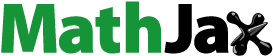
ABSTRACT
Lake water levels are an important indicator of water balance and water cycles, and are essential for climate and environmental change studies and water resource evaluation. Currently, lake level measurements are scarce or inconsistent throughout the country, and traditional gauge measurements of many lakes are not feasible, so satellite altimetry is a vital alternative to gauge lake levels. However, the accuracy and sampling frequency of lake level time series are usually low because of time and space coverage limitations; therefore, it is necessary to utilize multi-altimeter data to monitor lake levels and obtain lake level changes over long time series. In this study, we extracted the water level changes in 988 lakes (>10 km2) in China between 2002 and 2023 based on ICESat/-2, Cryosat-2, Jason-1/2/3, and Sentinel-3A/3B altimetry data using waveform retracking, lake level extraction, lake level time series construction, the fusion of multi-altimeter lake level time series, and outlier removal. A total of 55% of the lakes in this dataset have been monitored for more than 10 years, and 34% have more than 12 times the annual average water level monitoring. At the same time, in situ data from 21 lakes were used for validation, and the average root mean square error (RMSE) for each of the datasets of ICESat/-2, Cryosat-2, Jason-1/2/3, and Sentinel-3A/3B versus the in situ lake levels are 0.223 m, 0.163 m, 0.207 m, 0.596 m, 0.295 m, 0.275 m, 0.243 m, and 0.317 m, respectively, and the mean RMSE of the fused lake levels reaches 0.332 m. During the monitoring period, the water levels in Chinese lakes generally increased. The overall annual average rate of change at the 20 and 10-year scales was 0.123 m/a and 0.151 m/a, respectively, among which the overall water levels in large lakes increased significantly. The lakes with a faster rate of decline in the water level were primarily small. The water storage in each lake region in China shows an upward trend, with the most significant increase in the Tibetan Plateau region, where the average annual water level change rate has remained above 0.15 m/a over the past two decades. This dataset has high spatiotemporal coverage and accuracy and can support the estimation of changes in lake water storage, analysis of lake level trends, plateau flooding, and the relationship between lake ecosystems and water resources.
1. Introduction
Lakes are crucial carriers of water–heat exchange, a significant indicator of sensitivity to global and regional climate change responses, and critical parameters for basin water resources assessment and water balance analysis. At the same time, lakes are related to many aspects of human survival, social development, energy security, etc., and are essential to sustainable human development. Therefore, it is vital for scientific management and rational water resource utilization to address lake hydrological studies and monitor dynamic lake level changes (Gao et al., Citation2019; Zhu et al., Citation2020). However, the natural environment and maintenance costs limit the traditional lake level monitoring method, and it is challenging to deploy observation equipment in most areas, or in situ data cannot be publicly shared (Frappart et al., Citation2006; Gleick, Citation2003; Shu et al., Citation2020). Since the 1990s, satellite altimetry technology, which is characterized by high-coverage and multi-frequency, has been widely used for monitoring lake level changes (Birkett, Citation1998; Cooley et al., Citation2021; Cretaux et al., Citation2016; Liao et al., Citation2014; Song et al., Citation2015). At present, the altimeters aboard satellites such as Sentinel-3A/3B and ICESat-2 have shown significant improvement in observation accuracy, and the Cryosat-2 and Sentinel-3 satellite missions employing synthetic aperture radar (SAR) technology, capable of monitoring water body information with less along-track resolution and denser ground transit trajectories.
The current single altimeter type for lake level monitoring is often limited by the spatiotemporal coverage and accuracy of waveform processing, and the accuracy and monitoring frequency of the acquired lake level time series are usually low, which makes it challenging to meet the needs of water level change monitoring in many lakes on a national scale; therefore, multi-altimeter data are used to monitor lake levels. For example, water level changes were obtained by Gao et al. (Citation2013) for 51 lakes on the Tibetan Plateau from 2002 to 2012 upon fusing ENVISAT, Cryosat-2, and Jason-1/2 data and analyzing the driving effects of snow, ice, and permafrost melting. Song et al. (Citation2015) used Cryosat-2 and ICESat data to monitor water level changes in 131 lakes (>1 km2) on the Tibetan Plateau. Jiang et al. (Citation2017) used Cryosat-2 SARIn data and ICESat data to monitor water level changes in 70 lakes (>100 km2) on the Tibetan Plateau and analyzed the influence of climatic factors such as precipitation, evapotranspiration and other drivers. Li et al. (Citation2020) performed dynamic observations of lake levels in a long time series (2002–2017) in the middle and lower reaches of the Yangtze River by integrating ICEsat, ENVISAT, and Cryosat-2 data. Hwang et al. (Citation2019) obtained the water level changes in 61 lakes (>100 km2) on the Tibetan Plateau from 2003 to 2017 using Cryosat-2, SARAL, ICESat, and Jason-2 data at different temporal and spatial scales. Chen and Liao (Citation2020) fused Jason-2, Jason-3, CryoSat-2, and Sentinel-3A data to obtain the water level changes in 340 Chinese lakes (>10 km2) from 2016 to 2019, most of which showed an upward trend. However, the choice in retracking algorithm for multi-source radar altimetry data needs to be considered when performing water level inversion using multi-source radar altimeter data (Chen et al., Citation2021; Jain et al., Citation2015; Jiang et al., Citation2020), and the systematic bias between different altimetry data and retracking algorithms can affect the accuracy of lake level fusion to some extent.
