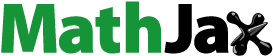
ABSTRACT
Dryland river waterholes provide critical habitat and serve as refugia for aquatic animals during droughts, but the quality of these waterholes can often be severely compromised by hypoxic conditions that can lead to mass fish kills and loss of biodiversity. To assist river management, we developed a waterhole-scale ecohydrology model representing thermal stratification and dissolved oxygen regimes during prolonged drought periods in northern Murray-Darling Basin dryland rivers in Queensland, Australia. Model development focused around 6 typical waterholes in these rivers that were shallow (<5 m deep), highly turbid, and stratified with low dissolved oxygen. The model simulations utilised regional climate corrected for local factors such as diurnal vegetation shading and wind sheltering and successfully reproduced the prolonged stratification and hypoxia measured during drought conditions. The simulations highlight the distinct local climate each waterhole experiences due to the combined effects of river morphology and canopy cover that provide various degrees of solar shading and wind sheltering. The model can serve as a tool to inform water management decisions and climate adaptation strategies. Example scenarios demonstrate that (1) even where the canopy shading effect was small (5% at one site), further loss of riparian vegetation could increase temperature by 2–4 °C in warmer months with prolonged stratification; and (2) under an example RCP 8.5 climate change scenario, water temperature is likely to increase 2–10 °C, and oxygen saturation will decrease by 10% to 20% in the middle layers for most of the no-flow period by 2080–2099.
Introduction
Drylands make up >50% the world’s land area (Tooth Citation2000). Rivers that flow through these regions often have intermittent, highly variable, and unpredictable flow linked to irregular rainfall events (Acuña et al. Citation2014). Many dryland rivers cease to flow for extended periods, and with most of the river channels dry, the river networks exist as a series of disconnected waterholes for much of the time (Sheldon et al. Citation2010). During droughts, waterholes provide critical aquatic habitats in these landscapes and serve as refugia for aquatic biota until the return of flow, increasing their survival probability and the resilience of their regional populations (Bunn et al. Citation2006, Sheldon et al. Citation2010, Marshall et al. Citation2021). However, as water levels continue to recede and waterholes contract during prolonged drought, the quality of waterholes as drought refugia can become limited, in part through the ongoing deterioration of water quality, particularly from increasing hypoxia/anoxia (Preite and Pearson Citation2017, Vertessy et al. Citation2019, Marshall et al. Citation2021).
Hypoxia and anoxia are common phenomena in rivers and lakes worldwide (Agostinho et al. Citation2021), and the frequency of occurrence is increasing globally, accelerated by human activities (Diaz Citation2001). Mass fish kills resulting from hypoxia events are also on the rise (Pollock et al. Citation2007). The impacts of anoxia on fish in dryland river waterholes was graphically demonstrated by the well-publicised mass fish kills in Australia’s Darling River during summer 2018–2019 (Vertessy et al. Citation2019) and repeated in autumn 2023. The combined influences of drought, extreme heatwaves, waterhole stratification, algal blooms, water resource development, and local weather impacts have possibly led to rapid and prolonged anoxia, which suffocated and killed the fish confined in isolated waterholes (Sheldon et al. Citation2022). However, little is known about the stratification and oxygen dynamics in these systems during no-flow periods, and the ability to better predict and prevent fish kills from prolonged hypoxia/anoxia events was identified as a priority (Sheldon et al. Citation2022). Further, climate change threatens to increase the intensity and duration of extreme events like droughts and heatwaves on the global scale (IPCC Citation2021), as well as in the Murray-Darling Basin in Australia (Kirono et al. Citation2020, MDBA Citation2019), yet the extent to which these changes will impact the water quality of river waterholes and their role in sustaining aquatic biota remains unclear.
Ecohydrological models have been useful tools for assisting river management, such as informing water planning decisions of water allocation and environmental flow provisions (McGregor et al. Citation2018) and assessing the hypoxia risk related to carp biomass decomposition (Brookes and Hipsey Citation2019). However, at present, modelling the quality aspects of dryland river waterholes for managers and policy makers to use as a template to identify where and when risks arise and plan effective short- and long-term strategies to support the function of waterholes as effective drought refugia is limited (Stocks et al. Citation2021, Sheldon et al. Citation2022). While models are being used to manage blackwater events (Mosley et al. Citation2021) and algal bloom risks (Hamilton et al. Citation2007, Davidson et al. Citation2016, Kim et al. Citation2017), the extension of river models to capture the subtle dynamics that control water quality when flow ceases is questionable; in this case, river waterholes become shallow lentic environments with extended periods of low mixing and the potential for persistent stratification (Bormans and Webster Citation1997, Wallace et al. Citation2017).
Given the critical ecological importance of dryland river waterholes as drought refugia, the need is urgent to investigate and predict how their thermal and oxygen dynamics govern their quality as habitats for aquatic biota at a resolution relevant to management and that can be used to design and plan for both emergency interventions and longer-term restoration strategies (Biswas and Mosley Citation2019, Stewart et al. Citation2020). Important attributes include the subtle effects of controls on the water balance and quality, such as wind-sheltering effects on evaporation, vertical temperature stratification, oxygen variability, turbidity, and ground-water seepage (Wallace et al. Citation2017). In particular, the role that wind and light attenuation play in shaping diurnal and longer-term patterns of thermal stratification and hypoxia (Wallace et al. Citation2017) is critical to establish the functional requirements for waterholes to act as effective drought refugia for aquatic species (Rayner et al. Citation2009).
Shallow waterbodies are not typically thought to be associated with stratification because small amounts of wind or evaporative cooling can drive water column turnover (Padisak and Reynolds Citation2003). However, a reduction in wind-induced mixing, such as from dense macrophyte growth in shallow lakes that dissipates turbulent kinetic energy and absorbs heat, can induce thermal stratification (Vilas et al. Citation2018). Similarly, river waterholes are subject to substantial wind-sheltering and shading effects from the riparian vegetation on both banks of rivers (), creating a relatively stable environment for the development of stratification during no-flow periods. Further, because water clarity can strongly influence the progress of thermal stratification via vertical light and heat attenuation (Heiskanen et al. Citation2015), river waterholes have a higher stratification potential from the high turbidity that persists through the no-flow periods (Sheldon and Fellows Citation2010). More recent studies have demonstrated that shallow lakes can have different mixing regimes (rarely, intermittently, or often mixed), primarily regulated by surface area and depth (Holgerson et al. Citation2022). Persistent stratification impedes vertical mixing of gases such as oxygen in the water column, promoting anoxic conditions at the bottom because oxygen consumed by or near the sediment cannot be replenished (Branco et al. Citation2005). Therefore, considering the effects of local microclimatic conditions on water stability is critical in river waterholes, which can be significantly different from the surrounding open landscape.
Figure 1. Reilly’s Weir study site showing dense vegetation surrounding the waterhole in the Murray-Darling Basin, Australia (photo credit: James Fawcett, Queensland Government, Department of Regional Development, Manufacturing and Water).

