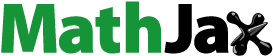
ABSTRACT
Smartphones are indispensable tools for daily tasks; nevertheless, their short lifespan of about two years contributes to a disproportionate increase in environmental waste. Although current initiatives explore reusing specific smartphone components, such as metallic parts, the scope of these efforts still needs to be expanded, necessitating more comprehensive strategies to address the growing waste generation. Additionally, end-users play a pivotal role in determining the fate of obsolete smartphones and in adopting sustainable disposal practices to mitigate environmental damage after the devices are no longer useful to them. This proposal describes the scope to obtain a circular economy approach at a macro-level environmental impact. The strategy aims to employ obsolete smartphones while reducing environmental im pacts and facilitating efficient device disposal. The authors employ the S4 framework to support sustainable product development at a macro-level, emphasising sensing, smart, sustainability, and social dimensions. However, it is essential to note that the current solution applies only to smartphones with functional features, including intact displays, operational batteries, accessible cameras and sensors, and functional power systems, obtaining a level three according to the S4 framework, which describes limited human intervention.
1. Introduction
Economic business models traditionally prioritise meeting societal needs through production and manufacturing processes. However, these models often heavily rely on natural resources, which can have detrimental effects on the environment OECD (Citation2007). Such production practices are characteristic of a linear economy, where increased energy consumption correlates with heightened economic activity and industrial productivity. This results in an escalated material output, which may or may not undergo recycling or reusing processes (Neves and Marques Citation2022).
There is a growing consensus that industrial development and supply chain management must address ecological pollution concerns to advance green supply chain management practices (Hsu et al. Citation2013). The concept of the circular economy (CE) emerges as a viable option for mitigating the impact of waste. It achieves this by reducing waste through adherence to a life cycle of products and facilitating the return of raw materials to the loop of the cycle (Garcés-Ayerbe et al. Citation2019).
The concept of CE was raised in the 70s with the generation of new business models. Nevertheless, the CE approach capacity has been limited because all the stockholders must be considered in the business model (Velenturf and Purnell Citation2021). The work proposed by Geissdoerfer, Savaget, Bocken, and Hultink (Citation2017) propose a system based on the CE approach, aiming to reduce resource input and waste, emissions, and energy loss through the incorporation of durable design, upkeep, repair, reuse, remanufacturing, refurbishment, and recycling strategies. In the next paper (Korhonen, Honkasalo, and Seppälä Citation2018) mention that one of the limitations of the CE is the problems generated throughout the product’s life cycle because it is complicated to follow up once the product ends its life cycle. For this reason, it is essential to implement techniques that allow us to maintain the circularity of products even when they end their life cycle.
Electronic waste (e-waste) significantly contributes to the proliferation of mobile devices (Rizos et al. Citation2019). Approximately 50 million tons of e-waste were generated globally, with smartphones accounting for 10% of this total (Repairing—Not Recycling—Is the First Step to Tackling Smartphone E-waste Citation2021). Estimates from 2017 suggest that around 700 million devices were in a state of hibernation, indicating that they were not in active use, and only 5 to 10% were recycled. Factors such as embedded batteries, damaged displays, and the difficulty of repairing these devices contribute to a relatively short lifespan of 21.6 months for users (Cordella et al. Citation2021). In a study by Hazelwood and Pecht (Citation2021), the potential for reusing materials from mobile devices, which contain electronic components primarily made of aluminium, gold, silver, tungsten, copper, tin, cobalt, and steel, is described. Manufacturers of these devices must take proactive measures to monitor the circularity of all device elements.
Han et al. (Citation2023) describe how digital technology’s (DT) impacts on developing products with sustainable design because these technologies improve the process positively in the economic, social, and environmental dimensions. Besides, manufacturers are restricted to the product’s life cycle once it is no longer helpful to the user. Machine learning, virtual reality, and augmented reality monitor the process and human-to-machine communication, allowing data collection, facilitating human notification of system problems, and improving safety conditions (Kamble, Gunasekaran, and Gawankar Citation2018).
The S4 framework is used to design functional prototypes and provide user-friendly sensing, smart, sustainable, and social features (Méndez et al. Citation2019). They defined each”S” as follows:
• Sensing (S1): the ability of a system to recognise occurrences, gather information, and assess variations by utilising sensors that enable the observation of physical or environmental circumstances.
• Smart (S2): denotes the integration of physical components, intelligent elements, and connectivity enhancements, enabling a product to possess intelligence and the ability to connect with other devices.
• Sustainability (S3): Considers the environmental and economic factors to produce balanced and optimised performance. Here, the social aspect pertains to how a product positively impacts people’s quality of life.
• Social (S4): refers to the ability to independently observe, record, analyse, and modify consumer behaviour, as well as adapt its online/offline features to enhance its performance or market acceptance.
Therefore, the S4 framework involves features that must be considered to develop sustainable products.
1.1. Smartphones
Smartphones have transformed human lives by enabling communication in various forms. However, the environmental impact of smartphone manufacturing is inevitable. This paper (Pont, Robles, and Gil Citation2019) emphasises the significance of designing and developing technologies with awareness of their environmental impact. Electronic devices like smartphones contain elements that adversely affect human health and the environment. Therefore, there is a need for awareness regarding the negative impact of these elements. Additionally, it is essential to explore innovative alternative solutions to address this underlying issue.
Analysing components recoverable from obsolete smartphones is crucial for reintegrating elements into CE models (Kastanaki and Giannis Citation2022). However, the effectiveness of this alternative approach depends on users’ awareness of sustainability impacts, as individuals determine the end-of-life of their devices (Tu, Zhang, and Huang Citation2018). This solution is limited in scope and immediate impact, highlighting the need to explore additional alternatives.
