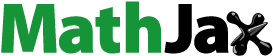
ABSTRACT
In this investigation, we tested the hypothesis that a physiologically based pharmacokinetic (PBPK) model incorporating measured in vitro metrics of off-target binding can largely explain the inter-antibody variability in monoclonal antibody (mAb) pharmacokinetics (PK). A diverse panel of 83 mAbs was evaluated for PK in wild-type mice and subjected to 10 in vitro assays to measure major physiochemical attributes. After excluding for target-mediated elimination and immunogenicity, 56 of the remaining mAbs with an eight-fold variability in the area under the curve (: 1.74 × 106 −1.38 × 107 ng∙h/mL) and 10-fold difference in clearance (2.55–26.4 mL/day/kg) formed the training set for this investigation. Using a PBPK framework, mAb-dependent coefficients F1 and F2 modulating pinocytosis rate and convective transport, respectively, were estimated for each mAb with mostly good precision (coefficient of variation (CV%) <30%). F1 was estimated to be the mean and standard deviation of 0.961 ± 0.593, and F2 was estimated to be 2.13 ± 2.62. Using principal component analysis to correlate the regressed values of F1/F2 versus the multidimensional dataset composed of our panel of in vitro assays, we found that heparin chromatography retention time emerged as the predictive covariate to the mAb-specific F1, whereas F2 variability cannot be well explained by these assays. A sigmoidal relationship between F1 and the identified covariate was incorporated within the PBPK framework. A sensitivity analysis suggested plasma concentrations to be most sensitive to F1 when F1 > 1. The predictive utility of the developed PBPK model was evaluated against a separate panel of 14 mAbs biased toward high clearance, among which area under the curve of PK data of 12 mAbs was predicted within 2.5-fold error, and the positive and negative predictive values for clearance prediction were 85% and 100%, respectively. MAb heparin chromatography assay output allowed a priori identification of mAb candidates with unfavorable PK.
Introduction
Nearly a fifth of new drug approvals are biologics, and their commercial viability has never been stronger than it is now with the recent approval of the 100th monoclonal antibody (mAb) product.Citation1 The success of immunoglobulin G (IgG)-based biotherapeutics is dependent on their high target specificity, Fc-modulated functionality, and favorable pharmacokinetic (PK) properties of long half-life (), and slow clearance. MAb half-lives can range from a few days up to 21 days. Mechanisms of mAb elimination include target-mediated clearance, intracellular catabolism following nonspecific fluid-phase pinocytosis, and interaction with host anti-drug antibodies.Citation2 In the early stages of drug discovery, the strategic focus is usually on identifying an optimal target affinity mAb with high expression, low aggregation propensity, ideal solubility, high chemical stability, low viscosity, and minimal nonspecific binding.Citation3 High off-target binding (herein, off-target binding refers to binding to proteins unrelated to the target or target homologs) is an impediment to mAb development, and downstream risks, including short plasma half-lives, can threaten the success of the therapeutic program. For mAbs known to have minimal target-mediated drug disposition (TMDD), differences in nonspecific binding may be a significant source of inter-antibody variability in mAb plasma clearance. Early identification of mAbs with high nonspecific binding could lead to the replacement, reduction, and refinement of animals used in research according to the “3Rs alternatives” and the Food and Drug Administration Modernization Act 2.0, reduce late-stage attrition, and accelerate the timeline toward the commencement of first-in-human clinical trials.Citation4,Citation5
Several in vitro assays that screen mAbs for undesirable off-target binding based on in silico predicted or observed physiochemical properties have been reported, including isoelectric point (pI),Citation6–9 charge patches,Citation10–12 and hydrophobicity.Citation13 Other published assays measure mAb biophysical properties and interactions that are of potential in vivo relevance, including baculovirus particle assay,Citation14 yeast presentation system assay,Citation3 viscosity,Citation15 affinity-capture self-interaction nanoparticle spectroscopy (AC-SINS),Citation16–22 cross-interaction chromatography,Citation17,Citation21,Citation23 neonatal Fc receptor (FcRn) chromatography,Citation24,Citation25 FcRn AlphaScreen chromatography,Citation26 and heparin chromatography.Citation27 These assays are typically robust, high throughput, and adaptable to early discovery screening paradigms to establish quantitative thresholds that discriminate between fast and slow clearing mAbs in vivo. However, these in vitro assays are often validated using a small set of mAbs and may fail to predict the PK of a larger diverse panel of mAbs with dissimilar scaffolds. Bivariate statistical analyses, including Pearson, Kendall, or Spearman, are commonly used to establish relationships between physiochemical metrics and PK parameters (i.e., clearance and ). In many cases, the strength of association between a specific biophysical metric and a PK parameter is limited to mAbs representing a small subset of the chemical space or involves panels that are so small their statistical significance is questionable. A comprehensive evaluation of in vitro in vivo relationships between PK parameters and diverse mAb physiochemical properties using a multivariate statistical approach in a preclinical setting is lacking. To address this gap, in this investigation, we measured in vivo mouse PK and evaluated 10 assays selected to exploit a broad range of mAb physiochemical attributes across a diverse panel of 83 mAbs.
A PK model was used to evaluate the effectiveness of diverse physiochemical properties to predict PK profile for the mAb panel. Physiologically based pharmacokinetic (PBPK) models are considered the ‘gold standard’ for characterizing biodistribution of mAbs.Citation28 These models use a comprehensive mathematical representation of critical physiological processes governing antibody uptake, distribution, and elimination. For the population of selected mAbs, we hypothesized that within a PBPK framework, experimentally determined in vitro physiochemical assay metrics could serve as covariates of uptake clearance or convective transport to explain the inter-antibody variability in mAb plasma PK. Here, we modified an existing PBPK platform to establish meaningful relationships between mAb-specific in vitro metrics of off-target binding and tissue uptake clearance. The objective was to evaluate the predictive utility of this PBPK model to triage and de-risk antibodies in early discovery preclinical screening allowing a priori characterization of mAb concentration-time profiles.
Results
mAb panel selection
In order to build a robust dataset linking antibody sequence and physiochemical properties to PK, we selected and recombinantly produced a set of 83 antibodies in a consistent human IgG1 isotype. The selected isotype, IgG1z stable effector functionless (SEFL) 2.2, is an aglycosylated IgG1 of the G1m17 allotype that has been engineered with an N297G mutation to reduce binding to Fcγ receptors and R292C and V302C mutations to install an engineered disulfide bond to improve thermal stability.Citation29 The 83 Fv domains were carefully selected from both internal and publicly available external databases to exhibit a broad range of physiochemical behaviors, with an emphasis on colloidal stability at high concentration, and without consideration of target biology. The final set of 83 antibodies included binders to 33 different target antigens. For the current analysis, we eliminated 19 mAbs with mouse cross-reactivity (data not shown) to avoid potential TMDD, and a further eight mAbs due to observed immunogenicity, which enabled focused characterization of the impact on clearance of nonspecific binding and other physiochemical properties for the remaining 56 mAbs.
In vitro assessment of the mAb panel
We expected several assays to provide data that might be useful for PK prediction based on previous reports in the literature, including self-interaction assays,Citation21,Citation30–32 and cross-interaction assays to a variety of substrates,Citation30,Citation33,Citation34 including those of hydrophobic (membrane preparation (membrane_prep) enzyme-linked immunosorbent assay (ELISA), hydrophobic interaction chromatography (HIC)), negatively charged (heparin chromatography,Citation27 polyethyleneimine (PEI) ELISA), positively charged (poly-D-lysine ELISA), or mixed (baculovirus particle (BVP) ELISA)Citation14 characteristics. We also measured the interaction of each mAb with FcRn using FcRn chromatography,Citation24 and FcRn AlphaScreen binding assay,Citation26 in order to assess the impact of diverse Fab sequences on FcRn engagement by our invariant Fc sequence.Citation10,Citation35 Additionally, we evaluated nonspecific protease stability using a novel thermolysin assay (PK study and assay details included in ).
