainable, Human-Centric and Resilient European Industry European Commission. Luxembourg, LU: Directorate-General for Research and Innovation.
- Bui, T. D., M. H. Ali, F. M. Tsai, M. Iranmanesh, M. L. Tseng, and M. K. Lim. 2020. “Challenges and Trends in Sustainable Corporate Finance: A Bibliometric Systematic Review.” Journal of Risk and Financial Management 13 (11): 264. https://doi.org/10.3390/jrfm13110264.
- Cerchione, P., R. Centobelli, and M. Ertz. 2020. “Managing supply chain resilience to pursue business and environmental strategies.” Business Strategy and the Environment 29 (3): 1215–1246. https://doi.org/10.1002/bse.2428.
- Chen, N., J. F. Cai, Y. R. Ma, and W. T. Han. 2022. “Green Supply Chain Management Under Uncertainty: A Review and Content Analysis.” International Journal of Sustainable Development & World Ecology 29 (4): 349–365. https://doi.org/10.1080/13504509.2021.2021561.
- Chrisandina, N. J., S. Vedant, E. Iakovou, E. N. Pistikopoulos, and M. M. El-Halwagi. 2022. “Multi-Scale Integration for Enhanced Resilience of Sustainable Energy Supply Chains: Perspectives and Challenges.” Computers & Chemical Engineering 164:107891. https://doi.org/10.1016/j.compchemeng.2022.107891.
- Christopher, M., and H. Lee. 2004. “Mitigating Supply Chain Risk Through Improved Confidence.” International Journal of Physical Distribution & Logistics Management 34 (5): 388–396. https://doi.org/10.1108/09600030410545436.
- Cosimato, S., O. Troisi, S. Ho, J. Martin, and P. Claudio Baccarani. 2015. “Green Supply Chain Management: Practices and Tools for Logistics Competitiveness and Sustainability. The DHL Case Study.” The TQM Journal 27 (2): 256–276. https://doi.org/10.1108/TQM-01-2015-0007.
- Demir, K. A., G. Döven, and B. Sezen. 2019. “Industry 5.0 and Human-Robot Co-Working.” Procedia Computer Science 158:688–695. https://doi.org/10.1016/j.procs.2019.09.104.
- Deweerdt, T., P. Dargusch, and G. Hill. 2022. “A Case Study of How DHL Practices Carbon Management.” Advances in Environmental and Engineering Research 3 (1): 010. https://doi.org/10.21926/aeer.2201010.
- Doyle-Kent, M., and P. Kopacek 2020. Industry 5.0: Is the Manufacturing Industry on the Cusp of a New Revolution? In Proceedings of the International Symposium for Production Research 2019; Springer: Cham, Switzerland, 432–441.
- Dwivedi, A., D. Agrawal, A. Jha, and K. Mathiyazhagan. 2023. “Studying the Interactions Among Industry 5.0 and Circular Supply Chain: Towards Attaining Sustainable Development.” Computers & Industrial Engineering 176:108927. https://doi.org/10.1016/j.cie.2022.108927.
- Erol, I., I. M. Ar, I. Peker, and C. Searcy. 2022. “Alleviating the Impact of the Barriers to Circular Economy Adoption Through Blockchain: An Investigation Using an Integrated MCDM-Based QFD with Hesitant Fuzzy Linguistic Term Sets.” Computers & Industrial Engineering 165:107962. https://doi.org/10.1016/j.cie.2022.107962.
- Frederico, G. F. 2021. “From Supply Chain 4.0 to Supply Chain 5.0: Findings from a Systematic Literature Review and Research Directions.” Logistics 5 (3): 49. https://doi.org/10.3390/logistics5030049.
- Ganguly, K., and G. Kumar. 2019. “Supply Chain Risk Assessment: A Fuzzy AHP Approach.” International Journal of Operations and Supply Chain Management 12 (1): 1–13. https://doi.org/10.31387/oscm0360217.