One of the alternatives involves employing the circular economy model, which entails analysing product design and procedures conducted when the product’s lifetime is fulfilled, as outlined in (Mangers, Amne Elahi, and Plapper Citation2023). Efforts exist to implement CE models, with the primary strategies commonly used by companies to extend the lifecycle of devices, including durable design, maintenance, repair, re-use, remanufacturing, refurbishing, and recycling (Deng et al. Citation2021). In Cordella, Alfieri, and Sanfelix (Citation2021), various scenarios are proposed to estimate the increasing impact of smartphones to minimise environmental repercussions. Additionally, there are positive benefits when the device’s lifecycle is extended by at least two years, as it mitigates the need for updated devices and reduces environmental impact.
According to Hanski and Valkokari (Citation2020), generic circular lifecycles are typically used to describe CE solutions, such as product-as-a-service, renewability, product life extension, resource efficiency, and recycling. Despite evidence of the implementation of CE models, the interconnected nature of elements throughout the transition process to a CE has yet to be fully understood and conceptualised (Toth-Peter et al. Citation2023).
The evidence supporting the use of digital tools for data collection and analysis demonstrates the potential to enhance process efficiency. This work proposed by Subramoniam, Sundin, Subramoniam, and Huisingh (Citation2021) utilises these technologies to monitor a process, collecting and analysing data to develop a waste reduction strategy in manufacturing. Similarly, the next paper (Turner et al. Citation2022) employs data analysis to visually indicate vehicle maintenance needs through an ontology-based approach. Data enables the exploration of new alternatives to enhance procedures, exemplified by (Plociennik et al. Citation2022), which gathers information on product components, materials, chemical substances, repairability, spare parts, and proper disposal methods. These elements facilitate information exchange among all stakeholders involved in a product’s lifecycle. While digital tools offer strategies to reduce waste in processes, their potential to positively impact the environment still needs to be explored. Likewise, this paper by Abou Baker, Stehr, and Handmann (Citation2022) propose a vision system to detect recyclable devices such as smartphones to reduce e-waste.
While smartphone production significantly impacts the environment, PC manufacturing also has a substantial environmental footprint (O’Connell and Stutz Citation2010). Despite the environmental impact of a PC being five times greater than a smartphone’s, its longer lifecycle is attributed to its design for extended use. However, this proposal suggests replacing high-performance tasks with smartphones to mitigate the global warming potential of greenhouse gas emissions. The present proposal analyzes the impact of smartphones on executing complex architecture in place of high-performance operations to estimate the environmental impact at a macro level.
This document (Geissdoerfer, Vladimirova, and Evans Citation2018) proposes several strategies to establish new business models towards a circular economy, including maximising material and energy efficiency, closing resource loops, and developing sustainable scale-up solutions. Virtualisation emerges as a solution enabling additional features on devices with limited resources, such as smartphones, which contain resources to provide specific functionalities. The current state of the art recognises lifetime extension as a method for reducing e-waste.
2. Background and research gaps
The evidence of digital tools to collect and analyse data allows for improving process efficiency. In this paper (Subramoniam et al. Citation2021) employ digital technologies to track a procedure that collects and analyzes data to create a strategy to reduce waste in a manufacturing process. Similarly, the authors of this work (Turner et al. Citation2022) employ data analysis to visually indicate the maintenance that a vehicle should receive through an ontology-based response. The data enables new alternatives to improve procedures, such as the work presented in (Plociennik et al. Citation2022), which collects information on components, materials, chemical substances, repairability, spare parts, or proper disposal for a product, allowing an exchange of information among all actors involved in the life-cycle of a product. Also, the next contribution (Abou Baker, Stehr, and Handmann Citation2022) propose a vision system to detect devices such as smartphones with characteristics to be recycled and thus reduce e-waste. Although digital tools provide strategies to reduce waste in a procedure, these technologies have much potential to increase the positive impact on the environment.
All the works aim to establish new business models towards a circular economy (CE), aiming to maximise material and energy efficiency, close resource loops, and develop sustainable scale-up solutions. Based on the requirements of previous strategies, digital technologies (DTs) enable new features to provide specific functionalities for devices with limited resources, such as smartphones. The current state of the art identifies lifetime extension as a method for reducing e-waste. illustrates that life extension begins once the user has satisfied the requirements of each device. Therefore, DTs enable these devices to incorporate novel features.
Figure 1. Product life cycle description based on Deng et al. (Citation2021).

The research described by (Jabbour et al. Citation2023) emphasises the importance of educating people about the proper disposal of e-waste and their inclination to purchase circular products, including recycled, reconditioned, or second-hand items. It investigates consumers’ willingness to pay a premium for circular products and the factors influencing their decisions. The study advocates for involving consumers in recycling, recovery, and reuse efforts, either at designated facilities or at the point of sale, thereby promoting a business model aligned with the circular economy. In the context of smartphones, the paper discusses the significance of reusing, repairing, and recycling components to enhance sustainability. It underscores the importance of designing smartphones with circularity in mind, focusing on features such as easy disassembly, component upgradeability, and the use of recyclable materials.
While some circular models tend to involve consumers, there is a period at the end of the daily use of products. However, the device remains useful for satisfying specific needs. For this reason, the authors propose extending the product’s lifecycle to mitigate the effects and limitations imposed by manufacturers. Additionally, since products with a defined lifecycle lack circularity in all device elements, this research suggests returning devices classified as e-waste and enabling new characteristics through digital technologies such as machine learning, virtual reality, and augmented reality.
On the other hand, given the importance of incorporating a sustainable approach into the supply chain, the smart factory must integrate smart objects with big data analytics for high flexibility and efficiency, enabling the production of customised small-lot products resulting in increased profitability (Wang et al. Citation2016). Similarly, automated vehicles manage the transportation of raw materials and final products in industrial sites, picking up pallets from production lines and delivering them to warehouses or shipment points (Cardarelli et al. Citation2017). This problem involves a complex system that perceives the environment and makes decisions based on the collected data to avoid possible collisions. In addition, autonomous agents require specific equipment to move within the environment. However, the elemental resources to employ the path planning solution are in smartphones. For example, the camera perceives the environment, while the processing unit defines the control of movements to generate safe paths.