Table 1. Data collected on the antibody panel.
Evaluation of PK data
The parameters collected from these assays and mouse PK studies performed in female BALB/c mice at 2 mg/kg using the intravenous (IV) route of administration and cassette dosing are reported in Supplementary Table S1. After excluding mAbs with potential TMDD or immunogenicity based on known evidence or visual check of plasma concentration-time profiles, 56 molecules remained for further analysis. These mAbs exhibit modest variability in their PK profiles and nonspecific clearance (). An approximately eightfold difference in the area under the curve (AUC) was observed, with the minimal and maximal being 1.74 × 106 and 1.38 × 107 ng∙h/mL, respectively. Accordingly, a roughly 10-fold variability in clearance was obtained (2.55–26.4 mL/day/kg). Only six mAbs were classified as high clearance drugs, using an internal threshold value of 12 mL/day/kg (roughly corresponding to
cut-off of 3.9 × 106 ng∙h/mL at dose of 2 mg/kg IV in the dataset). Consistently, the majority of these mAbs exhibit similar PK profiles, with a
of about 7–14 days, and five high clearance mAbs Ab84, Ab14, Ab36, Ab63, and Ab39 have
of only 2–5 days. Notably, one high clearance drug, Ab76, displays a very atypical PK profile, where plasma concentrations drop rapidly initially, followed by a relatively shallow terminal phase, with a normal
of 9 days (, highlighted in red).
Figure 1. Overview of PK results of 56 mAbs in the training set following 2 mg/kg IV dosing in mice. (a) bar chart of clearance of different mAbs, with the red dotted line indicating a threshold value of 12 mL/day/kg, above which a mAb is identified as a high clearance molecule. (b) spaghetti plot of PK profiles of the mAbs. The red curve indicates the atypical PK profile of Ab76.

Identification of covariate(s) in the PBPK model
We used the base PBPK model structure reported by Shah and BettsCitation36 and adopted the formalism of antibody variability proposed by Chen and Balthasar.Citation37 Briefly, was multiplied by the pinocytosis rate (
) to reflect variability in cellular uptake, whereas
was multiplied by organ lymphatic flow (
) to represent variability in the convection (). Antibody-dependent
and
values were estimated for each of the 56 mAbs by fitting individual PK profiles using the PBPK model, with their final estimates and the associated precision listed in Supplementary Table S2.
values were estimated with very good precision (CV%<15%), and the estimates range from 0.419 to 3.61, with the median, mean, and standard deviation of 0.799, 0.961, and 0.593, respectively.
values were generally estimated with good precision as well; however, when
was estimated to be extremely small (<1e-5), the associated CV% became extremely large, even if a numerical transformation of F2 was applied. Further investigation suggested that PK profiles are not sensitive to F2 when F2 < 0.5 (data not shown). The range, median, mean, and standard deviation of
are [1.20e-7, 17.6], 1.44, 2.13, and 2.62, respectively.
Figure 2. General schematic of PBPK model and tissue sub-compartments for mAb. a. Whole body model. A physiologically based pharmacokinetic model depicts the distribution of antibodies across several key organ sites, which are connected anatomically by plasma flow (Q) and lymphatic flow (L). b. Organ sub-compartment. Every organ compartment is divided into vascular, endosomal, interstitial space and cellular space/sub-compartments. mAb can enter the vascular space via convection, the interstitial space via the lymph modulated by the vascular reflection coefficient (σv), and the endosomal space by fluid-phase pinocytosis (Clup). F1 and F2 are coefficients that account for inter-antibody variability in tissue uptake applied to modulate the rate of endosomal uptake (Clup) and σv. The KD regulates the formation of the mAb-FcRn complex in the endosomes. FcRn-mAb complexes can recycle to vascular space or interstitial space. Unbound mAb in the endosomal space can undergo degradation. Unbound mAb can be removed from the interstitial space by the lymph modulated by the lymph reflection coefficient (σL).

Given the fact that a diverse array of in vitro assays was conducted and that some assays are likely to be correlated, we implemented principal component analysis (PCA) to explore inter-dependencies of these assays as well as clustering patterns of F1/F2 values (). Indeed, the PCA plot indicates that many assay outputs are highly correlated, such as BVP, BVP_high, Heparin_RT (Heparin chromatography retention time), Heparin_pB_buffer (Heparin percent B buffer), poly_D_lysine, PEI, membrane_prep, and calculated pI (), which are all assays that evaluate charge-based nonspecific interactions. The pairwise correlation analysis also supports that conclusion and shows stronger evidence of correlations between FcRn_RT (FcRn chromatography retention time) and those assays detecting nonspecific binding, compared to the PCA result (Supplementary Figure S1). Both the PCA results, and the correlation matrix suggest that AC-SINS, HIC, FcRn_AlphascreenBinding_IC50, and thermal stability experiments are relatively orthogonal to nonspecific binding assays. To facilitate the identification of clusters or patterns and
were each categorized into three groups (high, medium, or low values). The F1 PCA plot shows no separation between the low- and medium-
classes, but the mAbs with high
estimates all have high scores in nonspecific binding assays (). In contrast, there is no clear pattern on the
PCA result (). Accordingly, we did not further pursue establishing a covariate relationship for
.
Figure 3. Statistical analysis of individually estimated and
values. (a) and (c) are PCA results on estimated
and
values, respectively. (b) is an enlarged view of (a) showing several highly positively correlated assay outputs, including BVP_high, BVP, Heparin_pB_buffer, Heparin_RT, poly_D_lysine, PEI, membrane_prep, and pI. (d) a sigmoidal relationship between
value and Heparin_RT. The red curve represents a mathematical expression that gives sigmoidal response, and the blue dashed line indicates a threshold Heparin_RT value (16.5 min) above which a mAb can have much higher
values. Unfilled circles represent Ab37 and Ab30 that were identified as false positives and removed from the training dataset. Since Ab76 has a Heparin_RT of 31.54 min that is much higher than all the other mAbs and displays an atypical PK profile, it is not included in this plot.

A more detailed examination of correlations between estimated values and individual assays reveals that Heparin_RT is the most significant variable against which
displays a trend (). Subsequently, we excluded three molecules (Ab37, Ab30, and Ab76) when developing a mathematical expression for
using Heparin_RT as a covariate based on the following rationale. Ab76, the mAb with the highest clearance in the panel, displays a distinct PK profile (). While other high clearance mAbs all have steep terminal elimination phase, Ab76 exhibits an unusually rapid drop in the concentration during the distribution phase, and a relatively shallow terminal elimination phase.
mainly impacts the distribution phase, whereas
determines the terminal elimination phase. Expectedly, its
was estimated to be extremely high, and the
estimate was only modest (Supplementary Table S2). Such a profile will not be captured by a model whose variability is completely controlled by
. Moreover, compared to other compounds, Ab76 exhibited an extremely large Heparin_RT (31.5 min vs. 20.3 min); it was also in the high range for pI (9.22) and BVP_high binding (1.67), and exhibited the lowest HIC retention time scores (pH 7.4 = 35.9 min; pH 6.0 = 33.8 min). Furthermore, Ab76 has a high number (4) of positive charge patches in our in silico analysis (Supplementary Figure S2A). Because Ab76 is the only mAb that shows these unique properties, it was removed from the model training process. On the other hand, Ab37 and Ab30 both have high scores in many nonspecific interaction assays, such as FcRn_RT, Heparin_RT, BVP binding, membrane_prep, poly_D_lysine, PEI, and Thermolysin_Stability, yet their PK profiles, and the estimated
values fall in the normal range. We reason that these molecules will be recognized as false positives in risk models based on current best knowledge and developing a covariate relationship based on such outlier molecules will compromise the ability of the model to predict PK profiles for true positives (i.e., molecules with both bad assay outputs and bad PK results).