- Ghobakhloo, M., M. Fathi, M. Iranmanesh, P. Maroufkhani, and M. E. Morales. 2021. “Industry 4.0 Ten Years On: A Bibliometric and Systematic Review of Concepts, Sustainability Value Drivers, and Success Determinants.” Journal of Cleaner Production 302:127052. https://doi.org/10.1016/j.jclepro.2021.127052.
- Ghobakhloo, M., M. Iranmanesh, M. E. Morales, M. Nilashi, and A. Amran. 2023. “Actions and Approaches for Enabling Industry 5.0-Driven Sustainable Industrial Transformation: A Strategy Roadmap.” Corporate Social Responsibility and Environmental Management 30 (3): 1473–1494. https://doi.org/10.1002/csr.2431.
- Ghobakhloo, M., M. Iranmanesh, M. F. Mubarak, M. Mubarik, A. Rejeb, and M. Nilashi. 2022. “Identifying Industry 5.0 Contributions to Sustainable Development: A Strategy Roadmap for Delivering Sustainability Values.” Sustainable Production and Consumption 33:716–737. https://doi.org/10.1016/j.spc.2022.08.003.
- Glickmann, T. S., and S. C. White. 2006. “White Security, Visibility and Resilience: The Keys to Mitigating Supply Chain Vulnerabilities.” International Journal of Logistics Systems & Management 2 (2): 107–119. https://doi.org/10.1504/IJLSM.2006.009554.
- Govindan, H., and H. Gholizadeh. 2021. “Robust Network Design for Sustainable-Resilient Reverse Logistics Network Using Big Data: A Case Study of End-Of-Life Vehicles.” Transportation Research Part E: Logistics & Transportation Review 149:102279. https://doi.org/10.1016/j.tre.2021.102279.
- He, L., Z. Y. Wu, X. Wei, M. Goh, Z. T. Xu, W. Y. Song, X. G. Ming, and X. Wu. 2020. “A Novel Kano-QFD-DEMATEL Approach to Optimise the Risk Resilience Solution for Sustainable Supply Chain.” International Journal of Production Research 59 (6): 1–22. https://doi.org/10.1080/00207543.2020.1724343.
- Hosseini, S., D. Ivanov, and A. Dolgui. 2019. “Review of Quantitative Methods for Supply Chain Resilience Analysis.” Transportation Research Part E: Logistics & Transportation Review 125:285–307. https://doi.org/10.1016/j.tre.2019.03.001.
- Hsu, C.-H., M. G. Li, T. Y. Zhang, A. Y. Chang, S. Z. Shangguan, and W. L. Liu. 2022. “Deploying Big Data Enablers to Strengthen Supply Chain Resilience to Mitigate Sustainable Risks Based on Integrated HOQ-MCDM Framework.” Mathematics 10 (8): 1233. https://doi.org/10.3390/math10081233.
- Hsu, C.-H., R.-Y. Yu, A.-Y. Chang, W.-H. Chung, and W.-L. Liu. 2021. “Resilience-Enhancing Solution to Mitigate Risk for Sustainable Supply Chain-An Empirical Study of Elevator Manufacturing.” Processes 9 (4): 596. https://doi.org/10.3390/pr9040596.
- Humayun, M. 2021. “Industrial Revolution 5.0 and the Role of Cutting Edge Technologies.” International Journal of Advanced Computer Science and Applications 12 (12): 12. https://doi.org/10.14569/IJACSA.2021.0121276.
- Johansson, H. 2017. Profinet Industrial Internet of Things Gateway for the Smart Factory. Master’s Thesis, Chalmers University of Technology, Gothenburg, Sweden.
- Juniati, G., P. Paulina, and T. Carol. 2020. “Sustainable Development Goal Disclosures: Do They Support Responsible Consumption and Production?” Journal of Cleaner Production 246:118989. https://doi.org/10.1016/j.jclepro.2019.118989.