A wide range of lake stratification models have been applied to shallow lakes (e.g., Condie and Webster Citation2001, Sun et al. Citation2020), which generally experience strong daytime stratification and mixing at night (Losordo and Piedrahita Citation1991, Condie and Webster Citation2001, Herb and Stefan Citation2004, Branco and Torgersen Citation2009, Andersen et al. Citation2017, Vilas et al. Citation2018, Martinsen et al. Citation2019). Three-dimensional models have also been developed showing the importance of diurnal changes in oxygen metabolism in response to in situ productivity and oxygen demand (Liu et al. Citation2020). Aside from these applications, an approach is yet to be developed to model river waterholes, which have a different stratification phenology relative to other lotic environments.
The objective of this study was to develop a flexible and adaptable river waterhole ecohydrology model that can reproduce the strong stratification and hypoxic conditions in these shallow dryland river waterholes during drought periods. More specifically, we aimed to use the General Lake Model (GLM), linked with the Aquatic Ecodynamics (AED) water quality model, as the base platform applied to 6 waterholes in dryland rivers in Australia. The model uses high resolution data (hourly or subhourly), taking into account the diurnal shading pattern and sheltering effects of riparian vegetation and the highly turbid environment to accurately predict temperature and dissolved oxygen (DO) stratification. Applications of the model are discussed, such as how change in canopy shading and future climate may affect waterhole stratification dynamics and the implications for management.
Materials and methods
Study sites and field data collection
We focused model development around 6 waterholes in the northern Murray-Darling Basin rivers, which have recently been intensively monitored by the Queensland Government. The waterholes, Kooroon, Kurmala (Moonie River), Brenda Weirpool, Weribone, Reilly’s Weir, and Trafalgar (Condamine-Balonne River), are located ∼400 km inland from Brisbane, Queensland (). All waterholes are relatively shallow (<5 m deep), highly turbid, and surrounded by riparian vegetation (), typical examples of waterholes in the region. Bathymetric data for Kooroon, Kurmala, and Brenda Weirpool were provided by the Department of Environment and Science ().
Figure 2. Location of the 6 study waterholes in the Murray-Darling Basin, their nearby flow gauges, and Australian Bureau of Meteorology (BoM) weather stations.

Figure 3. Waterhole bathymetry for (a) Kooroon and (b) Brenda and (c) hypsographic curves for all waterholes. The hypsographic curves show both the survey data with offset (lines) and model input points in the General Lake Model (GLM) (*). Note that because no bathymetric data were available for Reilly’s Weir, Trafalgar, or Weribone, the same hypsographic curve was assumed and shown as Reillys_GLM (yellow points). Detailed descriptions are in the model setup.

Water temperature and DO data were recorded every 10 min at various depths in each waterhole from mid-2019 to late 2020 (PME T-chain RS 232 sensor with Campbell Scientific CR300-RF412 datalogger [Logan, UT, USA] at Kooroon, Kurmala, and Brenda; PME MiniDOT [Precision Measurement Engineering, Inc., Vista, CA, USA] at Weribone, Reilly’s Weir, and Trafalgar), capturing the escalation and then the breaking of a severe, regional, hydrological drought (Marshall et al. Citation2021; ; GPS coordinates in Supplemental Table S1). Water depths (barometric compensated pressure) were recorded at 10 min intervals at Kooroon, Kurmala, and Brenda (PME T-chain RS 232 sensor with Campbell Scientific CR300-RF412 datalogger) and 30 min intervals at Weribone, Reilly’s Weir, and Trafalgar (PME TD Diver). For Kooroon, Kurmala, and Brenda, logger chains were fixed to a floating station, and node depths were indicated as distance from the surface (). For Weribone, Reilly’s Weir, and Trafalgar, chains were fixed to the substrate, and node depths were indicated as distance from the substrate. As a result, some logger nodes may have been logging in sediment or above the water surface when water levels receded. All such data points were removed before analysis.
Table 1. Dissolved oxygen (DO) and water temperature data availability at 6 Murray-Darling Basin waterholes in Australia (date is month/year).
All 6 waterholes were strongly stratified prior to receiving major inflows in February 2020, with Weribone experiencing the steepest temperature gradient of up to 18 °C between the top and bottom layers (). Stratification persisted throughout the no-flow period with no nighttime destratification in any of the waterholes except Reilly’s Weir and Trafalgar. Thermal stratification was eliminated or significantly dampened during the major flood event during February–March 2020, which ended the drought. DO concentrations were low in all 6 waterholes during the no-flow period, with prolonged hypoxic conditions below the thermocline observed at Kooroon, Kurmala, and Weribone. Kurmala was the most hypoxic, with <4 mg/L DO (50% air saturation) at just 30 cm below surface.
Model description
Temperature
The hydrodynamics of the waterholes were modelled with the 1D GLM, an open-source model code designed to simulate the hydrodynamics of lakes, reservoirs, and wetlands (Hipsey et al. Citation2019). GLM computes vertical profiles of temperature, salinity, and density by accounting for the effect of inflows and outflows, mixing, and surface heating and cooling (a). GLM incorporates a flexible Lagrangian layer structure that allows layers to change thickness by contracting and expanding in response to inflows, outflows, mixing, and surface mass fluxes. Layer thicknesses are adjusted by the model to sufficiently resolve the vertical density gradient. Unlike the fixed grid design in which mixing algorithms are typically based on vertical velocities, numerical diffusion of the thermocline is limited, particularly suiting GLM to investigations where stratification is persistent and for coupling with biogeochemical models to explore the role of stratification and vertical mixing on the dynamics of aquatic ecosystem. Examples of GLM applications include coupling GLM with the AED water quality model (Hipsey Citation2022) to simulate long-term thermal and anoxia dynamics in eutrophic Lake Mendota, USA (Ladwig et al. Citation2021), and using GLM to predict mountain lake thermal regimes in future climates (Christianson et al. Citation2020).
Oxygen
The AED water quality model (Hipsey Citation2022) was coupled to simulate DO dynamics. The AED modelling library is an open-source community-driven library of model components for simulating water quality and aquatic ecosystem dynamics as well as terrestrial and aquatic habitats. The AED library consists of numerous modules configured according to ecosystem conceptualisations. This application adopts the oxygen module, which simulates DO dynamics accounting for atmospheric exchange and sediment oxygen demand (SOD; b). AED has been applied to simulate oxygen dynamics in various settings, including investigating hydrodynamic controls on oxygen regimes in the Yarra River estuary in Victoria, Australia (Bruce et al. Citation2014), and assessing the effectiveness of oxygenation operation regimes in the Swan-Canning estuary in Western Australia (Huang et al. Citation2018).
The instantaneous flux of oxygen at the air–water interface, (mmol O2/m2/s), was calculated based on the oxygen gradient between the air and water and the gas transfer, or piston velocity,
(m/s):
(1)
(1) where
is the oxygen concentration in the surface waters near the interface and
is the concentration of oxygen in the air phase near the interface (mmol O2/m3). The gas exchange velocity method proposed by Wanninkhof (Citation1992) was adopted in this study. A positive flux represents input of oxygen from the atmosphere to the water.
One of the key oxygen sinks in the waterhole environment is the SOD that, in conjunction with water stratification, creates a highly hypoxic environment at the bottom of the waterhole. The SOD can be estimated in the model as a function of the overlying water temperature and DO concentration:
(2)
(2) where
is the maximum oxygen flux (mmol O2/m2/d) across the sediment–water interface at 20 °C, and
is the Arrhenius temperature multiplier for sediment oxygen flux;
is the oxygen limitation, computed based on:
(3)
(3) where
(mmol O2/m3) is the half-saturation constant controlling the SOD. Further details of the AED oxygen module are described in Hipsey (Citation2022).