Fitting of the PBPK model incorporating the Heparin_RT covariate
After removing Ab76, Ab37, and Ab30, 53 mAbs remain in the training dataset. Since the correlation plot () suggests a sigmoidal relationship between mAb-specific and Heparin_RT, we expressed
as follows:
so that remains steady when Heparin_RT is smaller than a threshold value (e.g., 16.5), increases rapidly once Heparin_RT exceeds that value, and soon reaches another plateau. Although no covariate relationship was defined for
, we fixed
to 1.45, the median
value in this panel, to capture distribution phases of most of the molecules. The PBPK model incorporating the covariate relationship of
was fitted simultaneously using PK profiles of all the 53 mAbs. The estimates and associated CV% for the coefficients/constants are shown in , and the fitted profiles superimposed on actual data are shown in .
Figure 4. PK of mAbs in the training set that have (a) low clearance and (b) high clearance. Different mAbs were dosed IV into mice at a 2 mg/kg dose. Observed data are shown as solid dots, whereas model-fitted profiles are shown in solid lines.

Table 2. Parameter estimation results of simultaneous fitting of 53 mAbs in the training set.
This updated PBPK model captures PK profiles of the majority of the mAbs with either high or low clearance. Notably, significant over-prediction of plasma concentrations was observed for Ab45 and Ab51 in the low clearance panel (), and the reasons can be quite different. Failure to capture PK of Ab45 may be attributed to immunogenicity or TMDD, as individual fitting for Ab45 (i.e., and
estimated) did not yield a satisfactory result either, given its 3-phase PK profile. Ab45 was not excluded during initial screening of immunogenicity and TMDD because we were quite conservative about removing any mAbs from the panel and Ab45 did not show binding to the mouse target homolog (data not shown). However, potential specific off-target binding of Ab45 not detected in our assay was still likely to compromise PK.Citation38 Moreover, this molecule never eluted from the column (elution time >500 min) in the HIC assay at pH 6 (Supplementary Table S1), suggesting extraordinarily high hydrophobicity at acidic pH, which may expedite degradation of the mAb within endosomes, ultimately leading to poor PK. On the other hand, further investigation of Ab51 reveals a significant positive charge patch compared to Ab54, a closely related mAb that differs by only two amino acids (Supplementary Figure S2b). Many of the nonspecific interaction assays did not capture differences between these two mAbs, except that Ab51 had a fairly high score on membrane_prep (8.27). However, membrane_prep is overall not a good predictor, since many molecules with higher membrane_prep values still exhibit good PK (Supplementary Table S1). In the high clearance panel, the PK profile of Ab63 was overpredicted, because its Heparin_RT of 12.7 min is not flagged (), indicating that more covariates may be needed to fully identify mAbs with poor PK.
It is interesting that a single value of about 0.68 can describe PK profiles of mAbs reasonably well when Heparin_RT values are smaller than 16.5 (), despite nontrivial variability in the individually estimated
(). This is not surprising, as the sensitivity analysis suggests that plasma concentrations are only sensitive to
when
> 1 (). This also indicates that there is marginal benefit to further account for the variability in PK when
< 1.
Figure 5. Sensitivity analysis of . PK profiles following a 2 mg/kg IV dose were shown. The color bar from red to blue indicates an
range of 0.4 to 4.

Validation of the full PBPK model using a post-hoc test dataset
The biggest caveat of the training dataset is that the majority of the molecules had good PK and low Heparin_RT values (), making us less confident in the capability of the model to project molecules with bad PK. Coincidentally, Amgen had an internal panel of bispecific antibodies that exhibited very poor PK with reasons unexplored. To test whether Heparin_RT is a useful predictor and if the full PBPK model could a priori predict PK data, we constructed monospecific SEFL2 antibody formats of these antibodies and conducted heparin chromatography only and in vivo PK studies in a manner similar to the training set.Citation29 It was anticipated that this test panel of monospecific mAbs (n = 14) would be biased toward exhibiting poor PK.
shows model-predicted concentration-time profiles superimposed with raw data, and the percent prediction error (%PE) in AUC is shown in Supplementary Table S3. Only one molecule, Ab97, has a Heparin_RT of 15.1 min, and all the other 13 mAbs have high Heparin_RT values greater than 16.5 min. Although 10 mAbs possess Heparin_RT values larger than the maximum Heparin_RT used in the training dataset (i.e., >20.296 min), the model still did a decent job in capturing PK data from this validation set. Quantitatively, significant underpredictions were observed for Ab87, Ab89, and Ab90, and significant overprediction was observed for Ab95 and Ab98. The result was also evaluated in a qualitative manner. Using the preset clearance threshold value of 12 mL/day/kg, the molecules can be categorized as high or low clearance mAbs (Supplementary Table S3). The positive predictive value is 85% and the negative predictive value is 100%. The false-positive rate and false-negative rate are 67% and 0%. Since this test set was built for the purpose of having a series of bad molecules, these statistical metrics do not reliably reflect the holistic predictability of the current PBPK model.
Figure 6. PK of mAbs in the test set. Different mAbs in the test set were dosed IV into mice at a 2 mg/kg dose. Observed data are shown as solid dots, whereas model-predicted profiles using obtained Heparin_RT as inputs are shown in solid lines.

Discussion
Therapeutic mAbs containing the same Fc domain can exhibit a wide range of clearance values that often cannot be accounted for by TMDD.Citation14,Citation35,Citation39 Here, we used a PBPK model to predict the clearance and PK profiles of a panel of 53 mAbs in mice. To account for differences between the mAbs in the model, we evaluated the panel in 10 in vitro assays designed to interrogate a range of mAb physiochemical attributes. Using PCA and nonlinear regression, we found that Heparin_RT was a key covariate of clearance variability in mice, more specifically, in the pinocytotic uptake coefficient F1 in our PBPK model. Utilization of this covariate improved our ability to predict mAb PK, and our model was validated using an additional test set of 14 mAbs. Furthermore, heparin chromatography has now been implemented as a routine assay in-house to screen mAbs for potential PK issues prior to running in vivo studies.
Existing modelling frameworks to predict mAb PK from in vitro or in silico parameters
A plethora of models have been reported to correlate preclinical or clinical mAb clearance with in vitro or in silico metrics. These models have varying degrees of complexity and can be categorized into three types: (1) threshold cut-off models, (2) correlation/regression models, and (3) mechanistic PBPK models. provides a summary of published studies that used models from each of these categories.
Table 3. Summary of published models reported to predict in vivo mAb clearance from in vitro or in silico data.
The threshold cutoff category includes empirical, binary models where threshold values of in vitro assay readouts or in silico parameters serve as warning flags that discriminate mAbs with poor PK from those with acceptable clearance. For example, a mean fluorescence intensity value above 500 from the polyspecificity reagent (PSR) binding assay was predictive of high clearance in C57BL/6 mice,Citation30 and a BVP binding score above five was associated with increased risk of fast clearance in both cynomolgus monkeys and humans.Citation14 However, other studies found it difficult to establish such clear-cut relationships using a single assay. Avery et al.Citation46 reported that while threshold values from six in vitro assay parameters could identify rapid clearance in Tg32 mice, a number of false positives always existed. The authors suggested a conservative strategy where only mAbs scoring above the threshold in three assay classes (nonspecific interactions, self-association, and FcRn binding) simultaneously should be rejected without further investigation.Citation33 In another study, low retention times on heparin chromatography alone or in combination with low retention on FcRn chromatography allowed the prediction of antibodies with good PK in cynomolgus monkeys.Citation27 Abnormal clearance in cynomolgus monkeys was also associated with either high hydrophobicity of certain complementarity-determining regions (CDRs) or extreme Fv charge at pH 5.5,Citation15 but this in silico, two-dimensional criteria performed poorly in mice and humans.Citation30,Citation40 On the other hand, a random forest machine learning (ML) algorithm identified PSR binding and sequenced-based predicted pI as top-ranked biophysical properties influencing human clearance.Citation40 In general, these qualitative models are useful de-risking tools that facilitate ‘go,’ ‘no-go’ decisions during early development to minimize the likelihood of mAbs with good in vitro pharmacological effects but poor PK properties progressing.