- Khan, S. A. R., Y. Zhang, H. Golpîra, A. Sharif, and A. Mardani. 2020. “A State-Of-The-Art Review and Meta-Analysis on Sustainable Supply Chain Management: Future Research Directions.” Journal of Cleaner Production 278:123357. https://doi.org/10.1016/j.jclepro.2020.123357.
- Khan, S. A. R., Y. Zhang, A. Kumar, E. Zavadskas, and D. Streimikiene. 2020. “Measuring the Impact of Renewable Energy, Public Health Expenditure, Logistics, and Environmental Performance on Sustainable Economic Growth.” Sustainable Development 28 (4): 833–843. https://doi.org/10.1002/sd.2034.
- Koberg, E., and A. Longoni. 2018. “A Systematic Review of Sustainable Supply Chain Management in Global Supply Chains.” Journal of Cleaner Production 278:123357. https://doi.org/10.1016/j.jclepro.2018.10.033.
- Kwak, D.-W., Y.-J. Seo, and R. Mason. 2018. “Investigating the Relationship Between Supply Chain Innovation, Risk Management Capabilities and Competitive Advantage in Global Supply Chains.” International Journal of Operations & Production Management 38 (1): 2–21. https://doi.org/10.1108/IJOPM-06-2015-0390.
- Lam, J. S. L., and X. Bai. 2016. “A Quality Function Deployment Approach to Improve Maritime Supply Chain Resilience.” Transportation Research Part E: Logistics & Transportation Review 92:16–27. https://doi.org/10.1016/j.tre.2016.01.012.
- Lee, K. H., and S. Vachon 2016. “Supply Chain Sustainability Risk.” In Business Value and Sustainability. London: Palgrave Macmillan. https://doi.org/10.1057/978-1-137-43576-7_8.
- Lina, H., W., Zhenyong, W., Xiang, M., Goh, X., Zhitao, W., Song, X., Ming, and W. Xiao. 2021. “A Novel Kano-QFD-DEMATEL Approach to Optimise the Risk Resilience Solution for Sustainable Supply Chain.” International Journal of Production Research 59 (6): 1714–1735.
- Liu, L., X. Liu, and G. Liu. 2018. “The Risk Management of Perishable Supply Chain Based on Coloured Petri Net Modeling.” Information Processing in Agriculture 5 (1): 47–59. https://doi.org/10.1016/j.inpa.2017.12.001.
- Liu, H. C., M. Y. Quan, Z. W. Li, and Z. L. Wang. 2019. “A New Integrated MCDM Model for Sustainable Supplier Selection Under Interval-Valued Intuitionistic Uncertain Linguistic Environment.” Information Sciences 486:254–270. https://doi.org/10.1016/j.ins.2019.02.056.
- Lotfi, R., Y. Z. Mehrjerdi, M. S. Pishvaee, A. Sadeghieh, and G. W. Weber. 2021. “A Robust Optimization Model for Sustainable and Resilient Closed-Loop Supply Chain Network Design Considering Conditional Value at Risk.” Numerical Algebra, Control and Optimization 11 (2): 221–253. https://doi.org/10.3934/naco.2020023.
- Maddikunta, P. K. R., Q. V. Pham, B. Prabadevi, N. Deepa, K. Dev, T. R. Gadekallu, R. Ruby, and M. Liyanage. 2021. “Industry 5.0: A Survey on Enabling Technologies and Potential Applications.” Journal of Industrial Information Integration 26:100257. https://doi.org/10.1016/j.jii.2021.100257.
- Ma, S., Y. He, R. Gu, and S. Li. 2021. “Sustainable Supply Chain Management Considering Technology Investments and Government Intervention.” Transportation Research Part E: Logistics & Transportation Review 149:102290. https://doi.org/10.1016/j.tre.2021.102290.
- Masoomi, B., I. G. Sahebi, M. Ghobakhloo, and A. Mosayebi. 2023. “Do Industry 5.0 Advantages Address the Sustainable Development Challenges of the Renewable Energy Supply Chain?” Sustainable Production and Consumption 43:94–112. https://doi.org/10.1016/j.spc.2023.10.018.
