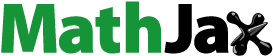
Abstract
This paper examines the potential of ChatGPT, a large language model, as a financial advisor for listed firm performance forecasts. We focus on the constituent stocks of the China Securities Index 300 and compare ChatGPT’s forecasts for major financial performance measures with human analysts’ forecasts and the realised values. Our findings suggest that ChatGPT can correct the optimistic biases of human analysts. This study contributes to the literature by exploring the potential of ChatGPT as a financial advisor and demonstrating its role in reducing human biases in financial decision-making.
Introduction
The recent development of large language models (LLMs) has led to numerous applications across various disciplines. With a large number of parameters, these models can be fine-tuned to receive input instructions and generate human-like responses. In such a context, a body of literature has emerged to explore their applications in the field of finance. In this paper, we investigate the capability of ChatGPT, the most renowned LLM, in forecasting listed firm performance. In addition, we examine whether ChatGPT can reduce the optimistic biases of human analysts.
Although ChatGPT is primarily a language model and not specifically designed for financial decision-making (Ko and Lee Citation2023), its ability to efficiently extract and process a wide range of information makes it suitable to serve as a financial advisor. We provide a detailed review of the usage of ChatGPT in finance in the next section.
In this paper, we investigate ChatGPT’s role in forecasting listed firm performance. Analyst forecasts involve intensive information extraction and processing activities in which machines have an advantage, given their high computation efficiency, over humans (Boyacı, Canyakmaz, and de Véricourt Citation2023). Research consistently shows that machines, or machine-augmented analysts, outperform humans in earnings and stock price predictions (e.g. Chen et al. Citation2022; Coleman, Merkley, and Pacelli Citation2022; Cao et al. Citation2023). Therefore, we expect ChatGPT to produce more accurate performance forecasts than human analysts do.
Furthermore, we explore the channels through which improved forecast accuracy is achieved. Human analysts often exhibit optimistic biases (Easterwood and Nutt Citation1999; Lim Citation2001; Wu et al. Citation2018), which stem from their involvement in the forecast process (Duru and Reeb Citation2002) and conflicts of interest (Hovakimian and Saenyasiri Citation2010). Machines, however, are impartial (Tantri Citation2021) and less affected by human biases (Liaudinskas Citation2022; Liu Citation2022). These biases can explain many anomalies (van Binsbergen, Han, and Lopez-Lira Citation2023) and may be related to the memory process. Drawing evidence from the pricing of artistic works, Aubry et al. (Citation2023) show that machines can reduce human experts’ conscious rational biases and unconscious behavioural biases, thus improving the forecast accuracy of auction outcomes. Similarly, we posit that ChatGPT’s superior ability in forecasting firm performance results from mitigating human analysts’ optimistic biases.
One of the major challenges in studying ChatGPT’s forecasts involves restricting the information set. It is crucial to ensure that the model does not use future information that includes the realised outcomes. However, as ChatGPT operates as a black box, it is not possible to prevent it from using data beyond a certain time point simply by giving it instructions. Fortunately, ChatGPT’s training data extend only up to September 2021. Leveraging on this setting, we instruct ChatGPT to forecast the performance of each firm in the China Securities Index 300 (CSI 300) from 2021 to 2023 and then compare its forecasts with those of human analysts and the realised performance. For human analysts’ forecasts, we only use analyst reports issued in September 2021 to ensure that human analysts and ChatGPT have access to a comparable information set. Empirically, we focus on seven major financial performance measures, namely the price-to-earnings ratio (PE), the price-to-book ratio (PB), earnings per share (EPS), the return on assets (ROA), the return on equity (ROE), Revenue Growth, and Profit Growth.
In the comparison between ChatGPT and human analysts, we find that ChatGPT is significantly more conservative than human analysts across all performance dimensions and forecast horizons, i.e. the end of the years 2021, 2022 and 2023. Using realised performance as a benchmark, human analysts exhibit systematic and persistent optimistic biases. They overestimate all seven performance measures across all time horizons. The upward biases are more pronounced for the long-term horizon, with the exception of the PE forecasts. In contrast, ChatGPT does not exhibit one-sided upward biases. For the short-term horizon of 2021, its forecasts are not significantly different from the realised performance for five of the seven measures, while the forecasted values of the remaining two are lower than the realised ones. For the long-term horizon of 2022, ChatGPT exhibits biases towards higher values for five of the seven measures. However, the forecast errors are quantitatively smaller than those of human analysts, indicating that ChatGPT at least partially mitigates the optimistic biases in human analysts’ forecasts.