The primary contribution of the presented work is to describe the impact of employing the methodology of the S4 framework for delineating the macro-level impact of a digital strategy aimed at reducing the environmental footprint generated by smartphones. The proposal examines the utilisation and repercussions of DTs in enabling novel features that extend the fundamental functionalities of products and advance a CE model to attain circularity for e-waste devices after the device is considered unuseful for ordinary users. Additionally, it addresses the disposal of underutilised devices, guaranteeing their proper transition to an end-of-life stage, thereby averting environmental contamination.
The remaining sections of this manuscript are structured as follows. Section. The suggested work is introduced in Section 3. Section 4 illustrates the outcomes and analyses of the experiments. The conclusion is then stated in Section 5.
3. Methodology
This section outlines the S4 methodology and details each characteristic that enables the description of environmental impact at the macro level. The focus on macro-level analysis stems from a previous examination of micro-level impact, which delved into the reuse of discarded devices Additionally (Maldonado-Romo and Aldape-Pérez Citation2021), describe other features such as the efficiency of local resources and the impact on the user interface. In this proposal, the user interface enables new features because it interacts with digital content that interacts with the environment. However, the S4 framework facilitates a broader-scale estimation, allowing for a more comprehensive understanding of environmental impact.
3.1. Sensing, smart, sustainable, and social (S4) framework
Continuous updating and innovation are necessary to develop new products and solutions and enhance the value of a product, process, or service. For this reason (Ponce et al. Citation2019), describes the Sensing, Smart, and Sustainable (S3) framework for developing and designing novel products, processes, manufacturing systems, and business models. Besides (Ponce et al. Citation2022), introduced the social dimension, which primarily serves as a communication platform for user-product and product-to-product communication. This dimension complements the framework by considering communication as an essential element in the interaction of new products, thereby expanding the scope of the Sensing, Smart, Social, and Sustainable (S4) framework. Thus, this feature collaborates to incorporate novel experiences into product development.
3.1.1. Sensing dimension
Sensing dimension is an essential function of any intelligent system, referring to acquiring pertinent data and information for decision-making. Based on this concept, Sensing for the DT is defined by a module that senses a virtual environment to comprehend it. This model utilises a conventional camera to estimate the location of the object. Virtual reality engines generate virtual samples to extract knowledge from a physical environment employing a conventional camera. Depth and segmentation information from a physical world sample is utilised to estimate its position in a virtual environment. Relative Error (rel) (EquationEquation (1)(1)
(1) ), Average (
) Error (EquationEquation (2)
(2)
(2) ), and Root Mean-Squared Error (RMSE) (EquationEquation (3))
(3)
(3) are used to describe the performance.
3.1.2. Smart dimension
A smart system is constructed based on intelligent systems using artificial intelligence techniques, employing data and automated reasoning for decision-making and action execution. This proposal utilises the A-star and Rapidly Exploring Random Tree (RRT) algorithms to generate secure paths in the virtual environment. In the second phase, solutions based on deep learning are proposed to estimate a course, with a deep convolutional neural network determining a characteristic vector shared by these solutions using virtual samples. The third phase describes the connection of the two domains through a Generative Network (GN), as is shown in . This network transitions from the physical to the virtual domain, reducing the number of samples in the physical domain. Given the substantial time required by the GN, the transfer-learning strategy is proposed as a solution to this problem. In the fourth stage, the GN, characteristic vector, and domain changes are replaced with the transfer-learning strategy. In the final step, rather than employing the GN and characteristic vector as separate systems, both are included and placed as estimates of the virtual path using deep-learning approaches derived from physical samples. This approach reduces the architecture’s cost and enhances its performance. To describe the performance, Euclidean distance (EquationEquation (4)(4)
(4) ), Manhattan distance (EquationEquation (5)
(5)
(5) ), and cosine similarity (EquationEquation (6))
(6)
(6) are employed.
Figure 2. Digital technology approach to reduce the path planning generator. (a) An unknown sample is approximate to a known sample. (b) A transfer learning approach reduces the number of operations. (c) A safe path is generated based on previous knowledge of virtual environment safe paths. Taken from (Maldonado-Romo, Maldonado-Romo, and Aldape-Pérez Citation2022).

3.1.3. Sustainability dimension
The section outlines sustainability at a macro-level, which entails integrating economic, environmental, and social factors to achieve balanced and optimised performance.
• Economic feature: Profitability minimises operational costs while enhancing productivity or value generation. Principal features of the economic dimension, as described by (Miranda et al. Citation2019), encompass (I) raw material cost, (II) production cost, (III) energy cost, (IV) recycling cost, and (V) remanufacturing cost.
• Environmental feature: Environmental sustainability concerns the rate of resource consumption enabling the environment’s regeneration. The authors in this contribution (Frey, Harrison, and Billett Citation2006) utilise a life-cycle assessment methodology to quantify and transform the environmental responsibilities linked to mobile phone production and usage into the instantaneous rate of resource consumption. The time-step method, elucidated in EquationEquation (7)(7)
(7) , applies to non-mature market products and does not necessitate continuous input, rendering it suitable for markets with variable product lifetimes like smartphones Araújo, Magrini, Mahler, and Bilitewski (Citation2012).
where WEEE is the estimation of e-waste, is the sales of the device in a period
,
and
are the stock of the devices in use in two consecutive periods.
• Social feature: According to Méndez et al. (Citation2019), the social aspect within the sustainability feature refers to the impact on the consumer’s quality of life. This dimension does not consider the interaction between the consumer and the product.
3.1.4. Social dimension
The S4 framework Méndez et al. (Citation2019) describes the social dimension as the communication between users and products, manifesting in natural and non-natural forms. Consumer data define the former, while the latter reinforces consumer behaviour. Therefore, for this proposal, the appropriate definition is the non-natural concept. This dimension encompasses the use of augmented reality to enable new experiences. To gauge the performance of the experience, this paper (Perritaz, Salzmann, and Gillet Citation2009) determine the Quality of Experience (QoE), with the principal feature to be measured being the delay time. Furthermore, this contribution (Tan, Ronkkö, and Gencel Citation2013) describes the system’s safety and accessibility. EquationEquation (8)(8)
(8) is proposed to account for possible collisions in a path to measure the safety of the system. The ideal coefficient is one, signifying no crashes, and decreases if a collision occurs on the course.