- Mehdiabadi, A., V. Shahabi, S. Shamsinejad, M. Amiri, C. Spulbar, and R. Birau. 2022. “Investigating Industry 5.0 and Its Impact on the Banking Industry: Requirements, Approaches and Communications.” Applied Sciences 12 (10): 5126. https://doi.org/10.3390/app12105126.
- Moktadir, M. A., A. Dwivedi, N. S. Khan, S. K. Paul, S. A. Khan, S. Ahmed, and R. Sultana. 2021. “Analysis of Risk Factors in Sustainable Supply Chain Management in an Emerging Economy of Leather Industry.” Journal of Cleaner Production 283:124641. https://doi.org/10.1016/j.jclepro.2020.124641.
- Motienko, A. 2020. “Integration of Information and Communication System for Public Health Data Collection and Intelligent Transportation System in Large City.” Transportation Research Procedia 50:466–472. https://doi.org/10.1016/j.trpro.2020.10.055.
- Mubarik, M. S., N. Naghavi, M. Mubarik, S. Kusi-Sarpong, S. A. Khan, S. I. Zaman, and S. H. A. Kazmi. 2021. “Resilience and Cleaner Production in Industry 4.0: Role of Supply Chain Mapping and Visibility.” Journal of Cleaner Production 292:126058. https://doi.org/10.1016/j.jclepro.2021.126058.
- Nahavandi, S. 2019. “Industry 5.0-A Human-Centric Solution.” Sustainability 11 (16): 4371. https://doi.org/10.3390/su11164371.
- Nayeri, S., Z. Sazvar, and J. Heydari. 2023. “Towards a Responsive Supply Chain Based on the Industry 5.0 Dimensions: A Novel Decision-Making Method.” Expert Systems with Applications 213:119267. https://doi.org/10.1016/j.eswa.2022.119267.
- Nikkhil, P., B. Manas, and G. Dibyajyoti. 2021. “An Analysis of Critical Success Factors Towards Sustainable Supply Chain Management – in the Context of an Engine Manufacturing Industry.” International Journal of Sustainable Engineering 14 (6): 6, 1496–1508. https://doi.org/10.1080/19397038.2021.1966128.
- Ostergaard, E. H. Industry 5.0-Return of the Human Touch. (2016). Accessed January 3, 2022. https://blog.universal-robots.com/industry-50-return-of-the-human-touch.
- Ozdemir, D., M. Sharma, A. Dhir, and T. Daim. 2022. “Supply Chain Resilience During the COVID-19 Pandemic.” Technology in Society 68:Article 101847. https://doi.org/10.1016/j.techsoc.2021.101847.
- Paschek, D., A. Mocan, and A. Draghici 2019. Industry 5.0-The Expected Impact of Next Industrial Revolution. In Proceedings of the Thriving on Future Education, Industry, Business, and Society, Proceedings of the Make Learn and TIIM International Conference, Piran, Slovenia, 15–17.
- Ponomarov, S. Y., and M. C. Holcomb. 2009. “Holcomb Understanding the Concept of Supply Chain Resilience.” The International Journal of Logistics Management 20 (1): 124–143. https://doi.org/10.1108/09574090910954873.
- Ralston, P., and J. Blackhurst. 2020. “Industry 4.0 and Resilience in the Supply Chain: A Driver of Capability Enhancement or Capability Loss?” International Journal of Production Research 58 (16): 5006–5019. https://doi.org/10.1080/00207543.2020.1736724.
- Raut, R. D., S. Luthra, B. E. Narkhede, S. K. Mangla, B. B. Gardas, and P. Priyadarshinee. 2019. “Examining the Performance Oriented Indicators for Implementing Green Management Practices in the Indian Agro Sector.” Journal of Cleaner Production 215:926–943. https://doi.org/10.1016/j.jclepro.2019.01.139.