China is a vast country with numerous lakes, whose formation and evolution show regional characteristics compatible with the natural environment. According to the distribution and hydrological characteristics of lakes, combined with geomorphological and climatic conditions, Chinese lakes can be classified into five major lake regions: Tibetan Plateau, Inner Mongolia–Xinjiang, Yunnan–Guizhou Plateau, Northeast Plain and Mountain, and Eastern Plain. Lakes in China are widely distributed but relatively concentrated. The Tibetan Plateau has the world’s largest group of high-altitude lakes, accounting for about 51.3% of the country’s total lake area, and has been an important area for studying of global climate change. The middle and lower reaches of the Yangtze River are full of lakes, accounting for about 24.1% of the country’s total lake area, which has great importance in China’s socio-economic development, so it is necessary to monitor the lakes in this region (Ma et al., Citation2011). Several laboratories implemented their own datasets at a global scale (Birkett et al., Citation2017; Crétaux et al., Citation2011; Schwatke et al., Citation2015; Tourian et al., Citation2022), such as GREALM, HydroSat, DAHITI, HYDROWEB. However, the above datasets hardly meet the demand for research on lake levels in China. For example, in China, HYDROWEB provides data on water level changes for only 64 lakes, and GREALM has less than 40 lakes. This study aims to improve the process of large-scale regional and long-time-series lake level monitoring based on multi-altimeter data and to analyze the spatial and temporal changes in lake levels in China between 2002 and 2023.
2. Materials and methodology
2.1. Data sources
2.1.1. Multi-altimeter data
In this study, eight satellite altimeter data types, including ICESat/-2, Cryosat-2, Jason-1/2/3, and Sentinel-3A/3B, were used to monitor the water level changes in 988 lakes in China between 2002 and 2023, and the corresponding altimeter data transit monitoring lakes are shown in . Jason-1/2/3 and Sentinel-3A/3B have low orbital density, large footprints, and fewer transits over lakes, but they have short revisit cycles and high monitoring frequency for the same lake. ICESat/-2 and Cryosat-2 have high orbital density, small footprints, and a large number of transits over lakes, but they have long revisit cycles. Among them, Cryosat-2 had the largest number of lakes in its transit path, reaching 733 lakes.
Table 1. Multi-altimeter data used in this study.
The orbits of the Jason series satellites change during operation. Specifically, when the Jason-2 satellite was launched in 2008, the Jason-1 satellite moved to a new orbit and operated until it was retired in 2013, with the Jason-2 satellite operating on the original orbit of Jason-1. Similarly, when the Jason-3 satellite was launched in 2016, the Jason-2 satellite moved to a new orbit. However, in July 2017, due to the degradation of key onboard components and control systems, the Jason-2 satellite left its new orbit and was maneuvered into a slightly lower orbit. Therefore, Jason-2 has operated in three different orbits, covering a large number of lakes. However, its lake transit corresponding monitoring time series is relatively short (Li et al., Citation2019).
2.1.2. In situ data
These in situ data are mainly obtained from the Water Level Yearbook of the People’s Republic of China, which contains single-day measured water level data from various hydrological stations, including Xiashe Station at Qinghai Lake, Kangshan and Duchang Stations at Poyang Lake, Dapukou Station at Tai Lake, Gaoyou High Station at Gaoyou Lake, Huailinzhen Station at Chao Lake, and Weishan Station at Weishan Lake. These in situ data for Namco and Zhari Namco were obtained from the Third Pole Environment Data Center (http://www.tpedatabase.cn/), which were obtained from manually placed water level meters. This study collected in situ water level data from 21 lakes to validate altimeter-obtained lake levels ().
Table 2. Details of in situ data for 21 lakes used for validation.
2.1.3. Lake mask data
In this study, the GLAKES dataset published by Pi et al. (Citation2022) was used as mask data for lake extent determination, which is the maximum extent of lakes obtained from the GSWO dataset (Pekel et al., Citation2016) with a deep learning classification algorithm in the period of 1984–2019, and also contains information on lake area, location, and contours. This study selected 1140 Chinese lakes from the dataset with an area greater than 10 km2.
2.2. Methodology
As shown in , the lake-level time series construction process in this study was divided into two parts: laser altimetry data and radar altimetry data, which mainly includes mass data preprocessing, single-day lake level extraction, lake level time series construction, fusion of multi-altimeter lake level time series and outlier removal. In these steps, the waveforms of radar altimetry data need to be retracked, and the altimetry errors are corrected to attain accurate water levels. In addition, this section describes the methodology for calculating the rate of lake level change.
2.2.1. Data preprocessing
Upon batch inputting these massive altimetry data from each satellite mission, Chinese transit data are screened and read according to the latitude and longitude information, from which parameters such as latitude, longitude, time, satellite orbital altitude, surface elevation value of the footprint, altimetry error correction value, and echo waveform are selected, and then screened to attain the transit dataset for each lake based on lake mask data.
2.2.2. Single-day lake level extraction and time series construction
For each observation day, a number of possible lake levels are obtained, considered as a “point cloud” of lake levels for a single day. Within this “point cloud” of lake levels at each observation site, this study determines the consistent value of lake levels for each observation site by finding the best lake level candidate.
2.2.2.1. Laser altimetry
For each lake water body, 10% to 90% of its single-day point-cloud was selected for single-day lake level extraction. When the number of single-day point-cloud of lake levels for a water body is less than three, the observation for that day was removed (Cooley et al., Citation2021). In addition, lake level observations with an orbital standard deviation greater than 0.25 m in the single-day point-cloud were removed. The median of the single-day point-cloud was selected as the single-day lake level, and the standard deviation of the single-day point-cloud was calculated.
The time series of lake levels were constructed upon screening lake level observations that differed by more than three standard deviations from all observation mean during the monitoring period and removing observations in the lake level series that fluctuated in a range that exceeded 40 m above the topographic elevation of the lake in the MERIT hydrologic dataset (Yamazaki et al., Citation2019), and those that exceeded the median of the lake level observations by 2.5 times the standard deviation.
2.2.2.2. Radar altimetry
The single-day lake level was extracted using the following equations (Chen & Liao, Citation2020),
where is the lake surface height,
is the satellite altitude,
is the distance between the altimeter and lake surface,
is the ionospheric correction,
is the solid earth tide,
is the wet troposphere,
is the dry troposphere,
is the retracking correction, and
is the geoid height with respect to the ellipsoid, for which the 2008 Earth Gravitational Model (EGM2008) (Pavlis et al., Citation2012). Except for
, all the above corrections are included in the altimetry data product, of which Jason-1/2/3 and Sentinel-3A/3B are L2-level data products and Cryosat-2 is an L1b-level data product.