The second model category is composed of empirical, quantitative approaches that explicitly express PK parameters as mathematical functions of assay outputs based on correlation or regression analysis. On a biolayer interferometry platform, a hybrid parameter termed “fold change in FcRn binding rate,” which accounted for both association rate at pH 6 and dissociation rate at pH 7.4 between FcRn and IgG, demonstrated linear correlation with human Citation41 Furthermore, transcytosis in FcRn-overexpressing Madin-Darby canine kidney (MDCK) cells exhibited a strong and linear relationship with human mAb clearance.Citation42 In addition to linear regression against a single parameter, more statistically vigorous approaches have been applied. For a panel of eight mAbs, the least absolute shrinkage selection operator regression (LASSO) ML technique was used to identify the best combination of two in vitro parameters associated with human clearance and
as FcRn binding at pH 7.0 and thermal stability (as indicated by the change in heat capacity). Multiple regression was then performed using the two predictors. The resultant formulae trended well with human clearance and
Citation43 Compared to binary models, these regression approaches allow rank-ordering of drug candidates as well as in vivo clearance prediction, thus further reducing the number of molecules advanced to the next development stage.
The third category of published models are mechanistic PBPK models that address the time-course of mAb concentrations rather than a single parameter such as clearance or based on in vitro and/or in silico metrics. The first attempt of this category was by Jones et al.Citation31 who incorporated nonspecific charge-mediated binding of mAbs with endothelial cell membrane followed by internalization into a PBPK model. In vitro in vivo correlation (IVIVC) was achieved by expressing nonspecific binding the dissociation rate constant (KD) as a function of AC-SINS score, and the resultant model yielded PK profiles in good agreement with observations in Tg32 mice and humans. Hu and D’ArgenioCitation44 went further and elucidated between-mAb differences in linear PK following subcutaneous (SC) dosing in humans using a two-pore PBPK model. Using IV and SC PK data, the authors found that mAb-specific pinocytosis rate and pre-systemic clearance were positively correlated with patches of positive charge in the CDR vicinity, an in silico term derived from the Therapeutic Antibody Profiler platform.Citation47 More recently, Hu et al. updated this framework model by incorporating mAb PK data in humans with results from 14 different published in vitro assays. Only heparin chromatography retention time was found to be significantly associated with the pinocytosis rate.Citation45
Utilization of IVIVC in PBPK models not only allows better delineation of both α and β phases of different mAb PK profiles but also specifically links assay performance to associated physiological mechanisms. In this work, our approach was analogous to Hu et al.Citation45 which allows for inter-antibody variability on pinocytosis rate and convective transport. In particular, we used preclinical PK data from mice that exhibited a broader range of clearance values compared to human data (~8-fold vs. ~3-fold differences in Hu et al.)Citation45 since clinically tested mAbs are greatly biased toward favorable physiochemical properties and hence clearance.
Parsing disparate predictive parameters identified across different modelling frameworks: PBPK model-estimated pinocytosis correlates with heparin retention time
Intriguingly, across these studies, different research groups came to quite distinct conclusions. Established models frequently failed to reproduce satisfactory correlations with mAb PK in follow-up publications, which might be attributed to small n per study, resulting in a lack of statistical power, overfitting of models (especially when more than one assay parameter was utilized), and individual study differences including a limited range of mAb clearance values, species or strain differences, divergent assays and assay formats, differences in clinical-stage vs preclinical-stage mAbs, and so on. Despite this, the most robust predictors of mAb PK arguably remain nonspecific binding as determined in assays such as heparin chromatography, BVP ELISA, and DNA/insulin binding ELISA, or rooted in risk factors such as patches of positive charge or predicted pI. In agreement with Hu and coworkers,Citation45 we found that model-estimated pinocytosis correlates with Heparin_RT, although when a larger panel of mAbs with more diverse Heparin_RT was examined, a sigmoidal curve instead of linear regression more appropriately delineated such association. Our finding further supports this assay as a useful and robust in vitro parameter predictive of mAb PK.
What may seem a bit controversial is the absence of FcRn_RT and AC-SINS as covariates in the work of Hu et al.,Citation45 and in our model, as these two assays were reported as significant predictors of clearance in some studies. It is well recognized now that both high-affinity mAbs FcRn binding at pH 6 and efficient dissociation of the mAb-FcRn complex at pH 7.4 are critical for prolonged systemic circulation of mAbs. Although binding at acidic pH often fails to correlate with in vivo clearanceCitation14,Citation48 researchers have observed correlations between dissociation at pH 7.4 or FcRn chromatography retention time and PK in Tg32 mice, non-human primates, and humans.Citation10,Citation48 However, such correlations do not fully justify futile FcRn recycling as the primary mechanism of compromised PK in all the studies. Kelly et al. demonstrated that some mAbs exhibiting prolonged retention by FcRn chromatography also display more rapid clearance in FcRn knockout mice, suggesting a significant contribution of a more universal mechanism, such as non-specificity accounting for poor PK.Citation35 This example implies that assays aimed to characterize atypical FcRn binding interactions as a surrogate measure for suboptimal endosomal recycling efficiency need to consider the potential for contributing mechanisms to poor PK in the context of endosomal/lysosomal trafficking. Specifically, the manifestation of nonspecific interactions between negatively charged proteins and cell surfaces with positive charge patches on mAbs are of high potential consequence to rapid clearance. This is further supported by our observation of modest correlations between FcRn_RT and several nonspecific binding assays, as well as the apparent correlations between FcRn_RT and heparin column retention time reported by Kraft et al.Citation27 Moreover, longer retention time on an FcRn column not only reflects the delayed release of IgG from FcRn resulting in faster clearance but is also observed for extension constructs such as YTE mAbs, making the interpretation of the chromatography data more complicated.Citation24 We do agree that the significant retention of mAbs on FcRn at extracellular pH can result in poor PK, especially when the possibility of nonspecific binding has been rejected.Citation49 Therefore, to separate out the impact of FcRn interaction on PK, variability should be placed on FcRn-mediated processes instead of on pinocytosis or paracellular convection, but the associated identifiability issue of the existing model precludes such strategy.
Finally, AC-SINS has also been demonstrated to positively correlate with clearance in Tg32 mice and human,Citation31,Citation33 but other studies, including ours, failed to establish such correlation using different datasets in wild-type mice and human.Citation30,Citation40,Citation45 Mechanistically, self-association (determined via AC-SINS) and nonspecific binding (measured via heparin chromatography) represent two facets of antibody specificity,Citation13,Citation50 with the former mainly linked to low solubility and high viscosity in vitro and the latter linked to high clearance in vivo. Indeed, in the study where AC-SINS appeared to correlate with clearance, the majority of mAbs with above-threshold values in AC-SINS were also identified as red flags in nonspecific binding assays.Citation33 By contrast, the AC-SINS readout is uncorrelated with any of the nonspecific binding assays in our dataset, and therefore it is not surprising to see that this parameter did not provide predictive value for in vivo clearance here.
Mock et al.Citation51 pursued the same panel of 83 mAbs to investigate an ML approach to predict mAb PK. Mock and coworkersCitation51 used protein sequence data, in silico predicted protein structure, a more diverse set of in vitro experimental data, and ML-based computational strategies to predict key mAb PK parameters (AUC). Their ML approach identified nonspecific binding assays (membrane prep assay score, poly-D-Lysine assay, and BVP ELISA score) as the lead predictor of the AUC parameter. Evidently, these assays were found to be highly correlated to heparin chromatography retention time in our PCA analysis. Although, we note several differences between the ML approach used by Mock et al.Citation51 and our PBPK approach, both investigations are in agreement that the aforementioned in vitro nonspecific binding assays are reliable predictors of mAb PK and can be used in the early discovery phase to identify candidates with unfavorable PK.