- Sadeghi, Z., and O. Boyer Hasani. 2019. “A Multi-Objective Optimization Mathematical Model for Design and Planning of Sustainable Resilience Supply Chain Under the Risk of Supply Disruption.” Journal of Quality Engineering and Management 9 (3): 212–225.
- Sá, M. M. D., P. L. D. S. Miguel, R. P. D. Brito, and S. C. F. Pereira. 2020. “Supply Chain Resilience: The Whole Is Not the Sum of the Parts.” International Journal of Operations & Production Management 40 (1): 92–115. https://doi.org/10.1108/IJOPM-09-2017-0510.
- Sami, A., U. Manzoor, A. Irfan, and F. Sarwar. 2023. “Characterizing Circular Supply Chain Practices in Industry 5.0 with Respect to Sustainable Manufacturing Operations.” Journal of Management and Research 10 (1): 84–105. https://doi.org/10.29145/jmr.101.04.
- Sheffi, Y., and B. Rice 2005. A Supply Chain View of the Resilient Enterprise. Technical report, MIT Sloan Management review.
- Sindhwani, R., S. Afridi, A. Kumar, A. Banaitis, S. Luthra, and P. L. Singh. 2022. “Can Industry 5.0 Revolutionize the Wave of Resilience and Social Value Creation? A Multi-Criteria Framework to Analyze Enablers.” Technology in Society 68:101887. https://doi.org/10.1016/j.techsoc.2022.101887.
- Singh, N. 2020. “Developing Business Risk Resilience Through Risk Management Infrastructure: The Moderating Role of Big Data Analytics.” Information Systems Management 39 (1): 34–52. https://doi.org/10.1080/10580530.2020.1833386.
- Singh, C. S., G. Soni, and G. K. Badhotiya. 2019. “Performance Indicators for Supply Chain Resilience: Review and Conceptual Framework.” Journal of Industrial Engineering International 15 (S1): 105–117. https://doi.org/10.1007/s40092-019-00322-2.
- Spieske, A., and H. Birkel. 2021. “Improving Supply Chain Resilience Through Industry 4.0: A Systematic Literature Review Under the Impressions of the COVID-19 Pandemic.” Computers & Industrial Engineering 158:107452. https://doi.org/10.1016/j.cie.2021.107452.
- Stone, J., and S. Rahimifard. 2018. “Resilience in Agri-Food Supply Chains: A Critical Analysis of the Literature and Synthesis of a Novel Framework.” Supply Chain Management: An International Journal 23 (3): 207–238. https://doi.org/10.1108/SCM-06-2017-0201.
- Syed, M. W., J. Z. Li, M. Junaid, X. Ye, and M. Ziaullah. 2019. “An Empirical Examination of Sustainable Supply Chain Risk and Integration Practices: A Performance-Based Evidence from Pakistan.” Sustainability 11 (19): 5334. https://doi.org/10.3390/su11195334.
- Tange, K., M. De Donno, X. Fafoutis, and N. Dragoni. 2020. “A Systematic Survey of Industrial Internet of Things Security: Requirements and Fog Computing Opportunities.” IEEE Communications Surveys & Tutorials 22 (4): 2489–2520. https://doi.org/10.1109/COMST.2020.3011208.
- Tavakoli Haji Abadi, Y., and S. Avakh Darestani. 2021. “Evaluation of Sustainable Supply Chain Risk: Evidence from the Iranian Food Industry.” Journal of Science and Technology Policy Management 14 (1): 127–156. https://doi.org/10.1108/JSTPM-08-2020-0121.
- Tortorella, G., F. S. Fogliatto, S. Gao, and T. K. Chan. 2022. “Contributions of Industry 4.0 to Supply Chain Resilience.” The International Journal of Logistics Management 33 (2): 547–566. https://doi.org/10.1108/IJLM-12-2020-0494.