We quantify the humans’ optimistic biases corrected by ChatGPT in a formal regression setting. We use the upward forecast errors, calculated as the differences between forecasted and realised values, as the dependent variables, and regress them against a ChatGPT dummy variable indicating whether the forecast is issued by ChatGPT. ChatGPT exhibits fewer optimistic biases in all seven measures than human analysts, and the differences are statistically significant for ROA, ROE, Revenue Growth, and Profit Growth. This implies that ChatGPT has the potential to help correct the optimistic biases of human analysts.
This study contributes to the literature by exploring the potential of ChatGPT as a financial advisor, and it deepens our understanding of the strengths and limitations of investment based on advice from artificial intelligence (AI). In addition, it contributes to the discussion on the interaction between machines and humans by demonstrating how machines can reduce human biases.
Literature review
We summarise the research on the application of ChatGPT in finance in three aspects: financial concept comprehension, academic use, and investment decision-making.
The first strand of literature explores whether ChatGPT is able to comprehend key financial concepts, explain financial reporting to non-professionals, and assume the role as a personal financial advisor. ChatGPT can accurately explain financial concepts such as alpha values, crowdfunding, alternative finance, financial risk, financial crises, the Basel framework, and banking products (Wenzlaff and Spaeth Citation2022; Hofert Citation2023; Lakkaraju et al. Citation2023; Ren, Lee, and Hu Citation2023; Yue et al. Citation2023), although its elaboration of mathematical facts needs improvement (Hofert Citation2023). Niszczota and Abbas (Citation2023) examine whether ChatGPT is capable of serving as a financial advisor and find that it exhibits a higher level of financial literacy than human investors who make random guesses.Footnote1 Overall, ChatGPT is comparable to financial professionals and demonstrates high levels of accuracy and expertise (Ren, Lee, and Hu 2023). In a similar setting, Wei, Wu, and Chu (Citation2023) find that ChatGPT’s answers to auditing questions imitate those from experienced financial auditors.
In addition to helping laypeople comprehend financial and accounting concepts, ChatGPT is capable of explaining the jargon in plain language. Ren, Lee, and Hu (2023) show that ChatGPT’s answers to financial and accounting questions are more understandable to laypeople than those from human experts. Neilson (Citation2023) provides more direct evidence that ChatGPT can recommend superannuation contribution plans to non-professionals. By asking ChatGPT questions or giving it directions such as ‘explain the meaning of alpha in finance to my grandmother’, Yue et al. (Citation2023) show that ChatGPT can further customise the complexity of its explanations when given indications of its audience. Notwithstanding its merits, Lakkaraju et al. (Citation2023) and Neilson (Citation2023) warn of the possible limits of ChatGPT in numeric reasoning, its inconsistency, and its ignorance of various relevant issues, such as local regulatory requirements.
Regarding the academic use of ChatGPT in economics and finance research, evidence of ChatGPT’s performance is mixed depending on the specific jobs that it undertakes. ChatGPT does well in coding support, data analyses, and the interpretation of findings (Alshater Citation2022; Dowling and Lucey Citation2023; Feng, Hu, and Li Citation2023; Korinek Citation2023). However, its performance is unsatisfactory in literature synthesis, the development of testing frameworks, domain-specific expertise, and idea origination (Alshater Citation2022; Dowling and Lucey Citation2023).
The literature investigating the role of ChatGPT in investment decision-making is closely related to our research context. The literature is inconclusive regarding ChatGPT’s understanding and interpretation of financial texts in a zero-shot setting (i.e. no example of expected responses provided in prompts). Some papers conclude that ChatGPT is accurate and efficient in extracting the opinions and sentiments in news, Fedspeak,Footnote2 and corporate disclosures (Hansen and Kazinnik Citation2023; Jha et al. Citation2023). Conversely, others claim that ChatGPT struggles in tasks such as financial named entity recognition (FinNER) and sentiment analyses (Lan et al. Citation2023; Li et al. Citation2023). Wang et al. (Citation2023) demonstrate that assessing the performance of ChatGPT is complicated: it generates reasonable answers, which may not always be relevant to the prompts being given; however, at the same time, it outperforms fine-tuned bidirectional encoder representations from transformers (BERT) models in sentiment analyses. One possible explanation of the conflicting results may be the use of different data sources and performance measures. Studies relying on only one data set tend to overevaluate the performance of ChatGPT (Jha et al. Citation2023; Hansen and Kazinnik Citation2023), whereas those using multiple data sets and different tasks observe more of its limitations (Li et al. Citation2023; Wang et al. Citation2023).