Due to the large number of radar altimetry point-cloud of lake levels and the large fluctuation range of outliers, the nearest-neighbor linear interpolation method was first used to perform preliminary outlier removal for the single-day point-cloud, and then the outliers that are more than three times the standard deviation of the mean value of the single-day point-cloud were removed. The mean value of the single-day point-cloud was selected as the single-day lake level. The same-track standard deviation of the single-day lake level series was calculated. Observations with a same-track standard deviation of the single-day lake level series greater than 0.4 m were removed.
The time series of lake levels was constructed by screening lake level observations that differed from all observation means by more than three standard deviations during the monitoring period.
2.2.3. Waveform retracking
Since the lake mask data are the maximum extent of the lake from 1984 to 2019, there may be more noise in the waveform data caused by terrain reflection signals or non-nadir scattering signals. In this study, an automatic multiscale-based peak detection retracking algorithm (AMPDR) (Chen et al., Citation2021) was used, which consists of three steps: multiscale-based adaptive sub-waveform extraction, multiscale-based adaptive sub-waveform retracking calculation, and shortest-path algorithm searching for the optimal retracking point. The goal is to retrieve the leading edge of waveforms most likely to contain water surface reflection signals using a statistical method and obtain the stabilized lake level from land-contaminated multi-waveforms.
2.2.4. Fusion of multi-altimeter lake level time series
Due to the differences in different altimeters and changes in satellite orbits, there is a systematic bias among the lake level series extracted from multi-altimeter data. In this study, the systematic bias was eliminated by calculating the difference between the mean values of lake level series during overlapping periods with the formula as follows,
where is the initial lake level to be calibrated,
is the mean value of the base lake level in overlapping period, and
is the mean value of the initial lake level in the overlapping period.
In the lake level dataset from each satellite mission, Cryosat-2 measured the largest number of lakes and had the longest time series (), so it was used as the initial lake level series. Based on the accuracy of the lake level series in each satellite mission () and the time period of the lake level series overlap, the lake level datasets from ICESat-2, Sentinel-3A/3B, Jason-3/2/1, and ICESat were fused in order. Taking Zhari Namco as an example, as shown in , the accuracy of the lake level series for each satellite mission can be used as the basis of the fusion order to minimize the influence of lower-accuracy lake level series on the accuracy of fused lake level series.
Table 3. Comparison between the lake levels of satellite missions and in situ lake levels.
However, not all the multi-altimeter lake level time series can be successfully fused according to the above steps. In the case of Qinghai Lake, its fused lake level series is from 2015 to 2023, which has no overlapping period with the lake level series of ICESat (2003.09–2009.09) and Jason-1 (2009.12–2012.01), and therefore it cannot be fused successfully.
2.2.5. Rate of change in lake level estimation
In this study, we estimated the lake level trend by simplifying the cycle series fluctuation into 1-year and half-year cycles using the following equations (Gao et al., Citation2013).
where is the time in years,
is a constant term,
is the annual rate of lake level change (m/a), c, d are the coefficients of 1-year cycles, e, f are that of half-year cycles, and v is the fitting error. The above parameters were calculated using the nonlinear least squares method.
3. Data records
This dataset contains water level time series for 988 lakes in China between 2002 and 2023 and is saved in the xlsx format and named as “Chinalake_LakeID”, and LakeID is the unique identification ID for each lake in the GLAKES dataset (Pi et al., Citation2022). Each xlsx file contains three tables: contains the date of the satellite transit and the corresponding single-day lake level; contains the monitoring month and average water level for the month; contains the monitoring year and the average water level for the year. In addition, the data package contains a table of English and Chinese lake names corresponding to their LakeIDs, named “lake_information.xlsx”. Due to limited information about the lakes, some lakes in the table have incomplete English and Chinese names, with only numbers designations instead of names. Because of the difference in transit coverage by altimetry satellites, the lake level series obtained for 988 lakes do not all span the period of 2002–2023, and the time span for each lake is clearly shown in the dataset.
4. Technical validation
To verify the reliability of the lake level dataset, this study evaluated and validated it in terms of the frequency and accuracy of lake level observations. The accuracy was verified by comparing the lake levels from each satellite mission and the fused lake levels with in situ lake levels on the same day.
In the fused lake level dataset on Chinese lakes, there are 128 lakes with time series of more than 15 years, 420 lakes from 10 to 15 years, 350 lakes from 5 to 10 years, and 90 lakes with time series of less than 5 years, for a total of 988 lakes. Of these, 327 lakes have more than 12 average annual water level observations, 91 lakes have 6 to 12 average observations, and 570 lakes have less than 6 average annual water level observations.
4.1. Accuracy of lake level time series of satellite missions
In this study, as shown in , the number of validation points on the same day in both the verified lake level and in situ lake level is larger, and the coincidence period between these two is longer, which can ensure the reliability and accuracy of the verification results.
Among them, 79% of the accuracy verification results have an accuracy better than 0.3 m. The accuracy of some lake level sequences reaches the centimeter level, and the lake level time series of ICESat/-2, Cryosat-2, and Sentinel-3A have higher accuracy.
4.2. Accuracy of the fused lake level time series
Some large lakes in this study have lower accuracy validation results () because of their large lake extent, which can lead to lower lake level validation accuracy when the location of the measured hydrological station is farther away from the satellite’s footprints. Taking Kangshan Station of Poyang Lake as an example, the distance between this hydrological station and Jason-1’s bottom point is 102 km. From the point of view of satellite altimetry, there is a difference in the actual water level between these two locations in Poyang Lake, but the trend in both is basically the same. On the contrary, when the location of the measured hydrological station is closer to the satellite’s footprint, the lake level verification accuracy is enhanced (see ).
Figure 3. Comparison of fused lake level series and in situ lake levels (a) Qinghai lake, (b) Tai lake, (c) Gyaring lake, (d) Dongping lake.

Table 4. Comparison between the multi-source fusion lake levels and in situ lake levels.