- Um, J., and N. Han. 2021. “Understanding the Relationships Between Global Supply Chain Risk and Supply Chain Resilience: The Role of Mitigating Strategies.” International Journal Of 26 (2): 240–255. https://doi.org/10.1108/SCM-06-2020-0248.
- Um, K. H., and S. M. Kim. 2019. “The Effects of Supply Chain Collaboration on Performance and Transaction Cost Advantage: The Moderation and Nonlinear Effects of Governance Mechanisms.” International Journal of Production Economics 217:97–111. https://doi.org/10.1016/j.ijpe.2018.03.025.
- Valinejad, F., and D. Rahmani. 2018. “Sustainability Risk Management in the Supply Chain of Telecommunication Companies: A Case Study.” Journal of Cleaner Production 203:53–67. https://doi.org/10.1016/j.jclepro.2018.08.174.
- Wackett, M. 2020. Coronavirus May Be Costing Container Shipping Lines $350m Every week”, The Loadstar. https://theloadstar.com/coronavirus-may-be-costing-container-shipping-lines-350m-every-week/.
- Xu, X. Y., S. H. Chung, K. Y. L. Chris, and A. C. L. Yeung. 2022. “Sustainable Supply Chain Management with NGOs, NPOs, and Charity Organizations: A Systematic Review and Research Agenda.” Transportation Research Part E: Logistics & Transportation Review 164:102822. https://doi.org/10.1016/j.tre.2022.102822.
- Xu, M., Y. Cui, M. Hu, X. Xu, Z. Zhang, S. Liang, and S. Qu. 2019. “Supply Chain Sustainability Risk and Assessment.” Journal of Cleaner Production 225:857–867. https://doi.org/10.1016/j.jclepro.2019.03.307.
- Yang, J., H. Xie, G. Yu, and M. Liu. 2021. “Antecedents and Consequences of Supply Chain Risk Management Capabilities: An Investigation in the Post-Coronavirus Crisis.” International Journal of Production Research 59 (5): 1573–1585. https://doi.org/10.1080/00207543.2020.1856958.
- Yazdani, M., P. Chatterjee, E. K. Zavadskas, and S. Hashemkhani Zolfani. 2017. “Integrated QFD-MCDM Framework for Green Supplier Selection.” Journal of Cleaner Production 142:3728–3740. https://doi.org/10.1016/j.jclepro.2016.10.095.
- Zhang, M., C. Dong, D. Zhang, M. L. Tseng, J. Wei, and N. Xiong. 2022. “An Intelligent Classification Diagnosis Based on Blood Oxygen Saturation Signals for Medical Data Supply Chain Including COVID-19 in Industry 5.0.” IEEE Transactions on Industrial Informatics 19 (3): 3310–3320. https://doi.org/10.1109/TII.2022.3152809.
- Zulfiquar, N. A., K. Ravi, and S. Ravi. 2020. “Evaluation and Ranking of Solutions to Mitigate Sustainable Remanufacturing Supply Chain Risks: A Hybrid Fuzzy SWARA-Fuzzy COPRAS Framework Approach.” International Journal of Sustainable Engineering 13 (6): 473–494. https://doi.org/10.1080/19397038.2020.1758973.
Appendix A
Table A1. 30 SSCRs factors.
Table A2. 34 SCRE indicators.
Table A3. 123 I5.0 drivers.
Appendix B
Fuzzy Delphi Method (FDM)
FDM is an indicator identification method that combines fuzzy theory and the Delphi method proposed by Murray et al. The detailed steps are as follows.
Step A: Design the FDM questionnaire. Set the value range to evaluate the importance of the evaluation target. The most conservative value and optimistic value are evaluated. The larger the value is, the more important the index.
Step B: We calculate the most conservative and optimistic values given by all experts and remove extreme values that more than double the standard deviation. According to fuzzy theory, the minimum , geometric mean
, and maximum values
of the conservative values are calculated, and the conservative values of triangular fuzzy numbers
are obtained. The minimum value
, geometric mean
, and the maximum value of the optimistic value
are calculated, and the optimistic value of triangular fuzzy number is obtained.