Model setup
GLM simulations were forced with a set of meteorological datasets, including air temperature, solar radiation, humidity, and wind speed, to predict surface thermodynamics and mixing. These meteorological datasets were first obtained from regional weather stations and then localized to consider the sheltering effects from the littoral vegetation. In addition, bathymetric and hydrological data were included in the model where available. A workflow diagram for the model setup () and the details of the setup of each component are described.
Figure 6. Workflow diagram for the model input (GLM = Generalized Lake Model; BoM = Australian Bureau of Meteorology; AED = Aquatic Ecodynamics Model; DO = dissolved oxygen).

Weather localisation
Because river waterholes are subject to substantial wind-sheltering and shading effects from riparian vegetation, which favours a relatively steady environment for stratification, the meteorological data obtained from nearby Australian Bureau of Meteorology (BoM) stations were localised before being applied to the GLM model (). Meteorological data from the BoM St. George Airport station (043109) and Miles Constance St. (042112) were used as reference conditions for hourly air temperature, relative humidity, and precipitation (, ; example of weather record in Supplemental Fig. S1). Because regional weather stations are usually installed in exposed conditions with wind speed measured 10 m above ground, which can be significantly different from the conditions experienced at the waterhole surface, wind speed data obtained from these stations were adjusted by using a shelter index for small waterbodies fringed by natural vegetation, Sw, estimated as 0.53–0.71 (where 0 represents no sheltering and 1 complete sheltering; Hipsey and Sivapalan Citation2003). These values were used as a reference when applying wind scaling factor (1 − Sw) to the regional weather data in the model.
Table 2. Australian Bureau of Meteorology weather station datasets used as model input for the 6 Murray-Darling Basin waterholes. * indicates data were adjusted for local conditions. AP = airport.
Because considerable spatial variability exists in shortwave radiation due to changes in cloud cover, several BoM stations close to each waterhole were used to quantify shortwave radiation at waterhole sites (, ). Shortwave radiation was only available at daily timescales and thus required disaggregation into hourly intervals before incorporating into the model using the Bird Clear Sky Model (Bird and Hulstrom Citation1981) for each waterhole based on its latitude, longitude, and zenith angle. The calculated daily total theoretical clear sky radiation was compared with measured daily solar radiation from nearby BoM stations for each site to generate an adjustment factor for each day, which was then applied to the disaggregated hourly clear sky radiation estimates for that day.
Another major factor affecting the amount of shortwave radiation reaching water surface is the shading effects created by the surrounding vegetation, which changes throughout the day depending on the sun’s position. The diurnal vegetation shading percentage was estimated based on changes in tree shadow length throughout the day due to changes of sun angle. The shadow length (L) at a particular time (t) was calculated by:
(4)
(4) where H is tree height, and
is the angle between sun and horizon in radians at time t.
An hourly factor (i.e., multiplier, ) was introduced to calculate the relative changes (ratio) of shadow length throughout the day, with a factor of 1 representing the shortest shadow length on that day (at midday; ). Because tree shadow length gradually increases towards dawn or dusk, the factor also gradually increases, and can be calculated by:
(5)
(5) where
is the shadow length at midday, and
is the factor at midday (= 1).
Table 3. Example of hourly canopy shade (S) during daylight hours at Brenda on 1 December 2019, derived from sun angle (a), tree shadow length (L, assuming a tree height of 10 m), and factor (F). * indicates a factor of 1 is assigned to 1200 h everyday; ^ indicates the canopy shade at 1200 h is estimated from satellite imagery.
The hourly canopy shade percentage can then be calculated based on
by:
(6)
(6) where
is the shade percentage at midday, visually estimated from satellite images (ESRI World Imagery at 1 m resolution, acquired in 2021) for each waterhole at locations where the loggers were installed. Canopy shade percentages were 5% at Kooroon and Weribone; 10% at Brenda, Reilly’s Weir, and Trafalgar; and 7.5% at Kurmala (see example of calculated hourly canopy shade at Brenda on 1 December 2019; ). The final hourly shortwave radiation input into the model was then calculated by multiplying the hourly canopy shade percentage. The actual tree height H can be assumed to be any number because it is eliminated during the calculation of
. However, several assumptions were made: (1) shadow length is a proxy for shade area, which means the surrounding trees/shrubs are assumed to be a regular rounded shape as opposed to a tall tree form with a bare trunk; and (2) vegetation density is similar on both banks. These assumptions are generally realistic for our study sites based on aerial images and onsite photographs. Because of the sinuous shape of our waterholes, the orientation of the river channels is heterogeneous and not considered to significantly impact this overall shading pattern at whole waterhole scales. In addition, a study by Garner et al. (Citation2017) suggested that channel orientation would have limited the impact on net solar radiation received by the water when canopy cover overhanging the stream is <30% (as in this study) or >70%.
Hourly cloud cover was calculated from the difference between field solar radiation and theoretical clear sky radiation. Because cloud cover could not be calculated during the night, we assumed it to be the same as that of the last daylight hour on that day.
Hydrology
Flow data for each waterhole was approximated from data obtained from the Queensland Water Monitoring Information Portal (https://water-monitoring.information.qld.gov.au/) for the nearest flow monitoring station (; flow gauge names in Supplemental Table S1). Flow gauges were selected based on absolute distance from study sites regardless of their position upstream or downstream of the sites because options were limited. The flow gauge data from the nearby gauge (Moonie_Flinton 417205A) were used in Kooroon and Kurmala because simulations at these sites included periods of flow. The Moonie-Flinton gauge is located ∼20 km upstream of Kooroon but >50 km downstream of Kurmala. As a result, some discrepancies between the modelled and observed timing and magnitude of water depth change were expected at these 2 sites. For other sites, we only considered drought periods for the modelling investigation, and therefore no flow was included. In this situation, the water balance was dominated by evaporation and seepage according to the parameters regulating these processes ().
Bathymetry
An offset was applied to the bathymetry for Kooroon, Kurmala, and Brenda in the GLM to remove a small portion at the bottom of the waterhole where areas were close to 0 below certain depths (c). The removed portion likely represents a small dent in the riverbed that accounts for a negligible volume of water but does not form part of the general morphology of the waterhole. The depth offsets applied were 0.7, 1, and 0.4 m for Kooroon, Kurmala, and Brenda, respectively. The associated volume of water accounted for 0.015%, 0.072%, and 0.05% of the total volume of each waterhole, respectively, at their cease-to-flow depths. For Reilly’s Weir, Trafalgar, and Weribone, the overall width and length of each waterhole were estimated from satellite images (ESRI World Imagery at 1 m resolution, acquired in 2021), and the same generic hypsographic curve was assumed because no specific bathymetry information was available for these sites (c).
In addition, the hypsographic curves were slightly extrapolated beyond the actual depth of the waterholes in GLM (c). This extra surface area around the waterhole acts as a pseudo catchment that collects rainfall runoff and drains to the waterhole when catchrain is set to TRUE in GLM (). However, in this study, this phenomenon is only relevant to Kooroon and Kurmala, where water depths were calibrated for a longer period that included the periods of rainfall ().