In summary, the average RMSE values of lakes transited by ICESat/-2, Cryosat-2, Jason-1/2/3, and Sentinel-3A/3B were 0.223 m, 0.163 m, 0.207 m, 0.596 m, 0.295 m, 0.275 m, 0.243 m, and 0.317 m, respectively. The average RMSE of the fused lake level was 0.332 m. The P-value (Chen & Liao, Citation2020) is an appropriate referential indicator of uncertainty, which can reflect the confidence level of a lake level series (see and Appendix A). The accuracy validation results also show that the AMPDTR algorithm is adopted in multi-altimeter data fusion to monitor lake levels, which can obtain a stable time series.
5. Data analysis
In this study, the water level time series for 988 lakes in China between 2002 and 2023 were measured using a multi-altimeter, and the details of these lakes and their water level changes are shown in Appendix A. In order to accurately analyze the trend in water level changes in Chinese lakes, this study selected 528 lakes with more than 10 years of monitoring time series and more than one water level observation every half year for water level trend analysis in the fused lake level dataset. Among them, 78 lakes have been monitored for more than 20 years (2002–2023), and 450 lakes have been monitored for more than 10 years (2008–2023); there are differences in water level changes in Chinese lakes reflected by these two sets due to the difference in time scales; therefore, they are discussed separately in this study.
5.1. Trends for change in lake levels in China (2002–2023)
Over the past two decades, China’s overall lake level change has shown a clear upward trend, with an overall average annual rate of change (calculated according to the weighted average method, with the weight being the size of the lake region) of 0.123 m/a, and the lake area with rising water levels accounting for 76% of the overall lakes. The total lake area with a rising water level is 19,293 km2, and its rate of water level rise is 0.131 m/a, while the total lake area with a falling water level is 5891 km2, and its rate of water level falling is −0.008 m/a.
There are differences in the response of lakes at different magnitudes to the ecological environment and climate change, which need to be studied to deepen the understanding of lake level changes. This study analyzed the lake level change trend based on lake area grading. In general, since the beginning of this century, the water levels in Chinese lakes of all sizes have increased, and the average rate of increase is significantly higher than the average rate of decrease, which is most significant in large lakes, and the rate of decrease in water level is the fastest in small lakes ().
Table 5. The trends for changes in lake levels in China during 2002–2023.
shows the water level changes over 20 years in typical lakes at each size classification. A total of seven lakes with an area larger than 1000 km2 were monitored, of which five showed an increasing trend in water level, with an average increase rate of 0.182 m/a; two lakes showed a decreasing trend in water level, with an average decrease rate of −0.018 m/a. The lakes with the highest average annual increase and decrease in water level were Chibzhang Co (0.403 m/a) and Hongze Lake (−0.021 m/a), respectively. A total of six lakes with an area of 500–1000 km2 were monitored, of which five showed an increasing trend in water level, with an average increase rate of 0.123 m/a; the lake with a decreasing trend in water level was Gaoyou Lake (−0.0009 m/a). The lake with the highest average annual rate of rise in water level was Dogai Coring (0.224 m/a). A total of 24 lakes with an area of 100–500 km2 were monitored, of which 17 showed a rising trend in water level, with an average rising rate of 0.193 m/a; seven lakes showed a declining trend in water level, with an average declining rate of −0.044 m/a. The lakes with the highest annual average rate of rise and decline in water level were Yang Lake (0.657 m/a) and Xiashan Reservoir (−0.144 m/a), respectively. A total of 10 lakes with an area of 50–100 km2 were monitored, of which six showed a rising trend in water level, with an average rising rate of 0.045 m/a; four lakes showed a declining trend in water level, with an average declining rate of −0.030 m/a. The lakes with the highest annual average rate of increase and decrease in water level were Nanshan Lake (0.115 m/a) and Orpa Co (−0.046 m/a), respectively. A total of 31 lakes with an area of 10–50 km2 were monitored, of which 19 showed a rising trend in water level, with an average rising rate of 0.136 m/a; 12 lakes showed a declining trend in water level, with an average declining rate of −0.191 m/a. The lakes with the highest annual average rate of increase and decrease in water level were Long Lake (0.776 m/a) and Yanhaizi Lake (−0.717 m/a), respectively.
Figure 4. Lake level changes in China during 2002–2023 (a) >1000 km2; (b) 500–1000 km2; (c) 100–500 km2; (d) 50–100 km2; (e) 10–50 km2.

Overall (, ), after taking into account the lake area and the rate of lake level change, except for a slight decline in the overall lake level in the Eastern Plain, the lake level changes in various lake regions in China showed an upward trend, of which, the Tibetan Plateau lakes showed a significant increase in water level over 20 years. During the past 20 years, lakes with declining water levels in China were distributed in areas west of 90°E longitude and dominated by small lakes; the total lake area with declining water levels in the Eastern Plain was larger, amounting to 3370.2 km2.
Tibetan Plateau. A total of 31 lakes were monitored, of which 25 showed an upward trend in water level, with an average rate of increase of 0.217 m/a and a total area of 10,643.3 km2, concentrated in the inner plateau area. The remaining six lakes showed a downward trend in water level, with an average rate of decline of −0.004 m/a and a total area of 764.4 km2, primarily distributed in the western and southern parts of the inner plateau of the Tibetan Plateau. Over 20 years, the fastest rising lake was Yang Lake (0.657 m/a), the fastest declining lake was Langa Co (−0.139 m/a), and the largest monitored lake was Selin Co (1156.9 km2) with an annual average rate of change of 0.259 m/a.
Eastern Plain. A total of 16 lakes were monitored, of which eight showed an upward trend in water level, with an average rate of increase of 0.013 m/a and a total area of 827.1 km2. The remaining eight lakes showed a downward trend in water level, primarily distributed in the middle and lower reaches of the Yangtze River, with an average rate of decline of −0.018 m/a and a total area of 3370.2 km2. Over 20 years, the fastest rising lake in the lake region was Dalangdian Reservoir (0.193 m/a), and the fastest declining was Xiashan Reservoir (−0.144 m/a). The largest monitored lake was Hongze Lake (1678.3 km2), with an annual average rate of change of −0.021 m/a.