Finally, current biologics pipelines now include diverse formats such as t1/2 extended mAbs, tetravalent bispecifics, Fc-fusion peptides, and antibody-peptide conjugates. We foresee the strategic principles used in this investigation to identify the significant covariate(s) and its incorporation into a PBPK framework could apply to novel formats to enhance the efficiency of early discovery screening and accelerate drug development. Biologics that are less than 60 kDa in size would require consideration of diffusive transport and additional non-target mediated clearance mechanisms, such as the renal clearance pathway that may be susceptible to the impact of physiochemical factors including charge.
Heparin chromatography as a pre-PK screening tool and mechanistic underpinnings of false positives and false negatives
A secondary goal of the current work was to summarize a minimal set of in vitro assays necessary for mAb characterization in preclinical development, with a focus on predicting in vivo PK. Jain et al. observed five clusters of biophysical properties captured by a series of 12 in vitro assays, i.e., cross/self-interaction, thermodynamic stability, hydrophobic interaction, long-term aggregation propensity, and nonspecific binding.Citation13 Although we did not perform a stringent hierarchical clustering in this case, similar yet slightly different groupings of properties were observed in PCA, including charge-based binding (heparin chromatography, poly-D-lysine assay, PEI ELISA, BVP ELISA, membrane prep assay), hydrophobicity (HIC), self-association (AC-SINS), protease stability (thermolysin assay), and FcRn association (FcRn chromatography). Many of these diverse assays are highly correlated and redundant. Considering that heparin chromatography is the best predictor of PK in this study, and false negatives could not be confidently flagged in other nonspecific interaction assays, heparin chromatography is now preferred and routinely run prior to running in vivo studies to evaluate clearance. Assays such as HIC, despite lacking correlations with pinocytosis rate and hence PK, can sometimes capture abnormal behavior of mAbs that also predicts poor PK. Moreover, false negatives and false positives in the panel of mAbs across assays have inspired our interest in the underlying mechanisms.
For the false-negative molecules (i.e., low scores in nonspecific binding assays but above-threshold clearance), in silico analyses can reveal additional risk factors not fully captured by in vitro assays, such as positive charge/hydrophobic patches. Additionally, cellular assays containing relevant IgG receptors such as liver sinusoidal endothelial cell uptake and transcytosis in FcRn-overexpression MDCK cells can simultaneously evaluate nonspecific uptake, receptor-mediated uptake, and FcRn-mediated salvage in a more integrative manner, providing valuable information absent in simpler assays, although the throughput is limited.Citation42,Citation52 The reasons for false positives (i.e., mAbs with high scores in nonspecific binding assays but with acceptable PK) remain puzzling. One possibility is that the SEFL constructs have removed some risks of extreme molecules in vivo via some mechanism not captured in this set of assays. Interestingly, even though false positives in this study exhibited good PK as monospecific mAbs, once they were transformed into bispecific antibodies, poor PK was frequently observed (data not shown); this finding requires further investigation. Another phenomenon of interest is the distinct PK profiles of mAbs with accelerated clearance. Notably, Ab76 displayed an extremely steep α phase followed by a relatively normal β phase, as opposed to the average α phases and steep β phases observed for most other mAbs with poor PK involved in this study (training and validation sets altogether). There are no notable differences between these mAbs in terms of assay readouts, so further mechanistic studies should be carried out to identify the unique factors underlying the atypical behavior of mAbs like Ab76.
Study limitations
Four key hurdles exist in utilizing the current PBPK model to predict human PK based on heparin chromatography. First, we lack PK data of sufficient mAbs with unfavorable physiochemical properties, making it difficult to establish reliable covariate relationships and/or validate a clinical model. Even in this preclinical study, investigational mAbs are still enriched for low clearance, posing a challenge in developing IVIVC in PBPK models. In the model training process, we excluded two false positives (i.e., mAbs with flags in many nonspecific binding assays yet with acceptable PK profiles), considering that we only had a limited number of molecules with bad PK initially, and these two mAbs would have been falsely flagged in many models discussed above based on our current knowledge. Our rationale is that the stringency of the model development process can be compromised to ensure the generalizability and usefulness of the resultant model if the scientific reason is valid.
Second, although Heparin_RT appears the top predictor of mAb PK in this practice, we still notice huge mAb PK variability when Heparin_RT is large, leading to PK underprediction almost 30% of the time (). This may be attributed to other confounding factors impacting mAb PK that are not well understood as yet.
Third, the present study utilized wild-type mice. BALB/c mice are relatively inexpensive, easy to procure, and are often the genetic background strain for the development of disease models used to screen mAb candidates in the early discovery phase. However, these mice are known to be inferior to Tg32 mice in terms of projecting human clearance,Citation46 since the human IgG – mouse FcRn interaction is 15-fold higher than the human IgG – human FcRn interaction.Citation53 Nevertheless, we expect the association between heparin chromatography retention time and the pinocytosis rate to still hold in Tg32 mice and humans, but its contribution to the overall PK will likely need to be altered by adjusting the coefficient values in the covariate formula.
Finally, to reduce the number of animals used while assessing the in vivo PK of a large number of molecules, we used a cassette dosing strategy in which up to five mAbs were co-injected in each animal group. The major limitation of this approach is the impact of immunogenicity, as ADAs that arise against one mAb may also affect the clearance of the other co-dosed mAbs in that animal, as they share the same IgG backbone. An additional possibility is that competition for FcRn binding and recycling could affect the clearance of the individual mAbs because FcRn is a saturable pathway. However, at the dose levels used in our study, with a combined total of 10 mg/kg, it is unlikely that FcRn saturation levels were reached, and therefore unlikely that clearance of our molecules was affected. To test this, a select number of molecules were also run individually and no significant differences to the results from the cassette dosing study were observed (data not shown).
Conclusion
With the inclusion of heparin chromatography to inform model-estimated pinocytosis, our validated application of PBPK modeling to predict mAb clearance preclinically in wild-type mice provides further validation of a similar approach in humans that was recently published by Hu et al.Citation45 Taken together, these studies further substantiate mounting evidence that nonspecific binding, largely modulated by interactions between positively charged patches on the mAb surface and negatively charged surfaces in systemic circulation, the interstitium, the cell surface, and within endosomes, is a significant driver of mAb PK and an attribute that should routinely be evaluated in vitro using heparin chromatography prior to testing in vivo in animals and humans.
Materials and methods
PK study
Animals
Mice were housed in groups at an AAALAC, International accredited facility. Animals were cared for in accordance with the Guide for the Care and Use of Laboratory Animals, 8th Edition. All research protocols were reviewed and approved by the Amgen Institutional Animal Care and Use Committee (IACUC). Female BALB/c mice were ordered at 6–8 weeks of age from Charles River Laboratories (Hollister, CA). For each dosing group, the test articles were combined into a single vial to generate the cassettes (a total of 5 mAbs/cassette). The dose level for each mAb per cassette was 2 mg/kg. For cassettes with less than 5 mAbs an equivalent amount of isotype control antibody was included to maintain the same total antibody load for each group. The mice received the test article cassettes through an IV bolus dose via the lateral tail vein. Whole blood samples were collected and processed to serum at various time points (n = 3 per time point).
Animal sample analysis
Quantitation of mAbs in mouse serum was performed using multi-plex electro-chemiluminescent immunoassays using the Meso Scale Discovery (Rockville, MD) U-PLEX system on a Sector 600 instrument. In brief, biotinylated recombinant target proteins (proprietary internal reagent) for each mAb were used as capture reagents by coupling to U-PLEX Linkers. The U-PLEX Linkers then self-assemble onto unique spots on the U-PLEX plate. After incubation of the samples on the coated plates, an anti-human Fc antibody (proprietary internal reagent) labeled with ruthenium (MesoScale Discovery; catalog# R91AO) was used as the detection reagent for all mAbs. In all assays, the analyte serum concentrations were interpolated from standard curves using the corresponding analyte prepared in pooled mouse serum. Noncompartmental analyses were performed using the mean of three animals per time point in non-GLP Watson LIMS v7.5 SP1 (Thermo Fisher Scientific, Philadelphia, PA). AUC values were calculated using the linear trapezoidal linear/log interpolation method.