Table 4. Key parameters in General Lake Model (GLM) and Aquatic Ecodynamics (AED) models calibrated for Murray-Darling Basin study sites.
Table 5. Calibration and validation period for temperature, dissolved oxygen, and water depth at 6 Murray-Darling Basin waterholes (date is day/month/year).
Model calibration and validation
The models were calibrated against field temperature profiles and water depths in GLM to optimise stratification model performance before calibrating against the field DO profiles in AED. GLM can adjust the number and resolution of vertical layers within the pre-set minimal and maximal layer thickness. In our models, the minimum and maximum layer thickness were set to 0.05 and 0.2 m, respectively, compared to the thermistor chain vertical resolution of 0.2 m. The model was run at an hourly timestep and required <1 min of computing time for a 1 yr simulation. Correlation coefficients () and root mean square error (RMSE) between simulated and observed hourly data were calculated as model performance metrics. For temperature and oxygen, comparisons were made at each depth node at each time step. Peak flow period was excluded from error metrics calculation for validation period because flow data were insufficient (flow gauges located not in proximity) and the model was not calibrated for periods with flow. Details of model calibration are described in the following sections.
Water depth
Water depths were calibrated between late 2019 and mid/late 2020 for Kooroon and Kurmala, including periods with and without flow. For the remaining sites, water depths were calibrated for the no-flow period (). Parameters tested during this calibration include seepage rate and runoff threshold.
Temperature
All 6 waterholes were calibrated from the no-flow period between mid/late 2019 and early 2020, before the start of flow events, to optimise model performance under no-flow conditions when risk of stratification is high (). For waterholes with longer periods of data (Kooroon and Kurmala), models were also validated through to mid/late 2020, which included periods of flow. Parameters tested during this calibration include scaling factors for shortwave radiation, wind speed, air temperature, and relative humidity as well as light extinction coefficient and atmospheric stability configuration. The wind speed was manually adjusted by applying a scaling factor between 0.2 and 0.35 considering the riparian vegetation sheltering effects (with reference to the shelter index) to achieve a good fit between modelled and observed temperature profiles (). Light extinction coefficients, Kw, were calibrated with reference to photosynthetically active radiation (PAR) measurements at Kooroon and Kurmala for 2006 summer (Kooroon: 20.1/m in Jan and 30.1/m in Feb; Kurmala: 12.4/m in Jan and 16.7/m in Feb; Queensland Government, unpubl. data).
Oxygen
Oxygen models were calibrated for the same period as temperature models. SOD () was calibrated with reference to the range observed in various reaches in the same region (Condamine, Balonne, and Culgoa rivers, −70.4 to 4.8 mmol O2/m2/d; P. M. Davies, unpubl. data). Key parameter values for all models were reported (). For Kooroon, which has the most complete dataset, the model was also validated through mid/late 2020, which included periods of flow. However, because oxygen concentration data from inflow were missing, we assumed it to be the same as the oxygen concentration of the surface layer at Kooroon.
Example climate change scenario
To assess how waterhole stratification and hypoxia will change under climate change, we applied the model to Representative Concentration Pathway (RCP) 8.5 conditions at the Kooroon site as an example. Gridded climate projection data were acquired from The Long Paddock website (https://www.longpaddock.qld.gov.au/qld-future-climate/dashboard), which includes mean air temperature, relative humidity, surface wind speed, solar radiation, and precipitation from 1980 to 2099. The climate model outputs used in this study to illustrate the approach for waterhole risk assessment was ACCESS 1.0, and we chose the high RCP 8.5 to demonstrate the upper limit of potential changes.
Climate point data were extracted for Kooroon, and monthly mean percentage difference between the 20-year average of future (2080–2099) and current (2001–2020) was calculated for each climate variable, except wind speed, for which gridded data were unavailable. This percentage change in future climate was then applied to the current local climate at the waterhole to simulate temperature and oxygen concentrations. For wind speed, the seasonal absolute change projected for Moonie River basin was applied as an offset in our model ().
Table 6. Projected climate change in 2080–2099 relative to 2001–2020 at Kooroon under RCP 8.5 from the ACCESS 1.0 climate model output.
Results
Temperature
Changes in water depth during the no-flow period due to evaporation and seepage were generally well captured for all sites (). Overall, the model was able to accurately reproduce the range of temperatures observed at each waterhole, the onset of stratification and stratification strength, and the onset of mixing (; Supplemental Fig. S2–S6). On average, error in temperature predictions ranged from 0.85 to 2.24 °C during the no-flow period (), but in some instances model prediction was less accurate. At Reilly’s Weir, the model predicted stratified conditions around December 2019 when water depths reduced to 0.5 m, whereas it was well-mixed in the field. Among the 6 waterholes, Kurmala was particularly challenging to calibrate because the model tended to underpredict temperature throughout the depth profile in November but overpredict during mid- to late-December (Supplemental Fig. S3). In addition, stratification was more persistent in the field than predicted by the model. In the field, Kurmala never fully destratified between November and December 2019, with a minimum temperature difference between the top and bottom node of 0.74 °C, whereas the model predicted destratification (i.e., 0 °C difference) on 3 occasions. Overall, field data indicated that 97% of this period was stratified (i.e., with >1 °C difference between top and bottom node) compared to 85% modelled. However, satisfactory model performance metrics were achieved in most of the waterholes ().
Table 7. Correlation coefficients (r) and root mean square error (RMSE) between modelled and field data over calibration (calib) and/or validation (valid) periods at 6 Murray-Darling Basin waterholes. RMSE in m, °C, and mmol O2/m3 for water depth, temperature and oxygen, respectively.
The models also performed well at Kooroon and Kurmala, which included periods of flow at the end of the drought period ( for Kooroon; Supplemental Fig. S6), with an error of 2.33 and 2.45 °C, respectively (). The model successfully captured the magnitude of water depth change due to inflow and precipitation at both waterholes, although mismatches of the timing of the events occurred because of the distance between the modelling sites and the gauge stations. At Kooroon, the modelled first inflow (mid-Jan 2020) was ∼7 days ahead of that observed because the inflow data used were from 20 km upstream of the site. Temperature profiles were well represented in the model during the wetter period between February and August 2020, through to the recommencement of stratification in September–October (). At Kurmala, a ∼2-week delay occurred in the predicted first flow event (late-Jan to early Feb 2020) in the model because the flow gauge was >50 km downstream of Kurmala. Despite the mismatch in timings, the simulated temperature profiles were generally in agreement with field observations.
Oxygen
Overall, most models achieved good calibration results regarding the temporal and vertical profiles of DO (, Supplemental Fig. S7–S10, ). The oxygen model was able to simulate the range of DO concentrations observed in these waterholes and their vertical DO gradients through time. On average, error in DO predictions ranged from 7.08% to 19.29% over the no-flow period (). However, at Reilly’s Weir, the model under-predicted hypoxia development around November–December as water depth dropped below 0.5 m, which coincided with the mismatch between observed and modelled thermal stratification. Modelled oxygen levels were mostly >50% air saturation during this period except near the sediment, whereas field data indicated the entire water column frequently experienced oxygen levels <20% air saturation. At Brenda, the model tended to slightly under predict oxygen concentrations at the surface in October 2019 but over predict in late January 2020, when it was completely anoxic in the field (Supplemental Fig. S7). The models were also validated at Kooroon after the high flow period (), with an overall 14.2% DO error (excluding peak flow period; ).