Inner Mongolia−Xinjiang. A total of 15 lakes were monitored, of which seven showed an upward trend in water level, with an average rate of increase of 0.048 m/a and a total area of 710.8 km2. The remaining eight lakes showed a downward trend in water level, with an average rate of decline of −0.097 m/a and a total area of 763 km2, primarily concentrated in the western part of the lake region, specifically, lakes in Xinjiang showed a downward trend in water level over twenty years. Over 20 years, the fastest rising lake in the lake region was Ayakkum Lake (0.364 m/a), and Yanhaizi Lake (−0.717 m/a) was the fastest declining lake. The largest monitored lake was Bosten Lake (1156.9 km2) with an annual average rate of change of −0.014 m/a.
Northeast Plain and Mountain. A total of 16 lakes were monitored, of which 12 showed an upward trend in water level, with an average rate of increase of 0.104 m/a and a total area of 1504 km2, primarily distributed in the western part of the lake region. The remaining four lakes showed a downward trend in water level, with an average rate of decline of −0.016 m/a and a total area of 500.1 km2. Over 20 years, the fastest rising lake in the lake region was Long Lake (0.776 m/a), and the fastest declining lake was Yangsha Lake (−0.210 m/a). Khanka Lake (4498.8 km2) was the largest monitored lake, with an annual average rate change of 0.051 m/a.
Figure 5. Spatial distribution of lake level change trends in China during 2002–2023. The lowercase letters indicate different lake regions. (a) Northeast plain and Mountain; (b) Eastern Plain; (c) Yunnan–Guizhou Plateau; (d) Inner Mongolia–Xinjiang; (e) Tibetan Plateau.

Table 6. The trends of changes in lake levels of different lake regions in China during 2002–2023.
5.2. Trends for changes in lake levels in China (2008–2023)
Over the recent decade, Chinese lake water reserves have continued to grow, with an overall average annual rate of change of 0.151 m/a. The total lake area with rising water levels accounts for 71% of the overall lake area, covering a total area of 26,049.8 km2 and a rate of water level rise of 0.169 m/a. The total lake area with falling water levels is 10,453.9 km2, with a rate of falling water level of −0.019 m/a. Compared with lake level changes over the past 20 years, the rate of rising water levels in China’s lakes has been faster in the past 10 years. The total lake area with rising water levels in various size classifications is much larger than lakes with falling water levels (). Large lakes showed a decline, but due to the small number in this classification category and the changes in water levels of large lakes over the past 20 years, the rise in large lake levels in China is still significant. The rate of decline in water levels is still the fastest for small lakes.
Table 7. The trends for changes in lake levels in China during 2008–2023.
shows the water level changes in typical lakes in each size classification over 10 years. A total of three lakes with an area larger than 1000 km2 were monitored; of these, Nam Co showed a rising trend in water level (0.006 m/a), while two lakes showed a decreasing trend in water level, with an average decrease rate of −0.022 m/a. The lake with the highest average annual water level decrease was Ebinur Lake (−0.040 m/a). A total of eight lakes with an area of 500–1000 km2 were monitored, of which seven showed a rising trend in water level, with an average rising rate of 0.285 m/a; the lake showing a declining trend in water level was Yamzho Yum Co (−0.026 m/a). The lake with the highest average annual rate of rise in water level was Aqqikkol Lake (0.613 m/a). A total of 79 lakes with an area of 100–500 km2 were monitored, of which 60 showed a rising trend in water level, with an average rising rate of 0.309 m/a; 19 lakes showed a declining trend in water level, with an average declining rate of −0.088 m/a. The lakes with the highest annual average rate of rise and decline in water level were Margai Caka (3.24 m/a) and Huolunuo’er (−0.653 m/a), respectively. A total of 79 lakes with an area of 50–100 km2 were monitored, of which 57 showed a rising trend in water level, with an average rising rate of 0.202 m/a, 22 lakes showed a declining trend in water level, with an average declining rate of −0.057 m/a. The lakes with the highest annual average rate of increase and decrease in water level were Xuemei Lake (0.611 m/a) and Qiafuqi Lake (−0.367 m/a), respectively. A total of 281 lakes with an area of 10–50 km2 were monitored, of which 192 showed an upward trend in water level, with an average rising rate of 0.158 m/a; 89 lakes showed a declining trend in water level, with an average rate of decrease of −0.107 m/a. The lakes with the highest rates of increase and decrease in the annual average water level were Hehua Lake (1.030 m/a) and Yangzong Lake (−0.758 m/a), respectively.
Figure 6. Lake level changes in China during 2008–2023 (the lowercase letters indicate five size classification showed as in ).

Overall (, ), the lake level changes in the Tibetan Plateau and Inner Mongolia–Xinjiang have shown an upward trend over the past 10 years. In the Tibetan Plateau, there are more lakes with declining water levels in the eastern and western parts of the inner plateau. In contrast, lakes with rising water levels are concentrated in the central part of the inner plateau. The lake level changes in Mongolia–Xinjiang are generally rising, but there is still a large proportion of lakes with declining water levels in this region. Considering that the number of lakes monitored in the Yunnan–Guizhou Plateau and the Northeast Plain is small, they are not discussed. It is worth noting that although human activities significantly influence the water level changes in lakes or reservoirs in the Eastern Plain, results show that a considerable proportion (1100.6 km2) of lakes in this region have rising water levels.
Tibetan Plateau. A total of 351 lakes were monitored, of which 264 showed an upward trend in water level, with an average rate of increase of 0.178 m/a and a total area of 22,205.8 km2. The remaining 87 lakes showed a downward trend in water level, with an average rate of decline of −0.016 m/a and a total area of 7791.9 km2, primarily distributed in the southern part of the inner plateau. Over 10 years, the fastest rising lake in this region was Margai Caka (3.235 m/a), and the fastest declining lake was Huolunuo’er (−0.652 m/a). The largest monitored lake was Nam Co (2016.9 km2), with an annual average rate of change of 0.006 m/a.