Mouse cross-reactivity determination
Mouse cross-reactivity of mAbs was performed using electro-chemiluminescent immunoassays using the MSD system on a Sector 600 instrument. Biotinylated recombinant target proteins for each mAb were used as capture reagents by coating onto MSD streptavidin plates. For each target both mouse and human proteins were coated, with the human proteins serving as positive controls. The antibodies of interest were incubated at a range of 0.064 to 1000 ng/mL, followed by detection with an anti-human Fc antibody (proprietary internal reagent) labeled with ruthenium (MesoScale Discovery; catalog# R91AO). Antibodies exhibiting strong binding to the recombinant mouse protein were determined to be cross-reactive and excluded from further analyses.
MAb generation
The antibodies were produced under consistent conditions using a stable Chinese hamster ovary batch production, purified by protein A affinity capture followed by cation exchange chromatography, and formulated into a consistent buffer (10 mM sodium acetate, 9% sucrose, pH 5.2). All final proteins lots were > 95% pure as measured by both analytical size exclusion chromatography and non-reducing microcapillary electrophoresis. In all cases, we produced >200 mg of protein to support a comprehensive evaluation of each protein lot in a battery of assays and a mouse PK study.
Non-compartmental analysis
Non-compartmental analysis (NCA) parameters were calculated using PKNCA package in R software.Citation54
Individual fitting of antibody PK using the base PBPK model
The base PBPK model is a combination of the platform PBPK model developed by Shah and BettsCitation36 and the variability terms described by Chen and BalthasarCitation37 (). Briefly, 15 major tissues are connected by blood flow and lymph flow (). Each tissue is further divided into vascular, endosomal, and interstitial spaces (). Antibodies enter tissues via either paracellular convection, represented by the lymph flow- and reflection coefficient-dependent term, , or pinocytosis (
) into endothelial endosomes, where mAbs bind to FcRn and get recycled to the plasma (
) or interstitial space (
), and the unbound mAbs are degraded (
). In the interstitial space, mAbs exit the tissue via lymph flow (
). Since mice do not express relevant antigens, no antigen–antibody interaction is included in the base model. Essential differential equations are provided in Supplementary Methods 2. The parameter glossary and values are the same as in Shah and BettsCitation36 except that the
value is set to 0.24 L/h/L. Antibody-specific
and
values were estimated by fitting PK profiles of individual mAbs separately.
Principal component analysis
First, to prepare the dataset for PCA, individually estimated values within the ranges of [1.8, +∞), [1.1, 1.8), and (0, 1.1), are categorized into “High”, “Medium”, and “Low”
classes. These boundary values were determined based on inflection points in the waterfall plot of
values. Similarly, mAb-specific
within [1.88, +∞), (0.519, 1.88), and (0, 0.519], are classified as “High”, “Medium”, and “Low”
values. Second, each variable (i.e., in vitro assay outputs) was standardized so that different assay results contributed equally to the analysis. Subsequently, PCA was conducted in R. The first two principal components from PCA were plotted to identify any clusters of
or
classes. Pearson correlations among individual variable were analyzed using the R package, “corrplot”.Citation55
Simultaneous fitting of antibody PK using full PBPK model
The relationship between and Heparin_RT was found to be best described by the following formula:
where ,
, and
are coefficients, and
is the exponent. This equation was incorporated into the PBPK model, and
,
,
, and
were estimated by simultaneously fitting PK data of all the selected 53 mAbs in the training set.
Estimation and simulation
Both estimation and simulation were carried out in R using the package, “Ubiquity”. Maximum likelihood estimates of and
, or
,
,
, and
were obtained assuming a proportional error variance model. The %PE was calculated to quantitatively assess model predictability as follows:
Where and
are model-predicted and observed plasma AUC from time 0 to the last sampling time point calculated using the PKNCA package.
Abbreviations
%PE | = | percent predictive error |
σv | = | vascular reflection coefficient |
σL | = | lymph reflection coefficient |
AC-SIN | = | Saffinity-capture self-interaction nanoparticle spectroscopy |
AUC | = | area under the curve |
BVP | = | baculovirus particle |
CDR | = | complementarity-determining regions |
CLup | = | pinocytosis uptake rate |
CV% | = | coefficient of variation |
ELISA | = | enzyme-linked immunosorbent assay |
Heparin_pB_buffer | = | heparin percent B buffer |
Heparin_RT | = | heparin chromatography retention time |
IVIVC | = | in vitro in vivo correlation |
FcRn | = | neonatal Fc receptor |
FcRn_RT | = | FcRn chromatography retention time |
HIC | = | hydrophobic interaction chromatography |
IACUC | = | Institutional Animal Care and Use Committee |
IgG | = | immunoglobulin G |
IV | = | intravenous |
KD | = | dissociation rate constant |
L | = | lymph flow |
LASSO | = | least absolute shrinkage selection operator regression |
mAb | = | monocloncal antibody |
MDCK | = | Madin-Darby canine kidney |
ML | = | machine learning |
NCA | = | non-compartmental analysis |
PBPK | = | physiologically-based pharmacokinetic modeling |
PCA | = | principal component analysis |
PEI | = | polyethylenimine |
pI | = | isoelectric point |
PK | = | pharmacokinetics |
PSR | = | polyspecificity reagent binding |
Q | = | plasma flow |
SC | = | subcutaneous |
SEFL | = | stable effector functionless |
t½ | = | half-life |
TMDD | = | target mediated drug disposition |
Supplemental Material
Download MS Word (3.5 MB)Acknowledgments
The authors acknowledge the Biotechnology Institute of Singapore for the production of antibodies, and the Syngene Amgen Research and Development Center (SARC), Benjamin Alba, Fuyi Chen, Michelle Hortter, and Ling Liu for the production of Fabs. The authors recognize Dhritiman Jana, Philip An, Evelyn Yang, and Heidi Jones for their support in registering protein lots, and Kevin Kalenian for running the FcRn AlphaScreen assay. The authors thank Isabel Figueroa for her technical review of the manuscript. The authors are grateful to John M. Harrold for his scientific input during discussions.
Disclosure statement
The authors declare the following competing financial interest(s): S.C.H., K.D.C., K.P.C., M.Y., R.P.e, M.S., R.P.c, M.L., M.M., and V.A.T. are full-time employees and shareholders of Amgen Inc. A.R.C., A.W.J., and R.S. are shareholders of Amgen Inc.
Supplementary material
Supplemental data for this article can be accessed online at https://doi.org/10.1080/19420862.2023.2263926
Additional information
Funding
References
- Mullard A. FDA approves 100th monoclonal antibody product. Nat Rev Drug Discov. 2021;20(7):491–15. doi:10.1038/d41573-021-00079-7.
- Thomas VA, Balthasar JP. Understanding inter-individual variability in monoclonal antibody disposition. Antibodies (Basel). 2019;8(4):56. doi:10.3390/antib8040056.
- Xu Y, Roach W, Sun T, Jain T, Prinz B, Yu T-Y, Torrey J, Thomas J, Bobrowicz P, Vásquez M, et al. Addressing polyspecificity of antibodies selected from an in vitro yeast presentation system: a FACS-based, high-throughput selection and analytical tool. Protein Eng Des Sel. 2013;26(10):663–70. doi:10.1093/protein/gzt047.
