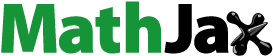
ABSTRACT
Excipients are added to biopharmaceutical formulations to enhance protein stability and enable the development of robust formulations with acceptable physicochemical properties, but the mechanism by which they confer stability is not fully understood. Here, we aimed to elucidate the mechanism through direct experimental evidence of the binding affinity of an excipient to a monoclonal antibody (mAb), using saturation transfer difference (STD) nuclear magnetic resonance (NMR) spectroscopic method. We ranked a series of excipients with respect to their dissociation constant (KD) and nonspecific binding constants (Ns). In parallel, molecular dynamic and site identification by ligand competitive saturation (SILCS)-Monte Carlo simulations were done to rank the excipient proximity to the proteins, thereby corroborating the ranking by STD NMR. Finally, the excipient ranking by NMR was correlated with mAb conformational and colloidal stability. Our approach can aid excipient selection in biologic formulations by providing insights into mAb–excipient affinities before conventional and time-consuming excipient screening studies are conducted.
Introduction
Therapeutic proteins, if not formulated well, are susceptible to physical (e.g., aggregation) and chemical degradation from small perturbations in their structures that can affect drug potency and lead to immune response in vivo.Citation1,Citation2 Overcoming stability issues and maintaining the stability of therapeutic proteins expected to be commercially viable have been substantial challenges for formulation development.Citation3,Citation4 The addition of excipients to the protein solution is the most commonly used approach to enhance protein stability and stabilize the drug product.Citation5 The most common composition of a biopharmaceutical formulation consists of a buffer (e.g., histidine, phosphate), stabilizer (e.g., carbohydrates, sugars, polyols, amino acids), surfactant (e.g., Polysorbate 20, Polysorbate 80) and antioxidant (e.g., ethylenediaminetetraacetic acid (EDTA); diethylenetriaminepentaacetic acid (DTPA)).Citation6–11 The stability of proteins varies widely, depending on the protein concentration, pH, buffer, buffer concentration, and the type of excipients. Excipient compatibility screening and physicochemical stress studies at accelerated conditions are conducted to finalize the excipient selection and composition.Citation12–15 Lyophilization (freeze-drying) of the protein formulation in selected excipients is also done to further enhance protein stability, especially for formulations with significant stability challenges.Citation16–18
Systematic screening is conducted to choose the right excipients for a given protein, but the mechanisms by which the excipients provide stability to the protein are not fully understood. Understanding why some excipients are better stabilizers of proteins can help with developing robust biopharmaceutical formulations in an accelerated manner. Moreover, having an analytical tool to quantify and rank the factors leading to their stability simplifies the excipient selection process, making it systematic and practical. However, few studies that provide a mechanistic understanding of the stabilizing effect of excipients to maintain protein stability have been published, and no direct evidence for protein–excipient interactions was identified.Citation19–22 Preferential exclusion by carbohydrates is one of the most prevalent mechanisms by which protein can be stabilized, adding beneficial effects on aggregation and the conformational stability of the protein.Citation19–21 Using isothermal titration calorimetry (ITC), Kim et al. identified proteins with a high binding affinity to carbohydrates, probably due to the hydrogen bond formation between the protein-binding sites with the carbohydrate molecules.Citation19 Souillac et al. used Fourier transform infra-red (FTIR) spectroscopic studies to show that, in the presence of carbohydrates, the secondary structure was replenished by hydrogen bonds formed between the polar groups on the surface of the protein and carbohydrate moieties during the lyophilization process.Citation22
The aim of our study is to provide direct experimental evidence of the binding affinity of the excipient to a monoclonal antibody (mAb), using a saturation transfer difference (STD) nuclear magnetic resonance (NMR) spectroscopic method. A series of excipients were ranked with respect to their dissociation constant (KD) and nonspecific binding constants (Ns),Citation23,Citation24 and, in parallel, molecular dynamic (MD) and Monte Carlo (MC) simulations were conducted to rank the excipient proximity to the proteins, thereby corroborating the STD NMR results and ranking. The study also provided insights into potential specific binding sites on proteins and the preferential conformation of the excipient to the protein, especially for sucrose and mannitol. Finally, the excipient ranking by NMR was correlated to mAb stability by comparing both thermal stability (Tm, the mid-point of thermal transition) measurements and colloidal stability (B22, the second osmotic virial coefficient) measurement. Tm represents where the folded and unfolded states of the protein are in equilibrium, and a higher value of Tm indicates a higher conformational stability of the protein, provided by the excipient.Citation25–27 B22 indicates the direction and magnitude of the interaction between two protein molecules in solution in presence of the chosen excipients as measured by static light scattering (SLS), with a positive value indicating net repulsive interactions, and a negative value indicating net attractive interactions.Citation26,Citation27 This approach has aided and accelerated the excipient selection in biologic formulations by providing insights into mAb–excipient affinities before conducting a conventional and time-consuming excipient screening study.
Results
Ranking of excipients by STD NMR
In this study, six excipients (sucrose, trehalose, mannitol, sorbitol, succinic acid, and glycine) were chosen to investigate and compare their binding affinities with a Bristol Myers Squibb mAb (BMSmAb, a type of IgG4). From the basic principles of saturation transfer, STD experiments rely on intermolecular transfer of magnetization from protein (mAb) to its binding ligand molecule (excipient) during a selective saturation time. Ligand (excipient) protons in closer proximity to the protein (mAb) receive higher degrees of saturation, which reflects in greater STD effects and can be used to map the mAb–excipient molecular interaction at atomic resolution and estimate their apparent binding affinities. It can be inferred that the intensity of an STD signal corrected by the excess of ligand (STD amplification factor, STDaf) gives direct information about the concentrations of mAb–excipient complexes in solution. STD NMR results from titration experiments (using a concentration series) can thus be used to derive mAb–excipient binding affinities.Citation24,Citation28 The derived mAb–excipient binding can be specific or nonspecific in nature. For specific interactions, the ligand resides in a specific binding pocket of the protein, and for nonspecific interactions the ligand is most likely distributed on the surface of the protein with no specific conformation.
It is to be noted that the mAb-excipient molecular interactions that are too strong could not be detected by STD. Also, when interactions between excipients and BMSmAb are not strong enough to be maintained in vivo, the addition of excipients is unlikely to affect the biological activity of BMSmAb. The pseudo-2D STD NMR quantitative experiments were used to get specific dissociation constants (KD) and nonspecific binding constants (Ns) of the chosen excipients of the BMSmAb. The STD NMR experiments were acquired at 283 K and 293 K for each BMSmAb–excipient series. The structures with numbering and 1D NMR spectral proton assignments of each excipient are shown in .