Recent papers extend this literature by directly examining whether ChatGPT can extract value-relevant signals from the financial context in investment decision-making. Lopez-Lira and Tang (Citation2023) conduct a sentiment analysis of news headlines for stocks using ChatGPT, classifying news as good, bad, or irrelevant. They construct a ‘ChatGPT score’ for each stock and find that it is positively correlated with subsequent daily stock returns. Kim, Muhn, and Nikolaev (Citation2023) instruct ChatGPT to summarise information contained in management discussions and analyses, annual reports, and earnings conference calls, and generate refined summaries with pronounced sentiments. The refined summaries exhibit more significant explanatory power over the abnormal returns surrounding the disclosure days than the original texts. However, ChatGPT has limitations. Xie et al. (Citation2023) use ChatGPT to predict the direction of future stock movements with inputs of historical stock prices. Results show that the performance of ChatGPT is poor as the predictions are less accurate than those of logistic regressions.
Applying ChatGPT to investment, Ko and Lee (Citation2023) find that ChatGPT outperforms a randomly selected portfolio. Using a three-stage procedure, Chen et al. (Citation2023) provide further evidence on how ChatGPT utilises the information it extracts to achieve superior investment performance. They first give financial news prompts to ChatGPT and ask which companies are positively or negatively affected. Then, they construct graphs that visualise the relationships. Finally, they use machine learning methods, including graph neural networks and long short-term memory neural networks, to make predictions of stock price movements with higher accuracy than those of ChatGPT.
Our paper differs from the above research in three distinct aspects. First, we examine ChatGPT’s use in investment using a comprehensive set of performance measures. We investigate the accuracy of ChatGPT in predicting stock valuation, profitability, and growth. Second, in addition to comparing ChatGPT and humans, we discuss the use of ChatGPT to reduce human biases. By showing how ChatGPT is more impartial than human analysts and less subject to optimistic biases, this paper reveals the potential for the collaboration between ChatGPT and humans in investment decision-making.
Data description
We restrict our sample to the constituent stocks of the CSI 300. The CSI 300 was introduced on 8 April 2005 by the Shanghai and Shenzhen stock exchanges. It consists of the 300 most actively traded Chinese A-share stocks, which account for over 70% of the combined market capitalisation of the two exchanges. The index is widely recognised as a comprehensive indicator of broad movements in the Chinese stock markets (Hou and Li Citation2014).
We designate seven measures of firm performance, which are commonly used and discussed by human analysts in evaluating firm performance. For stock valuation, we use PE, calculated as the share price divided by the earnings per share, and PB, constructed as the share price over the book value per share. Regarding profitability, we use profit divided by the outstanding shares (EPS), net income over total assets (ROA), and net income over total equity (ROE). We measure firm growth using the rates of Revenue Growth and Profit Growth.
To obtain ChatGPT’s forecasts, we input each firm’s name and stock code and request ChatGPT to forecast PE, PB, EPS, ROA, ROE, Revenue Growth, and Profit Growth at the end of 2021, 2022, and 2023. The responses from ChatGPT are highly dependent on the prompts, which are essentially the user’s questions. Korinek (Citation2023) finds that minor tweaks in the prompts might result in different outcomes. Therefore, we try different prompts to retrieve the desired results. Following the guidance by OpenAIFootnote3 and Alshater (Citation2022), we construct the prompt as follows:
Provide a table of price-to-earnings (P/E) forecasts at the end of 2021, 2022 and 2023 for the firms below, as of September 2021:
[A list of firm names and tickers]
There should be four columns in the table: Ticker, P/E 2021, P/E 2022, P/E 2023.
We make our prompt as clear as possible. The measure, time horizons, firms, and as-of time are specified explicitly in the prompt. In the absence of time horizons, ChatGPT responds with the latest realised values as of September 2021. In the absence of the as-of time, ChatGPT declines the assignment and explains it is unable to provide information after September 2021. ChatGPT makes the forecasts that we need only when the prompt contains both the time horizon and the as-of time. The table output format that we request hides ChatGPT’s language analyses of each firm’s future performance and keeps the forecasted values only.Footnote4 We demarcate the prompt with delimiters: using one newline delimiter to indicate firms and two newline delimiters to indicate instructions and the firm list.
There is a context length limit in one single prompt–response pair. We split the 300 sample firms into 10 batches with 30 firms in each batch. This reasonably small batch size avoids length overflow and eliminates biases introduced by both forecast breaks within a firm (across horizons) and resumption prompt interference, thus enabling the continuation of forecasting.
In some text generation tasks, few-shot prompting, or providing dialogue examples in the prompt, can improve generation quality. By adopting zero-shot prompting instead of few-shot prompting, we take a strictly neutral stance and do not bias ChatGPT with human forecast examples (Zhao et al. Citation2021). We always start a new chat when moving to the next performance measure to ensure that the outputs are not affected by previous instructions.