Discussion
Model advantage and limitations
Although thermal stratification in shallow lakes and ponds has been well documented, these systems often stratify and destratify daily or remain stratified for only a short period (several days). Some extreme cases were found where stratification persisted for several weeks or even months in these small and shallow inland waterholes, each with unique thermal and DO patterns (). Nevertheless, the GLM was able to successfully capture stratification phenology and oxygen dynamics in all 6 waterholes. Adjusting regional BoM meteorological data to local conditions at each site, in particular solar radiation and wind speed, was crucial in resolving the heat balance and preventing discrepancies, highlighting the distinct local climate experienced by each waterhole due to the combined effects of river morphology and riparian canopy that provide various degrees of solar shading and wind sheltering. However, even after accounting for diurnal vegetation shading patterns, an adjustment factor of <1 for shortwave radiation (sw_factor; ) was still required at some sites, possibly because local factors affecting heat balance, such as shading from elevated riverbanks, were unaccounted for in this model (Webb and Zhang Citation1997).
Figure 7. Contour plot of observed and simulated water temperature (°C) at (a and b) Kooroon and (c and d) Reilly’s Weir during the no-flow period in 2019 (day/month). Note the different time axes between Kooroon and Reilly’s Weir. The black lines indicate water level. For ease of comparison, the top 0.3 m layer was removed in the Kooroon model result to match field data.

Figure 8. Contour plot of (a) observed and (b) simulated water temperature at Kooroon between 1 November 2019 and 31 October 2020 (day/month/year). The black lines indicate water level. For ease of comparison, the top 0.3 m layer was removed in the model result to match field data.

Figure 9. Contour plot of observed and simulated dissolved oxygen saturation (%) at (a and b) Kooroon and (c and d) Reilly’s Weir during the no-flow period in 2019 (day/month). The black lines indicate water level. For ease of comparison, the top 0.3 m layer was removed in the Kooroon model result to match field data.

Figure 10. Contour plot of (a) observed and (b) simulated dissolved oxygen at Kooroon between 1 November 2019 and 31 October 2020 (day/month/year). The black lines indicate water level. For ease of comparison, the top 0.3 m layer was removed in the model result to match field data.

Good model performance was achieved at all sites for both the thermal stratification and oxygen models by using a wind speed scaling factor between 0.2 and 0.35, which agreed with the reference wind-sheltering index (Hipsey and Sivapalan Citation2003). The calibrated oxygen demand () was also within the range observed in the Condamine-Balonne rivers (P. M. Davies, unpubl. data). However, while a good model fit was achieved by using the reference Kw at Kurmala, it was less effective for Kooroon. When applying the reference Kw for Kooroon (20.1–30.1/m), the model tended to cross the mixing threshold more readily than observed, especially on windy days. Applying a lower wind factor could compensate for this discrepancy in the stratification model but would subsequently underpredict DO near the surface. A Kw value of 10/m, considerably lower than the calculated value, was found to produce more satisfactory results, possibly because the reference values were derived from measurements taken nearly 20 years ago (early 2003), and turbidity, and thus light attenuation, may have changed during this time. This explanation also highlights the role of turbidity in stratification dynamics and the need for local measurements for model calibration (Hocking and Straškraba Citation1999, Liu et al. Citation2022).
Field data review and modelling work suggested bank shading and wind sheltering effects altered the local weather condition, subsequently affecting the vertical mixing of waterholes. However, local weather conditions were approximated from weather records at nearby weather stations, which were 70–170 km from study sites. Local measurements of key meteorological parameters such as shortwave radiation and wind speed at the surface of waterholes are therefore recommended to quantify the shading and sheltering effects accurately and improve model robustness.
Field observations and GLM models indicated high turbidity, high levels of light attenuation, and strong stratification at the surface. Shortwave radiation decayed quickly with water depth; however, the current monitoring system at Kooroon, Kurmala, and Brenda did not record the top 0.3 m layer, and consequently the models are not calibrated to this top layer. Similarly, at Reilly’s Weir, Weribone, and Trafalgar, data in the top 0.5 m layer were lost during the dry period because of the logger chain configuration. Key information may be lost not only for thermal stratification in the top layer but also for peak water temperatures near the surface, which could be an important niche and/or refuge for aquatic organisms under hypoxic conditions. Therefore, additional information in the top layers could be valuable in future model refinement, and a better understanding of temporal variability in turbidity driving light attenuation would improve model accuracy.
The main purpose of this modelling study was to capture stratification and oxygen dynamics during the drought period when waterholes are at high risk of developing hypoxia; therefore, models were only calibrated to a relatively short period (2–6 months). For longer-term simulations that include both the non-flowing and flowing periods, flow gauge data in the vicinity of each waterhole are needed.
Note that for the 3 waterholes without bathymetry data (Reilly’s Weir, Trafalgar, and Weribone), a generic lake morphometry was assumed considering only the overall length, width, and depth of each waterhole. The lake morphometry (the shape of its hypsographic curve) may affect how heat is transferred and distributed within the water column, especially for smaller waterbodies (Elo Citation2005). For example, waterbodies with a larger total volume stay stratified longer, and cooling is delayed by the large amount of heat absorbed; therefore; incorporating more accurate bathymetric data is recommended to further improve model reliability at these sites.
Finally, water depth was calibrated without distinguishing natural or anthropogenic causes, such as groundwater seepage or water abstraction by local residents, respectively. Previous studies of waterholes in the region have concluded that surface water–groundwater interactions were absent or limited, including for Kooroon, Kurmala, and Brenda waterholes (DSITI Citation2015), but not necessarily for all waterholes (Lobegeiger Citation2010). Future field surveys on the local flow regimes can help reduce the uncertainty in the modelling predictions of water balances.
Implications for management
The models developed in this study could be a valuable tool to inform waterhole management and planning decisions and climate adaptation strategies. For example, a comparison of water temperatures with and without the estimated canopy shade at Weribone suggested that although the estimated canopy shading effect was only 5% at midday, an increase in water temperature of ∼2–4 °C in warmer months (Nov–Dec) was predicted after removing the shade, along with longer duration of stratification (). This scenario suggests that restoring riparian vegetation, or in emergency situations the artificial shading of waterholes, could help reduce the risk to aquatic life caused by stratification and hypoxic conditions during extreme climate events (). Similarly, given the importance of local wind conditions in governing stratification, the feasibility of local solutions, such as baffles to focus wind energy over the surface of waterholes, could offer innovative management possibilities worthy of future consideration.
Figure 11. Water temperatures in simulations for 6 Murray-Darling Basin (Australia) waterholes are significantly lower (a) with canopy shade than (b) without canopy shade for 2019 (day/month).

Figure 12. Conceptual diagram showing the effects of (a) vegetation shading on waterhole stratification, and the effects of (b) artificial mixing on waterhole oxygen dynamics (GPP = gross primary production; DO = dissolved oxygen).