Figure 1. Structures and 1H 1D solution NMR spectra of sucrose, trehalose, mannitol, sorbitol, succinic acid, and glycine. For each excipient, the peaks under study are numbered in the structure and assigned in the spectra. 1H spectra in display were acquired at 283 K using bruker 700 MHz spectrometer.

KD and Ns were determined by monitoring the excipient STD NMR signals as a function of the excipient concentration. The concentration titrations of excipients ranged from 0 mM to 500 mM, which is a large excess compared to a typical excipient concentration in a formulation, which is ~250 mM. The STD amplification factor (),Citation23 which represents the experimentally observed STD effects, was calculated using EquationEq (1
(1)
(1) )
where () represents the ligand signal intensity in the STD NMR spectrum,
is the ligand peak intensity in an off-resonance NMR spectrum (reference), and
is the ligand peak intensity in an on-resonance NMR spectrum;
is the ligand excess relative to a fixed and constant protein concentration.
All the STD NMR experiments were collected using a recycle time of 12 s (longer than 5 × ) and the STDaf was corrected for different T1 relaxation times as proposed by Kemper et al.Citation24 The
s belonging to the same proton were combined first. The experimental buildup curves of
of each proton with respect to ligand concentration were first fitted with the modified Michaelis–Menten equation, which assumes single-site binding and includes a nonspecific binding term in EquationEq (2
(2)
(2) )
where is the relaxation time measured from NMR experiments,
is the maximum
,
is the total concentration of free ligand.
If the buildup curves failed to fit EquationEq (2(2)
(2) ), they were fitted with the EquationEq (3
(3)
(3) ) for nonspecific binding.
A 5% permissible error threshold was chosen for 1H STD NMR and T1 measurement experiments, given the uncertainty of peak position and imperfection of baseline correction. For the fitting, the concentration was cut off at 250 mM since a typical excipient concentration in a biologic formulation is at or below 250 mM due to high viscosity effects at higher concentrations. Moreover, excipient–excipient interactions could dominate excipient–protein interactions at higher concentrations. The viscosity measurement results are shown in Supplementary Table S2.
Among all the excipients, only the experimental data for sucrose is well fitted to EquationEq (2(2)
(2) ) at both 283 K and 293 K. The fitted plots for all the protons at both temperatures are shown in . The values of KD and Ns are shown in . KD values of proton H5/H9 at 283 K and proton H5/H9/H17/H19 at 293 K were rejected by P test. Ns values of proton H7/H13 at both 283 K and 293 K were rejected by Q test. The average KD values are 67.83 mM and 88.63 mM at 283 K and 293 K, respectively. As for trehalose, mannitol, sorbitol, succinic acid and glycine, the buildup curves are fitted to EquationEq (3
(3)
(3) ) and the fitted plots are shown in . The values of Ns are shown in . The proton H4/H12/H17/H19 at 283 K and mannitol H9 at 293 K were rejected by Q test. All outliers were excluded for further investigation.
Figure 2. Experimental data points and fitting plots for sucrose at 283 K (black) and 293 K (blue). Site-specific binding curves fitted by use of EquationEq (2(2)
(2) ) are shown in dashed lines. Fitting curves with orange marks are outliers based on P test.

Figure 3. Experimental data points and fitting plots for a) trehalose, b) sorbitol, c) mannitol, d) succinic acid and e) glycine at 283 K (black) and 293 K (blue). No site-specific binding is observed, and the nonspecific binding curves fitted by use of EquationEq (3(3)
(3) ) are shown in solid lines. Fitting curves with orange marks are outliers (Trehalose H10 was rejected because of peak overlapping).

Table 1. T1 relaxation times, site-specific dissociation constants (KD), nonspecific binding constants (Ns) and statistics (P test) for sucrose at 283 K and 293 K.
Table 2. T1 relaxation times, nonspecific fitting constants (Ns) and statistics (P test) for trehalose, mannitol, sorbitol, succinic acid, and glycine at 283 K and 293 K.
The nonspecific binding constant (Ns) for all six excipients was determined and ranked in . At 283 K, sucrose has the largest binding constant (0.065 mM−1 in average), trehalose ranks second (0.034 mM−1 in average), and the other four excipients have a similar binding constant (range of 0.010–0.020 mM−1 in average). The ranking is the same at 293 K. We observed that the STD effects are stronger at 283 K than at 293 K for all the excipient protons.
Figure 4. Ranking of excipients of nonspecific binding constant by STDNMR (a), thermal stability measurement (b), B22 measurement (c) and excipient count by MD (within 2 Å) (d) of MD simulation with 3D illustration (e). (a) the nonspecific binding constant of each proton (outliers excluded) at 283 K (blue) and 293 K (yellow) were ranked with error bar depicted. Ranking: Sucrose > Trehalose > Mannitol ≈ Sorbitol ≈ Succinic acid ≈ Glycine. (b) the thermal stability measurement (Tm) of each excipient at 293 K were ranked with error bar depicted. Control: 20 mM His buffer with BMSmAb (bar 1). Sucrose, trehalose, mannitol, and sorbitol increases the thermal stability of BMSmAb, whereas succinic acid and glycine decrease it (bar 2–7). Ranking: Sucrose ≈ Trehalose > Mannitol ≈ Sorbitol > Succinic acid > Glycine. (c) the colloidal stability measurement (B22) of each excipient at 293 K were ranked with error bar depicted. Control: 20 mM His buffer with BMSmAb (bar 1). Ranking: Sucrose ≈ Trehalose > Mannitol ≈ Sorbitol > Glycine > Succinic acid. (d) the block average (using 8 ns blocks) for the last 80 ns of the simulation of each excipient with an atom within 2 Å of any atom on the BMSfAb was counted (normalized by SASA) and ranked with error bar depicted. Ranking: Sucrose > Trehalose > Mannitol > Sorbitol > Glycine ≈ Succinic acid. (e) the number of binding sites as a function of LGFE value for different excipients. Ranking: Trehalose ≈ Sucrose > Sorbitol ≈ Mannitol > Succinic acid > Glycine.