Another challenge is that ChatGPT may produce slightly different forecasts even when given the same prompt multiple times. Fortunately, the results are highly similar and comparable despite minor differences (Ko and Lee Citation2023). To mitigate generation idiosyncrasy, we independently repeat the process 35 times and take the average.Footnote5 This approach gives us three-year forecasts on the seven measures for the 300 firms of the CSI 300.
We obtain financial analysts’ forecasts from the China Stock Market & Accounting Research (CSMAR) database. To ensure that human analysts and ChatGPT use a comparable information set, we restrict the human forecasts to those issued in September 2021. Some reports provide forecasts for different time horizons. We obtain a total of 10,550 forecast-year observations from 582 analyst reports. The realised performance data come from the CSMAR and Wind, but we only have the data for 2021 and 2022. The 2023 data were not available at the time of writing this paper.
presents the summary statistics of ChatGPT’ forecasts, human analysts’ forecasts, and the realised performance, which are displayed in three rows for each measure. The first row contains ChatGPT’ forecasts with 900 observations (3 years × 300 firms per year), the second row contains the human analysts’ forecasts, and the last row contains the 600 observations of realised performance (2 years × 300 firms per year). As many stocks in our sample are followed by multiple analyst teams, a stock may have more than one forecast from human analysts for a given year. Therefore, the sample of human analysts’ forecasts can be larger than 900. In addition, an analyst report may not include all seven measures, leading to an unbalanced number of observations across different performance measures.Footnote6
Table 1. Summary statistics.
reveals four noticeable patterns. First, the average and median forecasted values from ChatGPT are lower than those from human analysts in all seven measures. Second, human analysts consistently exhibit upward biases. Third, ChatGPT’s forecasted values are closer to the realised ones, except for the forecast of PE. Fourth, ChatGPT’s forecast errors are two-sided. It overestimates PB, EPS, ROE, Revenue Growth, and Profit Growth, but its forecasted values of PE and ROA are lower than the realised values. The summary statistics in are consistent with our conjecture that ChatGPT outperforms human analysts by reducing their optimistic biases.
The above results pool the forecasts for different time horizons together, making it difficult to interpret the differences. In , we perform mean difference t-tests for each performance measure and each time horizon between ChatGPT and human analysts in Columns (1)–(3). We find evidence that human analysts are more optimistic than ChatGPT. In Columns (4) and (5), we compare human analysts’ forecasts with the realised performance. We only have results for 2021 and 2022 because the 2023 data of realised performance are not available yet. Human analysts overestimate all performance measures significantly, and the magnitude of upward biases increases with the time horizon. In contrast, ChatGPT does not exhibit any optimistic biases for the 2021 horizon (Column [6]); its forecasts are not significantly different from the realised performance for five measures, except for ROA and Revenue Growth, which are slightly underestimated. However, ChatGPT’s accuracy does not persist for the long-term horizon of 2022. The forecast errors in Column (7) are significantly non-zero in six of the seven performance measures, with the exception of PE. ChatGPT overestimates five of the seven measures, but the biases are much fewer than those come from human analysts.
Table 2. Mean difference results.
Empirical results
We present formal regression-based evidence to quantify the human analysts’ optimistic biases that ChatGPT corrects. We use a full sample of forecasts from both ChatGPT and human analysts for the horizons of 2021 and 2022 (i.e. forecasted values for firm performance at the end of 2021 and 2022). Our dependent variables are upward forecast errors, calculated as the differences between the forecast, E(y), and the realised performance, y. Our focal variable is ChatGPT, a dummy variable indicating whether a forecast is issued by ChatGPT or human analysts.
We include a set of control variables for factors that are associated with analyst forecast errors as documented in the literature. Abarbanell (Citation1991) shows that analyst forecasts do not fully incorporate past stock price information and are insufficiently efficient. This implies that price increases predict downward biased forecast errors. To control for this effect, we include the annualised return 52 weeks prior to September 2021.
Uncertainty affects forecast errors (Das, Levine, and Sivaramakrishnan Citation1998; Lim Citation2001). Analysts tend to issue forecasts in favour of firms in exchange for private information from the management team. Following Lim (Citation2001), we use the annualised volatility 52 weeks prior to September 2021 as a market-based control for uncertainty.
Analysts perform poorly in forecasting long-term performance (Harris Citation1999). Forecast errors increase with the time horizon, which means that it becomes harder for analysts to forecast performance accurately in the more distant future. Following Dong et al. (Citation2021) and Bolliger (Citation2004), we control for the forecast horizon.