Extended periods of hypoxia and acute destratification events can lead to loss of biodiversity (Diaz Citation2001, Bunn and Arthington Citation2002). Our models, developed specifically to simulate stratification dynamics in river waterholes, offer the possibility to predict the duration and severity of future hypoxia and the risks of acute destratification events given future weather conditions and provide critical information for water and fisheries managers, including the potential to use them to evaluate risks under future climate change scenarios. Given the prolonged stratification and extreme oxygen limitation of waterholes, both measured and modelled, and the critical role of these waterholes for maintaining viable populations of fishes and other aquatic organisms in dryland rivers, implementing artificial mixing where large bubbles of compressed air are released from a jet on the waterhole bottom to promote vertical mixing may occasionally be needed (Müller and Stadelmann Citation2004; ). This approach was implemented by the New South Wales Government during the extremes of the 2018–2020 drought to help reduce the risks of acute destratification events, which had previously resulted in mass fish kills in the Darling River system (Baldwin et al. Citation2022). Further research, including the potential use of our models to simulate the effectiveness of artificial mixing approaches, is needed to manage aquatic oxygen levels and provide benefits to aquatic organisms.
In the case of the Menindee mass fish kill events, algal blooms were a key component driving hypoxia, and the first fish kill event was identified as a driver of subsequent events as dead fish decomposed (Sheldon et al. Citation2022). Our observed and modelled results that anoxic and hypoxic conditions are common and long-lasting in stratified waterholes during periods without flow suggest that increases in biological oxygen demand, such as those caused by algal blooms or bacterial decomposition of dead biota, may perpetuate greater risks of catastrophic failures of waterhole quality and fish kills. This possibility has implications for environmental risks in the region if the Australian National Carp Control Plan (NCCP) option to control invasive common carp (Cyprinus carpio) using the lethal cyprinid herpesvirus 3 is implemented (FRDC Citation2022). With limited access across the dryland rivers of the northern Murray-Darling Basin and poor visibility due to high turbidity in remote waterholes, a situation is created where successful dead carp biomass removal by manual means is questionable, and where carp mortality events can further aggravate existing low oxygen conditions (Marshall et al. Citation2018, Kopf et al. Citation2019). Managing invasive species in ways that could avoid any increased anoxia in these rivers is therefore recommended, and where possible to build resilience in native populations by having restocking strategies in place after carp removal. The models we present here provide means for a more realistic assessment of these risks, which highlight the need for local oxygen and temperature data to locally calibrate adjustments to fully assess the risk.
Potential response to climate change
In addition to current challenges, a particular challenge is how waterhole stratification and hypoxia will change under climate change. Based on the modelled results at Kooroon using the example scenario, an increase of 2–10 °C in water temperature by 2080–2099 with the surface layer reaching 38 °C in December–January (a–c) may cause thermal stress in confined fish populations in these disconnected waterholes. Temperature plays a vital role in the regulation of metabolic, physiological, and behavioural processes in fish (Jobling Citation1997, Beitinger et al. Citation2000). A recent study suggested that Murray cod (Maccullochella peelii), an iconic Australian native and important apex species in the Murray-Darling Basin has an upper thermal tolerance of ∼38 °C when acclimated to average summer temperature (McPhee et al. Citation2023). Although fish can respond to unfavourable conditions by behavioural adjustments (Haesemeyer Citation2020), such as moving to deeper and thus cooler regions in the waterhole, they will likely be facing the dilemma that these regions are subject to prolonged anoxic or hypoxic conditions as oxygen saturation is predicted to be ∼10–20% lower for most of the no-flow period and up to 40% lower at times (f). This prediction implies that in future climate, the niche in these waterhole refugia that can support fish survival during drought is likely to narrow, potentially increasing competition or causing fish death under extreme conditions. In addition, the current model considered only local-scale changes in climate in a snapshot of time, without accounting for cumulative effects on river hydrology such as increase in drought duration and frequency. Under the RCP 8.5 scenario, duration of extreme droughts in the Moonie River region where Kooroon is located was predicted to increase by 1.3 months per year by 2080–2099 (average projection of 12 climate models; Syktus et al. Citation2020), which will further reduce quantity and quality of habitat.
Figure 13. Comparison of water temperature (°C) and dissolved oxygen saturation (%) between (a and d) current climate, (b and e) future climate (2080–2099; day/month/year) under RCP 8.5, and (c and f) difference at Kooroon.

The analysis provides a conceptual framework for risk assessment and shows how the model approach can be used to ascertain the nature of future risks in dryland river waterholes. The results provide initial insights into the mechanisms by which climate change can amplify the existing risks of fish kills. We do not suggest these results reflect the exact conditions that would be experienced in the 2090s; obviously many other factors impacting catchment and river hydrology will impact waterhole persistence by that time (McJannet et al. Citation2014, Cockayne Citation2021). The results, however, do demonstrate that shifts in stratification phenology within waterholes brought about by a warming climate will only further stress fish communities compared to present conditions. The extent of the habitat reduction will depend on the specific trajectory of climate change we follow, noting that in this example we chose the upper extreme scenario (RCP 8.5) for illustrative purposes. As confidence in this model approach is further developed, we recommend implementing more comprehensive climate change risk assessments considering the range of plausible climate scenarios and addressing uncertainty in these projections using prediction ensembles and uncertainty analysis methodologies to better quantify future likely states.
Conclusion
We presented the development of a river waterhole-scale ecohydrology model using GLM coupled with AED for modelling stratification and DO regimes during dry period in the northern Murray-Darling Basin rivers in Australia. All study sites exhibited persistent stratification (weeks to months) with hypoxia/anoxia in the hypolimnion during the dry periods, each with unique thermal and DO patterns. Our models resolved the local factors such as diurnal vegetation shading patterns and wind sheltering and successfully predicted the prolonged stratification and hypoxia in these unique shallow waterholes with 0.85–2.45 °C error for temperatures and 7.08–19.29% error for oxygen saturation. To obtain a reliable model calibration, local factors to capture each waterhole’s microclimate were needed because of the combined effects of river morphology and canopy cover that provide various degrees of solar shading and wind sheltering.
The models developed in this study could be a valuable tool to inform waterhole management and planning decisions and climate adaptation strategies. Restoration of riparian vegetation may be beneficial to aquatic organisms by reducing waterhole temperature in summer, stratification, and the severity of hypoxia development. For example, water temperature could be lowered by ∼2–4 °C in summer from having as little as 5% canopy cover. Applications also include evaluation of the risks and benefits of management under different water resource allocation and environmental flows options, local waterhole management interventions such as increasing shading and wind exposure or artificial aeration of waterholes, and viral biocontrol of invasive common carp.
Our exploration of a future climate change scenario (RCP 8.5) suggests increased risks to waterhole quality, with a temperature increase of 2–10 °C by 2080–2099 and with the surface layer reaching 38 °C in December–January, along with a reduction in oxygen saturation of 10% to 20% in the middle layers for most of the no-flow period. The sensitivity to acute and chronic exposure to hypoxia of different species that utilise waterholes as drought refugia remains a key knowledge gap in translating hypoxia risk into ecological risk, and we recommend this topic for future research.
Acknowledgements
We thank landowners Walter and Alison Brown (Kooroon), Greg Williams (Kurmala), Peter McSwan (Brenda), Russell Topp (Reilly’s Weir), Jonathan and Kathryn Burrell (Trafalgar), and David Palmer (Weribone) for allowing access to waterholes on their properties.
Disclosure statement
No potential conflict of interest was reported by the author(s).