Ranking of excipients by Tm and B22 measurement
The excipient ranking by NMR was validated by correlating the NMR ranking to both the thermal stability (Tm, ) and colloidal stability (B22, ) values with the chosen excipients. A higher value of Tm indicates a higher thermal stability of the protein, provided by the excipient. For a single excipient, higher concentration gives higher Tm values (Supplementary Figure S1). For the series of excipients under study, trehalose and sucrose have the highest Tm values and increased the thermal stability of the BMSmAb, followed by mannitol and sorbitol, whereas glycine and succinic acid decreased the thermal stability of the mAb () in comparison to mAb with no excipients, which was used as the control. A higher value of B22 indicates a decrease in net attractive interactions between proteins, suggesting that the excipient is either strengthening repulsive protein–protein interactions (PPI) or weakening attractive PPI. Previous studies have shown that proteins with more repulsive interactions (or high B22 values) have higher colloidal stability. For the series of excipients under study, sucrose and trehalose have the highest B22 values, followed by sorbitol and mannitol. The B22 values of glycine and succinic acid are similar to the control mAb in buffer ().
Ranking of excipients by MD and SILCS-MC methods
The block average (using 8 ns blocks) for the last 80 ns of the simulation of any excipient with an atom within 2 Å of any atom on the BMSmAb’s antigen-binding fragment (BMSfAb) was counted and ranked for the chosen excipients and weighted by the excipient surface area relative to the highest surface area excipient, trehalose, which accounts for the size difference between different excipients. The plot is shown in and the 3D illustrations of excipient clusters with BMSfAb are shown in . The ranking of excipients by MD is in good agreement with the STD-NMR ranking where sucrose has the most interaction with the BMSmAb, followed by trehalose, mannitol, and sorbitol, whereas glycine and succinic acid show the least interaction.
Figure 5. 3D maps of the screened excipient clusters where dense clusters (>85% occupancy) are shown as space filling models and less dense cluster (>20% occupancy) are shown as ball and stick models along a blue-green-red color gradient by occupancy. These 3D illustrations are generated by VMD.

Similar observations were noted based on the ligand grid free energy (LGFE) values, as depicted in . As LGFE indicates the probability of finding the excipient atoms interacting with the protein surface, a lower ΔG value (or more negative value) would indicate a relatively stronger binding affinity of the excipient on the protein surface.Citation29 shows the number of binding sites as a function of LGFE value for different excipients. A larger number of binding sites (~200) are observed for glycine at lower LGFE values (−1 kcal/mol), but the binding clusters decrease rapidly to around 75 for LGFE = −2 kcal/mol and eventually fall off at −4 kcal/mol. On the other hand, for sucrose, the number of binding sites is half that of glycine at −1 kcal/mol, with a more gradual fall in the binding sites up to −10 kcal/mol. A lower number of binding sites for sucrose are more likely due to its bulkier structure than glycine at LGFE = −1 kcal/mol.
Similar numbers of binding sites are observed for sucrose and trehalose and mannitol and sorbitol, which can be attributed to their similar molecular structures. SILCSBio LGFE results show a binding free energy cutoff for the clustering algorithm below which a cluster is not considered because these clusters are too transient to be captured by this analysis. Thus, only the lower energy-binding sites are captured by the MD clustering algorithm, with the lowest energy-binding sites being the densest clusters. This is the reason that, despite having the highest number of binding sites at −1 kcal/mol (~200) in SILCSBio, glycine does not show many clusters in the MD analysis. The dense clusters in MD, which are more highly occupied throughout the simulation, most closely agree with the strongest binding sites from LGFE of an excipient from SILCSBio. The binding sites, however, are likely not identical due to differences between the two methods (MC vs MD). The observed ranking using MD and SILCS-MC based on the LGFE values as binding sites approach zero for excipients with stronger binding to lower binding onto the protein surface is as follows: Trehalose (−10 kcal/mol) ≈ Sucrose (−10 kcal/mol) > Sorbitol (−7 kcal/mol) > Mannitol (−6 kcal/mol) > Succinic acid (−5 kcal/mol) > Glycine (−4 kcal/mol) (). Our results indicate that SILCSBio is a suitable tool for quickly screening excipients for rank ordering by the number of binding sites and has potential in predicting the presence of some stable clusters using sites with the lower energy. Taken together, SILCSBio is proved to be a quick tool to pre-screen excipient steps before conducting long MD trajectories and provide excipient recommendations for formulations.
Binding site and conformation of excipient molecules to BMSmAb
STD-NMR experiments have been used to characterize the interaction between ligands and proteins because the stronger STD effects reflect the closer contact of the ligand to the protein, enabling binding epitope mapping.Citation23,Citation30,Citation31 In our study, the STD amplification factor was corrected for differing T1 relaxation times; therefore, the difference in the relative STD effects for each proton should reflect the relative distance of that proton to the protein-binding site. The potential binding site and conformation of sucrose and mannitol were studied because their protons could be easily distinguished and assigned without much overlap of the peaks in 1D 1H NMR experiments, which give more accurate STD effect values. The STD effect curves for the assigned protons at 283 K and 293 K are shown in . For an STD effect comparison, the proton with the most intense STD effect is set to 100% and all other protons are calculated accordingly. The protons with relatively large STD effects are circled in the structure of the excipients from red to yellow in . Single carbons with multiple protons were separated by parentheses (1) and (2). The proton with shorter distance dominates the STD effect in the same carbon. For sucrose at 293 K, H7 should be the most involved proton in binding and the closest to BMSmAb, followed by H13(1), and then H12 and H10. Other protons are more distant from BMSmAb and more solvent exposed. For mannitol at 293K, either H3 or H11 is the most involved proton at the BMSmAb binding site, followed by H2.
Figure 6. Binding site of sucrose (left) and mannitol (right) with BMSmAb characterized by STD effect analysis and MD simulation. First row: structure of each excipient with the affected proton highlighted in circle (ranking from red for the strongest effect to yellow). Second (283 K) and third (293 K) row: STD effect plot of different proton of each excipient at different concentration. Different protons show different amplitude of STD effect. Fourth row: the 3D illustration of most probable binding position of each excipient with BMSmAb calculated by MD simulation. The closest two distances of each proton which are less than 3 Å are shown. These 3D illustrations are generated by pymol.

The results from MD simulation greatly support our statements above. We noticed that the STD effect is very sensitive and drops from 100% to 30% within 1 Å distance difference. For sucrose, five potential binding sites with BMSmAb were found by MD simulation based on the occupation time (β value). Interestingly, sucrose position 3 best matches the STD-NMR results that H7 is closest to BMSmAb, which is 2.0 Å, followed by H13(1) with 2.1 Å, H12 with 2.3 Å, and H10 with 2.6Å. This indicates that the STD effect is mostly affected by this position (dominant position). As for mannitol, only one potential binding site is found. The STD effect of mannitol is much smaller than sucrose and the closest distance of them with BMSmAb is 2.6 Å. The 3D illustrations of sucrose position 3 and mannitol are shown in . The site map, density map and interaction profile derived from MD simulation are depicted in Supplementary Figure S2. All the information from sucrose positions 1, 2, 4 and 5 are also depicted in Supplementary Figure S3. As a summary, the shortest two distances of all the models are listed in Supplementary Table S3, with the ranking in superscript.