The market accumulates more information about a firm as it ages. Maskara and Mullineaux (Citation2011) illustrate that both forecast errors and firm age are related to information asymmetry. Following Amir, Lev, and Sougiannis (Citation2003), we include firm age as a control variable.
Moreover, ownership structure affects analyst forecast errors (Ackert and Athanassakos Citation2003), particularly in the unique setting of the Chinese capital market (Huang and Wright Citation2015; Liu Citation2016), where sharp distinctions exist between state-owned and non-state-owned firms. We include a control variable indicating whether a firm is state-owned.
Finally, we include lagged firm characteristic measures, following Ali, Klein, and Rosenfeld (Citation1992), Cen, Hilary, and Wei (Citation2013), So (Citation2013), and Dong et al. (Citation2021).
We specify the regression model in the equation below. The subscripts denote firm i and performance measure j in horizon year t:
presents the cross-sectional regression results. Compared with human analysts, ChatGPT exhibits significantly fewer optimistic biases in four of the seven measures (ROA, ROE, Revenue Growth, and Profit Growth). The coefficients of ChatGPT are also negative (but not significant) when the dependent variables are the upward forecast errors of PE, PB, and EPS. These results are consistent with the results of the mean difference t-tests in . The results concerning control variables also align with expectations. The optimistic biases increase with the forecast horizon and decrease with the annualised return at the time when the forecast is made, which is consistent with the finding of Abarbanell (Citation1991).
Table 3. Cross-sectional regressions of forecast errors.
Motivated by Adiwardana et al. (Citation2020), who propose the sample-and-rank approach, we address the randomness in ChatGPT’s forecasts by selecting its most confident forecast to conduct a robustness test. In the sample-and-rank approach, an LLM picks the candidate text sequence with the highest predicted probability as the final output. Following the same idea, we pick the median of 35 responses as the final forecast of ChatGPT. We report the robustness test in , and the results are even stronger than those in . We observe that ChatGPT reduces human analysts’ optimistic biases in six of the seven dimensions, with PE being the only exception.
Table 4. Cross-sectional regressions of forecast errors (robustness).
Conclusion
In this paper, we utilise ChatGPT, an LLM, to forecast the performance of CSI 300 firms and compare its forecasts with those of human analysts issued in September 2021, which coincides with the cut-off date of ChatGPT’s training data. By using the realised performance as a benchmark, we consistently find that ChatGPT outperforms human analysts, achieving smaller upward forecast errors. Human analysts tend to provide optimistic forecasts, whereas ChatGPT is more conservative. The superior accuracy of ChatGPT’s forecasts can be attributed to its ability to correct the optimistic biases inherent in human analysts’ forecasts.
We consider that LLM applications such as ChatGPT are not meant to fundamentally replace human financial analysts. Rather, ChatGPT can assist and improve human financial forecasting. In this article, we provide evidence of ChatGPT’s ability to forecast financial performance and reduce humans’ optimistic biases, which suggests the potential for ChatGPT to assist analysts and investors. ChatGPT holds the promise of reducing overconfidence of or conflicts of interests among human analysts. On a cautionary note, we warn against overextending our results, as investors should neither solely rely on ChatGPT nor use its forecasts as the ‘correct’ answers. The result that ChatGPT outperforms human analysts on average does not mean it is always more accurate than human analysts. We interpret our findings as proving the value of ChatGPT in supplementing human analysts’ and investors’ forecasts. As this paper represents the first attempt to uncover the forecast differences between ChatGPT and human analysts, we encourage future researchers to explore in depth the reasons for these differences.
This research has some limitations, which also serve as warnings to our readers. First, the analysis only covers a brief period of two-year forecasts; thus, it is insufficient when considering a wide range of market dynamics. As a result, more evidence is needed about ChatGPT’s forecast performance across long cycles. The short time span raises concerns regarding the generalisability of our findings, especially when market conditions change. As data availability increases, future researchers should compare ChatGPT’s forecasts with those of human analysts over a longer historical span.
Second, due to the black-box nature of LLMs, we know little about the internal processes in which ChatGPT makes financial forecasts and delivers its forecasts with fewer optimistic biases than humans. Possible channels may include ChatGPT’s superior ability to process fundamental information and synthesise beliefs and/or its greater impartiality compared with humans. This limitation suggests another direction for future research, that is, to leverage novel research designs and uncover the internal mechanisms of ChatGPT through which fewer optimistically biased forecasts are made.