Eastern Plain. A total of 19 lakes were monitored, of which 15 showed an upward trend in water level, with an average rate of increase of 0.104 m/a and a total area of 1100.6 km2, concentrated in the southern part of this region, with the most significant being in the middle and lower reaches of the Yangtze River. The remaining four lakes showed a downward trend in water level, distributed in the northern part of this region, with an average rate decline of −0.009 m/a and a total area of 75.3 km2. Over 10 years, the fastest rising lake within this region was Meishan Reservoir (0.819 m/a), while Lake 10,340 declined the fastest (−0.557 m/a). The largest monitored lake was Zhelin Reservoir (235.9 km2), with an annual average rate change of 0.021 m/a.
Inner Mongolia−Xinjiang. A total of 75 lakes were monitored, of which 36 showed an upward trend in water level, with an average rate of increase of 0.148 m/a and a total area of 2453.1 km2, concentrated in Northwestern Xinjiang and its southernmost areas. In comparison, the remaining 39 lakes showed a downward trend in water level, with an average rate of decline of −0.035 m/a and a total area of 2530.2 km2, primarily concentrated in northern Xinjiang areas. Over 10 years, within this region, Bandao Lake rose at its fastest (0.785 m/a), while Bolakelakeli Lake declined at its fastest (−0.734 m/a). The largest monitored lake was Ebinur Lake (1042.7 km2) with an annual average rate change at −0.040 m/a.
Yunnan−Guizhou Plateau: A total of three lakes were monitored; two showed an upward trend in water level with an average rate of increase of at 0.007 m/a and a total area of 290.4 km2. The lake showing a downward trend was Yangzong Lake, with a rate of decline of −0.758 m/a and a total area for declining lakes at 30 km2. Over 10 years, Erhai Lake (241.1 km2) was monitored as having the largest area within this region with an annual average rate of change of 0.002 m/a.
Northeast Plain and Mountain: A total of two lakes were monitored; both showed a downward trend with an average rate of decline of −0.086 m/a and a total area for declining lakes at 26.4 km2. Yulong Lake declined the fastest over 10 years within this region (−0.126 m/a).
Figure 7. Spatial distribution of lake level change trends in China during 2008–2023 (the lowercase letters indicate the different lake regions shown in ).

Table 8. The trends for changes in lake levels of different lake regions in China during 2008–2023.
6. Conclusion
In this study, water levels in 988 Chinese lakes were obtained between 2002 and 2023 based on ICESat/-2, Cryosat-2, Jason-1/2/3, and Sentinel-3A/3B altimetry data through waveform retracking, lake level extraction, lake level time series construction, the fusion of multi-altimeter lake level time series, and outlier removal. This dataset includes 128 lakes with more than 15 years of monitoring time series, 420 lakes with 10 to 15 years of monitoring time series, 350 lakes with 5 to 10 years of monitoring time series, and 90 lakes with less than 5 years of monitoring time series. Of these, 327 lakes have more than 12 average annual water level measurements, 91 lakes have 6 to 12 average annual water level measurements, and 570 lakes have less than 6 average annual water level measurements. The average RMSEs for each of the satellite datasets from ICESat/-2, Cryosat-2, Jason-1/2/3, and Sentinel-3A/3B versus the in situ lake levels are 0.223 m, 0.163 m, 0.207 m, 0.596 m, 0.295 m, 0.275 m, 0.243 m, and 0.317 m, respectively. The average value of the RMSEs of fused lake level from multi-source satellites reaches 0.332 m, indicating that the present dataset has a high spatial and temporal coverage and accuracy.
In this study, the extracted multi-altimeter fused water level dataset was divided into two time scales of 10-year and 20-year to analyze the water level changes in Chinese lakes during the period of 2002–2023 from three perspectives: the overall changes in lake level, and the changes in each size classification and each lake region. In general, the water level changes in China’s lakes at the 20 and 10-year scales are consistent with an upward trend, and the overall annual average change rates are 0.123 m/a and 0.151 m/a, respectively. From the perspective of area grading, the overall water level in large lakes rises significantly, and the rate in small lakes is the fastest among the lakes with decreasing water level trends. Considering both lake area and lake level change rates, Chinese lakes’ water reserves show an upward trend, with the most significant increase in the Tibetan Plateau lakes. The average annual rate of change in water level over these two decades remains at 0.15 m/a or higher, primarily distributed in the central plateau. It is worth noting that a large lake area (3445.5 km2) of lakes in the Eastern Plain showed a decreasing trend in water level; in addition, a large number of lakes in the western and northern parts of Xinjiang in the Inner Mongolia–Xinjiang region showed a decreasing trend in water level over the monitoring period. The previous studies presented that the water levels of lakes in China showed a mean increasing trend of 0.343 m/a between 2016 and 2019. The annual lake level change rate increased from south to north and decreased from west to east in terms of spatial distribution (Chen & Liao, Citation2020). The changes in lake area, level, and volume over the Tibetan Plateau slightly decreased from 1976 to the mid-1990s, followed by a continuous rapid increase (Zhang et al., Citation2020). These studies are consistent with the results of this study, indicating our findings are credible and reasonable.
The dataset in this study contains the water level change series from 988 Chinese lakes between 2002 and 2003, filling the data gap on lake levels in China since the beginning of this century. This dataset can be used individually to analyze the trend in lake levels, short-term monitoring of lake overflows, plateau flood studies, etc. It can be combined with data on lake area changes to support the estimation of lake water storage changes and quantitatively describe the role of lakes in the river basin water cycle process.
Finally, it should be pointed out that this study uses the maximum lake extent to extract the water level, which may be disturbed by outliers. To further improve the accuracy of lake level extraction, it is recommended to use a more sophisticated method to detect and identify outliers in lake level time series. At the same time, in principle, satellite altimetry is used to monitor lake level changes through the height of the lake water surface, so the effect of uplifting the water surface by sediment accumulation at the bottom of lakes or reservoirs can overestimate the lake level. For example, in the Eastern Plain region, the lake reservoirs in this lake region are basically located in the middle and lower reaches of the basin, and sediment accumulation on the lake beds is more serious. Hence, the actual change in water storage in these lakes or reservoirs still needs further discussion. In the future, fully focused SAR (FFSAR) altimetry data will provide better results for higher precision lake level monitoring.