Discussion
STD-NMR results show that, among the six chosen excipients, only sucrose has specific binding. As for trehalose, mannitol, sorbitol, succinic acid and glycine, their binding is nonspecific, which means they do not have a specific binding pocket and distribute on the surface without a fixed orientation. Through the STD NMR experiments, the excipients were ranked according to the nonspecific binding constant (Ns), and direct evidence of the stabilizer excipient interactions to the mAb was established. This corroborated studies in the literature where Kim et al.Citation19 showed that the proteins investigated in their study had high binding affinity to the carbohydrates due to hydrogen bond formation between protein binding sites with the carbohydrate molecules and where FTIR studies by Souillac et al.Citation22 showed that, in the presence of carbohydrate, the secondary protein structure was preserved by hydrogen bonds formed between the polar groups on the surface of the protein and carbohydrate moieties. Literature reports have hypothesized, however, that mAbs are stabilized by excipients via a preferential exclusion mechanism, whereby excipient molecules rearrange the solvent molecules surrounding the mAbs.Citation19–21 Although increased exothermic enthalpy while mixing excipients and proteins was seen by Kim et al., no specific interactions could be detected between proteins and excipients.Citation19 In our work, NMR experiments detected protein–excipient interactions at a molecular level and showed specific interactions of sucrose molecules with BMSmAb.
Although succinic acid and glycine show similar STD effects compared to mannitol and sorbitol, the Tm measurements indicate that succinic acid and glycine destabilize the protein, whereas mannitol and sorbitol stabilize the protein. Our hypothesis for the decreased thermal stability caused by glycine and succinic acid is that mAb destabilization is probably occurring due to the chemical nature of the molecules, which form zwitterionic and charged moieties that are not found in the carbohydrate molecules used in the study. The trending and the correlation between the degree of binding by STD NMR to Tm and B22 (both stability indicators) for the excipients under study suggests that stronger excipient binding is likely causing improved protein stability (thermal and colloidal). Moreover, closer proximity of the excipient to the mAb may also provide better conformational stability to the mAb, as in the case of nonspecific binding interactions, but the exact mechanism for this increase in stability needs to be elucidated. Interestingly, Svilenov et al. Citation32 related the protein–surfactant interaction with protein stability, taking a similar approach by correlating STD NMR binding affinities to circular dichroism (CD), nanodifferential scanning fluorimetry (nanoDSF) and long-term storage stability data on protein aggregation. Even though the surfactant ligands did not affect the tertiary structure and the thermal stability of the protein, as evident from CD and nanoDSF measurements of the protein with or without surfactants, the long-term stability data showed increased protein stability with stronger protein–ligand binding affinities. On the other hand, Zalar et at.Citation33 showed that the overall protein–excipient binding can be a poor criterion for choosing excipients enhancing formulation stability. However, the advantage of techniques like NMR is that the molecular-level understanding of protein–ligand binding provided can be used to better understand the mechanism by which excipients bind to proteins. And such techniques can be used for troubleshooting and de-risking when unusual or contradictory results are obtained by other stability-indicating biophysical methods.
Overall, in this study, we found sucrose to be the best stabilizer of the BMSmAb, followed by trehalose, mannitol, and sorbitol. The approach of ranking the excipients using STD-NMR experiments and computational MD/MC simulations aided and accelerated the excipient selection in biologic formulations by providing direct evidence of mAb–excipient affinities before a conventional and time-consuming excipient screening study was conducted. By validating this approach of correlating STD-NMR experiments and MD/MC simulations to Tm and B22 values, and therefore to the protein stability, future mAb development can perhaps directly rely on using the ranking by MD/MC simulations for excipient selection in protein formulations. Moreover, STD NMR can be applied to help determine the dominant interacting structural motif of excipients with mAbs and rule out the less possible positions, which will further characterize the excipient interaction with mAbs. This level of molecular-level information on protein–ligand binding obtained by STD NMR, MD, and MC also contributes to a better understanding of the mechanism by which stabilizer excipients bind to proteins and can be used for troubleshooting and de-risking when unusual results are obtained by other stability-indicating biophysical methods. We are extending this work to other BMS mAbs for further validation of this approach.
Materials and methods
Materials
Deuterium oxide (D2O, CAS #: 7789-20-0), sucrose (CAS #: 57-50-1), D-mannitol (CAS #: 69-65-8), sorbitol (CAS #: 50-70-4), D-(+)-trehalose dihydrate (CAS #: 6138-23-4), glycine (CAS #: 56-40-6), succinic acid (CAS #: 110-15-6), sodium succinate dibasic hexahydrate (CAS #: 6106-21-4) and sodium trimethylsilyl propionate (TSP, CAS #: 37013-20-0) were purchased from Sigma-Aldrich. L-Histidine (CAS #: 71-00-1) and L-Histidine monohydrochloride monohydrate (CAS #: 5934-29-2) were purchased from JT Baker.
STD NMR method
All stock solutions and NMR samples were prepared in 99.9% D2O buffer containing 20 mM histidine at pH 6.0. The excipient stock solution concentration was 750 mM. Concentrations of excipient stock solutions were calibrated by stock solution containing 80 mM TSP. For each excipient series, 16 BMSmAb–excipient samples were prepared with various concentrations of excipients (ranging from 0 mM to 500 mM) and a constant concentration of BMSmAb (final concentration 0.3 mM) in final 180 µL histidine D2O buffer and transferred into 3 mm NMR tube for STD NMR experiments and T1 measurement experiments. The concentration of each excipient is shown in Supplementary Table S1.
All pseudo-2D STD NMR experiments and T1 measurement experiments of BMSmAb-excipient samples were acquired at 283 K and 293 K on a Bruker NEO 700 MHz (16.4 T) spectrometer equipped with a 3 mm probe head. The Larmor frequency was 700.1 MHz for 1H. 1H chemical shift was referenced with respect to the internal standard TSP at 0.0 ppm. For STD NMR experiments, typical 90° pulse length was 8.71 µs for 1H, and the recycle delay was 12 s and an STD-pulse train saturation period of 10 s. A total of 64 scans were recorded. Selective on- and off-resonance frequencies were set at 0.4 and −10 ppm, respectively. The saturation pulse trains were composed of selective Gaussian pulses of 50 ms duration and 200 Hz amplitude. All STD NMR spectra were processed and analyzed in Bruker TopSpin (version 4.1.3) and ACD Spectrus software. Proton T1-measurements were performed in samples containing ~0.18 mM of excipients using the inversion recovery method.