To conclude, this paper provides empirical evidence on the applications of ChatGPT, one of a growing number of LLMs, in forecasting listed firms’ performance, and highlights ChatGPT’s potential in the provision of financial advice. In addition, our results elucidate the role of LLMs in mitigating human biases in financial decision-making. Moving forward, future researchers may consider exploring other applications of LLMs in finance and investigating their effectiveness in various decision-making contexts.
Disclosure statement
No potential conflict of interest was reported by the author(s).
Additional information
Funding
Notes
1 The paper also documents that humans tend to overestimate the model’s performance and warns of the risk of overreliance on ChatGPT.
2 Fedspeak is the technical language used by the Federal Reserve (Fed) of the United States to communicate on monetary policy decisions. ChatGPT is able to classify the Fed’s statements into hawkish or dovish, according to Hansen and Kazinnik (Citation2023).
3 Available at: https://platform.openai.com/docs/guides/gpt-best-practices.
4 ChatGPT can provide reasons using human-like language on why it issues such forecasts along with the forecasted values, which basically include analyses of a firm’s fundamental outlook. In this paper, we keep the forecasted values only by requesting the table output format.
5 For instance, ChatGPT’s 35 forecast responses for the PE of Kweichow Moutai at the end of 2021 are 28.5, 39.2, 33.8, 34.8, 29.1, 32.1, 33.5, 34.2, 34.3, 34.5, 34.2, 33.2, 34.2, 34.6, 37.2, 35.2, 39.2, 27.4, 43.2, 39.2, 37.5, 32.8, 30.2, 42.3, 35.4, 30.5, 41.2, 34.6, 35.2, 36.7, 28.2, 35.2, 38.1, 38.2, and 40.1. We calculate the average, 35.1, as the final forecast.
6 One potential problem in is that it uses 2021–2023 forecasts from ChatGPT and human analysts, but only 2021–2022 data for realised performance. In unreported results, we exclude ChatGPT’s and human analysts’ forecasts for 2023 and find consistent patterns.
References
- Abarbanell, Jeffery S. 1991. “Do Analysts’ Earnings Forecasts Incorporate Information in Prior Stock Price Changes?” Journal of Accounting and Economics 14 (2): 147–165.
- Ackert, Lucy F., and George Athanassakos. 2003. “A Simultaneous Equations Analysis of Analysts’ Forecast Bias, Analyst Following, and Institutional Ownership.” Journal of Business Finance & Accounting 30 (7–8): 1017–1042.
- Adiwardana, Daniel, Minh-Thang Luong, David R. So, Jamie Hall, Noah Fiedel, Romal Thoppilan, Zi Yang, et al. 2020. “Towards a Human-like Open-domain Chatbot.” arXiv. https://arxiv.org/pdf/2001.09977
- Ali, Ashiq, April Klein, and James Rosenfeld. 1992. “Analysts’ Use of Information about Permanent and Transitory Earnings Components in Forecasting Annual EPS.” The Accounting Review 67 (1): 183–198.
- Alshater, Muneer. 2022. “Exploring the Role of Artificial Intelligence in Enhancing Academic Performance: A Case Study of ChatGPT.” https://doi.org/10.2139/ssrn.4312358
- Amir, Eli, Baruch Lev, and Theodore Sougiannis. 2003. “Do Financial Analysts Get Intangibles?” European Accounting Review 12 (4): 635–659.
- Aubry, Mathieu, Roman Kräussl, Gustavo Manso, and Christophe Spaenjers. 2023. “Biased Auctioneers.” The Journal of Finance 78 (2): 795–833.
- Bolliger, Guido. 2004. “The Characteristics of Individual Analysts’ Forecasts in Europe.” Journal of Banking & Finance 28 (9): 2283–2309.
- Boyacı, Tamer, Caner Canyakmaz, and Francis de Véricourt. 2023. “Human and Machine: The Impact of Machine Input on Decision Making under Cognitive Limitations.” Management Science. https://doi.org/10.1287/mnsc.2023.4744
- Cao, Sean, Wei Jiang, Baozhong Yang, and Alan L. Zhang. 2023. “How to Talk When a Machine Is Listening: Corporate Disclosure in the Age of AI.” The Review of Financial Studies 36 (9): 3603–3642.
- Cen, Ling, Gilles Hilary, and K. C. John Wei. 2013. “The Role of Anchoring Bias in the Equity Market: Evidence from Analysts’ Earnings Forecasts and Stock Returns.” Journal of Financial and Quantitative Analysis 48 (1): 47–76.
- Chen, Xi, Yang Ha (Tony) Cho, Yiwei Dou, and Baruch Lev. 2022. “Predicting Future Earnings Changes Using Machine Learning and Detailed Financial Data.” Journal of Accounting Research 60 (2): 467–515.