Appendix_1209.docx
Download MS Word (165 KB)Disclosure statement
No potential conflict of interest was reported by the author(s).
Data availability statement
The data that support the findings of this study are openly available in the Science Data Bank at https://doi.org/10.57760/sciencedb.10395
Supplementary material
Supplemental data for this article can be accessed online at https://doi.org/10.1080/20964471.2023.2295632.
Additional information
Funding
Notes on contributors

Shanmu Ma
Shanmu Ma received the B.S. and M.S. degrees at China University of Geosciences, Beijing, respectively in 2019 and 2022. Currently, he is working toward the Ph.D. degree in cartography and geography information system at the Key Laboratory of Digital Earth Science, Aerospace Information Research Institute, Chinese Academy of Sciences, Beijing, China. His research interests include satellite altimetry and hydrologic modeling.

Jingjuan Liao
Jingjuan Liao received the B.S. and M.S. degrees in geosciences from Nanjing University, respectively in 1987 and 1990, and the Ph.D. degree in geophysics from the Institute of Geophysics, Chinese Academy of Sciences in 1993. Since 1993, she has been working on radar remote sensing applications as a researcher at the Institute of Remote Sensing Applications, Chinese Academy of Sciences. She has rendered the institute great service in a number of research projects. Since 2007, she has been working on microwave remote-sensing application as a Professor with the Center for Earth Observation and Digital Earth and the Institute of Remote Sensing and Digital Earth, Chinese Academy of Sciences. She is currently working at the Key Laboratory of Digital Earth Science, Aerospace Information Research Institute, Chinese Academy of Sciences, Beijing, China. She has completed several research projects, and published more than 100 paper in relevant journals. Her current research interests include microwave scattering model, data processing, and surface parameters estimation.

Ruofan Jing
Ruofan Jing received his B.E. degree in Remote Sensing Science and Technology from China University of Geosciences, Wuhan, in 2023. Currently, he is pursuing the Ph.D. degree in cartography and geography information system at the Key Laboratory of Digital Earth Science, Aerospace Information Research Institute, Chinese Academy of Sciences, Beijing, China. His research interests primarily focus on the altimetry data for land applications, especially on the lakes and rivers.

Jiaming Chen
Jiaming Chen received his M.S. degree at the Aerospace Information Research Institute, Chinese Academy of Sciences, China, in 2021. Currently, he is pursuing the Ph.D. degree with the Institute of Geodesy and Geoinformation, University of Bonn. His research interests primarily focus on monitoring water resource using SAR altimetry, including river discharge and lake volume changes.
References
- Birkett, C. M. (1998). Contribution of the TOPEX NASA radar altimeter to the global monitoring of large rivers and wetlands. Water Resources Research, 34(5), 1223–1239. https://doi.org/10.1029/98WR00124
- Birkett, C. M., Ricko, M., Beckley, B. D., Yang, X., & Tetrault, R. L. (2017). G-REALM: A lake/reservoir monitoring tool for drought monitoring and water resources management. In AGU Fall Meeting Abstracts. https://agu.confex.com/agu/fm18/meetingapp.cgi/Paper/374138
- Chen, J. M., & Liao, J. J. (2020). Monitoring lake level changes in China using multi-altimeter data (2016–2019). Canadian Journal of Fisheries and Aquatic Sciences, 590, 590. https://doi.org/10.1016/j.jhydrol.2020.125544
- Chen, J. M., Liao, J. J., & Wang, C. (2021). Improved lake level estimation from radar altimeter using an automatic multiscale-based peak detection retracker. IEEE Journal of Selected Topics in Applied Earth Observations & Remote Sensing, 14, 1246–1259. https://doi.org/10.1109/JSTARS.2020.3035686
- Cooley, S. W., Ryan, J. C., & Smith, L. C. (2021). Human alteration of global surface water storage variability. Nature, 591(7848), 78–81. https://doi.org/10.1038/s41586-021-03262-3
- Cretaux, J. F., Abarca-Del-Rio, R., Berge-Nguyen, M., Arsen, A., Drolon, V., Clos, G., & Maisongrande, P. (2016). Lake volume monitoring from space. Surveys in Geophysics, 37(2), 269–305. https://doi.org/10.1007/s10712-016-9362-6
- Crétaux, J. F., Jelinski, W., Calmant, S., Kouraev, A., Vuglinski, V., Bergé-Nguyen, M., Gennero, M. C., Nino, F., Del Rio, R. A., Cazenave, A., & Maisongrande, P. (2011). SOLS: A lake database to monitor in the near real time water level and storage variations from remote sensing data. Advances in Space Research, 47(9), 1497–1507. https://doi.org/10.1016/j.asr.2011.01.004
- Frappart, F., Calmant, S., Cauhope, M., Seyler, F., & Cazenave, A. (2006). Preliminary results of ENVISAT RA-2-derived water levels validation over the Amazon basin. Remote Sensing of Environment, 100(2), 252–264. https://doi.org/10.1016/j.rse.2005.10.027
- Gao, L., Liao, J. J., & Shen, G. Z. (2013). Monitoring lake-level changes in the Qinghai–Tibetan Plateau using radar altimeter data (2002–2012). Journal of Applied Remote Sensing, 7(1), 073470. https://doi.org/10.1117/1.JRS.7.073470
- Gao, Q., Makhoul, E., Escorihuela, M. J., Zribi, M., Segui, P. Q., Garcia, P., & Roca, M. (2019). Analysis of retrackers’ performances and water level retrieval over the Ebro River basin using sentinel-3. Remote Sensing, 11(6), 11. https://doi.org/10.3390/rs11060718