The following case study primarily emphasises a macro analysis, focusing on the sustainable and social dimensions of the S4 framework. Notably, the sensing and smart dimensions are elucidated based on evidence derived from previous developments. Moreover, the S4 framework is tailored for developing sophisticated products, facilitating the validation of using DTs for intricate tasks.
3.2. Case study: path planning problem
The path planning problem, as proposed by (Schwartz and Sharir Citation1983), is conceptualised as the interaction of a physical object analysing its environment to extract data for generating restrictions defining potential obstacles along a path. ) illustrates the traditional architecture, comprising planner and perception modules. The real-time planner module generates collision-free paths based on constraints the perception module provides. While these modules operate independently, their interaction occurs at the same frequency. In contrast, ) depicts a simplified architecture where DTs preserve all potential path estimates within a virtual environment. This architecture combines the two principal modules of conventional architecture into a single module. The primary objective is to capitalise on obsolete smartphones, given their vast numbers of nearly 8.6 billion in 2021 (This infographic shows the rise of mobile device subscriptions worldwide Citation2022). A smartphone can execute one thousand operations per second, making its computational power advantageous for tasks with limited memory, procedure types, and computer architectures.
Figure 3. Path planning architecture. (a) Conventional path planning generator. (b) Reduced path planning architecture.
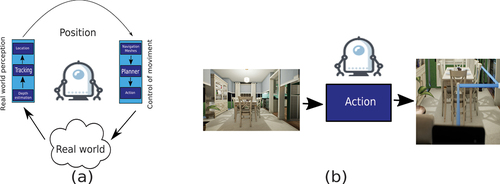
Alt Text. Figure depicts the traditional route planner consisting of a perception module and a planning module in a decoupled manner. On the other hand, it describes the unified way module that uses only one module integrated by a generative network.
4. Results and discussions
The discussion entails a description of each dimension comprising the S4 framework to facilitate the creation of smart products without human intervention.
In summary, the product based on the S4 framework utilises augmented reality in the physical world for user interface interaction with the environment. The generated path constitutes a sequence of points designed to avoid obstacles. illustrates a virtual course that circumvents potential collisions through two approaches. ) depicts a controlled environment where the path benefits from additional information, such as velocity expressed through colours. Contrarily, ) employs samples whose features broaden the range of solutions, as an unknown sample approximates a known solution using a transfer style approach.
Figure 4. Generated path using virtual elements. (a) Path employs a paired approach. (b) Path employs unpaired approach. Taken from (Maldonado-Romo, Maldonado-Romo, and Aldape-Pérez Citation2022).

4.1. Sensing dimension
The sensing dimension is comprehensively explored in a prior study, focusing on the functionality of a conventional camera interfaced with a virtual environment. A generative network architecture connects two domains: The first domain encompasses all potential combinations generated by the path-planning generator within a virtual representation of the physical environment. However, noise exists in the connection between these domains, prompting the implementation of noise reduction techniques based on convolutional networks to enhance accuracy in determining performance adequacy.
illustrates the performance metrics, focusing on the error between the expected and generated values. The findings reveal that the generative network architecture and noise reduction yielded the best performance with 50 samples. Notably, human involvement is absent from the module. However, due to the need for human supervision, the expected performance is rated at level 3 according to the sensing scale established by the S4 framework, which means it is at a lower level than the highest one. Inadequate performance arises due to constant changes in illumination within the environment, leading to shadows and occlusions in the system.
Table 1. Performance of this proposal and its standard deviation in 50 samples. Where Low is better, and
Up is better. Taken from (Maldonado-Romo, Maldonado-Romo, and Aldape-Pérez Citation2022).
4.2. Smart dimension
This dimension is delineated in previous works, where metrics detail the performance of machine learning models such as hierarchical clustering and autoencoder by (Maldonado-Romo, Maldonado-Romo, and Aldape-Pérez Citation2022). Given that a path is defined as a set of points in a 3D space, each point is represented as a vector. Consequently, the best paths are obtained using the conventional path planning architecture, which generates a safe path as the expected vector. Once the expected vector is generated, the machine learning approach produces the estimated vector. Metrics comparing vectors describe the performance, as shown in . Additionally, human intervention is excluded from the module. Therefore, the module achieves level 4 according to the smart scale of S4. However, since the modules lack feedback from user interaction, the solution is only partially level 4, highlighting an area for improvement in future updates. Therefore, the final scale is level 3.
Table 2. Path planning generator using augmented reality. Performance of the quantitative metrics in our approach and the standard deviation of 50 samples. , lower is better;
, higher is better. Based on (Maldonado-Romo, Maldonado-Romo, and Aldape-Pérez Citation2022).
4.3. Sustainable dimension
4.3.1. Economic feature
The impact in this dimension pertains to the cost of employing the technology, which requires a smartphone to be classified as e-waste. The device requires a conventional camera, with a smartphone from 2017 utilised for this study. Consequently, the investment cost for implementing this solution is low compared to technologies necessitating specific hardware. Additionally, the solution harnesses DTs, such as machine learning techniques, which reduce computational power requirements. Moreover, the embedded device’s GPU can execute tasks related to convolutional networks, sufficient for employing this solution with acceptable energy usage and high frames per second. Once the embedded device completes its operational lifespan with DTs extension, issues like camera, display, and power connection problems arise. These devices with issues can be collected and sent to specialised sites for electronic component recycling, completing the circular economy of a smartphone until its components are recycled. Therefore, the disposal of the device is entirely collected, resulting in a positive economic outcome due to the low investment in acquisition and waste management. However, strategies must be defined to ensure that devices deemed unusable by the average user and waste are directed to specialised facilities.