Molecular dynamics methodology
System set-up
A homology model of the fAb region of the BMSmAb was generated using Molecular Operating Environment (MOE) and prepared at pH 6.0 inside a water box of 122 × 122 × 122 Å3 using the AMBER parameters.Citation34,Citation35 Water was then substituted out for a histidine buffer and counter ions to generate a starting point for each of the unique excipients. Individual systems for each excipient (glycine, trehalose, sucrose, mannitol, succinic acid (−1) and sorbitol) were generated using MOE by substituting water to place 200 excipient molecules randomly in the box. Each of the excipient systems was then prepared for full MD that were run on a p3.2×large AWS, with 1 nvidia tesla v100 GPU using NAMD (Nanoscale Molecular Dynamics).Citation34,Citation36 MD was performed using NAMD starting with minimization for 1 ns, followed by 10 ns of equilibration at 300 K and 100 kPa, and 250 ns of production run at 300 K and 100 kPa. Where relevant, the Langevin thermostat with a damping coefficient of 5 ps−1 and barostat with a period of 200 fs and a decay of 100 fs were used with particle-mesh Ewald electrostatics with a 1 Å mesh spacing, a 10 Å non-bonded cutoff, and a 2 fs time step.Citation36
Post-processing
All the trajectories of each simulation were loaded into Visual Molecular Dynamics (VMD), and a new unified trajectory was formed for each excipient with the coordinates wrapped around the periodic boundary conditions of the simulation box.Citation37 A.tcl script that was run through VMD to count the nearby excipients at each time step was executed measuring the excipients within 2 Å around the BMSfAb. The last 80 ns of each of the time traces were then block averaged with 8 ns blocks for each excipient to compare how well each excipient associates with the BMSfAb and weighted by the excipient surface area normalized by the highest surface area excipient, trehalose, to account for the size difference between excipients. While this showed a clear trend in the preference for excipients, it is unclear from these results how consistent these associations are, so a clustering algorithm was developed to measure the frequency of each association.
To start the clustering algorithm, a new trajectory was made that moved the fAb to the center of the simulation box, oriented the fAb uniformly across all frames, and stripped away all water molecules. The coordinates for each nearby excipient at every time step were then saved to and exported to another script that clusters them together. All the clusters for each excipient were then weighted against the densest cluster.
SILCS-MC methodology
An alternative approach was also adapted to rank order the excipients using a novel in-silico approach – SILCS-Biologics. SILCS enables modeling of interactions of different excipients in a formulation at an atomistic level. SILCS simulations with GROMACSCitation38 were performed using the SILCSBio package (provided by SILCSBio, LLC) where the homology model of the BMSmAb generated using MOE at pH 6.0 was used. A combination of p3.2×large AWS with 1 nvidia tesla v100 GPU and m5.16×large with x86_64 CPU architecture was used to run MC simulations. The protocol of SILCS simulations is described in Somani et al.Citation39 Briefly, the BMSmAb was divided into its fAb and fc domains and 10 independent simulation systems involving BMSmAb domain (Fab or fc), water, and eight probe molecules that represent diverse functional groups that are common in small organic molecule to protein interaction, such as hydrophobic or nonpolar (benzene, propane), hydrogen bond acceptor and donor (methanol, formamide, acetaldehyde, imidazole), positive (methylammonium) and negative (acetate) charge interactions, were prepared. The choice of probe molecules and the validation of the SILCS approach in replicating protein–ligand interactions have been investigated in prior works.Citation40–42 Each system was minimized and equilibrated using 1 ns of MD simulation followed by 100 cycles of grand canonical Monte Carlo (GCMC)/MD simulation.Citation39 During each cycle, 200,000 steps of GCMC simulation were performed that drive the sampling of water and probe molecules, followed by 1 ns of MD simulation of the entire system, resulting into an aggregated simulation time of 1 µs (10 × 100 ns). The protein, probe and water molecules were described using the CHARMM36m protein force field,Citation43,Citation44 the CHARMM general force field (CGenFF),Citation45 and the TIP3P water model modified for the CHARMM force field.Citation46
The GCMC/MD-based approach resulted in the generation of fragment maps (FragMaps) for fAb and fc domains by binning the selected probe atoms into the voxels of a 1Å spaced grid spanning the simulation system. The voxel occupancy was used to measure a grid-based free energy (GFE, ΔG) using the equation where
is the universal gas constant,
is the system temperature,
is the observed voxel occupancy of the probe at grid point
and
is the expected voxel occupancy of the probe alone in the bulk solution. Therefore, GFE is a measure of the free energy change of moving an atom from the bulk state to the grid point
. For instance, if the GFE of a voxel near protein is −1.5 kcal/mol, then the probability of probe atoms is about 12 times more likely to be found in the voxels than in the voxels that are far away from the protein (“bulk”) at room temperature.
Individual systems for each excipient were generated using the excipient docking and screening algorithm (SILCS-MC) to rank order excipients, which involves MC sampling of the ligand (excipient) in translational, rotational, and torsional space.Citation41 The main purpose is to sample ligand binding affinity in the field of FragMaps along with the SILCS exclusion map. The exclusion map prevents the sampling of the ligand in the interior region of the protein where no water or probe molecules visited during the SILCS GCMC/MD step. The energy associated with ligand conformation is based on CGenFF intramolecular forces along with the LGFE score that is an approximate representation of the minimum free energy binding conformation, defined as the sum of atomic GFEs.Citation41,Citation42 LGFEs have been shown to correlate well with the binding affinities of small, drug-like molecules to a range of proteins.Citation42
Viscosity measurements
All stock solutions and samples were prepared in a 20 mM histidine buffer with pH 6.0. For each excipient series, four BMSmAb–excipient samples were prepared with various concentrations of excipient and constant concentration of BMSmAb (final concentration 0.3 mM) in final 1 mL histidine buffer. For sucrose, trehalose, mannitol, and sorbitol, the four concentrations were 4 mM, 80 mM, 250 mM, and 500 mM. For succinic acid, the four concentrations were 4 mM, 80 mM, 250 mM, and 470 mM. For glycine, the four concentrations were 4 mM, 80 mM, 250 mM, and 445 mM.