- Russell WMS, Burch RL. The principles of humane experimental technique. Methuen; 1959. https://norecopa.no/textbase/the-principles-of-humane-experimental-technique
- Han JJ. FDA modernization act 2.0 allows for alternatives to animal testing. Artif Organs. 2023;47(3):449–50. doi:10.1111/aor.14503.
- Igawa T, Tsunoda H, Tachibana T, Maeda A, Mimoto F, Moriyama C, Nanami M, Sekimori Y, Nabuchi Y, Aso Y, et al. Reduced elimination of IgG antibodies by engineering the variable region. Protein Eng Des Sel. 2010;23(5):385–92. doi:10.1093/protein/gzq009.
- Li B, Tesar D, Boswell CA, Cahaya HS, Wong A, Zhang J, Meng YG, Eigenbrot C, Pantua H, Diao J, et al. Framework selection can influence pharmacokinetics of a humanized therapeutic antibody through differences in molecule charge. MAbs. 2014;6(5):1255–64. doi:10.4161/mabs.29809.
- Boswell CA, Tesar DB, Mukhyala K, Theil F-P, Fielder PJ, Khawli LA. Effects of charge on antibody tissue distribution and pharmacokinetics. Bioconjug Chem. 2010;21(12):2153–63. doi:10.1021/bc100261d.
- Bumbaca Yadav D, Sharma VK, Boswell CA, Hotzel I, Tesar D, Shang Y, Ying Y, Fischer SK, Grogan JL, Chiang EY, et al. Evaluating the use of antibody variable region (Fv) charge as a risk assessment tool for predicting typical cynomolgus Monkey pharmacokinetics. J Biol Chem. 2015;290(50):29732–41. doi:10.1074/jbc.M115.692434.
- Schoch A, Kettenberger H, Mundigl O, Winter G, Engert J, Heinrich J, Emrich T. Charge-mediated influence of the antibody variable domain on FcRn-dependent pharmacokinetics. Proc Natl Acad Sci U S A. 2015;112(19):5997–6002. doi:10.1073/pnas.1408766112.
- Datta-Mannan A, Thangaraju A, Leung D, Tang Y, Witcher DR, Lu J, Wroblewski VJ. Balancing charge in the complementarity-determining regions of humanized mAbs without affecting pI reduces non-specific binding and improves the pharmacokinetics. MAbs. 2015;7(3):483–93. doi:10.1080/19420862.2015.1016696.
- Datta-Mannan A, Lu J, Witcher DR, Leung D, Tang Y, Wroblewski VJ. The interplay of non-specific binding, target-mediated clearance and FcRn interactions on the pharmacokinetics of humanized antibodies. MAbs. 2015;7(6):1084–93. doi:10.1080/19420862.2015.1075109.
- Jain T, Sun TW, Durand S, Hall A, Houston NR, Nett JH, Sharkey B, Bobrowicz B, Caffry I, Yu Y, et al. Biophysical properties of the clinical-stage antibody landscape. Proc Natl Acad Sci U S A. 2017;114(5):944–49. doi:10.1073/pnas.1616408114.
- Hötzel I, Theil FP, Bernstein LJ, Prabhu S, Deng R, Quintana L, Lutman J, Sibia R, Chan P, Bumbaca D, et al. A strategy for risk mitigation of antibodies with fast clearance. MAbs. 2012;4(6):753–60. doi:10.4161/mabs.22189.
- Sharma VK, Patapoff TW, Kabakoff B, Pai S, Hilario E, Zhang B, Li C, Borisov O, Kelley RF, Chorny I, et al. In silico selection of therapeutic antibodies for development: viscosity, clearance, and chemical stability. Proc Natl Acad Sci U S A. 2014;111(52):18601–06. doi:10.1073/pnas.1421779112.
- García CD, Hadley DJ, Wilson WW, Henry CS. Measuring protein interactions by microchip self-interaction chromatography. Biotechnol Prog. 2003;19(3):1006–10. doi:10.1021/bp025788z.
- Tessier PM, Sandler SI, Lenhoff AM. Direct measurement of protein osmotic second virial cross coefficients by cross-interaction chromatography. Protein Sci. 2004;13(5):1379–90. doi:10.1110/ps.03419204.
- Ahamed T, Ottens M, van Dedem GW, van der Wielen LA. Design of self-interaction chromatography as an analytical tool for predicting protein phase behavior. J Chromatogr A. 2005;1089(1–2):111–24. doi:10.1016/j.chroma.2005.06.065.
- Johnson DH, Parupudi A, Wilson WW, DeLucas LJ. High-throughput self-interaction chromatography: applications in protein formulation prediction. Pharm Res. 2008;26(2):296. doi:10.1007/s11095-008-9737-6.
- Le Brun V, Friess W, Bassarab S, Mühlau S, Garidel P. A critical evaluation of self-interaction chromatography as a predictive tool for the assessment of protein–protein interactions in protein formulation development: a case study of a therapeutic monoclonal antibody. Eur J Pharm Biopharm. 2010;75(1):16–25. doi:10.1016/j.ejpb.2010.01.009.
- Liu Y, Caffry I, Wu J, Geng SB, Jain T, Sun T, Reid F, Cao Y, Estep P, Yu Y, et al. High-throughput screening for developability during early-stage antibody discovery using self-interaction nanoparticle spectroscopy. MAbs. 2014;6(2):483–92. doi:10.4161/mabs.27431.
- Bengali AN, Tessier PM. Biospecific protein immobilization for rapid analysis of weak protein interactions using self-interaction nanoparticle spectroscopy. Biotechnol Bioeng. 2009;104(2):240–50. doi:10.1002/bit.22392.
- Jacobs SA, Wu S-J, Feng Y, Bethea D, O’Neil KT. Cross-interaction chromatography: a rapid method to identify highly soluble monoclonal antibody candidates. Pharm Res. 2010;27(1):65. doi:10.1007/s11095-009-0007-z.
- Schlothauer T, Rueger P, Stracke JO, Hertenberger H, Fingas F, Kling L, Emrich T, Drabner G, Seeber S, Auer J, et al. Analytical FcRn affinity chromatography for functional characterization of monoclonal antibodies. MAbs. 2013;5(4):576–86. doi:10.4161/mabs.24981.
- Cymer F, Schlothauer T, Knaupp A, Beck H. Evaluation of an FcRn affinity chromatographic method for IgG1-type antibodies and evaluation of IgG variants. Bioanalysis. 2017;9(17):1305–17. doi:10.4155/bio-2017-0109.
- Wu Q, Lee HY, Wong PY, Jiang G, Gazzano-Santoro H. Development and applications of AlphaScreen-based FcRn binding assay to characterize monoclonal antibodies. J Immunol Methods. 2015;420:31–37. doi:10.1016/j.jim.2015.03.012.
- Kraft TE, Richter WF, Emrich T, Knaupp A, Schuster M, Wolfert A, Kettenberger H. Heparin chromatography as an in vitro predictor for antibody clearance rate through pinocytosis. MAbs. 2020;12(1):1683432. doi:10.1080/19420862.2019.1683432.
- Conner KP, Devanaboyina SC, Thomas VA, Rock DA. The biodistribution of therapeutic proteins: mechanism, implications for pharmacokinetics, and methods of evaluation. Pharmacology & Therapeutics. 2020;212:107574. doi:10.1016/j.pharmthera.2020.107574.
- Liu L, Jacobsen FW, Everds N, Zhuang Y, Yu YB, Li N, Clark D, Nguyen MP, Fort M, Narayanan P, et al. Biological characterization of a Stable Effector Functionless (SEFL) monoclonal antibody scaffold in vitro. J Biol Chem. 2017;292(5):1876–83. doi:10.1074/jbc.M116.748707.
- Kelly RL, Sun T, Jain T, Caffry I, Yu Y, Cao Y, Lynaugh H, Brown M, Vasquez M, Wittrup KD, et al. High throughput cross-interaction measures for human IgG1 antibodies correlate with clearance rates in mice. MAbs. 2015;7(4):770–77. doi:10.1080/19420862.2015.1043503.