4.3.2. Environmental feature
A device with a significant presence in the market generates 65 kg of carbon emissions, with 81% produced during production and 3% during transportation (Iphone 14 pro. Product Environmental Report, Citation2022). Estimations suggest that approximately 154 million devices are classified as e-waste, as depicted in , based on data collected from (Apple iPhone sales worldwide 2007–2018 Citation2022) and (iPhone User & Sales Statistics Citation2023). This accumulation results in nearly 54 kg of carbon emissions. Using DT as an alternative for extending the lifecycle, it is estimated that approximately 9.856 billion carbon emissions could be reduced in 2020 by employing embedded devices, thereby averting negative impacts on the environment’s lifecycle. This module achieves level 4 on the sustainability scale of the S4 framework, as the product primarily focuses on delivering a sustainable solution.
Figure 5. Estimation of e-waste for smartphones.
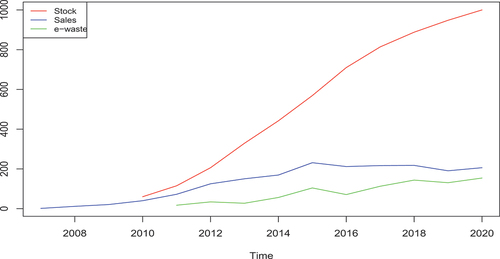
The S4 framework, aimed at developing sustainable and innovative products, defines impact across four dimensions. Tasks are executed for 3D estimation using a conventional camera. Algorithms based on generative networks facilitate the connection between a virtual representation and the physical environment to transfer knowledge for specific problems, such as the path planning generator. In terms of the sustainable dimension, employing obsolete smartphones to handle tasks could reduce greenhouse gas emissions, as this alternative circumvents the production phase, which is a primary emitter of these emissions. Additionally, the social dimension employs virtual elements to assist users and measures security through the coefficient of collision avoidance. Ultimately, the S4 framework enhances the conventional circular model of devices by adding value and promoting sustainability.
Therefore, the authors propose the following strategies to establish a circular model to mitigate the negative environmental impact of smartphones. The first strategy involves employing digital technologies to extend the lifetime of embedded devices and address specific tasks, as evidenced in the description, thereby obviating the need for new components. illustrates digital technologies as a strategy for extending embedded lifecycles to avoid acquiring specific devices for certain tasks. Since the end user determines the end-of-life of the devices, an additional strategy involves collecting each device and subjecting it to a treatment process conducted by specialised groups equipped with appropriate equipment to extract contaminating elements once it becomes incapable of completing its tasks. This approach aims to prevent the device from reaching an inappropriate end-of-life stage.
Figure 6. DT a strategy toward lifetime extension and improved smartphone circular model.

4.3.3. Social feature
The utilisation of DTs in extending the lifecycle of smartphones addresses environmental, economic, and social concerns, significantly impacting sustainability dimensions, as outlined by (Han et al. Citation2023). This impact is particularly evident in the enhancement of consumers’ quality of life through increased accessibility to technology and the promotion of a more sustainable consumption pattern. Repurposing and extending the life of smartphones reduce the constant production of new devices, decreasing resource demand and e-waste generation, thereby contributing to a healthier environment.
Moreover, this model fosters social inclusion by providing broader access to technology for various socioeconomic groups. By lowering the barriers to owning a smartphone, more individuals can access digital services and information, which is crucial in today’s digital age. This inclusivity enhances individual lives by providing access to educational resources, healthcare information, and social networks and stimulates economic opportunities by enabling participation in the digital economy.
Additionally, the initiative to recycle and responsibly dispose of electronic components at the end of their lifecycle educates consumers about the importance of sustainable practices (Jabbour et al. Citation2023). It encourages a shift in consumer behaviour towards more responsible consumption and disposal habits, further embedding sustainability into the social fabric. This educational aspect, coupled with the tangible benefits of reduced environmental impact and economic savings, underscores the multifaceted advantages of integrating the social dimension into the sustainability framework of the S4 model.
Thus, the social feature of employing DTs for smartphone lifecycle management enhances societal well-being by making technology more accessible, promoting social inclusion, and encouraging sustainable living practices among consumers.
This proposal aligns with circularity for devices that have reached the end of their useful life, reintegrating their base elements to reduce the impact of greenhouse gas emissions. Smartphone circularity considers all stakeholders and defines specific actions for each. However, this alternative is only beneficial for particular features of smartphones. For instance, the smartphone must be functional and have access to the system, camera, and sensors, with limited issues concerning the display and battery. Additionally, the devices must be received through a collection campaign, and companies that could benefit from the obsolete smartphone must be identified.
4.4. Social dimension
As (Méndez et al. Citation2019) emphasised, the social dimension observes, records, analyzes, and modifies consumer behaviour, adapting its online/offline features to enhance performance or market acceptance. The system generates 30 FPS, analysing cloud points by the AR core from Google only when the centroid distance between the current frame and the new frame changes exceeds 0.5. This approach prevents unnecessary analysis of samples, thereby improving performance. Additionally, the device’s efficiency relies on GPU technologies to accelerate operations using 16-bit word sizes. GPUs reduce processing time with shorter word sizes and alleviate the load on the CPU multicore. However, specific devices may leverage multicore performance, particularly when GPUs lack operations for convolutional networks.
outlines the performance of each machine-learning solution. In the paired approach, hierarchical clustering exhibits superior performance due to fewer potential collisions in the path. Similarly, in unpaired approaches, hierarchical clustering remains the top performer. Conversely, autoencoder models yield lower scores in both approaches, as the generated sequence results in points that may lead to collisions. It is notable that performance also varies depending on the approach: in paired approaches, the performance gap between hierarchical clustering and autoencoder models is narrower, whereas, in unpaired approaches, the difference is more pronounced, as the latter environment generates a broader solution space, leading to increased potential collisions.
Table 3. Coefficient free collision. Based on (Maldonado-Romo, Maldonado-Romo, and Aldape-Pérez Citation2022).