Step C: Calculate the degree of consensus . The higher the value of
, the more important the indicator is. The calculation formula is as follows:
Step D: Check for convergence. represents the grey area of fuzzy relation, and
represents the range of optimistic value and conservative value. When
, the expert’s opinion converges; otherwise it diverges, and steps A – C need to be repeated until convergence.
Step E: Set a reasonable threshold . While the value of
>
is retained, other factors smaller than the threshold are removed.
Fuzzy Interpretation Structure Model (FISM)
FISM introduces the concept of a fuzzy matrix, which can accurately reflect the relationship between complex systems. The main steps are as follows:
Step A: Select the membership function and obtain the fuzzy association strength function B using EquationEquation (2)(2)
(2) .
Step B: The Boolean incidence matrix. The threshold value is set to screen the correlation strength of matrix B. The smaller the threshold value is, the larger the scope covered by each element and the larger the system decomposition. According to EquationEquation (3)(3)
(3) , intensity matrix B is transformed into adjacency matrix A.
Analysis Network Procedure (ANP)
ANP verifies the weight relationship between various factors through analysis among different levels and determining the corresponding analysis matrix. The main steps are as follows:
Step A: Design an ANP questionnaire according to the questionnaire results of the previous stage and allow experts to objectively evaluate each factor through the evaluation matrix scale in . Assume n elements are present on the same level. Judge the importance degree between the factor of and factor of
: that is, get the value
.
Table B1. ANP assessment scale.
Step B: Collect the questionnaire and conduct consistency test to judge the validity of the data. First, according to the sum of eigenvalues of the judgement matrix and according to EquationEquation (4)(4)
(4) , if the sum of eigenvalues is
≥n, the absolute value of each component vector of the orthogonalization vector of the matrix can be obtained as the weight value of each element. Second, the importance vector
and
of each row is calculated according to EquationEquations (5)
(5)
(5) and (Equation6
(6)
(6) ).
Step C: Check the consistency of the results. According to EquationEquation (7)(7)
(7) , the consistency index (CI) can be calculated to determine whether the matrix is consistent. When =n and CI ≤ 0.1, the judgement matrix passes the consistency test.
Table B2. Average random consistency index.
When too many factors are evaluated by the judgement matrix, the calculation to be performed will be heavier. To test the consistency of each matrix, random index (RI) is introduced here to assist in testing, as listed in .
If CI > 0.1, its consistency ratio (CR) should be calculated according to EquationEquation (8)(8)
(8) . When CR ≤ 0.1, the matrix has passed the consistency test: that is, the expert’s evaluation of the factors and the calculation results are valid and reasonable. Otherwise, it fails. The questionnaire that fails the one-time test is deemed invalid and needs to be resent to the expert for filling to obtain as much valid data as possible and ensure it meets the consistency test.
The fourth step is calculating the average value in the judgement matrix that meets the requirements and obtaining the importance degree and ranking of each factor calculated via ANP.
TOPSIS method
The TOPSIS method takes the maximum positive ideal solution and the minimum negative ideal solution as the optimal decision. The specific steps are as follows:
Step A: Construct the initial matrix D, which contains n evaluation objects and m evaluation indicators.
Step B: Normalization. To standardise the calculation of each index, the matrix should first be normalised, where is the normalised evaluation value of the first scheme to the second scheme.
Step C: Construct the weighted standardised matrix according to the index weights
obtained via the ANP analysis method, or calculate objective weight based on the entropy weight.
Step D: Calculate positive ideal solutions and negative ideal solutions
.
Step E: Calculate the distance between positive and negative ideal solutions. The attribute of the index can be divided into the benefit type and achievement type. The benefit type should be as large as possible, whereas the cost type
should be as small as possible.
is used to represent the Euclidean distance,
represents the relative proximity, and the value range of
is (0,1). A large value implies that the decision is closer to the ideal solution, and the decision is more optimal if it is further away from the negative ideal solution.