- Chen, Zihan, Lei Zheng, Cheng Lu, Jialu Yuan, and Di Zhu. 2023. “ChatGPT Informed Graph Neural Network for Stock Movement Prediction.” https://doi.org/10.2139/ssrn.4464002
- Coleman, Braiden, Kenneth Merkley, and Joseph Pacelli. 2022. “Human versus Machine: A Comparison of Robo-analyst and Traditional Research Analyst Investment Recommendations.” The Accounting Review 97 (5): 221–244.
- Das, Somnath, Carolyn B. Levine, and K. Sivaramakrishnan. 1998. “Earnings Predictability and Bias in Analysts’ Earnings Forecasts.” The Accounting Review 73 (2): 277–294.
- Dong, Rui, Raymond Fisman, Yongxiang Wang, and Nianhang Xu. 2021. “Air Pollution, Affect, and Forecasting Bias: Evidence from Chinese Financial Analysts.” Journal of Financial Economics 139 (3): 971–984.
- Dowling, Michael, and Brian Lucey. 2023. “ChatGPT for (Finance) Research: The Bananarama Conjecture.” Finance Research Letters 53 (May): 103662.
- Duru, Augustine, and David M. Reeb. 2002. “International Diversification and Analysts’ Forecast Accuracy and Bias.” The Accounting Review 77 (2): 415–433.
- Easterwood, John C., and Stacey R. Nutt. 1999. “Inefficiency in Analysts’ Earnings Forecasts: Systematic Misreaction or Systematic Optimism?” The Journal of Finance 54 (5): 1777–1797.
- Feng, Zifeng, Gangqing Hu, and Bingxin Li. 2023. “Unleashing the Power of ChatGPT in Finance Research: Opportunities and Challenges.” https://doi.org/10.2139/ssrn.4424979
- Hansen, Anne Lundgaard, and Sophia Kazinnik. 2023. “Can ChatGPT Decipher Fedspeak?” https://doi.org/10.2139/ssrn.4399406
- Harris, Richard D. F. 1999. “The Accuracy, Bias and Efficiency of Analysts’ Long Run Earnings Growth Forecasts.” Journal of Business Finance & Accounting 26 (5–6): 725–755.
- Hofert, Marius. 2023. “Assessing ChatGPT’s Proficiency in Quantitative Risk Management.” https://doi.org/10.2139/ssrn.4444104
- Hou, Yang, and Steven Li. 2014. “The Impact of the CSI 300 Stock Index Futures: Positive Feedback Trading and Autocorrelation of Stock Returns.” International Review of Economics & Finance 33: 319–337.
- Hovakimian, Armen, and Ekkachai Saenyasiri. 2010. “Conflicts of Interest and Analyst Behavior: Evidence from Recent Changes in Regulation.” Financial Analysts Journal 66 (4): 96–107.
- Huang, Wei, and Brian Wright. 2015. “Analyst Earnings Forecast under Complex Corporate Ownership in China.” Journal of International Financial Markets, Institutions and Money 35 (March): 69–84.
- Jha, Manish, Jialin Qian, Michael Weber, and Baozhong Yang. 2023. “ChatGPT and Corporate Policies.” https://doi.org/10.2139/ssrn.4521096
- Kim, Alex G., Maximilian Muhn, and Valeri V. Nikolaev. 2023. “Bloated Disclosures: Can ChatGPT Help Investors Process Information?” https://doi.org/10.2139/ssrn.4425527
- Ko, Hyungjin, and Jaewook Lee. 2023. “Can ChatGPT Improve Investment Decision? From a Portfolio Management Perspective.” https://doi.org/10.2139/ssrn.4390529
- Korinek, Anton. 2023. “Language Models and Cognitive Automation for Economic Research.” NBER Working Paper, No. w30957. National Bureau of Economic Research. https://doi.org/10.3386/w30957
- Lakkaraju, Kausik, Sai Krishna Revanth Vuruma, Vishal Pallagani, Bharath Muppasani, and Biplav Srivastava. 2023. “Can LLMs Be Good Financial Advisors? An Initial Study in Personal Decision Making for Optimized Outcomes.” arXiv. https://arxiv.org/pdf/2307.07422.pdf
- Lan, Yinyu, Yanru Wu, Wang Xu, Weiqiang Feng, and Youhao Zhang. 2023. “Chinese Fine-grained Financial Sentiment Analysis with Large Language Models.” arXiv. https://arxiv.org/pdf/2306.14096.pdf
- Li, Xianzhi, Xiaodan Zhu, Zhiqiang Ma, Xiaomo Liu, and Sameena Shah. 2023. “Are ChatGPT and GPT-4 General-purpose Solvers for Financial Text Analytics? An Examination on Several Typical Tasks.” arXiv. https://arxiv.org/pdf/2305.05862.pdf
- Liaudinskas, Karolis. 2022. “Human vs. Machine: Disposition Effect among Algorithmic and Human Day Traders.” Working Paper, No. 6/2022. Norges Bank, Oslo. https://www.econstor.eu/handle/10419/264948
- Lim, Terence. 2001. “Rationality and Analysts’ Forecast Bias.” The Journal of Finance 56 (1): 369–385.