5. Conclusions
Due to the increased e-waste generated by smartphones, the authors have tried to propose a novel alternatives to reduce these devices with an inadequate end life that impacts the environment. In order to describe the benefits, it was used the S4 framework which describes the design of new products based on sensing, smart, sustainability, and social dimensions, whose impact tries to offer value to sustainable solutions.
In this case study, obsolete smartphones employ DTs that enable new features with a high impact on S4 dimensions that extend the lifecycle of the smartphone considered obsolete for common user because a simple camera senses the environment; machine learning transfers the knowledge to the device; the augmented reality displays the interface that guides the operator and determinates a possible collision based on a coefficient; and the estimation of greenhouse gas emissions that could avoid if we use smartphones.
Based on the behaviour of the generated data, the proposal to replace tasks of complex architectures with devices of lower computational capacity is still in the development phase and has yet to be considered feasible. However, the results are encouraging. In the next three years, devices considered obsolete will likely have up to five times the computational power, which could significantly increase the feasibility and impact of such initiatives.
Finally, the journey towards circularity entails several phases before reaching its final stage. While efforts have been made to raise awareness among users, further extending the life cycle by repurposing components and avoiding manufacturing new devices are crucial. This approach reduces environmental impact and lowers investment for industries exploring new technologies. Moreover, fostering closer interaction between products and consumers at competitive prices is vital, especially for emerging markets. These efforts collectively contribute to a more sustainable and environmentally conscious future.
Acknowledgments
The authors want to thank to the Institute of Advanced Materials for Sustainable Manufacturing and Tecnologico de Monterrey.
Disclosure statement
No potential conflict of interest was reported by the author(s).
Additional information
Notes on contributors
Javier Maldonado-Romo
Javier Maldonado-Romo received his professional studies in telematics, a master’s degree in computer technology, and Ph.D. in robotic and mechatronic systems from the Instituto Politecnico Nacional de Mexico. He also studied management, focusing on sustainability at the Universidad Nacional Autonoma de Mexico. He is a postdoctoral fellow at the Institute of Advanced Materials for Sustainable Manufacturing at the Tecnologico de Monterrey in Mexico City. He focuses on developing novel products, processes, and services in digital manufacturing.
Juana Isabel Méndez
Juana Isabel Méndezis an architect with a Master’s in Energy Management and a laureate Ph.D. in Engineering Sciences from Tecnologico de Monterrey, with a doctoral stay at UC Berkeley. She is a Certified Energy Manager from the Association of Energy Engineers (AEE). Isabel was awarded the Women Tec 2024 Award - category Environment. She won the best thesis award at TecScience Summit 2023. Currently, she is a postdoctoral researcher at the Institute of Advanced Materials for Sustainable Manufacturing. Her research focuses on building energy simulations and parametric design and tailored gamified dynamic interfaces using AI-based decision systems.
Mario Rojas
Mario Rojas is doing a postdoctoral fellowship at the Institute of Advanced Materials for Sustainable Development, from Tecnologico de Monterrey. He completed a bachelor’s and a master’s degree in Electronic Sciences. Additionally, he received his Ph.D. degree in Engineering Sciences focusing on Robotics and assistive technology from Tecnologico de Monterrey. His research pursuits also encompass human-machine interfaces, artificial intelligence, and sensors.
Luis Montesinos
Luis Montesinos, Assistant Research Professor at Tecnologico de Monterrey, holds Electronics, Image Processing, and Biomedical Engineering degrees. With 20 years of teaching experience, he has directed programs and departments at Tecnológico de Monterrey. A member of Mexico’s National System of Researchers, his work focuses on biomedical signal analysis, health data science, and sustainable healthcare. Recent projects involve machine learning for disease risk assessment and diagnosis. He has authored numerous papers and serves as a reviewer for esteemed journals. Dr. Montesinos is a Senior Member of IEEE, contributing to its Engineering in Medicine and Biology, Signal Processing, and Education Societies.
Pedro Ponce
Pedro Ponce is a control system and automation engineer. He did a master’s and doctoral degree in electrical engineering. His areas of interest are smart grids, microgrids, smart cities, AI, control systems, robotics, manufacturing, power electronics, digital twins, renewable energy, energy management, electric machines, and optimization. He is a research leader in the Institute of Advanced Materials and Sustainable Manufacturing and a professor in the mechatronics department at Tecnologico de Monterrey on the Mexico City campus. Pedro Ponce specializes in control and automation, smart grids, electrical machines, machine learning, soft computing, digital twins, and metaheuristic optimization.
Arturo Molina
Arturo Molina is a distinguished scholar and Director of the Institute of Advanced Material for Sustainable Manufacturing at Tecnologico de Monterrey. With a Ph.D. in Manufacturing Engineering from Loughborough University of Technology and a University Doctorate in Mechanical Engineering from the Technical University of Budapest, he has contributed significantly to the field. His research focuses on manufacturing, computer science, and sustainable technologies.
References
- Abou Baker, N., J. Stehr, and U. Handmann. 2022. “Transfer Learning Approach Towards a Smarter Recycling.” In Artificial Neural Networks and Machine Learning – Icann 2022, edited by E. Pimenidis, P. Angelov, C. Jayne, A. Papaleonidas, and M. Aydin, 685–696. Cham: Springer International Publishing.
- Apple iphone sales worldwide 2007-2018. 2022. Statista. Accessed July 6, 2023. https://www.statista.com/statistics/276306/global-apple-iphone-sales-since-fiscal-year-007/.
- Araújo, M. G., A. Magrini, C. F. Mahler, and B. Bilitewski. 2012. “A Model for Estimation of Potential Generation of Waste Electrical and Electronic Equipment in Brazil.” Waste Management 32 (2): 335–342. https://doi.org/10.1016/j.wasman.2011.09.020.