Additional information
Funding
References
- Acuña V, Datry T, Marshall J, Barceló D, Dahm CN, Ginebreda A. 2014. Why should we care about temporary waterways? Science. 343:1080–1081.
- Agostinho AA, Alves DC, Gomes LC, Dias RM, Petrere M Jr, Pelicice FM. 2021. Fish die-off in river and reservoir: a review on anoxia and gas supersaturation. Neotrop Ichthyol. 19(3):e210037.
- Andersen MR, Kragh T, Sand-Jensen K. 2017. Extreme diel dissolved oxygen and carbon cycles in shallow vegetated lakes. Proc Royal Soc B. 284:20171427.
- Baldwin DS, Boys CA, Rohlfs A-M, Ellis I, Pera J. 2022. Field trials to determine the efficacy of aerators to mitigate hypoxia in inland waterways. Mar Freshw Res. 73(2):211–222.
- Beitinger TL, Bennett WA, McCauley RW. 2000. Temperature tolerances of North American freshwater fishes exposed to dynamic changes in temperature. Environ Biol Fishes. 58:237–275.
- Bird RE, Hulstrom RL. 1981. A simplified clear sky model for direct and diffuse insolation on horizontal surfaces. Golden (CO): Solar Energy Research Institute. SERI/TR-642–761.
- Biswas TK, Mosley LM. 2019. From mountain ranges to sweeping plains, in droughts and flooding rains; River Murray water quality over the last four decades. Water Resour Manag. 33:1087–1101.
- Bormans M, Webster IT. 1997. A mixing criterion for turbid rivers. Environmental modelling and software. 12:329–333.
- Branco B, Torgersen T, Bean JR, Grenier G, Arbige D. 2005. A new water column profiler for shallow aquatic systems. Limnol Oceanogr Meth. 3:190–202.
- Branco B, Torgersen T. 2009. Predicting the onset of thermal stratification in shallow inland waterbodies. Aquat Sci. 71:65–79.
- Brookes JD, Hipsey MR. 2019. Water quality risk assessment of carp biocontrol for Australian waterways. Unpublished client report for the Fisheries Research and Development Corporation. Adelaide (South Australia): University of Adelaide, Environment Institute. http://nla.gov.au/nla.obj-2141056903
- Bruce LC, Cook, PLM, Teakle I, Hipsey MR. 2014. Hydrodynamic controls on oxygen dynamics in a riverine salt wedge estuary, the Yarra River estuary, Australia. Hydrol Earth Syst Sci. 18:1397–1411.
- Bunn SE, Arthington AH. 2002. Basic principles and ecological consequences of altered flow regimes for aquatic biodiversity. Environ Manag. 30(4):492–507.
- Bunn SE, Thoms MC, Hamilton SK, Capon SJ. 2006. Flow variability in dryland rivers: boom, bust and the bits in between. River Res Appl. 22:179–186.
- Christianson KR, Johnson BM, Hooten MB. 2020. Compound effects of water clarity, inflow, wind and climate warming on mountain lake thermal regimes. Aquat Sci. 82:6.
- Cockayne B. 2021. Climate change effects on waterhole persistence in rivers of the Lake Eyre Basin, Australia. J Arid Environ. 187:104428.
- Condie S, Webster I. 2001. Estimating stratification in shallow water bodies from mean meteorological conditions. J Hydraul Eng. 127:286–292.
- Davidson K, Anderson DM, Mateus M, Reguera B, Silke J, Sourisseau M, Maguire J. 2016. Forecasting the risk of harmful algal blooms. Harmful Algae. 53:1–7.
- Diaz RJ. 2001. Overview of hypoxia around the world. J Environ Qual. 30:275–281.
- [DSITI] Department of Science, Information Technology and Innovation. 2015. Waterhole refuge mapping and persistence analysis in the Lower Balonne and Barwon-Darling rivers. Brisbane (Australia): Queensland Government, DSITI.
- Elo AR. 2005. Modelling of summer stratification of morphologically different lakes. Hydrol Res. 36(3):281–294.
- [FRDC] Fisheries Research and Development Corporation. 2022. National carp control plan. Australia. https://www.frdc.com.au/knowledge-hub/national-carp-control-plan
- Garner G, Malcolm IA, Sadler JP, Hannah DM. 2017. The role of riparian vegetation density, channel orientation and water velocity in determining river temperature dynamics. J Hydrol. 553:471–485.
- Haesemeyer M. 2020. Thermoregulations in fish. Mol Cell Endocrinol. 518:110986.
- Hamilton GS, Fielding F, Chiffings AW, Hart BT, Johnstone RW, Mengersen K. 2007. Investigating the use of a Bayesian network to model the risk of Lyngbya majuscula bloom initiation in Deception Bay, Queensland, Australia. Hum Ecol Risk Assess. 13(6):1271–1287.
- Heiskanen JJ, Mammarella I, Ojala A, Stepanenko V, Erkkilä KM, Miettinen H, Sandström H, Eugster W, Leppäranta M, Järvinen H, et al. 2015. Effects of water clarity on lake stratification and lake-atmosphere heat exchange. J Geophys Res Atmos. 120:7412–7428.
- Herb WR, Stefan HG. 2004. Temperature stratification and mixing dynamics in a shallow lake with submersed macrophytes. Lake Reservoir Manag. 20:296–308.
- Hipsey MR. 2022. Modelling aquatic eco-dynamics: overview of the AED modular simulation platform. Zenodo Repository. doi:10.5281/zenodo.6516222
- Hipsey MR, Bruce LC, Boon C, Busch B, Carey CC, Hamilton DP, Hanson PC, Read JS, de Sousa E, Weber M, Winslow LA. 2019. A General Lake Model (GLM 3.0) for linking with high-frequency sensor data from the Global Lake Ecological Observatory Network (GLEON). Geosci Model Dev. 12:473–523.
- Hipsey MR, Sivapalan M. 2003. Parameterizing the effect of a wind shelter on evaporation from small water bodies. Water Resour Res. 39(12):1339.
- Hocking GC, Straškraba M. 1999. The effect of light extinction on thermal stratification in reservoirs and lakes. Int Rev Hydrobiol. 84:535–556.
- Holgerson MA, Richardson DC, Roith J, Bortolotti LE, Finlay K, Hornbach DJ, Gurung K, Ness A, Andersen MR, Bansal S, et al. 2022. Classifying mixing regimes in ponds and shallow lakes. Water Resour Res. 58(7):e2022WR032522.
- Huang P, Kilminster K, Larsen S, Hipsey MR. 2018. Assessing artificial oxygenation in a riverine salt-wedge estuary with a three-dimensional finite-volume model. Ecol Eng. 118:111–125.
- [IPCC] Intergovernmental Panel on Climate Change. 2021. Summary for policymakers. In: Masson-Delmotte VP, Zhai A. Pirani SL, Connors C, Péan S, et al., editors. Climate change 2021: the physical science basis. Contribution of Working Group I to the sixth assessment report of the Intergovernmental Panel on Climate Change. Cambridge (UK) and New York (NY): Cambridge University Press; p.3–32.
- Jobling M. 1997. Temperature and growth: modulation of growth rate via temperature change. In: Wodod CM, McDonald DG, editors. Global warming: implications for freshwater and marine fish. Seminar series – Society for Experimental Biology, No. 61. Cambridge (UK): Cambridge University Press; p. 225–254.