The viscosity of the BMSmAb solutions was determined using the m-VROC viscometer (RheoSense Inc.). The samples were filtered with 0.1 µm syringe filter and then measured at least in duplicate with A05 chip at a shear rate of 3760 s−1 for approximately 5 s. The system was washed with water between each BMSmAb–excipient set. All the measurements were performed at 20°C.
Differential scanning fluorimetry
All stock solutions and samples were prepared in a 20 mM Histidine buffer with pH 6.0. For sucrose, three BMSmAb–excipient samples were prepared with various concentrations of excipient (4 mM, 80 mM and 250 mM) and constant concentration of BMSmAb (final concentration 0.3 mM) in the final 0.5 mL histidine buffer. For other excipients, only 250 mM excipient and 0.3 mM BMSmAb in the final 0.5 mL histidine buffer were prepared. The BMSmAb sample without any additional excipient was used as the reference control.
DSF was used to monitor the stability of BMSmAb solutions in the presence of various excipients by measuring the thermal unfolding by detecting changes in BMSmAb intrinsic fluorescence during a thermal ramp. The measurements were performed using Prometheus NT.48 (NanoTemper). Twenty microliters of each sample were transferred in duplicate to the wells of a 384-well plate and loaded in a standard glass capillary chip simultaneously. Each sample was filtered with 0.1 µm syringe filter prior to the measurements. The instrument excitation power was adjusted to 10% to ensure fluorescence was in the optimal detection range. Fluorescence intensity at 350 nm and 330 nm was collected over a temperature range of 15 to 100°C with temperature slope of 1°C/min. The data analysis was performed on the PR Stability Analysis Software (NanoTemper) at which the unfolding temperatures, Tm, corresponding to the peaks of the first derivative of the fluorescence ratio 350/330 nm were automatically determined.
Static light scattering
All stock solutions and samples were prepared in 20 mM histidine buffer with pH 6.0. For each excipient series, samples were prepared with 250 mM of each excipient and 0.3 mM BMSmAb in final 5 mL histidine buffer. Matching buffers for each excipient were prepared without BMSmAb in the final 10 mL histidine buffer. NaCl stock solution was also prepared in water at a final concentration of 1 M. SLS measurements were performed to determine the second osmotic virial coefficient, B22, which is related to protein–protein interactions in the dilute concentration regime and provides direct information on colloidal stability properties of the protein solution. The experiments were conducted using Wyatt DynaPro Plate Reader III (Wyatt Technology) at 25°C.
Due to the sensitivity of the scattering measurements, all the stock samples and buffers were filtered through a 0.1 µm syringe filter to avoid interference from aggregates or dust particles. For each formulation condition tested, a concentration series of 2 to 10 mg/ml were prepared by diluting the stock solution with matching buffer. The concentration of each sample was confirmed by absorbance 280 nm measurements. Scattering measurements were also carried out for BMSmAb at the same formulation conditions, but in the presence of additional 100 mM NaCl, achieved by spiking 1 M NaCl solution in the sample and matching buffer. Sample volumes of 60 µl were loaded in a calibrated 384-well SensoPlate (Greiner Bio-One Inc). Data was collected with a 5 s acquisition time, 10 acquisitions per measurement (3 in total) at 20% laser power (Lp) and either 0% or 50% attenuation (At) to maintain the intensity counts in the optimal range. Data acquisition and data analysis were performed with Dynamics software (Wyatt Technology). Under diluted protein conditions, B22 is calculated from a linear fit of the equation below:
where K is the optical constant determined from the plate calibration; c is the protein concentration; is the refractive index increment of the protein solution;
is the excess Rayleigh ratio obtained experimentally and Mw is the apparent molecular weight of the protein in solution. Optilab (Wyatt Technology), an online refractive index detector, was used to measure the absolute refractive index of all excipient formulations (
). The measured absolute refractive index, specific density of protein (
~0.73 g/mL), refractive index of water (
~1.330) and refractive index increment of the protein in water (
= 0.185) to calculate the new
using the equation belowCitation47,Citation48:
Since is effectively independent of salt concentration, the same value has been used to calculate B22 for the formulations containing NaCl.
Abbreviations
At | = | attenuation |
B22 | = | second osmotic virial coefficient |
BMSmAb | = | Bristol Myers Squibb mAb |
CD | = | circular dichroism |
CGenFF | = | CHARMM general force field |
D2O | = | Deuterium oxide |
DTPA | = | diethylenetriaminepentaacetic acid |
EDTA | = | Ethylenediaminetetraacetic acid |
FTIR | = | Fourier transform Infra-Red |
GCMC | = | grand canonical Monte Carlo |
GFE | = | grid-based free energy |
ITC | = | isothermal titration calorimetry |
KD | = | dissociation constant |
LGFE | = | ligand grid free energy |
Lp | = | laser power |
mAb | = | monoclonal antibody |
MC | = | Monte Carlo |
MD | = | Molecular dynamic |
MOE | = | Molecular Operating Environment |
NAMD | = | Nanoscale Molecular Dynamics |
nanoDSF | = | nano differential scanning fluorimetry |
NMR | = | nuclear magnetic resonance |
Ns | = | nonspecific binding constant |
PPI | = | protein-protein interactions |
SILCS | = | Site Identification by Ligand Competitive Saturation |
SLS | = | static light scattering |
STD | = | saturation transfer difference |
STDaf | = | STD amplification factor |
Tm | = | mid-point of thermal transition |
TSP | = | sodium trimethylsilyl propionate |
VMD | = | Visual Molecular Dynamics |
Supplemental Material
Download Zip (3.2 MB)Acknowledgments
The authors would like to thank Janet Cortes, Tracy Gaebele and Arvind Mathur for this collaboration opportunity, and Dilbir Bindra for valuable discussions on expanding this work.
Disclosure statement
No potential conflict of interest was reported by the authors.
Supplementary material
Supplemental data for this article can be accessed online at https://doi.org/10.1080/19420862.2023.2212416.
Additional information
Funding
References
- Rosenberg AS. Effects of protein aggregates: an immunologic perspective. Aaps J. 2006;8(3):E501–13. doi:10.1208/aapsj080359.
- Moussa EM, Panchal JP, Moorthy BS, Blum JS, Joubert MK, Narhi LO, Topp EM. Immunogenicity of therapeutic protein aggregates. J Pharm Sci. 2016;105(2):417–30. doi:10.1016/j.xphs.2015.11.002.