- Cardarelli, E., V. Digani, L. Sabattini, C. Secchi, and C. Fantuzzi. 2017. “Cooperative Cloud Robotics Architecture for the Coordination of Multi-Agv Systems in Industrial Warehouses.” Mechatronics 45:1–13. https://doi.org/10.1016/j.mechatronics.2017.04.005.
- Cordella, M., F. Alfieri, C. Clemm, and A. Berwald. 2021. “Durability of Smartphones: A Technical Analysis of Reliability and Repairability Aspects.” Journal of Cleaner Production 286:125388. https://doi.org/10.1016/j.jclepro.2020.125388.
- Cordella, M., F. Alfieri, and J. Sanfelix. 2021. “Reducing the Carbon Footprint of Ict Products Through Material Efficiency Strategies: A Life Cycle Analysis of Smartphones.” Journal of Industrial Ecology 25 (2): 448–464. https://doi.org/10.1111/jiec.13119.
- Deng, Q., M. Franke, E. S. Lejardi, R. M. Rial, and K.-D. Thoben 2021. Development of a Digital Thread Tool for Extending the Useful Life of Capital Items in Manufacturing Companies - An Example Applied for the Refurbishment Protocol. In 2021 26th ieee international conference on emerging technologies and factory automation (etfa), Västerås, Sweden, 1–8.
- Frey, S. D., D. J. Harrison, and E. H. Billett. 2006. “Ecological Footprint Analysis Applied to Mobile Phones.” Journal of Industrial Ecology 10 (1–2): 199–216. https://doi.org/10.1162/108819806775545330.
- Garcés-Ayerbe, C., P. Rivera-Torres, I. Suárez-Perales, and D. I. Leyva-de la Hiz. 2019. “Is it Possible to Change from a Linear to a Circular Economy? An Overview of Opportunities and Barriers for European Small and Medium-Sized Enterprise Companies.” International Journal of Environmental Research and Public Health 16 (5): 851. https://doi.org/10.3390/ijerph16050851.
- Geissdoerfer, M., P. Savaget, N. M. Bocken, and E. J. Hultink. 2017. “The Circular Economy – A New Sustainability Paradigm?” Journal of Cleaner Production 143:757–768. https://doi.org/10.1016/j.jclepro.2016.12.048.
- Geissdoerfer, M., D. Vladimirova, and S. Evans. 2018. “Sustainable Business Model Innovation: A Review.” Journal of Cleaner Production 198:401–416. https://doi.org/10.1016/j.jclepro.2018.06.240.
- Han, Y., T. Shevchenko, B. Yannou, M. Ranjbari, Z. Shams Esfandabadi, M. Saidani, and G. Bouillass, K. Bliumska-Danko, G. Li. 2023. “Exploring How Digital Technologies Enable a Circular Economy of Products.” Sustainability 15 (3): 2067. https://doi.org/10.3390/su15032067.
- Hanski, J., and P. Valkokari. 2020. “Impact of Circular Economy on Asset Management – Lifecycle Management Perspective.” In Engineering Assets and Public Infrastructures in the Age of Digitalization, edited by J. P. Liyanage, J. Amadi-Echendu, and J. Mathew, 156–163. Cham: Springer International Publishing.
- Hazelwood, D. A., and M. G. Pecht. 2021. “Life Extension of Electronic Products: A Case Study of Smartphones.” IEEE Access 9:144726–144739. https://doi.org/10.1109/ACCESS.2021.3121733.
- Hsu, C.-W., T.-C. Kuo, S.-H. Chen, and A. H. Hu. 2013. “Using Dematel to Develop a Carbon Management Model of Supplier Selection in Green Supply Chain Management.” Journal of Cleaner Production 56:164–172. https://doi.org/10.1016/j.jclepro.2011.09.012.
- Iphone 14 pro. product environmental report. (2022). Apple. Accessed July 6, 2023. https://www.apple.com/environment/pdf/products/iphone/iPhone/14/Pro/PER/Sept222.pdf.
- iphone user & sales statistics. (2023). Demandsage. Accessed July 6, 2023. https://www.demandsage.com/iphone-user-statistics/.
- Jabbour, C. J. C., A. Colasante, I. D’Adamo, P. Rosa, and C. Sassanelli. 2023. “Customer Attitudes Toward Circular Economy in the e-Waste Context: A Survey Assessing Sustainable Consumption Dynamics.” IEEE Engineering Management Review 51 (4): 28–45. https://doi.org/10.1109/EMR.2023.3303209.
- Kamble, S. S., A. Gunasekaran, and S. A. Gawankar. 2018. “Sustainable Industry 4.0 Framework: A Systematic Literature Review Identifying the Current Trends and Future Perspectives.” Process Safety and Environmental Protection 117:408–425. https://doi.org/10.1016/j.psep.2018.05.009.
- Kastanaki, E., and A. Giannis. 2022. “Forecasting Quantities of Critical Raw Materials in Obsolete Feature and Smart Phones in Greece: A Path to Circular Economy.” Journal of Environmental Management 307:114566. https://doi.org/10.1016/j.jenvman.2022.114566.
- Korhonen, J., A. Honkasalo, and J. Seppälä. 2018. “Circular Economy: The Concept and Its Limitations.” Ecological Economics 143:37–46. https://doi.org/10.1016/j.ecolecon.2017.06.041.
- Maldonado-Romo, J., and M. Aldape-Pérez. 2021. “Sustainable Circular Micro Index for Evaluating Virtual Substitution Using Machine Learning with the Path Planning Problem As a Case Study.” Sustainability 13 (23): 13436. https://doi.org/10.3390/su132313436.
- Maldonado-Romo, J., A. Maldonado-Romo, and M. Aldape-Pérez. 2022. “Path Generator with Unpaired Samples Employing Generative Adversarial Networks.” Sensors 22 (23): 9411. https://doi.org/10.3390/s22239411.
- Mangers, J., M. Amne Elahi, and P. Plapper. 2023. “Digital Twin of End-Of-Life Process-Chains for a Circular Economy Adapted Product Design – A Case Study on Pet Bottles.” Journal of Cleaner Production 382:135287. https://doi.org/10.1016/j.jclepro.2022.135287.