- Jones HM, Zhang Z, Jasper P, Luo H, Avery LB, King LE, Neubert H, Barton HA, Betts AM, Webster R. A physiologically-based pharmacokinetic model for the prediction of monoclonal antibody pharmacokinetics from in vitro data. CPT Pharmacometrics Syst Pharmacol. 2019;8(10):738–47. doi:10.1002/psp4.12461.
- Betts A, Keunecke A, van Steeg TJ, van der Graaf PH, Avery LB, Jones H, Berkhout J, van Steeg TJ, van der Graaf PH. Linear pharmacokinetic parameters for monoclonal antibodies are similar within a species and across different pharmacological targets: a comparison between human, cynomolgus monkey and hFcrn Tg32 transgenic mouse using a population-modeling approach. MAbs. 2018;10(5):751–64. doi:10.1080/19420862.2018.1462429.
- Avery LB, Wade J, Wang M, Tam A, King A, Piche-Nicholas N, Kavosi MS, Penn S, Cirelli D, Kurz JC, et al. Establishing in vitro in vivo correlations to screen monoclonal antibodies for physicochemical properties related to favorable human pharmacokinetics. MAbs. 2018;10(2):244–55. doi:10.1080/19420862.2017.1417718.
- Dostalek M, Prueksaritanont T, Kelley RF. Pharmacokinetic de-risking tools for selection of monoclonal antibody lead candidates. MAbs. 2017;9(5):756–66. doi:10.1080/19420862.2017.1323160.
- Kelly RL, Yu Y, Sun T, Caffry I, Lynaugh H, Brown M, Jain T, Xu Y, Wittrup KD. Target-independent variable region mediated effects on antibody clearance can be FcRn independent. MAbs. 2016;8(7):1269–75. doi:10.1080/19420862.2016.1208330.
- Shah DK, Betts AM. Towards a platform PBPK model to characterize the plasma and tissue disposition of monoclonal antibodies in preclinical species and human. J Pharmacokinet Pharmacodyn. 2012;39(1):67–86. doi:10.1007/s10928-011-9232-2.
- Yao Y-D, Sun T-M, Huang S-Y, Dou S, Lin L, Chen J-N, Ruan J-B, Mao C-Q, Yu F-Y, Zeng M-S, et al. Targeted delivery of PLK1-siRNA by ScFv suppresses Her2+ breast cancer growth and metastasis. Sci Transl Med. 2012;4(130):130ra148. doi:10.1126/scitranslmed.3003601.
- Bumbaca D, Wong A, Drake E, Reyes AE 2nd, Lin BC, Stephan JP, Desnoyers L, Shen BQ, Dennis MS. Highly specific off-target binding identified and eliminated during the humanization of an antibody against FGF receptor 4. MAbs. 2011;3(4):376–86. doi:10.4161/mabs.3.4.15786.
- Liu S, Verma A, Kettenberger H, Richter WF, Shah DK. Effect of variable domain charge on in vitro and in vivo disposition of monoclonal antibodies. MAbs. 2021;13(1):1993769. doi:10.1080/19420862.2021.1993769.
- Grinshpun B, Thorsteinson N, Pereira JN, Rippmann F, Nannemann D, Sood VD, Fomekong Nanfack Y. Identifying biophysical assays and in silico properties that enrich for slow clearance in clinical-stage therapeutic antibodies. MAbs. 2021;13(1):1932230. doi:10.1080/19420862.2021.1932230.
- Souders CA, Nelson SC, Wang Y, Crowley AR, Klempner MS, Thomas W Jr. A novel in vitro assay to predict neonatal Fc receptor-mediated human IgG half-life. MAbs. 2015;7(5):912–21. doi:10.1080/19420862.2015.1054585.
- Chung S, Nguyen V, Lin YL, Lafrance-Vanasse J, Scales SJ, Lin K, Deng R, Williams K, Sperinde G, Li JJ, et al. An in vitro FcRn- dependent transcytosis assay as a screening tool for predictive assessment of nonspecific clearance of antibody therapeutics in humans. MAbs. 2019;11(5):942–55. doi:10.1080/19420862.2019.1605270.
- Goulet DR, Watson MJ, Tam SH, Zwolak A, Chiu ML, Atkins WM, Nath A. Toward a combinatorial approach for the prediction of IgG half-life and clearance. Drug Metab Dispos. 2018;46(12):1900–07. doi:10.1124/dmd.118.081893.
- Hu S, D’Argenio DZ. Predicting monoclonal antibody pharmacokinetics following subcutaneous administration via whole-body physiologically-based modeling. J Pharmacokinet Pharmacodyn. 2020;47(5):385–409. doi:10.1007/s10928-020-09691-3.
- Hu S, Datta-Mannan A, D’Argenio DZ. Physiologically based modeling to predict monoclonal antibody pharmacokinetics in humans from in vitro physiochemical properties. MAbs. 2022;14(1):2056944. doi:10.1080/19420862.2022.2056944.
- Avery LB, Wang M, Kavosi MS, Joyce A, Kurz JC, Fan YY, Dowty ME, Zhang M, Zhang Y, Cheng A, et al. Utility of a human FcRn transgenic mouse model in drug discovery for early assessment and prediction of human pharmacokinetics of monoclonal antibodies. MAbs. 2016;8(6):1064–78. doi:10.1080/19420862.2016.1193660.
- Raybould MIJ, Marks C, Krawczyk K, Taddese B, Nowak J, Lewis AP, Bujotzek A, Shi J, Deane CM. Five computational developability guidelines for therapeutic antibody profiling. Proc Natl Acad Sci U S A. 2019;116(10):4025–30. doi:10.1073/pnas.1810576116.
- Wang W, Lu P, Fang Y, Hamuro L, Pittman T, Carr B, Hochman J, Prueksaritanont T. Monoclonal antibodies with identical Fc sequences can bind to FcRn differentially with pharmacokinetic consequences. Drug Metab Dispos. 2011;39(9):1469–77. doi:10.1124/dmd.111.039453.
- Datta-Mannan A, Brown R, Key S, Cain P, Feng Y. Pharmacokinetic developability and disposition profiles of bispecific antibodies: a case study with two molecules. Antibodies (Basel). 2021;11(1):11. doi:10.3390/antib11010002.
- Starr CG, Tessier PM. Selecting and engineering monoclonal antibodies with drug-like specificity. Curr Opin Biotechnol. 2019;60:119–27. doi:10.1016/j.copbio.2019.01.008.
- Mock M, Jacobitz AW, Langmead CJ, Sudom A, Yoo D, Humphreys SC, Alday M, Alekseychyk L, Angell N, Bi V, et al. Development of in silico models to predict viscosity and mouse clearance using a comprehensive analytical data set collected on 83 scaffold-consistent monoclonal antibodies. MAbs. 2023;15:2256745. doi:10.1080/19420862.2023.2256745.
- Datta-Mannan A, Croy JE, Schirtzinger L, Torgerson S, Breyer M, Wroblewski VJ. Aberrant bispecific antibody pharmacokinetics linked to liver sinusoidal endothelium clearance mechanism in cynomolgus monkeys. MAbs. 2016;8(5):969–82. doi:10.1080/19420862.2016.1178435.
- Zhou J, Mateos F, Ober RJ, Ward ES. Conferring the binding properties of the mouse MHC class I-related receptor, FcRn, onto the human ortholog by sequential rounds of site-directed mutagenesis. J Mol Biol. 2005;345(5):1071–81. doi:10.1016/j.jmb.2004.11.014.
- Denney W, Duvvuri S, Buckeridge C. Simple, automatic noncompartmental analysis: the PKNCA R package. J Pharmacokinet Pharmacodyn. 2015;42:S65–S65.
- Wei T, Simko V. R package “corrplot”: visualization of a correlation matrix (version 0.84). Vienna; 2017.