- Liu, Miao. 2022. “Assessing Human Information Processing in Lending Decisions: A Machine Learning Approach.” Journal of Accounting Research 60 (2): 607–651.
- Liu, Sun. 2016. “Ownership Structure and Analysts’ Forecast Properties: A Study of Chinese Listed Firms.” Corporate Governance: The International Journal of Business in Society 16 (1): 54–78.
- Lopez-Lira, Alejandro, and Yuehua Tang. 2023. “Can ChatGPT Forecast Stock Price Movements? Return Predictability and Large Language Models.” https://doi.org/10.2139/ssrn.4412788
- Maskara, Pankaj K., and Donald J. Mullineaux. 2011. “Information Asymmetry and Self-selection Bias in Bank Loan Announcement Studies.” Journal of Financial Economics 101 (3): 684–694.
- Neilson, Ben. 2023. “Artificial Intelligence Authoring Financial Recommendations: Comparative Australian Evidence.” Journal of Financial Regulation: 9 (2): 249–257.
- Niszczota, Paweł, and Sami Abbas. 2023. “GPT Has Become Financially Literate: Insights from Financial Literacy Tests of GPT and a Preliminary Test of How People Use It as a Source of Advice.” Finance Research Letters 58 (December): 104333.
- Ren, Chen, Sang-Joon Lee, and Chenxi Hu. 2023. “Assessing the Efficacy of ChatGPT in Addressing Chinese Financial Conundrums: An In-depth Comparative Analysis of Human and AI-generated Responses.” Computers in Human Behavior: Artificial Humans 1 (2): 100007.
- So, Eric C. 2013. “A New Approach to Predicting Analyst Forecast Errors: Do Investors Overweight Analyst Forecasts?” Journal of Financial Economics 108 (3): 615–640.
- Tantri, Prasanna. 2021. “Fintech for the Poor: Financial Intermediation without Discrimination.” Review of Finance 25 (2): 561–593.
- van Binsbergen, Jules H., Xiao Han, and Alejandro Lopez-Lira. 2023. “Man versus Machine Learning: The Term Structure of Earnings Expectations and Conditional Biases.” The Review of Financial Studies 36 (6): 2361–2396.
- Wang, Zengzhi, Qiming Xie, Zixiang Ding, Yi Feng, and Rui Xia. 2023. “Is ChatGPT a Good Sentiment Analyzer? A Preliminary Study.” arXiv. https://arxiv.org/pdf/2304.04339.pdf
- Wei, Tian, Han Wu, and Gang Chu. 2023. “Is ChatGPT Competent? Heterogeneity in the Cognitive Schemas of Financial Auditors and Robots.” International Review of Economics & Finance 88: 1389–1396.
- Wenzlaff, Karsten, and Sebastian Spaeth. 2022. “Smarter than Humans? Validating How OpenAI’s ChatGPT Model Explains Crowdfunding, Alternative Finance and Community Finance.” https://doi.org/10.2139/ssrn.4302443
- Wu, Yanran, Tingting Liu, Liyan Han, and Libo Yin. 2018. “Optimistic Bias of Analysts’ Earnings Forecasts: Does Investor Sentiment Matter in China?” Pacific-Basin Finance Journal 49 (June): 147–163.
- Xie, Qianqian, Weiguang Han, Yanzhao Lai, Min Peng, and Jimin Huang. 2023. “The Wall Street Neophyte: A Zero-shot Analysis of ChatGPT over MultiModal Stock Movement Prediction Challenges.” arXiv. https://arxiv.org/pdf/2304.05351.pdf
- Yue, Thomas, David Au, Chi Chung Au, and Kwan Yuen Iu. 2023. “Democratizing Financial Knowledge with ChatGPT by OpenAI: Unleashing the Power of Technology.” https://doi.org/10.2139/ssrn.4346152
- Zhao, Zihao, Eric Wallace, Shi Feng, Dan Klein, and Sameer Singh. 2021. “Calibrate before Use: Improving Few-shot Performance of Language Models.” Proceedings of the 38th International Conference on Machine Learning 139: 12697–12706.