- Parkins DA, Lashmar UT. The formulation of biopharmaceutical products. Pharm Sci Technolo Today. 2000;3(4):129–37. doi:10.1016/S1461-5347(00)00248-0.
- Akers MJ. Excipient-drug interactions in parenteral formulations. J Pharm Sci. 2002;91(11):2283–300. doi:10.1002/jps.10154.
- Kamerzell TJ, Esfandiary R, Joshi SB, Middaugh CR, Volkin DB. Protein–excipient interactions: mechanisms and biophysical characterization applied to protein formulation development. Adv Drug Deliv Rev. 2011;63(13):1118–59. doi:10.1016/j.addr.2011.07.006.
- Stoll VS, Blanchard JS. [4] buffers: principles and practice. In Deutscher MP, ed. Methods Enzymol: Academic Press; 1990. p. pp. 24–38.
- Bhatnagar BS, Bogner RH, Pikal MJ. Protein stability during freezing: separation of stresses and mechanisms of protein stabilization. Pharm Dev Technol. 2007;12(5):505–23. doi:10.1080/10837450701481157.
- Chang LL, Pikal MJ. Mechanisms of protein stabilization in the solid state. J Pharm Sci. 2009;98(9):2886–908. doi:10.1002/jps.21825.
- Kumar R. Role of naturally occurring osmolytes in protein folding and stability. Arch Biochem Biophys. 2009;491(1–2):1–6. doi:10.1016/j.abb.2009.09.007.
- Arakawa T, Tsumoto K, Kita Y, Chang B, Ejima D. Biotechnology applications of amino acids in protein purification and formulations. Amino Acids. 2007;33(4):587–605. doi:10.1007/s00726-007-0506-3.
- Kerwin B. Polysorbates 20 and 80 used in the formulation of protein biotherapeutics: structure and degradation pathways. J Pharm Sci. 2008;97(8):2924–35. doi:10.1002/jps.21190.
- Dave VS, Haware RV, Sangave NA, Sayles M, Popielarczyk M. Drug-excipient compatibility studies in formulation development: current trends and techniques. AAPS FDD Sec Newslett. 2015;9–15.
- Patel PA, Ahir K, Patel VB, Manani L, Patel C. Drug-excipient compatibility studies: first step for dosage form development. J Pharm Innov. 2015;4:14–20.
- Bee JS, Chiu D, Sawicki S, Stevenson JL, Chatterjee K, Freund E, Carpenter JF, Randolph TW. Monoclonal antibody interactions with micro- and nanoparticles: adsorption, aggregation, and accelerated stress studies. J Pharm Sci. 2009;98(9):3218–38. doi:10.1002/jps.21768.
- Kopp MRG, Perez AMW, Zucca MV, Palmiero UC, Friedrichsen B, Lorenzen N, Arosio P. An accelerated surface-mediated stress assay of antibody instability for developability studies. MAbs. 2020;12(1):12. doi:10.1080/19420862.2020.1815995.
- Roughton BC, Iyer LK, Bertelsen E, Topp EM, Camarda KV. Protein aggregation and lyophilization: protein structural descriptors as predictors of aggregation propensity. Comput Chem Eng. 2013;58:369–77. doi:10.1016/j.compchemeng.2013.07.008.
- Wang W. Lyophilization and development of solid protein pharmaceuticals. Int J Pharm. 2000;203(1–2):1–60. doi:10.1016/S0378-5173(00)00423-3.
- Butreddy A, Janga KY, Ajjarapu S, Sarabu S, Dudhipala N. Instability of therapeutic proteins - an overview of stresses, stabilization mechanisms and analytical techniques involved in lyophilized proteins. Int J Biol Macromol. 2021;167:309–25. doi:10.1016/j.ijbiomac.2020.11.188.
- Kim NA, Thapa R, Jeong SH. Preferential exclusion mechanism by carbohydrates on protein stabilization using thermodynamic evaluation. Int J Biol Macromol. 2018;109:311–22. doi:10.1016/j.ijbiomac.2017.12.089.
- Arakawa T, Prestrelski SJ, Kenney WC, Carpenter JF. Factors affecting short-term and long-term stabilities of proteins. Adv Drug Deliv Rev. 2001;46(1–3):307–26. doi:10.1016/s0169-409x(00)00144-7.
- Avanti C, Saluja V, van Streun EL, Frijlink HW, Hinrichs WL, Permyakov EA. Stability of lysozyme in aqueous extremolyte solutions during heat shock and accelerated thermal conditions. PLos One. 2014;9(1):e86244. doi:10.1371/journal.pone.0086244.
- Souillac PO, Middaugh CR, Rytting JH. Investigation of protein/carbohydrate interactions in the dried state. 2. Diffuse reflectance FTIR studies. Int J Pharm. 2002;235(1–2):207–18. doi:10.1016/s0378-5173(01)00987-5.
- Mayer M, Meyer B. Group epitope mapping by saturation transfer difference NMR to identify segments of a ligand in direct contact with a protein receptor. J Am Chem Soc. 2001;123(25):6108–17. doi:10.1021/ja0100120.
- Kemper S, Patel MK, Errey JC, Davis BG, Jones JA, Claridge TDW. Group epitope mapping considering relaxation of the ligand (GEM-CRL): including longitudinal relaxation rates in the analysis of saturation transfer difference (STD) experiments. J Magn Reson. 2010;203(1):1–10. doi:10.1016/j.jmr.2009.11.015.
- Joshi S, Maharana C, Rathore AS. An application of nano differential scanning fluorimetry for higher order structure assessment between mAb originator and biosimilars: trastuzumab and rituximab as case studies. J Pharm Biomed Anal. 2020;186:113270. doi:10.1016/j.jpba.2020.113270.
- Svilenov HL, Kulakova A, Zalar M, Golovanov AP, Harris P, Winter G. Orthogonal techniques to study the effect of pH, sucrose, and arginine salts on monoclonal antibody physical stability and aggregation during long-term storage. J Pharm Sci. 2020;109(1):584–94. doi:10.1016/j.xphs.2019.10.065.
- Bailly M, Mieczkowski C, Juan V, Metwally E, Tomazela D, Baker J, Uchida M, Kofman E, Raoufi F, Motlagh S, et al. Predicting antibody developability profiles through early stage discovery screening. MAbs. 2020;12(1):1743053. doi:10.1080/19420862.2020.1743053.
- Angulo J, Enríquez-Navas PM, Nieto PM. Ligand-receptor binding affinities from saturation transfer difference (STD) NMR spectroscopy: the binding isotherm of STD initial growth rates. Chemistry. 2010;16(26):7803–12. doi:10.1002/chem.200903528.