- Méndez, J. I., P. Ponce, A. Meier, T. Peffer, O. Mata, and A. Molina 2019. “S4 Product Design Framework: A Gamification Strategy Based on Type 1 and 2 Fuzzy Logic.” In International conference on smart multimedia, Marseille, France, 509–524.
- Miranda, J., R. Pérez-Rodríguez, V. Borja, P. K. Wright, and A. Molina. 2019. “Sensing, Smart and Sustainable Product Development (S3 Product) Reference Framework.” International Journal of Production Research 57 (14): 4391–4412. https://doi.org/10.1080/00207543.2017.1401237.
- Neves, S. A., and A. C. Marques. 2022. “Drivers and Barriers in the Transition from a Linear Economy to a Circular Economy.” Journal of Cleaner Production 341:130865. https://doi.org/10.1016/j.jclepro.2022.130865.
- O’Connell, S., and M. Stutz 2010. “Product Carbon Footprint (Pcf) Assessment of Dell Laptop - Results and Recommendations.” In Proceedings of the 2010 ieee international symposium on sustainable systems and technology, Virginia, United States, 1–6.
- OECD. (2007). Building Jobs and Prosperity in Developing Countries. Accessed June 6, 2023. https://www.oecd.org/derec/unitedkingdom/40700982.pdf.
- Perritaz, D., C. Salzmann, and D. Gillet 2009. “Quality of Experience for Adaptation in Augmented Reality”. In 2009 IEEE international conference on systems, man and cybernetics, Texas, United States, 888–893.
- Plociennik, C., M. Pourjafarian, A. Nazeri, W. Windholz, S. Knetsch, J. Rickert, and A. Weidenkaff (2022, April 4– 6). “Towards a Digital Lifecycle Passport for the Circular Economy”. In Procedia CIRP The 29th CIRP Conference on Life Cycle Engineering, 105, Leuven, Belgium, 122–127. https://www.sciencedirect.com/science/article/pii/S221282712200021X.
- Ponce, P., O. Mata, E. Perez, J. R. Lopez, A. Molina, and T. McDaniel. 2022. “S4 Features and Artificial Intelligence for Designing a Robot Against COVID-19—Robocov.” Future Internet 14 (1): 22. https://doi.org/10.3390/fi14010022.
- Ponce, P., A. Meier, J. Miranda, A. Molina, and T. Peffer. 2019. “The Next Generation of Social Products Based on Sensing, Smart and Sustainable (S3) Features: A Smart Thermostat As Case Study.” IFAC-Papersonline 52 (13): 2390–2395. https://doi.org/10.1016/j.ifacol.2019.11.564.
- Pont, A., A. Robles, and J. A. Gil. 2019. “E-Waste: Everything an Ict Scientist and Developer Should Know.” IEEE Access 7:169614–169635. https://doi.org/10.1109/ACCESS.2019.2955008.
- Repairing—not recycling—is the first step to tackling smartphone e-waste. (2021). World Economic Forum. Accessed June 6, 2023. https://www.weforum.org/agenda/2021/07/repair-not-recycle-tackle-ewaste-circular-economy-smartphones/.
- Rizos, V., J. Bryhn, M. Alessi, A. Campmas, and A. Zarra 2019. Identifying the Impact of the Circular Economy on the Fast-Moving Consumer Goods Industry: Opportunities and Challenges for Businesses, Workers and Consumers - Mobile Phones as an Example.
- Schwartz, J. T., and M. Sharir. 1983. “On the “Piano movers” Problem. Ii. General Techniques for Computing Topological Properties of Real Algebraic Manifolds.” Advances in Applied Mathematics 4 (3): 298–351. https://doi.org/10.1016/0196-8858(83)90014-3.
- Subramoniam, R., E. Sundin, S. Subramoniam, and D. Huisingh. 2021. “Riding the Digital Product Life Cycle Waves Towards a Circular Economy.” Sustainability 13 (16): 8960. https://doi.org/10.3390/su13168960.
- Tan, J., K. Ronkkö, and C. Gencel 2013. A Framework for Software Usability and User Experience Measurement in Mobile Industry. In 2013 joint conference of the 23rd international workshop on software measurement and the 8th international conference on software process and product measurement, Ankara, Turkey, 156–164.
- “This infographic shows the rise of mobile device subscriptions worldwide”. (2022). World Economic Forum. Accessed June 6, 2023. https://www.weforum.org/agenda/2022/10/mobile-device-subscription-rise-technology/.
- Toth-Peter, A., R. Torres de Oliveira, S. Mathews, L. Barner, and S. Figueira. 2023. “Industry 4.0 As an Enabler in Transitioning to Circular Business Models: A Systematic Literature Review.” Journal of Cleaner Production 393:136284. https://doi.org/10.1016/j.jclepro.2023.136284.
- Turner, C., O. Okorie, C. Emmanouilidis, and J. Oyekan. 2022. “Circular Production and Maintenance of Automotive Parts: An Internet of Things (Iot) Data Framework and Practice Review.” Computers in Industry 136:103593. https://doi.org/10.1016/j.compind.2021.103593.
- Tu, J.-C., X.-Y. Zhang, and S.-Y. Huang. 2018. “Key Factors of Sustainability for Smartphones Based on Taiwanese consumers’ Perceived Values.” Sustainability 10 (12): 4446. https://doi.org/10.3390/su10124446.
- Velenturf, A. P., and P. Purnell. 2021. “Principles for a sustainable circular economy.” Sustainable Production and Consumption 27:1437–1457. https://doi.org/10.1016/j.spc.2021.02.018.
- Wang, S., J. Wan, D. Zhang, D. Li, and C. Zhang. 2016. “Towards Smart Factory for Industry 4.0: A Self-Organized Multi-Agent System with Big Data Based Feedback and Coordination.” Computer Networks 101:158–168. https://doi.org/10.1016/j.comnet.2015.12.017.