- Gleick, P. H. (2003). Global freshwater resources: Soft-path solutions for the 21st century. Science, 302(5650), 1524–1528. https://doi.org/10.1126/science.1089967
- Hwang, C., Cheng, Y. S., Yang, W. H., Zhang, G. Q., Huang, Y. R., Shen, W. B., & Pan, Y. J. (2019). Lake level changes in the Tibetan Plateau from Cryosat-2, SARAL, ICESat, and Jason-2 altimeters. Terrestrial Atmospheric and Oceanic Sciences, 30(1), 33–50. https://doi.org/10.3319/TAO.2018.07.09.01
- Jain, M., Andersen, O. B., Dall, J., & Stenseng, L. (2015). Sea surface height determination in the Arctic using cryosat-2 SAR data from primary peak empirical retrackers. Advances in Space Research, 55(1), 40–50. https://doi.org/10.1016/j.asr.2014.09.006
- Jiang, L. G., Nielsen, K., Andersen, O. B., & Bauer-Gottwein, P. (2017). Monitoring recent lake level variations on the Tibetan Plateau using CryoSat-2 SARIn mode data. Canadian Journal of Fisheries and Aquatic Sciences, 544, 109–124. https://doi.org/10.1016/j.jhydrol.2016.11.024
- Jiang, L. G., Nielsen, K., Dinardo, S., Andersen, O. B., & Bauer-Gottwein, P. (2020). Evaluation of sentinel-3 SRAL SAR altimetry over Chinese rivers. Remote Sensing of Environment, 237, 237. https://doi.org/10.1016/j.rse.2019.111546
- Liao, J. J., Gao, L., & Wang, X. M. (2014). Numerical simulation and Forecasting of water level for Qinghai lake using multi-altimeter data between 2002 and 2012. IEEE Journal of Selected Topics in Applied Earth Observations & Remote Sensing, 7(2), 609–622. https://doi.org/10.1109/JSTARS.2013.2291516
- Li, P., Li, H., Chen, F., & Cai, X. B. (2020). Monitoring long-term lake level variations in middle and lower Yangtze basin over 2002–2017 through integration of multiple satellite altimetry datasets. Remote Sensing, 12(9), 1448. https://doi.org/10.3390/rs12091448
- Li, X. D., Long, D., Huang, Q., Han, P. F., Zhao, F. Y., & Wada, Y. (2019). High-temporal-resolution water level and storage change data sets for lakes on the Tibetan Plateau during 2000–2017 using multiple altimetric missions and Landsat-derived lake shoreline positions. Earth System Science Data, 11(4), 1603–1627. https://doi.org/10.5194/essd-11-1603-2019
- Ma, R. H., Yang, G. S., Duan, H. T., Jiang, J. H., Wang, S. M., Feng, X. Z., Li, A. N., Kong, F. X., Xue, B., Wu, J. L., & Li, S. J. (2011). China’s lakes at present: Number, area and spatial distribution. Science China-Earth Sciences, 54(2), 283–289. https://doi.org/10.1007/s11430-010-4052-6
- Pavlis, N. K., Holmes, S. A., Kenyon, S. C., & Factor, J. K. (2012). The development and evaluation of the earth gravitational model 2008 (EGM2008). Journal of Geophysical Research-Solid Earth, 117(B4), 117. https://doi.org/10.1029/2011JB008916
- Pekel, J. F., Cottam, A., Gorelick, N., & Belward, A. S. (2016). High-resolution mapping of global surface water and its long-term changes. Nature, 540(7633), 418–422. https://doi.org/10.1038/nature20584
- Pi, X. H., Luo, Q. Q., Feng, L., Xu, Y., Tang, J., Liang, X. Y., Ma, E. Z., Cheng, R., Fensholt, R., Brandt, M., Cai, X. B., Gibson, L., Liu, J. G., Zheng, C. M., Li, W. F., & Bryan, B. A. (2022). Mapping global lake dynamics reveals the emerging roles of small lakes. Nature Communications, 13(1). https://doi.org/10.1038/s41467-022-33239-3
- Schwatke, C., Dettmering, D., Bosch, W., & Seitz, F. (2015). DAHITI – an innovative approach for estimating water level time series over inland waters using multi-mission satellite altimetry. Hydrology and Earth System Sciences, 19(10), 4345–4364. https://doi.org/10.5194/hess-19-4345-2015
- Shu, S., Liu, H. X., Beck, R. A., Frappart, F., Korhonen, J., Xu, M., Yang, B., Hinkel, K. M., Huang, Y., & Yu, B. L. (2020). Analysis of sentinel-3 SAR altimetry waveform retracking algorithms for deriving temporally consistent water levels over ice-covered lakes. Remote Sensing of Environment, 239, 239. https://doi.org/10.1016/j.rse.2020.111643
- Song, C. Q., Ye, Q. H., Sheng, Y. W., & Gong, T. L. (2015). Combined ICESat and CryoSat-2 altimetry for accessing water level dynamics of Tibetan lakes over 2003–2014. Water, 7(12), 4685–4700. https://doi.org/10.3390/w7094685
- Tourian, M. J., Elmi, O., Shafaghi, Y., Behnia, S., Saemian, P., Schlesinger, R., & Sneeuw, N. (2022). HydroSat: Geometric quantities of the global water cycle from geodetic satellites. Earth System Science Data, 14(5), 2463–2486. https://doi.org/10.5194/essd-14-2463-2022
- Yamazaki, D., Ikeshima, D., Sosa, J., Bates, P. D., Allen, G. H., & Pavelsky, T. M. (2019). MERIT hydro: A high-resolution global hydrography map based on latest topography dataset. Water Resources Research, 55(6), 5053–5073. https://doi.org/10.1029/2019WR024873
- Zhang, G. Q., Yao, T. D., Xie, H. J., Yang, K., Zhu, L. P., Shum, C. K., Bolch, T., Yi, S., Allen, S., Jiang, L. G., Chen, W. F., & Ke, C. Q. (2020). Response of Tibetan Plateau lakes to climate change: Trends, patterns, and mechanisms. Earth Science Review, 208, 103269. https://doi.org/10.1016/j.earscirev.2020.103269
- Zhu, J. Y., Song, C. Q., Wang, J. D., & Ke, L. H. (2020). China’s inland water dynamics: The significance of water body types. Proceedings of the National Academy of Sciences of the United States of America, 117(25), 13876–13878. https://doi.org/10.1073/pnas.2005584117