- Kim J, Lee T, Seo D. 2017. Algal bloom prediction of the lower Han River, Korea using the EFDC hydrodynamic and water quality model. Ecol Modell. 366:27–36.
- Kirono D, Round V, Heady C, Chiew FHS, Osbrough S. 2020. Drought projections for Australia: updated results and analysis of model simulations. Weather Clim. 30:100280.
- Kopf RK, Boutier M, Finlayson C, Hodges K, Humphries P, King A, Kingsford RT, Marshall J, McGinness HM, Thresher R, Vanderplasschen A. 2019. Biocontrol in Australia: Can a carp herpesvirus (CyHV-3) deliver safe and effective ecological restoration? Biol Invasions. 21:1857–1870.
- Ladwig R, Hanson PC, Dugan HA, Carey CC, Zhang Y, Shu L, Duffy CJ, Cobourn KM. 2021. Lake thermal structure drives inter-annual variability in summer anoxia dynamics in a eutrophic lake over 37 years. Hydrol Earth Syst Sci Discuss. 25:1009–1032.
- Liu J, Wang B, Oldham CE, Hipsey MR. 2020. Unravelling the metabolism black-box in a dynamic wetland environment using a hybrid model framework: storm driven changes in oxygen budgets. Sci Total Environ. 723:138020.
- Liu M, Zhang Y, Zhou Y, Shi K, Zhu G, Sun X. 2022. Thermal structure controlled by morphometry and light attenuation across subtropical reservoirs. Hydrol Processes. 36(2):e14502.
- Lobegeiger J. 2010. Refugial waterholes project: research highlights. Brisbane (Australia): Queensland Government, Department of Environment and Resource Management. http://tinyurl.com/357qnzb
- Losordo TM, Piedrahita RH. 1991. Modelling temperature variation and thermal stratification in shallow aquaculture ponds. Ecol Modell. 54(3–4):189–226.
- Marshall J, Davison AJ, Kopf RK, Boutier M, Vanderplasschen A. 2018. Biocontrol of invasive carp: risks abound. Science. 359:877.
- Marshall JC, Lobegeiger JS, Starkey A. 2021. Risks to fish populations in dryland rivers from the combined threats of drought and instream barriers. Front Environ Sci. 9:671556.
- Martinsen KT, Andersen MR, Sand-Jensen K. 2019. Water temperature dynamics and the prevalence of daytime stratification in small temperate shallow lakes. Hydrobiologia. 826:247–262.
- McGregor GB, Marshall JC, Lobegeiger JS, Holloway D, Menke N, Coysh J. 2018. A risk-based ecohydrological approach to assessing environmental flow regimes. Environ Manag. 61(3):358–374.
- McJannet D, Marvanek S, Kinsey-Henderson A, Petheram C, Wallace J. 2014. Persistence of in-stream waterholes in ephemeral rivers of tropical northern Australia and potential impacts of climate change. Mar Freshw Res. 65:1131–1144.
- McPhee D, Watson JR, Harding DJ, Prior A, Fawcett JH, Franklin CE, Cramp RL. 2023. Body size dictates physiological and behavioural responses to hypoxia and elevated water temperatures in Murray cod (Maccullochella peelii). Conserv Physiol. 28(11(1)):coac087.
- [MDBA] Murray–Darling Basin Authority. 2019. Climate change and the Murray–Darling Basin plan. MDBA publication no: 09/19.
- Mosley LM, Wallace T, Rahman J, Roberts T, Gibbs M. 2021 May 15. An integrated model to predict and prevent hypoxia in floodplain-river systems. J Environ Manag. 286:112213.
- Müller R, Stadelmann P. 2004. Fish habitat requirements as the basis for rehabilitation of eutrophic lakes by oxygenation. Fish Manag Ecol. 11:251–260.
- Padisak J, Reynolds CS. 2003. Shallow lakes: the absolute, the relative, the functional and the pragmatic. Hydrobiologia. 506–509:1–11.
- Pollock MS, Clarke LMJ, Dubé MG. 2007. The effects of hypoxia on fishes: from ecological relevance to physiological effects. Environ Rev. 15:1–14.
- Preite CK, Pearson RG. 2017. Water-quality variability in dryland riverine waterholes: a challenge for ecosystem assessment. Int J Limnol. 53:221–232.
- Rayner TS, Jenkins KM, Kingsford RT. 2009. Small environmental flows, drought and the role of refugia for freshwater fish in the Macquarie Marshes, arid Australia. Ecohydrology. 2:440–453.
- Sheldon F, Barma D, Baumgatner LJ, Bond N, Mitrovic SM, Vertessy R. 2022. Assessment of the causes and solutions to the significant 2018–19 fish deaths in the lower Darling River, New South Wales, Australia. Mar Freshw Res. 73:147–158.
- Sheldon F, Bunn ES, Hughes JM, Arthington AH, Balcombe SR, Fellows CS. 2010. Ecological roles and threats to aquatic refugia in arid landscapes: dryland river waterholes. Mar Freshw Res. 61:885–895.
- Sheldon F, Fellows CS. 2010. Temporal and spatial variability of water quality and water chemistry in two Australian dryland rivers. Mar Freshw Res. 61:864–874.
- Stewart IT, Rogers J, Graham A. 2020. Water security under severe drought and climate change: disparate impacts of the recent severe drought on environmental flows and water supplies in central California. J Hydrol. 7:100054.
- Stocks JR, Ellis LM, van der Meulen DE, Doyle JI, Cheshire JM. 2021. Kills in the Darling: assessing the impact of the 2018–20 mass fish kills on the fish communities of the lower Darling–Baaka River, a large lowland river of south-eastern Australia. Mar Freshw Res. 73:159–177.
- Sun L, Liang XZ, Ling T, Xu M, Lee X. 2020. Improving a multilevel turbulence closure model for a shallow lake in comparison with other 1-D models. J Adv Model Earth Syst. 12:e2019MS001971.
- Syktus J, Trancoso R, Ahrens D, Toombs N, Wong K. 2020. Queensland future climate dashboard: downscaled CMIP5 climate projections for Queensland. https://www.longpaddock.qld.gov.au/qld-future-climate/
- Tooth D. 2000. Process, form and change in dryland rivers: a review of recent research. Earth Sci Rev. 51:67–107.
- Vertessy R, Barma D, Baumgartner L, Mitrovic S, Sheldon F, Bond N. 2019. Independent assessment of the 2018–19 fish deaths in the lower Darling. Canberra (Australia): Australian Government.
- Vilas MP, Marti CL, Oldham CE, Hipsey MR. 2018. Macrophyte-induced thermal stratification in a shallow urban lake promotes conditions suitable for nitrogen-fixing cyanobacteria. Hydrobiologia. 806:411–426.
- Wallace J, Waltham N, Burrows D. 2017. A comparison of temperature regimes in dry-season waterholes in the flinders and gilbert catchments in northern Australia. Mar Freshw Res. 68:650–667.
- Wanninkhof R. 1992. Relationship between wind speed and gas exchange over the ocean. J Geophys Res. 97(C5):7373.
- Webb BW, Zhang Y. 1997. Spatial and seasonal variability in the components of the river heat budget. Hydrol Process. 11:79–101.