- Goel H, Hazel A, Ustach VD, Jo S, Yu W, MacKerell AD. Rapid and accurate estimation of protein–ligand relative binding affinities using site-identification by ligand competitive saturation. Chem Sci. 2021;12(25):8844–58. doi:10.1039/D1SC01781K.
- Viegas A, Manso J, Nobrega FL, Cabrita EJ. Saturation-transfer difference (STD) NMR: a simple and fast method for ligand screening and characterization of protein binding. J Chem Educ. 2011;88(7):990–94. doi:10.1021/ed101169t.
- Buchanan CJ, Gaunt B, Harrison PJ, Yang Y, Liu J, Khan A, Giltrap AM, Le Bas A, Ward PN, Gupta K, et al. Pathogen-sugar interactions revealed by universal saturation transfer analysis. Science. 2022;377(6604):eabm3125. doi:10.1126/science.abm3125.
- Svilenov H, Kopp K, Golovanov A, Winter G, Zalard M. Insights into the stabilization of interferon alpha by two surfactants revealed by STD-NMR spectroscopy. J Pharm Sci. 2022:1–7. doi:10.1016/j.xphs.2022.10.013.
- Zalar M, Svilenov H, Golovanova A. Binding of excipients is a poor predictor for aggregation kinetics of biopharmaceutical proteins. Eur J Pharm Biopharm. 2020;151:127–36. doi:10.1016/j.ejpb.2020.04.002.
- Molecular Operating Environment (MOE). 2020. 09. Chemical computing group ULC, 1010 Sherbrooke St West, suite #910. Montreal, QC, Canada H3A 2R7; 2022. http://www.chemcomp.com
- Case DA, Belfon K, Ben-Shalom IY, Berryman JT, Brozell SR, Cerutti DS, Cheatham TE III, Cisneros GA, Cruzeiro VWD, Darden TA, Duke RE, Giambasu G, Gilson MK, Gohlke H, Goetz AW, Harris R, Izadi S, Izmailov SA, Kasavajhala K, Kaymak MC, King E, Kovalenko A, Kurtzman T, Lee TS, LeGrand S, Li P, Lin C, Liu J, Luchko T, Luo R, Machado M, Man V, Manathunga M, Merz KM, Miao Y, Mikhailovskii O, Monard G, Nguyen H, O’Hearn KA, Onufriev A, Pan F, Pantano S, Qi R, Rahnamoun A, Roe DR, Roitberg A, Sagui C, Schott-Verdugo S, Shajan A, Shen J, Simmerling CL, Skrynnikov NR, Smith J, Swails J, Walker RC, Wang J, Wang J, Wei H, Wolf RM, Wu X, Xiong Y, Xue Y, York DM, Zhao S, and Kollman PA. Amber 2022. San Francisco: University of California; 2022.
- Phillips JC, Hardy DJ, Maia JDC, Stone JE, Ribeiro JV, Bernardi RC, Buch R, Fiorin G, Hénin J, Jiang W, et al. Scalable molecular dynamics on CPU and GPU architectures with NAMD. J Chem Phys. 2020;153(4):044130. doi:10.1063/5.0014475.
- Humphrey W, Dalke A, Schulten K. VMD: visual molecular dynamics. J Mol Graph. 1996;14(1). 33-8. doi:10.1016/0263-7855(96)00018-5.
- Abraham MJ, Murtola T, Schulz R, Páll S, Smith JC, Hess B, Lindahl E. GROMACS: high performance molecular simulations through multi-level parallelism from laptops to supercomputers. SoftwareX. 2015;1-2:19–25. doi:10.1016/j.softx.2015.06.001.
- Somani S, Jo S, Thirumangalathu R, Rodrigues D, Tanenbaum LM, Amin K, MacKerell AD Jr., Thakkar SV. Toward biotherapeutics formulation composition engineering using site-identification by ligand competitive saturation (SILCS). J Pharm Sci. 2021;110:1103–10. doi:10.1016/j.xphs.2020.10.051.
- Raman EP, Yu W, Guvench O, MacKerell AD. Reproducing crystal binding modes of ligand functional groups using site-identification by ligand competitive saturation (SILCS) simulations. J Chem Inf Model. 2011;51:877–96. doi:10.1021/ci100462t.
- Raman EP, Yu W, Lakkaraju SK, MacKerell AD Jr. Inclusion of multiple fragment types in the site identification by ligand competitive saturation (SILCS) approach. J Chem Inf Model. 2013;53:3384–98. doi:10.1021/ci4005628.
- Ustach VD, Lakkaraju SK, Jo S, Yu W, Jiang W, MacKerell AD. Optimization and evaluation of site-identification by ligand competitive saturation (SILCS) as a tool for target-based ligand optimization. J Chem Inf Model. 2019;59:3018–35. doi:10.1021/acs.jcim.9b00210.
- Best RB, Zhu X, Shim J, Lopes PE, Mittal J, Feig M, Mackerell AD Jr. Optimization of the additive CHARMM all-atom protein force field targeting improved sampling of the backbone φ, ψ and side-chain χ(1) and χ(2) dihedral angles. J Chem Theory Comput. 2012;8:3257–73. doi:10.1021/ct300400x.
- Huang J, Rauscher S, Nawrocki G, Ran T, Feig M, de Groot BL, Grubmüller H, MacKerell AD. Charmm36m: an improved force field for folded and intrinsically disordered proteins. Nat Methods. 2017;14:71–73. doi:10.1038/nmeth.4067.
- Vanommeslaeghe K, Hatcher E, Acharya C, Kundu S, Zhong S, Shim J, Darian E, Guvench O, Lopes P, Vorobyov I, et al. CHARMM general force field: a force field for drug-like molecules compatible with the CHARMM all-atom additive biological force fields. J Comput Chem. 2010;31:671–90. doi:10.1002/jcc.21367.
- Durell SR, Brooks BR, Ben-Naim A. Solvent-induced forces between two hydrophilic groups. J Phys Chem. 1994;98:2198–202.
- Wu D, Minton AP. Quantitative characterization of the interaction between sucrose and native proteins via static light scattering. J Phys Chem B. 2013;117:111–17. doi:10.1021/jp308880v.
- Holloway L, Roche A, Marzouk S, Uddin S, Ke P, Ekizoglou S, Curtis R. Determination of protein-protein interactions at high co-solvent concentrations using static and dynamic light scattering. J Pharm Sci. 2020;109:2699–709. doi:10.1016/j.xphs.2020.05.023.